1. Introduction
The increased reliance on big data and artificial intelligence (AI) for prediction and decision-making pervades almost all aspects of our existence.Footnote 1 Technology progress is transforming the financial sector worldwide and particularly credit markets in emerging countries, such as those found in Southeast Asia. This article focuses on algorithmic credit scoring, or “ACS”—a technology that combines big data and machine-learning algorithms to generate scorecards that reflect a loan applicant’s creditworthiness, defined as their likelihood and willingness to repay a loan. ACS is the tip of the spear of the global campaign for financial inclusion, which aims at including unbanked and underbanked citizens in financial markets and delivering them financial services, including credit, at fair and affordable prices.Footnote 2 While unbanked borrowers have no bank account or established credit history, underbanked borrowers may have a bank account but no credit history or an incomplete one. These borrowers are risky for credit institutions because of the latter’s lack of data to assess and the former’s creditworthiness. According to its advocates, ACS can solve this problem by modernizing traditional credit scoring, expanding data sources, and improving efficiency and speed in credit decisions.
However, ACS also raises serious public concerns about the dangers of opacity, unfair discrimination, and the loss of autonomy and privacy due to algorithmic governance and cybersecurity threats. Therefore regulators, credit providers, and consumers must understand its inner workings and legal limits to ensure that all parties make fair and accurate decisions. Whilst there is a long history of writings about ACS by authors from the Northern Hemisphere,Footnote 3 surprisingly little attention has been paid to regulating this technology in the Global South, especially in Southeast Asia.Footnote 4 This drawback is unfortunate as credit scores can significantly impact loan applicants’ lives at several financial, practical, and personal levels.Footnote 5 The problem stems from the fact that ACS is new and intertwines credit, privacy, and AI issues. It thus compels the regulator to work simultaneously on three fronts to propose a comprehensive legal approach addressing technical and ethical issues. This poses a challenge in emerging countries where the law is unstable and enforcement is weak.
The current article attempts to fill this information gap by using Vietnam as a case-study for the risks of engaging ACS without having adequate regulatory processes in place. Vietnam is leading Southeast Asia in terms of engagement with ACS, but other countries—such as Indonesia, Malaysia, and Thailand—are introducing ACS into their consumer finance market. Vietnam provides an excellent example because a leading consumer finance company, FE Credit, has significantly developed the market in a decade thanks to ACS and is pushing other credit institutions to follow its path. By utilizing Vietnam as a case-study for the development and regulation of ACS, we can provide helpful guidance to other countries that are starting to embrace these technological developments.
2. Using Vietnam as a case-study
Vietnam provides an interesting case-study to address the potential rewards and many risks of engaging with ACS and the consequent regulatory challenges. Consumer finance was virtually non-existent there ten years ago. It has grown considerably in the last decade, thanks to the efforts made by financial companies like FE Credit to attract tens of millions of unbanked borrowers in a country where more than half of the population is unbanked. Credit institutions have succeeded in developing the consumer finance market by providing a wide range of loans tailored to people’s everyday needs and by using sophisticated risk-management technologies, including ACS. But rapid ACS deployment carries potential risks for which the Vietnamese regulator is inadequately equipped: the credit law is poorly prepared to frame this technology and the myriad of new digital lending services that depend upon it; privacy law is piecemeal, under construction, and inadequately equipped to regulate extensive data mining and analytics; and AI regulations and ethical guidelines are still missing at this point.
2.1 Research question and contribution to the existing framework
In this article, we examine how emerging countries where ACS is deploying rapidly promote digital financial inclusion while protecting consumers from discrimination, privacy, and cybersecurity threats. Our research question considers how emerging Southeast Asian countries can most effectively engage with and regulate ACS to maximize the potential benefits of the technological developments whilst minimizing the many risks. We recognize the challenges in this research associated with cultural sensitivities and comparative legal developments.Footnote 6 Vietnam, though, is an excellent case-study as its ACS market has developed rapidly over the last ten years, allowing other countries to learn from its experiences. Our contribution is both empirical and theoretical. We provide an empirical case-study of ACS deployment in Vietnam based on qualitative interviews conducted in 2021 and 2022 with 22 bankers and finance specialists. The sample comprises two credit analysts, three staff from collection departments, three risks analysts, one director of a risk-management department, four loan appraisal officers, two credit support staff, one customer relations officer, one marketing manager, one branch manager, one transactional manager, and one senior banker. These individuals work for public banks such as BIDV, Vietcombank, and Petrolimex Bank; joint-stock banks such as PVCombank, Shinhan Bank, MB Bank, Maritime Bank, HDBank, and TPBank; and financial companies like FE Credit, Home Credit, Mcredit, Shinhan Finance, and FCCom. The sample also includes a senior officer from the Credit Department at the State Bank of Vietnam (SBV) and a deputy director of the Credit Information Centre, the national credit bureau under SBV supervision. Our research material also includes hundreds of news clips about banking and consumer finance issues in Vietnam, technical documents from the banking sector, and a broad range of laws, decrees, and regulations. Our theoretical contribution is a comprehensive legal framework that considers credit, privacy, and AI issues, and which could be accommodated in the heated debates about regulatory sandboxes and law revision in Vietnam. This framework draws inspiration from legal scholarship about traditional credit scoring and ACS. To provide concrete recommendations for steps forward, we engage with legal and ethical guidelines developed in several jurisdictions, considering what may be a fair and transparent ACS market in Southeast Asian countries embracing this technology for the first time.
2.2 Filling gaps in fintech in Asia, ACS regulation, and AI’s ethics
This article fills gaps in three literatures. First, it contributes to a small but growing body of work on financial technology (fintech) regulation in Asia. A recent edited volume entitled Regulating Fintech in Asia: Global Context, Local Perspectives shows how financial service providers, fintech start-ups, and regulators strive for innovation but must deal with uncertainty brought by technological progress and regulatory unpreparedness. Responses like policy experimentation and regulatory sandboxes do not always reassure stakeholders.Footnote 7 A chapter in this volume addresses fintech regulation in Vietnam. More than 150 fintech start-ups provide financial services, including digital payment, crowdfunding, peer-to-peer (P2P) lending, blockchain, personal finance management, and financial information comparators. To support their development, the Vietnamese government has set up a steering committee, designed a national financial inclusion strategy, and drafted a framework to operate regulatory sandboxes in five key domains.Footnote 8 In short, these discussions provide a broad context and policy recommendations to support fintech development, but leave ACS aside.Footnote 9 This article fills this gap by providing a case-study that highlights the technical, legal, and political challenges in regulating ACS.
Second, this article fills a gap in the literature on ACS regulation. In the US, federal laws ban discrimination in lending practices and grant borrowers the right to review their credit reports. However, they do not adequately render credit scoring transparent and unbiased, especially in the case of ACS, which derives scorings from digital data. When considering pervasive, opaque, and consequential automated scoring, several authors call for regulation that gives the regulator the right to test the scoring systems and gives consumers the right to challenge adverse decisions,Footnote 10 thus promoting accuracy, transparency, and fairnessFootnote 11 in ACS. In the UK, where borrowers are threatened by “the growing reliance on consumers’ personal data and behavioural profiling by lenders due to ACS, and coupled with the ineffectiveness of individualised rights and market-based mechanisms under existing data protection regulation,” Aggarwal calls for restricting the limits of personal data collection.Footnote 12 These works highlight the societal and political impacts of ACS in the Western world but leave aside those in emerging regions with radically different development trajectories and legal environments. This study fills this crucial gap by opening new lines of inquiry, prompting policy debate, and proposing legal revisions built on the rich findings from scholarship on ACS in the West.
Third, this article fills a gap in the vast literature on AI ethics and the legal regulation of these developments, particularly in the Global South. Significant and important scholarship has focused on the regulation of AI generally. This has included calling for operationalizing ethical principles into laws and policies,Footnote 13 developing new conceptual models for grasping legal disruption caused by AI,Footnote 14 and promoting transparency to tame unpredictability and uncontrollability,Footnote 15 as well as regulatory competition to reach an adequate balance between protection and innovation amidst technological uncertainty.Footnote 16 There has, however, been little research on ACS specifically. This is unfortunate, as these technological developments are inherently linked with financial exclusion and inclusion, which are vital to everyday consumers.
2.3 Structure of article
There are seven parts to this article. The current section outlines the background issues associated with ACS in Vietnam, including the impacts of big data and AI, the role of credit scoring, and the financial exclusion issues experienced by many people in the country. It also outlined the research question and how this article will contribute to the existing framework and literature. Technological developments offer many benefits and Section 3 analyses the potential rewards that can be gained from ACS, including modernizing the credit-scoring process, expanding potential data sources, making credit decisions more efficiently, and even addressing financial exclusion in the region. Section 4 then develops this discussion to address the potential risks of engaging more with ACS, including the risk of discrimination, potential invasions of privacy, the ability for mass surveillance, and cybersecurity threats. Section 5 applies the issues associated with ACS to Vietnam, especially to the challenges of financial inclusion, privacy, and cybersecurity threats. Section 6 consolidates the information from the previous sections and examines different international approaches to the regulation of ACS. In light of these approaches, the final Section 7 provides a potential framework for addressing these issues in Vietnam. We consider various jurisdictions and approaches to regulating ACS, including the US, the UK, the European Union, Singapore, and general International Guidelines. Despite the many potential downsides associated with this form of decision-making, it is clear that ACS is the way forward and will be utilized more and more by businesses wanting to make efficient and inexpensive lending decisions. It is therefore crucial to put a clear, transparent, and accessible legal regime in place to maximize the benefits of ACS whilst also ensuring an adequate level of protection for borrowers.
3. Potential rewards
This section highlights the multiple and significant benefits that can arise from a properly implemented and appropriately regulated ACS market. The benefits are inherently linked with financial exclusion. High levels of financial exclusion in many regions of the world, including Southeast Asia, have laid the groundwork for a global campaign to boost financial inclusion.Footnote 17 Financial inclusion is a top priority in the 2030 Sustainable Development Goals adopted by all UN Member States in 2015—a road map to foster peace and prosperity, eradicate poverty, and safeguard the planet.Footnote 18 The financial inclusion agenda strongly emphasizes availability of credit. According to its advocates, access to credit improves resource allocation, promotes consumption, and increases aggregate demand. In turn, a rise in demand boosts production, business activity, and income levels. Consumer credit thus enables households to cope with their daily spending; compensate for low wages that provide limited benefits and security; withstand economic shocks, especially in times of pandemic and depression; invest in production and human capital; and foster upward mobility. ACS is critical to enabling the expansion of credit markets, especially digital lending. This technology has the potential to address financial exclusion concerns in Vietnam and Southeast Asia more generally, based on the technical prospects of accuracy, efficiency, and speed in predicting credit risk.
3.1 Expanding potential data sources
Credit institutions and ACS fintech firms take an “all data is credit data” approach to achieve financial inclusion. The expression comes from Douglas Merrill, ZestFinance’s CEO, who stated in an article published in the New York Times that “[w]e feel like all data is credit data, we just don’t know how to use it yet.” Merrill highlights how virtually any piece of data about a person can potentially be analyzed to assess creditworthiness through extrapolation.Footnote 19 ACS fintech firms take advantage of the recent introduction of digital technologies and services, new electronic payment systems, and smart devices to collect alternative data that capitalize on high mobile phone and Internet penetration rates. These developments pave the way for ACS fintech firms that mine alternative data from borrowers’ mobile phones. These data are not directly relevant to their creditworthiness. Still, they generate detailed, up-to-date, multidimensional, and intimate knowledge about a borrower’s behaviour, especially their likelihood of repaying loans on time. Risk prediction is assessed through correlation, or the “tendency of individuals with similar characteristics to exhibit similar attitudes and opinions.”Footnote 20 For example, the predictive power of social network data is based on the principle of “who you know matters.” If connected borrowers act similarly, social network data should be able to predict their willingness to repay loans regardless of income and credit history. Borrowers’ personality and relationships become pivotal to assessing creditworthiness.Footnote 21
Another popular source of alternative data is telco data provided by mobile phone operators. Whilst this sounds controversial at best, many studies show the effectiveness of using mobile phone usage data,Footnote 22 credit-call records,Footnote 23 and airtime recharge dataFootnote 24 for credit-scoring purposes. These data offer glimpses of borrowers’ characteristics in terms of (1) financial capacities for repaying credit based on their income level, economic stability, and consumption pattern; (2) credit management abilities based on repayment behaviour and suspension management; (3) life pattern estimations based on residence stability, employment, and call networks; and (4) appetite for financial services.Footnote 25 In practice, prepaid top-up card phone users who make regular phone calls of a certain duration may indicate that they earn enough income to recharge their plan frequently and maintain their phone routines. The frequency with which they top up their phone card is another proxy for their income level. Lenders will trust top-up card users less than those who take post-paid plans with monthly payments, which indicates a better economic situation and financial stability. Another proxy, call location, provides insights into subscribers’ income level, job stability, job rotation, etc. If subscribers call from a residential area, their risk profile will be more credit favourable. In short, telco data provide 24-hour life insights about phone users that can be leveraged for risk prediction.
ACS fintech firms focus on accuracy to set themselves apart from credit registries and to promote their technology. Their predictions offer higher accuracy than traditional scoring by leveraging more data points relevant to risk behaviour and analyzing them with AI. ACS firms claim that the harvesting of this vast amount of alternative data significantly increases predictive accuracy. Peter Barcak, CEO of CredoLab, a Singaporean start-up that operates in Southeast Asia and beyond, highlights how ACS allows firms to move beyond the traditional one-dimensional borrowing history to review thousands of pieces of data, thereby providing a higher predictive power.Footnote 26 This difference impacts the Gini metric used by lenders to evaluate risk prediction accuracy. The Gini coefficient ranges between 0 and 1, indicating 50% and 100% accuracy rates, respectively. Credit institutions in Asia are accustomed to traditional credit-scoring systems with a 0.25–0.30 Gini coefficient or a 62–65% accuracy. However, CredoLab claims that its digital scorecards have a Gini coefficient of 0.40–0.50 or 70–75% accuracy. The 8–13% gain makes a difference for lenders, argues Barcak. For credit providers, it translates into a 20% increase in new customer approval, a 15% drop in loan defaults, and a 22% decrease in the fraud rate. It also reduces the time from scoring requests to credit decisions to a few seconds.
3.2 Modernizing credit scoring
ACS fintech firms make a strong argument for efficiency by comparing credit scoring based on traditional data and simple statistical tools with scoring based on alternative data and machine-learning algorithms. Numerous studies have compared predictive performances for traditional and machine-learning models. One of these studies highlights that (1) the machine-learning scoring model based on alternative data outperforms traditional scoring methods; (2) alternative data improve risk prediction accuracy; (3) machine-learning models are more accurate in predicting losses and defaults after a shock; (4) both models show efficiency as the length of the relationship between the bank and the customer increases, but traditional models do better in the long term.Footnote 27 Another study shows that the combination of e-mail usage, psychometric data, and demographic data measures creditworthiness with greater accuracy than a model based on demographic data alone, which is conventionally used to assess risk.Footnote 28
Traditional credit-scoring registries and bureaus primarily use past credit performance, loan payments, current income, and the amount of outstanding debt to profile borrowers. These data are fed to linear statistical protocols.Footnote 29 In many cases, appraisal and pricing depend on human discretion. In the US, the scoring model popularized by Fair, Isaac, and Corporation (FICO) relied until recently on three economic proxies that accounted for 80% of a single numeric score: consumers’ debt level, length of credit history, and regular and on-time payments.Footnote 30 However, these data are inherently limited. First, they do not render the economic profile of thin-file borrowers adequately. Second, they are not produced frequently enough to integrate the latest developments in borrowers’ lives. Third, they are not easily and quickly accessible at an affordable cost.Footnote 31 These limitations lower risk prediction accuracy, convenience, and utility for lenders. Another problem is that assumptions underlying traditional scoring models may be outdated. These models approach borrowers as workers with documented regular income and a linear and stable employment path. In post-Fordist countries, though, labour flexibility, neoliberal reforms, and austerity policies have given birth to the “precariat”—a new class of “denizens” who live in “tertiary time,” lack labour stability and economic security, and whose career paths are non-linear.Footnote 32 In emerging countries like Vietnam, the linear model bears little relevance to the lives of many precarious workers.Footnote 33 Only a minority of highly educated, middle-class workers build linear, future-oriented careers.Footnote 34 In brief, traditional scoring models show limitations in data sampling and credit risk modelling as opposed to the more efficient ACS models.
3.3 Faster credit scoring
Another argument advanced by its advocates is that ACS provides not only a faster mechanism for determining credit worthiness than traditional human-based decision-making, but also a “fast and easy credit” experience. The emphasis on shorter times for credit loan approval reflects another area in which ACS fintech firms outperform traditional scorers. Automated lending platforms that digitize the loan application process shorten the time for scoring, decision-making, approval, and disbursement to a few minutes.Footnote 35 The race for speed involves not only the collection of alternative data and their analysis by machine-learning algorithms, but the entire process of contacting a potential borrower, processing an application, generating a scorecard, making an appraisal, determining pricing, and disbursing the loan to a bank account. ACS also provides an “easy” credit experience by cutting down bureaucracy and human discretion, and facilitating the automation of lending processes. ACS also allows scorecards to be customized by modifying how credit risk is assessed for specific financial institutions and loan applicants. This process allows lenders to customize rating systems to best fit the unique risk characteristics of their company, partners, customers, and lending markets.
3.4 Findings of the section
ACS provides the potential to give increased financial options to people previously excluded from the credit market. When considering these issues, it is important to separate the promised or potential benefits from actual real-world advantages. ACS fintech firms promote their predictive technology as being more accurate, efficient, faster, and customizable than traditional models. However, there are currently no data showing that ACS fosters financial inclusion and consumer lending in emerging regions. Further empirical research is needed to ensure that the “possible” becomes the “actual.” Despite this lack of evidence, ACS has infiltrated lending markets in Western and emerging regions, and is likely to increase in importance and predominance. This technology does, however, carry several potential risks.
4. Potential risks
ACS makes big promises about financial inclusion, but simultaneously raises public concerns about the dangers of unfair discrimination and the loss of autonomy and privacy due to algorithmic governance and cybersecurity threats. These concerns emerge from the Western world, where AI is more advanced and embedded in people’s lives. In Southeast Asia, ACS fintech firms need to address some of these thorny matters, especially the cybersecurity issues. This section will map the potential risks of ACS and examine their relevance more generally.
4.1 Discrimination and biases
A common assumption is that big data and AI are superior to previous decision-making processes as technology is truthful, objective, and neutral. This optimistic view gives AI authority over humans to take on heavy responsibilities and make vital decisions. There is, however, increasing awareness and concern that algorithmic governance and decision-making may be biased and discriminate against historically vulnerable or protected groups. These groups include the poor, women, and minorities defined by race, ethnicity, religion, or sexual orientation.Footnote 36 The hidden nature of machine-learning algorithms exacerbates these concerns. Credit-scoring systems are designed to classify, rank, and discriminate against borrowers. To do this process via an algorithm creates a genuine concern that there may be unrecognized and unaddressed discriminatory processes. This is not just a theoretical concern, but one that we have already seen occurring with other similar financial processes. As outlined by Leong and Gardner, the UK regulatory authority reviewed the pricing practices of multiple insurance companies and was concerned that the data used in credit pricing models were based on flawed data sets that could contain factors implicitly or explicitly relating to the consumer’s race or ethnicity.Footnote 37 This is further supported by O’Neil, who highlights that nothing prevents credit-scoring companies from using addresses as a race proxy.Footnote 38 An algorithm trained to explicitly exclude forbidden characteristics such as race, gender, or ethnic origin may implicitly include them in the assessment by considering correlated proxies such as zip code, income, and school attendance.
Algorithms are popularly described as “black boxes” because they run autonomously, especially in unsupervised learning. The reason is that they sometimes operate without disclosing, even to their programmers, how they calculate the data sets or combinations of data sets used, which combinations of data sets are significant to predict outcomes, and how they achieve specific outcomes.Footnote 39 This is not, however, a completely accurate understanding of the process of algorithms, as human biases can easily be incorporated into machine learning. What we commonly refer to as an “algorithm” is made up of two parts. According to Kleinberg, the first is a “screener” that “takes the characteristics of an individual (a job applicant, potential borrower, criminal defendant, etc.) and reports back a prediction of that person’s outcome” that “feeds into a decision (hiring, loan, jail, etc.).” The second is a “trainer” that “produces the screening algorithm” and decides “which past cases to assemble to use in predicting outcomes for some set of current cases, which outcome to predict, and what candidate predictors to consider.”Footnote 40 In creditworthiness assessment, the trainer processes training data to establish which inputs to use and their weighting to optimize the output and generate the screener. In turn, the screener uses inputs and combines them in ways the trainer determines to produce the most accurate risk evaluation. Disparate treatment will arise if the screener includes variables like race and gender that are statistically informative to predict the outcome. Disparate impact will occur if the screener includes non-problematic proxies that will become problematic if they disadvantage vulnerable or protected groups excessively.Footnote 41
There are many possible ways for ACS to be infused with conscious or subconscious bias. For example, Petrasic highlights three potential sources in the development of the programme: (1) input bias—where the information included is imperfect; (2) training bias—which occurs in either the categorization or assessment of the information; and (3) programming bias that arises in the original design or modification of the process.Footnote 42 Other potential biases include an outcome basis, where the people using the algorithm utilize their own biases to determine an appropriate cause of action.Footnote 43 Unfair discrimination may also arise when algorithms consider factors stemming from past discrimination, which becomes a liability if it can be connected to disparate treatment or disparate impact. Lastly, an algorithm might also produce an unexpected imbalance that people may find unacceptable, such as gender disparity. It will then require assessment, even if the disparity does not concern the law.Footnote 44
4.2 Privacy and autonomy
ACS raises significant public concern about consumer autonomy and privacy. Autonomy refers to consumers’ ability to make choices and decisions free from outside influence. The use of ACS by consumer markets and jurisdictions to monitor and influence human behaviour is a double-edged sword. It can have a positive impact on assessing creditworthiness for the unbanked and underbanked, and foster financial inclusion. Conversely, it can also have detrimental effects on people’s lives, choices, and agency. The struggle for behaviour control is an uneven battle as scorers and lenders have diverse and powerful tools to impose it on borrowers. In contrast, borrowers have limited means to resist it. ACS helps financial markets determine borrowers’ inclination for financial services; monitor and shape their (repayment) behaviour through pricing, redlining and personalization; and collect up-to-date data about the risk that lenders can use to improve risk management. This, therefore, comes with a real risk of invasion of privacy and undermining of consumer autonomy.
Consumer lending markets use credit pricing and customization to discipline behaviour as well. In traditional credit scoring, the likelihood of repayment is based on assessing income and timely loan repayments from the past. This link between past and future behaviour makes it easy for borrowers whose loan application is rejected to review their credit records and improve their behaviour and future chances of approval. Furthermore, scorers and lenders can advise borrowers on how to improve their credit records and behaviour. This crucial level of transparency is missing in ACS. Scorers and lenders often cannot explain the motive for rejection and what data have proven significant in a particular decision. As a result, an advice industry is growing in the US to help people adjust their behaviour and improve their score and “life chances.”Footnote 45 Loan prices and conditions result from sorting and slotting people into “market categories” based on their economic performance.Footnote 46 Credit scores are “moving targets” that continuously change, based on borrowers’ endeavours.Footnote 47 They offer incentives for compliance (wider range of loans available, higher principals, lower prices, rates, and fees) and sanctions for failure (a small range of loans, lower principals, higher costs, rates, and fees).Footnote 48 This is a further example of the “poverty premium,” where the poor in society pay more for the same goods and services as their more financially stable counterparts.Footnote 49 Credit scores have become metrics to measure both creditworthiness and trustworthiness. A favourable credit score is too often a requirement to purchase a home, a car, a cellphone on contract, and to seek higher education, start a new business, and secure a job with a good employer. Conversely, bad scores hamper one’s “life chances.”Footnote 50 Credit scores greatly impact the individual’s quality of life. With ACS, however, individuals are often unable to determine what optimal behaviour they should develop to improve their “life chances.”
4.3 Mass surveillance
The previous concern was based on the impact that ACS can have at an individual level. There is, however, a related macro-level concern. ACS can provide a vehicle for mass surveillance systems designed to monitor and discipline human behaviour. An example is the social credit system currently tested in China.Footnote 51 This system uses traditional (public records) and non-traditional data to classify individuals, companies, social organizations, and institutions in red and black lists that carry rewards and sanctions, respectively. The concept of “social credit” (xinyong) blends creditworthiness and trustworthiness.Footnote 52 The system aims to improve governance and market stability, and foster a culture of trust as a bulwark against deviance, especially fraud, corruption, counterfeit, tax evasion, and crime.Footnote 53 At this early stage of development, a few dozen local governments implemented the programme with eight IT companies, including Tencent, Baidu, and Alibaba’s Ant Financial. Local governments are collecting traditional data from the Public Security Ministry, the Taxation Office, and legal financial institutes, whereas IT firms are collecting big data from their users, often through loyalty programmes. Their shared goal is to develop algorithms and procedures that can be centralized and applied nationally in the near future.Footnote 54
Alibaba’s Ant Financial runs a program that has generated considerable attention and controversy in the West: Sesame Credit (Zhima Credit in China). It leverages the vast database of Alipay, a payment app with over a billion users. It collects data through its built-in features to rank voluntary users based on “credit history, behaviour and preference, fulfilment capacity, identity characteristics, and social relationships.”Footnote 55 It rewards “trustworthy” users who act “responsibly” with high scores and privileges such as a fast-track security lane at Beijing Airport, fee waivers for car rental and hotel bookings, and higher limits for Huabei, Alipay’s virtual credit card. Low-score users may encounter difficulties in lending, renting, finding jobs, etc.Footnote 56 While social credit-scoring systems such as Sesame Credit generate deep anxiety about data mass surveillance in the West, Chinese citizens perceive it as an innocuous, trustworthy, and secure payment app that helps produce personal and social good.Footnote 57 For companies, those red-listed with good regulatory compliance history enjoy rewards such as “lower taxes, fast-tracked bureaucratic procedures, preferential consideration during government procurement bidding, and other perks” and those black-listed endure sanctions that include “punishments and restrictions by all state agencies.”Footnote 58 It should be noted that a few of the local governments testing the social credit system are sceptical about its capacity to develop a prosperous, trustworthy, and harmonious society, as claimed by the central government. Some of these governments even contest its legality and show resistance to its application.Footnote 59
4.4 Cybersecurity threats
A last primary concern about big data is shared worldwide: cybersecurity—the fraudulent use of data for profit. Cybersecurity issues include identity theft or new account fraud, synthetic identity fraud, and account takeover to make fraudulent purchases and claims. Fraudsters leverage networks, big data, and the dark web, and replicate good customer behaviour to game the system. The number of cyberattacks has been growing steadily with the expansion of digital banking, transactions, networks, and devices in circulation, and the decrease in face-to-face contact between customers and financial providers. A review of retail banks worldwide found that over half of respondents experienced increased fraud value and volume yet recovered less than a quarter of their losses.Footnote 60
ACS fintech firms take cybercrime seriously and heavily market their anti-fraud tools. Examples include face retrieval and customer identification solutions aimed at deterring fraudsters who impersonate customers using masks and digital images.Footnote 61 Furthermore, ACS fintech firms encrypt data from end to end when they share it with lenders and do not store them within their servers. By applying these safety measures, ACS fintech firms seek to reassure corporate customers and society of their safe services. However, they are still generating cyber risks by collecting, commodifying, and sharing big data with lenders and third parties. ACS fintech firms and credit institutions’ actions may therefore have unintended and far-reaching consequences for society and consumers, as shown by the persistent growth of cybercrime figures worldwide, and in Southeast Asia more specifically.Footnote 62
4.5 Findings of the section
The restrictions on in-person contact created by the COVID-19 pandemic have reinforced the incredible utility and benefits of technological advancements. Too often, however, the focus is unevenly on the many advantages of these steps forward, and the risks are not adequately considered or addressed. This section has therefore highlighted the many and significant risks posed by the use and expansion of ACS, including unfair discrimination and biases, the loss of autonomy and privacy, mass surveillance, and increased risk of cybersecurity threats.
5. ACS in Vietnam
The previous sections have presented the general benefits and potential risks of ACS. This section presents a case-study about ACS deployment in Vietnam against a background of rapid expansion of the consumer finance market.
5.1 Financial exclusion and the banking sector in Vietnam
There are significant concerns about financial exclusion in Vietnam and Southeast Asia more broadly, but reliable figures are difficult to access.Footnote 63 According to Bain & Company, Google, and Temasek,Footnote 64 70% of the region’s population is either unbanked or underbanked, totalling 458.5 million inhabitants out of 655 million. Yet this figure is not definitive, and it is difficult to characterize this group. Financial exclusion prevails in Vietnam—a country that experienced an economic collapse in the mid-1980s. This financial crisis led to macroeconomic reforms that put Vietnam on the path of a socialist-oriented market economy integrated into global exchanges. The economy and financial system have since developed immensely, helping Vietnam to emerge as a thriving lower-middle-income country with a gross domestic product (GDP) per capita that has risen more than tenfold in less than 35 years, from US$231 in 1985 to US$2,785 in 2020. While between 61% and 70% of 98 million Vietnamese still had no bank accounts a few years ago, two-thirds of them had bank accounts in 2022, according to the State Bank.Footnote 65 To boost financial inclusion even more, the government has recently approved the national inclusive finance strategy to include 80% of the population by 2025.Footnote 66
Financial exclusion in Vietnam results from a long-standing lack of trust in the banking system after years of warfare, political upheaval, changes in government, and bounding inflation.Footnote 67 In addition, banks are seen as an extension of the communist government, which is accused of opacity and corruption. Today, banks strive to show transparency and to change public attitudes, especially amongst young people. They also entice new customers by offering high but volatile rates for saving accounts.Footnote 68 However, the preference for cash and gold transactions remains strong.Footnote 69 To curb these problems, the government has approved a national inclusive finance strategy to raise the percentage of adults with bank accounts to 80% by 2025.Footnote 70 Meanwhile, high levels of financial exclusion remain a significant obstacle for credit institutions and bureaus to gain knowledge about tens of millions of consumers. ACS fintech firms and credit institutions assume that many of these individuals may seek loans and have the ability and willingness to repay them. This assumption lays the foundation for the development of consumer finance in Vietnam.
Consumer finance was virtually non-existent a decade ago. With an average annual growth rate of 20%, this sector has grown steadily to account for 20.5% of the total outstanding loans in the economy, which is 2.5 times higher than the figures in 2012. Yet, it only accounts for 8.7% of the total outstanding loans if housing loans are excluded, which is far behind Malaysia, Thailand, and Indonesia, where consumer finance (excluding mortgage) accounts for 15–35% of the total outstanding balance. This indicates that consumer finance still has room for growth in Vietnam.Footnote 71 The Vietnamese consumer finance market is segmented and comprises a broad mix of players: large state-owned banks such as BIDV, Vietcombank, and Vietinbank; smaller private joint-stock commercial banks including VPBank, PVCombank, and Sacombank; foreign banks such as ANZ, Citibank, and Shinhan Bank; and financial companies including FE Credit, Home Credit, HD Saison, and Mcredit, to name but a few. Vietnam is also home to two credit bureaus: the Credit Information Centre (CIC) gathering data from 30.8 million citizens, and the smaller Vietnam Credit Information Joint Stock Company (PCB).Footnote 72 Most banks provide housing, car, credit card, and some unsecured loans to low-risk customers with stable incomes. Conversely, financial companies such as FE Credit take a riskier approach and offer instalment plans, cash, and credit card loans to millions of low-income, unbanked, and “at-risk” consumers. Digital lenders, including peer-to-peer platforms, also provide microloans to consumers through easy-to-use apps. On the ground, consumers are bombarded with offers for (un)secured loans sent via SMS, emails, and social media.Footnote 73
5.2 ACS development in Vietnam
Lenders race to update their KYC or “Know Your Customer” and credit-scoring systems to break into the thriving consumer finance market. Financial companies require an identification document (ID) and household certificate to identify loan applicants, whereas banks require more paperwork such as income statements and bank-wired salary. However, not all applicants can provide proper documents, especially internal migrants.Footnote 74 In addition, applicants can have multiple IDs with different numbers. Lenders must then determine their creditworthiness. Scoring methods are not homogeneous across the industry. Credit institutions use different tools depending on their size, status, and risk appetite. Many banks use standard credit-scoring systems based on traditional statistical calculation and economic data, mainly labour and income data. Collecting and verifying labour and income data may also be challenging: some workers cannot provide records for their income, while others forge them.
However, financial companies and fintech start-ups are working to overturn this situation. They take advantage of the recent introduction of digital technologies and high Internet penetration rates to glean traditional and alternative consumer data to assess creditworthiness. In 2016, Vietnam’s Internet penetration rate had reached 52%, while smartphone ownership was 72% and 53% in urban and rural areas, respectively.Footnote 75 Furthermore, 132 million mobile devices were in circulation in 2017.Footnote 76 The leader in leveraging new technologies to assess risk is FE Credit, the consumer finance branch of VPBank. In 2010, FE Credit was the first credit institution to target risky yet lucrative segments ignored by banks and offer an array of financial products to the masses. Today it holds a consumer debt market share of 55%, with a total outstanding loan value of VND66 trillion in 2020. It possesses a database of 14 million customers, which is around 14% of Vietnam’s population. Many informants stressed FE Credit’s keen appetite for risky borrowers, aggressive marketing policy, and a lack of thorough risk assessment. In reality, FE Credit asserts its dominance by investing in a wide range of risk methods based on traditional and alternative data and machine-learning analytics. These methods combine multiple scores that help draw intimate “customer portraits” (chân dung khách hàng).
The first score is built in-house and based on demographic data (age, gender), identification documents (ID, household certificate), and labour and income data. Brokers provide additional data for verification—but not scoring—purposes. FE Credit checks taxable income to assess applicants’ declared salaries, social insurance data to determine whether applicants are self-employed or engaged in salaried labour or smaller trades, whether their phone number is registered to their ID, and their debt status with non-recognized credit providers such as P2P lending platforms that offer unregulated microloans. To gain more knowledge about customers, FE Credit also relies on vendor scores based on behavioural data provided by TrustingSocial, a fintech start-up based in Hanoi and Singapore. This firm gleans call/SMS metadata (when, where, and duration), top-up data, and value-added service transactions to determine borrowers’ income, mobility patterns, financial skills, consumption profile, social capital, and life habits.Footnote 77 Yet telco data may be inaccurate due to the high number of virtual subscriptions—people could buy SIM cards using pseudonyms for years, but nowadays cards are tied to subscribers’ names and ID numbers. FE Credit uses two other risk scores that are new to Vietnam. The first is a fraud score to detect suspicious behaviour and fraudulent orders based, for instance, on fake identification, which is a significant threat for financial companies (Vietnam Security Summit 2020). The second is a repayment score based on behavioural data about the customer’s interaction with the company. This score determines an optimal recovery strategy.Footnote 78 The use of vendor, fraud, and repayment scores puts FE Credit at the forefront of creditworthiness analysis in Vietnam, far above banks that use simple statistical tools.
To analyze data and generate scorecards, FE Credit uses machine-learning analytics. While many banks purchase models based on standard sets of variables from foreign providers like McKinsey, FE Credit has developed an in-house scoring model based on insights from its Vietnamese customers. Foreign models may assume that customers have a stable income, career paths, and verifiable documentsFootnote 79 —all variables that do not apply to FE Credit’s customers with low-income, informal, and unstable labour. Machine-learning technology allows adjustment of the inputs (variables) to maximize the outputs (scores). The most predictive variables inform selections and decisions for each case. Put differently, each customer’s scores result from a different set of maximized variables. The in-house score determines the interest rate, ranging from 20% to 60% per year, which also depends on the loans’ characteristics and package. It is key to drawing a customer’s portrait as it complements other data for appraisal, including the amount requested, down payment if relevant, the proportion of the loan relative to the price of the goods, the value of the debt contract, and so forth.
5.3 Improving financial inclusion through ACS
In Vietnam, ACS is at the early stage of deployment. Financial companies and some banks are increasingly using it to assess creditworthiness and make traditional and digital credit accessible to banked and un(der)banked borrowers. ACS is also challenging credit bureaus.Footnote 80 In Vietnam, the CIC under the State Bank is the official public credit registry. The CIC collects, processes, and stores credit data; analyses, monitors, and limits credit risk; scores and rates credit institutions and borrowers; and provides credit reports and other financial services. According to its website, the CIC gleans credit data from 670,000 companies and 30.8 million individuals with existing credit history and works with over 1,200 credit institutions, including 43 commercial banks, 51 foreign banks’ branches, over 1,100 people’s credit funds, and 27 finance and leasing firms. The CIC operates alongside PCB—a smaller private credit registry that 11 banks created in 2007. The PCB was granted a certificate of eligibility for credit information operation by the SBV in 2013. This registry delivers financial services to its clients, including credit reports.Footnote 81 Both the CIC and PCB provide essential services to the financial community. However, they use traditional credit data and scoring methods that limit their reach and scope. Moreover, they lack a national credit database system with an individual profile for every citizen based on the new 12-digit personal ID number.Footnote 82 They also use linear data that do not reflect unstable and multidirectional career paths, a norm in Vietnam. To enhance transparency and efficiency and reduce costs and waiting time, the CIC opened a portal to allow borrowers to track their credit data, prevent fraud, receive advice to improve their rating, and access credit packages from participating institutions.Footnote 83 On the whole, the CIC and PCB are poorly equipped today to compete with ACS fintech firms that pave the path to a lending ecosystem driven by big data and machine-learning algorithms. However, the advent of ACS is compelling credit bureaus and credit institutions to improve their data collection and scoring systems for competition purposes.
5.4 Privacy and mass surveillance
It is important to recognize the impact of ACS on consumers’ privacy rights, and the fact that these technological developments allow additional surveillance of people’s personal information. The ability for AI and big data to erode privacy has been an ongoing concern for many people in the Western world, and this issue has recently come under increased scrutiny. This concern is not, however, universal, and there are strong cultural and societal differences in the nexus and importance of privacy. In the context of Vietnam, Sharbaugh highlights that privacy fears are related almost solely to dangerous individuals obtaining data for criminal purposes. Citizens in Vietnam seem significantly less concerned about governmental or organizational scrutiny, and the ability to keep your personal data safe from these parties is not considered a fundamental right.Footnote 84
This cultural difference is clearly highlighted in the limited attention paid to ACS by the media and the public. This approach can be contrasted with the US, where the media and book industry take the initiative to educate borrowers on credit scoring and give advice on adjusting their behaviour to improve their scores and chances of obtaining loans.Footnote 85 A particular Vietnamese article is worth mentioning. In “Credit Score: An Important Factor in the Life of International Students in the US,” the journalist analyses the behaviour of Vietnamese students who seek to study in the US and borrow money to fund their studies and consumption.Footnote 86 He shares “the reasons why you should accumulate credit points and how to learn how to build credit effectively and safely while living in the US.” It also describes the factors affecting credit scoring and how to build the score by responsible credit card usage and “regularly moving money into your savings account [which] shows you take your finances seriously and are figuring out for the long-term future.” Even if it is too early to observe behaviour change among consumers due to the impact of credit scoring on their financial and personal lives, these materials reveal the gradual emergence of ACS and its normative power for guiding behaviour.
Another concern related to enhanced data collection is digital mass surveillance. Could Vietnam follow the Chinese social credit system? Administrative, cultural, and political structures, policies, and practices could lay the foundation. The social credit system in China aligns with the state’s long tradition of monitoring people and guiding their behaviour. It is also inspired by an old “public record” system (dang’an) that contains information on education; transcripts of results, qualifications, work approaches, political involvement and activities, awards and sanctions; and employment history.Footnote
87
Vietnam has its dang’an system called “personal resume” (). It facilitates expert classification of individuals and families and associated rewards and sanctions aimed at shaping citizens in a particular way. These data include one’s home address, ethnicity, religion, family background and composition, education and schools attended, language proficiency, occupation and qualification, salary level, date and place of admission to the Communist Party, social activity participation and rewards, and so forth. Local authorities collect these data where citizens have their household registration (hồ khẩu). The pending digitalization of the household registration system, which the “personal resume” system depends on, will make it easier for the government to collect and organize personal data.
This digitalization could also allow it to implement some form of social credit if the Vietnamese government decides to replicate the Chinese social credit system. Vietnam has a political regime similar to China’s. Like China, it has an authoritarian regime that restricts basic freedoms such as speech and protest, imprisons dissidents, and exerts strict control over the media. The Vietnamese government could deploy a social credit system to strengthen its grip over citizens and “nudge” their behaviour. The government subsidizes and collaborates with state-owned conglomerates such as FPT (formerly the Corporation for Financing and Promoting Technology), especially its AI division (FTP-AI), to unlock the power of AI and embrace the fourth industrial revolution. Like its Chinese counterpart, the Vietnamese government already uses AI to develop monitoring systems such as facial recognition to track citizens and human activity. In the near future, it could also co-opt big-data-based credit-scoring technology from FPT-AI and other Vietnamese fintech firms to test an experimental social credit system. At this stage, the government has not shown any interest in taking this path. It gains legitimacy by ensuring growth and political stability, and by supporting public companies. Replicating a look-alike Chinese social credit system to further control and suppress political freedom could tarnish Vietnam’s international image. Despite the severity of this possibility, as discussed in Section 2 above, credit-scoring start-ups have refrained from addressing issues of privacy and surveillance. Hence, this is a crucial risk that must be addressed by any regulatory framework developed.
5.5 Cybersecurity threats
Vietnam is a good case in point to reflect on ACS and cybersecurity risks, as new wealth breeds cybercrime. With rapid economic and digital growth and a growing and impressive number of Internet users (66% of 98 million inhabitants) and social media users (60%), Vietnam has been described as an “El Dorado for cyber-offenders.”Footnote 88 Cyberattacks and data breaches are widespread. In 2019, the Government Information Security Commission reported 332,029 access attacks (using improper means to access a user’s account or network) and 21,141 authentication attacks (using fake credentials to gain access to resources from a user).Footnote 89 Due to the absence of survey data on cybercrime, the only figures available are from law enforcement agencies, comprising only reported prosecutions and publicized cases. These cases form just the tip of the iceberg in a country where cybercrime remains largely underreported.Footnote 90 The Global Security Index compiled by the United Nations International Telecommunication Union ranked Vietnam 101st out of 195 countries in 2017. This is far behind its ASEAN peers; Thailand, Malaysia, and Singapore were ranked in the top 20.Footnote 91 In 2018, Vietnam made progress in the fight against cybercrime and rose to the 50th position. However, Vietnam still falls short of Singapore, Malaysia, Thailand, and Indonesia.Footnote 92
5.6 Findings of the section
This section has highlighted the practical and legal challenges posed by ACS in Vietnam on the basis of both existing literature and empirical research into the lived experiences of people in the country. Whilst we recognize that there will be legal, ethical, and cultural differences between various countries, the experiences in Vietnam with ACS can be used as an effective case-study to highlight the role of regulation in this area. The following section will develop on this discussion by considering what regulatory mechanisms are most suited for Vietnam and, by extension, other Southeast Asian countries that are starting to engage with ACS.
6. International regulation on ACS
As has been shown, ACS raises public concern about discrimination, the loss of individual privacy and autonomy, mass surveillance, and cybersecurity.Footnote 93 Regulation is critical to fostering innovation while safeguarding public interests. Western countries mitigate anxieties about ACS and meet the new challenges of the big data era by revising their credit laws, particularly the concepts of creditworthiness and discrimination.Footnote 94 They also develop ethical guidelines for responsible AI that sometimes lead to AI governance frameworks.Footnote 95 This section presents international regulatory measures on ACS and traditional credit scoring in the US, the UK, the European Union, Singapore, and general International Guidelines.
6.1 International regulatory approaches
6.1.1 United States
In the US, two laws regulate consumer risk assessment: the 1970 Fair Credit Reporting Act (FCRA) and the 1974 Equal Credit Opportunity Act (ECOA). The FCRA is a federal law governing the collection and reporting of consumer credit data. It aims to preserve fairness in credit scoring and reporting, ensuring that credit decisions are accurate and relevant. It also protects consumers’ privacy by limiting how data are collected, disclosed, kept, and shared. Furthermore, the FCRA grants consumers the right to access, review, and edit data on their credit scores, and to understand how lenders use their data for making financial decisions. However, the FCRA has several limitations when it comes to big data. First, it does not restrict the types of data that scorers and lenders can collect and use for scoring purposes. Second, the FCRA does not require credit bureaus to disclose their processing techniques for inspection to protect trade secrets. Third, the FCRA grants consumers the right to access credit data to check accuracy and dispute decisions. This right may, however, be impossible to exercise effectively with big data, as machine-learning algorithms process thousands of variables that cannot be disaggregated, and many times not identified. Overall, consumers are powerless against a law that throws them the burden of locating unfairness and having to challenge lenders’ credit decisions in court. The ECOA is the second law that regulates creditworthiness assessment. It prohibits discrimination against loan applicants based on proxies such as race, colour, religion, national origin, sex, marital status, age, and dependence on public aid. However, borrowers must again prove disparate treatment—a daunting task due to trade secrecy clauses and the opacity of machine-learning algorithms. In addition, lenders are free to inflict discrimination for business necessity, in which case the burden of proof shifts back to the discriminated. In short, the FCRA and ECOA give limited protection and recourse to borrowers while protecting scorers’ and lenders’ interests.Footnote 96
Hurley and Adebayo drafted a model Bill—the Fairness and Transparency in Credit Scoring Act (FaTCSA)—aiming to enhance transparency, accountability, and accuracy, and to limit biases and discrimination. It proposes that the regulator grant borrowers theright to inspect, correct, and dispute sources of data collected for scoring purposes; the data points and nature of the data collected; and credit scores that inform loan decisions. Consumers would acquire the rights to oversee the entire process, be provided with clear explanations on how the scoring process operates and motives for rejections, appeal rejections, and revise their credit data to improve their record.Footnote 97 The goal is to make all credit data, machine-learning technology, calculation, and decision-making processes open, accessible, and inspectable by the public, regulators including the Federal Trade Commission, and third parties through audits and grant licensing. Hurley and Adebayo also suggested shifting the burden of proof to scorers to ensure that they do not use predictive models and data sets that discriminate against vulnerable groups. They could adhere to “industry best practices to prevent discrimination based on sensitive variables or characteristics.” Understandably, this proposal could come under fire from industry players as they may object that transparency requirements could provide borrowers with critical knowledge to “game” credit-scoring models. Moreover, the disclosure of trade secrets could hamper innovation and competition in the industry. According to Hurley and Adebayo,Footnote 98 as well as Citron and Pasquale,Footnote 99 these risks are overridden by the urgent need for transparency, accountability, and limitation of bias and discrimination in a world in which AI is increasingly used to classify, rank, and govern societies. As argued by Aggarwal,Footnote 100 the goal for industry players from the fintech and finance sectors, the regulator, and society should be to negotiate normative trade-offs between efficiency and fairness, innovation, and public interest.
6.1.2 United Kingdom
In the UK, many of the risks of ACS are to an extent already anticipated and addressed by existing regulations. The most pertinent regulatory frameworks in this regard are the sectoral consumer credit regime supervised by the Financial Conduct Authority (FCA) and the cross-sectoral data protection regime led by the Information Commissioner’s Office (ICO). Other regulatory processes are, however, relevant, including the FCA and the Equality and Human Rights Commission, as well as being open to litigation by private individuals. Aggarwal highlights that the UK system of regulating ACS is heavily shaped by the European Union, namely the Consumer Credit Directive and the General Data Protection Regulation (GDPR).Footnote 101
The GDPR applies to all automated individual decision-making and profiling, and requires credit-scoring providers to (1) give individuals information about the processing of their information; (2) provide simple ways for them to request human intervention or challenge a decision; and (3) carry out regular checks to ensure systems are working as intended (Article 22). The basis for this approach is two key normative goals: allocative efficiency (allocating capital to the most valuable projects, minimizing consumer defaults) and distributional fairness (addressing societal inequalities). There is also a desire to ensure that the entire regime protects consumers’ privacy as much as possible.Footnote 102
Aggarwal, however, argues that the regulatory framework in the UK is not effective as it does not strike an appropriate balance between these three normative goals. Whilst privacy is said to be of significant importance, GDPR’s Article 22 under-regulates data protection and creates a privacy “gap” in the UK consumer credit market, particularly in terms of ACS.Footnote 103 In addition, standard regulatory approaches such as “informed consent” are not adequate for consumer safeguarding and further sector-specific monitoring is required.Footnote 104 Aggarwal calls for “substantive restrictions on the processing of (personal) data by credit providers, through legal as well as technical measures.”Footnote 105
As part of its approach to the regulation of ACS, the UK set up an advisory body, the Centre for Data Ethics and Innovation (CDEI), to provide independent research-driven guidance. Its role is to maximize the benefits of data-driven technologies for both society and the economy in the UK. In 2019, CDEI commissioned a Cabinet Office Open Innovation Team to undertake a Review of Algorithmic Bias. As part of this review, the CDEI confirmed that the current regulatory system was unreasonably opaque, and that the regulatory and policy responses were too slow. It therefore recommended that companies should sign up for voluntary codes of conduct to “bridge the gap” before formal legislation is enacted.Footnote 106
6.1.3 European Union
In early 2021, the European Commission proposed new rules to turn Europe into the global leader for trustworthy AI. It would provide the first-ever legal framework for AI and a Coordinated Plan to establish the concept of balancing the promotion of technology with protection for people—in an attempt to guarantee individuals’ safety, privacy, and rights. Importantly, this applies to all sectors, public and private.
This framework resulted in the Artificial Intelligence Act 2021 (AI Act). The AI Act provides a risk-based approach and divides different aspects of AI into three categories: “unacceptable risk,” “high-risk applications,” and activities that are neither unacceptable nor high-risk and are therefore largely left unregulated. “High-risk” systems under the AI Act include “AI systems intended to be used to evaluate the creditworthiness of natural persons or establish their credit score, except AI systems put into service by small scale providers for their use.” Credit scoring that denies people the opportunity to obtain a loan is therefore high-risk activity, and will be subject to strict obligations including risk assessment and mitigation systems, high quality of data sets to minimize the risk of discriminatory outcomes, logging of activity to ensure traceability of results, detailed documentation to assess its compliance, precise and adequate information for the user, adequate human oversight to minimize risk, and a high level of robustness, security, and accuracy.Footnote 107 These are all focused on increasing transparency of the decision-making processes—an ongoing issue with ACS discussed above.
The proposed rules are combined with a groundbreaking approach to penalties. Companies could be fined up to €30 million or 6% of their annual worldwide revenue (whichever is higher) for violations. There is a proposal AI Act, but it will need to be determined whether and how this will be implemented by the European Parliament and the Member States.
6.1.4 Singapore
The Monetary Authority of Singapore has been proactive in ensuring a responsible adoption of AI and data analytics in credit-scoring processes. It has developed a team of banks and industry players to develop metrics that can ensure the “fairness” of AI in these processes. This resulted in the Veritas initiative—a framework for financial institutions to promote the responsible adoption of AI and data analytics. Phase One of this initiative has been completed, and two White Papers have been published providing a detailed five-part methodology to assess the application of the fairness principles.Footnote 108 This requires businesses to reflect on the positive and negative impacts of the credit-scoring system, and ensure that human judgements are incorporated into the system boundaries. Phase Two is now underway, which looks into developing the ethics, accountability, and transparency processes.Footnote 109
Singapore has also been aware of and attempted to address the issues of AI and discrimination. The Singapore Model AI Governance Framework provides recommendations to limit unintended bias with models that use biased or inaccurate data or are trained with biased data. These propositions involve understanding the lineage of data; ensuring data quality; minimizing inherent bias; using different data sets for training, testing, and validation; and conducting periodic reviews and data set updates.Footnote 110
6.1.5 General international approaches
In 2019, the World Bank and the International Committee on Credit Scoring created Credit Scoring Approaches Guidelines to help regulators promote transparency with ACS. Several policy recommendations were made, including (1) a legal and ethical framework to govern and provide specific guidance to credit service providers; (2) a requirement for credit-scoring decisions to be explainable, transparent, and fair; (3) strengthening of data accountability practices; (4) credit-scoring models to be subject to a model governance framework; (5) collaboration and knowledge sharing to be encouraged; (6) the regulatory approach to strike a balance between innovation and risk; and (7) capacity-building of regulatory bodies and within credit service providers.Footnote 111
The general international approaches have been incorporated into various jurisdictions and approaches, as outlined in Table 1.
Table 1. Summary of international approaches
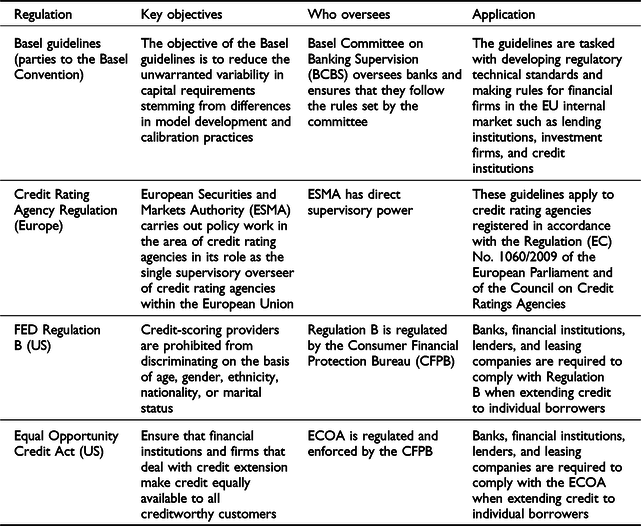
AI has become a priority for wealthy and transitional countries that position themselves as world and regional leaders in applying machine-learning algorithms to strategic sectors such as finance, health, education, transport, and services. To assuage the fears caused by the rapid growth of big data and AI, numerous governments and international organizations have set up ad hoc committees to draft ethical guidelines. Notable examples include the Council of Europe’s Ad Hoc Committee on AI, the OECD Expert Group on AI in Society, and the Singapore Advisory Council on the Ethical Use of AI and Data. These committees gather key actors from both the public and private sectors. In Singapore, the Advisory Council on the Ethical Use of AI and Data brings together representatives from the government; leading technology firms such as Google, Microsoft, and Alibaba; local firms using AI; and social and consumer interest advocates.Footnote 112 The private sector develops ethical guidelines as well, especially leading technology firms (Google, Microsoft, IBM, etc.), professional associations (Institute of Electric and Electronical Engineers, etc.), universities and research centres (Montreal University, Future of Humanity Institute, etc.), and NGOs (The Public Voice, Amnesty International, etc.). A recent review of the global landscape of AI ethical guidelines published in Nature Machine Intelligence identified 84 documents published worldwide in the past five years.Footnote 113 Although these ethical principles are not legally binding regulations, they carry advantages as they steer the private sector towards behaving in the desired way, and are destined to become regulations in the future.
6.2 Findings of the section
This section has highlighted the many different approaches that exist for regulating ACS. Each of the systems analyzed have different strengths and weaknesses, as well as specific cultural and societal backgrounds. The next section will build on this framework and, in light of the empirical research undertaken on ACS in Vietnam, provide a proposal for effective regulation in the country.
7. A proposal for regulating ACS in Vietnam
This part provides a proposal to regulate ACS in Vietnam based on recommendations from legal scholars and international regulatory approaches to this technology. There has been research on how to regulate many other areas related to AI,Footnote 114 but little focus on ACS—despite the significant potential impact on individuals’ financial options. The gap is particularly noticeable in developing and transitional regions. In Vietnam, the law is ill-equipped to regulate ACS. Whilst there are limitations to the role of regulation in such a technology-driven and fast-moving area as AI and ACS,Footnote 115 it is clear that operating in legal limbo is far too risky for consumers, businesses, and countries.
7.1 Current regulatory approaches in Vietnam
Except for Singapore, most Southeast Asian countries, including Vietnam, lag in the regulation of AI and particularly ACS. The lending industry deploys this technology without any regulatory framework, which is a source of concern. Before international approaches and recommended regulatory reforms can be considered, it is vital to address the current laws in place in Vietnam. This section highlights what protection is and is not available to people in terms of creditworthiness assessment, data collection privacy, and discrimination.
7.1.1 Assessing creditworthiness
ACS raises new regulatory challenges, such as how to address the concept of creditworthiness in credit law. In Vietnam, credit law is scattered across several legal instruments, including the 2010 Law on Credit Institutions as the general framework, Circular 39/2016/TT (on lending transactions of credit institutions and/or foreign banks), Circular 43/2016/TT (on stipulating consumer lending by financial companies), and Circular 02/2013/TT (on conditions of debt restructuring).
Circular 39/2016/TT requires credit institutions to request customers to provide documents that prove their “financial capability” to repay their debt (Articles 7, 9, 12, 13, 17, 31). “Financial capability” is defined as the “capacity with respect to capital, asset or financial resources” (Article 2). If a credit institution rejects a loan application, the customer must know why (Article 17). Credit institutions must also request customers to report the loans’ intended use and prove that loans are used legally and adequately (Article 7). For this purpose, the institution may inspect and supervise the consumer’s use of the loan (Article 94 of the 2010 Law on Credit Institutions, Article 10 of Circular 43/2016/TT). Circular 02/2013/TT regulates the collection of personal data for generating internal risk rating systems used to rate customers and classify loans based on risk. It specifies that customers’ risk assessment rests on financial qualitative and quantitative data, business and administration situation, prestige, and data provided by the CIC. Credit institutions must update internal risk ratings and submit them regularly to the SBV in charge of CIC to keep the CIC database up to date. The impact of these laws is that creditors and regulators must continually assess and categorize debtors and loans into risk categories.
These regulations on creditworthiness assessment were designed before the current growth of credit markets and the deployment of big data and AI in Vietnam. They therefore contain gaps and inadequacies. First, lenders must assess borrowers’ creditworthiness using traditional, financial, and (non)credit data, which they collect from borrowers and the CIC. This framework is inadequate to regulate the collection and processing of alternative data by ACS fintech firms. As shown, alternative data raise new challenges because of their nature. Examples of issues include their collection, use, transfer, and storage. Data property rights of the scorers, lenders, and third parties are unclear. At the same time, the relevance of the data and accuracy and fairness in human and automated credit decisions are also called into question. By leaving these issues unregulated, the legislator puts the lending industry and borrowers at risk.Footnote 116 Second, credit regulation requires credit institutions to give borrowers a reason for rejecting loan applications. The law does not, however, grant borrowers the right to correct credit data, appeal against human or automated decisions, request explanations on how decisions are made, or receive suggestions on improving their credit records and scores to avoid future rejections. Overall, legal provisions on creditworthiness assessment leave lenders unaccountable for their decisions and add an extra layer of opacity to credit scoring and decision-making, thereby disadvantaging borrowers.
7.1.2 Privacy and data collection
The state of Vietnamese law complicates the enforcement of privacy in data collection. There is no separate, comprehensive, and consistent law on data privacy. Instead, there are a number of conflicting, overlapping, inadequate, and unfit laws and regulations in the form of general principles relevant to this issue. They include Law 86/2015/QH13 on Cyber-Information Security (CIS), the Law 67/2006/QH11 on Information Technology (IT), the Law 51/2005/QH11 on E-transactions, the Decree 52/2013/ND-CP on E-commerce, and the Law 59/2010/QH12 on the protection of consumers’ rights.Footnote 117 In case of violation of personal data privacy, the current penalties of this body of laws and regulations are not deterrent enough.
The CIS defines “personal data” as “information associated with the identification of a specific person” (Article 3.15) and the “processing of personal data” as collecting, editing, utilizing, storing, providing, sharing, or spreading personal information (Article 3.17). The CIS and IT require data collecting companies to obtain consent from data owners prior to personal data collection, including highlighting the information’s scope, purpose, and use (Articles 17a, 21.2a). However, some exceptions apply. Under Decree 52/2013/ND-CP, e-commerce businesses are not required to obtain data owners’ consent when data are collected to “sign or perform the contract of sale and purchase of goods and services” (Article 70.4b) and “to calculate the price and charge of use of information, products and services on the network environment” (Article 70.4c). If lenders operate under e-commerce licences, collecting personal data without data owners’ consent is allowed to assess creditworthiness and determine loan pricing.
Personal data protection regulation grants rights to data owners, including the right to “update, alter or cancel their personal information collected or stored” by companies and to stop these companies from “providing such personal information to a third party” (CIS, Article 18.1). It also requires data owners to delete this information once it has been used or storage time has expired (CIS, Article 18.3). Article 22 of the CIS and IT give data owners the right to inspect, correct, or cancel the information (see also Article 22 of the IT). In addition, the CIS (Article 18.1) and IT (Article 22.1) prohibit companies from sharing personal information with third parties unless express permission is granted. The CIS also requires companies to delete the stored personal data once they have fulfilled their purpose or the storage time has expired, and notify the user about this operation (Article 18.3). Overall, the law provides data owners with a comprehensive set of rights and protections against companies that collect personal data, and promotes transparency and accountability.
7.1.3 Discrimination
Credit laws in Vietnam do not ban discrimination, especially disparate treatment and disparate impact against vulnerable borrowers through sensitive proxies like race, religion, national origin, sex, marital status, etc. Vietnam has no specific law about discrimination in general or credit discrimination in particular. Article 16 of the Constitution states: “All citizens are equal before the law. No one shall be discriminated against based on their political, civic, economic, cultural or social life.” Discrimination is also evoked in the Law on Gender Equality, which is primarily limited to employment issues. Consumer finance has recently increased sharply in Vietnam, and this has created a lack of regulation addressing credit discrimination.
The fundamental notion of discrimination in credit law is highly relevant as traditional and credit markets rapidly expand in Vietnam. Today, new credit markets target working and middle-class borrowers in urban centres, especially Ho Chi Minh City and Hanoi. Consumer lending remains relatively inaccessible in rural areas, where some 60 million inhabitants live, the majority of the Vietnamese population. However, as suggested by several legal scholars, adopting an input-centred approach that would prohibit ACS models from using sensitive characteristics like class, gender, and ethnicity is technically challenging, if not impossible. This is because algorithms may use correlated proxies such as zip code, income, education, phone use patterns, and other factors to enhance predictive accuracy in the assessment of creditworthiness.Footnote 118
7.2 Harnessing the potential: regulatory recommendations for Vietnam
This final section develops the analysis of the current regulation in Vietnam and the different international approaches to make three critical regulatory recommendations—amending the creditworthiness requirements, enforcing transparency and privacy in data collection, and creating AI Ethical Principles Guidelines.
7.2.1 Amending creditworthiness requirements
How should credit regulation be amended to enhance customers’ rights and protection with the onset of the digital credit revolution in Vietnam? Credit law in the country currently overlooks transparency and discrimination. The regulator should issue a decree to enshrine the above principles, updating the 2010 Law on Credit Institutions and circulars 39/2016/TT, 43/2016/TT, and 02/2013/TT. This decree could limit the type of data collected; how they will be used; the circumstances under which they can be transferred, stored, and sold; and whether they should be shared with the CIC. The decree could also ensure that consumers are given the possibility to oversee the entire scoring process and have the right to explanation in the case of rejection, appeal against rejection, and correct incorrect credit data to improve their chances of approval in the future. It could also detail safeguards and procedures on opening the scoring system for inspection by a public regulatory body—for instance, the SBV—and for audits by private and external actors. The decree could also address the fundamental issue of bias and discrimination, particularly the risk of disparate impact and unequal treatment of vulnerable groups. Such groups include the urban and rural poor, and ethnic and religious minorities excluded from credit markets since the launch of macroeconomic reforms in the early 1990s. The decree could ban the use of sensitive characteristics such as race, colour, religion, national origin, sex, marital status, age, dependence on public aid, and other proxies absent from the ECOA that could be relevant to Vietnam. The regulator could design this decree and negotiate optimal normative trade-offs between efficiency and fairness with industry players and civil society organizations.
7.2.2 Enforcing transparency and privacy in data collection
As we have seen, big data and AI raise public concerns over privacy, transparency, and accountability. These concerns are central to the regulation of personal data protection in Vietnam. Whilst—as discussed above—the country does have significant regulation in this area, it does not delimit what data companies can collect to protect privacy. This gap is problematic with regard to ACS, given the severe implications big data and AI could have in people’s lives. This debate is relevant to Vietnam. The government could begin by consulting with industry players, international bodies, data protection organizations, and civil society organizations to determine what personal data should or should not be collected and commodified for credit-scoring purposes. The goal would be to balance risk assessment and credit decision-making efficiency while preserving data owners’ privacy, intrinsic identities, and vital interests. The results from this consultation could be enforceable by issuing a decree that would amend the CIS, IT, and other privacy regulations. Meanwhile, the authorities should ensure that ACS fintech firms and local and international lenders operating in Vietnam follow the law on personal data protection to respect privacy. In particular, companies should always obtain consent from data owners before collection; grant them the right to update, alter, or cancel credit data; delete the data once they have been used for risk prediction; and inform them when data are transferred to third parties. These principles overlap with those described in the proposed decree to amend credit law. The regulator, industry players, and society could bring together their goals and policies, and negotiate a normative trade-off.
Aggarwal goes a step further and proposes a ban on collecting and processing certain types of personal data, such as relationships, health, and social media data, as these are intrinsic to a consumer’s identity and autonomy.Footnote 119 This suggestion raises a challenging question considering that privacy is not a universal concept, but rather one that is historically, socially, and politically situated. The question is how to determine what data are intrinsic—and therefore non-commodifiable—for preserving data owners’ privacy and intrinsic identities. In a similar vein, the World Bank and the Consultative Group to Assist the Poor (CGAP)Footnote 120 have called for “data minimisation” to protect the “vital interests of data subjects” and to limit the collection of data to what is strictly necessary for creditworthiness assessment. The task of determining the relevant data for this purpose would fall on the regulator. This proposal leads to Aggarwal’s conundrum: how to decide what data can be collected and commodified to reach normative trade-offs. Aggarwal and the World Bank leave this thorny question open as it requires extensive political, societal, and legal negotiation and compromises.
7.2.3 AI ethical principles guidelines
The Vietnamese government should also support the development of ethical principles for responsible AI. The country has recently embraced the “fourth industrial revolution”—an expression that refers to fusing the physical, digital, and biological worlds by incorporating new technologies such as AI, the Internet, and robotics. In 2019, the Vietnamese government signed Resolution 52-NQ/TW to kick-start the Industry 4.0 race, which provided guidelines to invest in science and technology, develop the digital economy, promote rapid and sustainable development, foster innovation, ensure national defence and security, and protect the environment.Footnote 121 The resolution also lays the ground for developing regulation in areas ranging from the digital economy to the financial and monetary systems, consumer privacy, and intellectual property. However, there is no timeline for developing this ambitious legal framework. Setting up an ad hoc committee, launching discussions and negotiations with private stakeholders and society, and publishing AI ethical guidelines are essential for competing in the Industry 4.0 race.
Jobin, Lenca, and VayenaFootnote 122 identified five primary ethical principles that converge in the corpus of ethical guidelines published globally: transparency, justice and fairness, non-maleficence, responsibility, and privacy (Table 2).
Table 2. Lenca and Vayena’s ethical principles

a PDPC, supra note 110, pp. 35–50.
These five ethical principles are all relevant to ACS. Transparency is often present in debates on ACS regulation, harm reduction, and individual privacy and autonomy protection. It is closely related to responsibility and accountability. Legal scholars propose that consumers have the right to inspect, correct, and dispute sources of traditional and non-traditional data collected for scoring purposes, the data points and nature of the data, and the credit scores used to make loan decisions (allocation or rejection, loan pricing). Borrowers should be allowed to oversee the entire process, from big data collection to decision-making. They should also be able to understand why their loan applications are accepted or rejected, review their credit data, appeal rejections, and be given clues on how to improve their credit record and score. The goal is to make all credit data, AI-based predictive technology, calculation, and decision-making open, explainable, inspectable, auditable, and interpretable by the public, regulators, and third parties. There are ongoing challenges associated with transparency, even in countries with a long history of ACS. For example, Pasquale highlights that of the three credit bureaus operating in the US, Experian, TransUnion, and Equifax, an analysis of half a million files showed that 29% of consumers had credit scores differing by at least 50 points. When applied to mortgage applications, these differences could result in tens of thousands of dollars difference over the life of the loan.Footnote 123
External controllers and AI may be used to monitor and ensure both fair decisions and lack of harm. In the US, Citron and PasqualeFootnote 124 argue that the Federal Trade Commission should be given access to credit-scoring systems, especially data sets, source codes, and notes on variables, correlations, and inferences so that bias, arbitrariness, and unfair mischaracterization can be tested. In Vietnam, the State Bank could be granted similar privileges to establish a similar supervisory role and ensure that there is transparency, fairness, and accountability. Lastly, ACS models could also be subject to licensing and audit imperatives. The regulator could be authorized to also use machine-learning technology to pinpoint and solve biases.Footnote 125
These ethical concerns and legal and procedural propositions are relevant to Vietnam, a country where ACS is being rapidly deployed. However, the regulators and policy-makers do not yet fully fathom the importance of its regulation. Furthermore, a look at how credit-scoring start-ups and lenders deploy this technology suggests they have an unpreparedness or unwillingness to embrace basic ethical guidelines for responsible AI. For Vietnam’s interests, we hope that the government sets up an ad hoc committee gathering public and private actors that could eventually produce a set of ethical guidelines on responsible AI. Hopefully, the guideline will inspire a model for AI governance and enforceable regulations, which ACS fintech firms and lenders operating in Vietnam could follow in spirit, if not to the letter.
7.3 Findings of the section
Our proposal aims to regulate ACS in Vietnam in a manner that maximizes the benefits and minimizes the risks associated with these technological developments. The regulator could accommodate our measures in the regulatory sandbox programme for fintech innovation launched in 2021. This sandbox programme deals with rapidly changing fintech progress and the urgent need for reactive legislation to govern it. It applies to technologies used by credit organizations acting as banks according to the 2010 Law on Credit Institutions and those developed independently by fintech firms to support the financial and banking sector. It covers technologies such as ACS, digital payments, API, P2P lending, and blockchain. Since this sandbox is being tested as we speak, it may be too early to draw conclusions on its implementation and this space should be followed closely.
8. Conclusion
This article sheds much-needed light on the potential advantages and disadvantages of ACS, utilizing Vietnam as a case-study for developing effective and appropriate regulation of this emerging industry. Because Vietnam has been using ACS for half a decade and is leading the way in these products, these lessons can benefit many Southeast Asian nations that are now starting to engage with ACS. Assessing its impact is relevant in light of this technology’s ambition—to include tens of millions of unbanked and underbanked citizens in financial services markets—and the mixed feelings it has stirred worldwide. On the one hand, the “fast-evolving fintech–philanthropy–development complex” composed of agencies, IT firms, donors, foundations, IGOs, NGOs, and civil society organizations that promote global financial inclusionFootnote 126 praises the efficiency of ACS and its potential for fostering distributional fairness. On the other hand, this technology fuels anxieties about unfair discrimination and the loss of individual autonomy and privacy.
Our study concludes that it is too early for a comprehensive assessment of the benefits and risks of ACS in emerging countries of Southeast Asia, including Vietnam. The outcome will most likely be ambiguous and result in a long process of technological and regulatory negotiation, experimentation, adaptation, and normalization.Footnote 127 A key finding of this study is that this technology raises more questions than answers at this early stage of deployment, especially in emerging countries with limited financial infrastructure and awareness about privacy and data protection. This article highlights the importance of regulation to support fintech innovation and positive socioeconomic change. It suggests a cautious, pragmatic, and gradual approach to ACS. Given that this technology thrives in legal limbo in many developing and emerging countries around the world, including Vietnam, the regulator should address new challenges posed by big data and AI; reflect on what constitutes fairness and individual’s “intrinsic identities” and “vital interests;” and achieve normative trade-offs as soon as possible. It should also explore how to implement legal safeguards and human oversight to support efficiency and accuracy while preserving public interest and privacy. Equally vital is the need to develop ethical guidelines for responsible AI and, eventually, an AI governance model. The authorities must also ensure that domestic and foreign ACS fintech firms and lenders enforce regulations and follow ethical principles.
Acknowledgements
We would like to thank Milford Bateman, Cassey Lee, Ivan Small, Sandra Annette Booysen, Minh Nguyen, Bezawit Chichaibelu, Marie Langevin, and Alex Zhou Qin for their incisive comments and suggestions on the different drafts of this working paper. We are also grateful to Devon Airey for her brilliant research assistance and Joey Erh Luo Zhen for her meticulous editing work.