Stroke is a multifactorial and complex neurological disorder characterised by the sudden interruption of blood supply to the brain, resulting in a cascade of cellular and molecular events that leads to tissue damage and subsequent neurological deficits(Reference Yoon and Bushnell1). The worldwide incidence of stroke is estimated to be 17 million cases annually(Reference Pu, Wang and Zhang2). It is the second leading cause of death worldwide and a significant cause of disability(Reference Pu, Wang and Zhang2). The incidence of stroke varies in different regions of the world. It is highest in low- and middle-income countries, accounting for about 85 % of all stroke cases. However, its incidence is also increasing in developed countries due to a rise in the ageing population and the increasing incidence of risk factors such as high blood pressure, diabetes and obesity(Reference Hindsholm, Damgaard and Gurol3).
Stroke incidence, types and risk factors vary among ethnic groups, including Asians and Caucasians(Reference Gardener, Sacco and Rundek4). A complex interplay of genetic, primary metabolic disorders, lifestyle and socio-economic factors influences stroke risk(Reference Boehme, Esenwa and Elkind5). The prevalence of stroke is ten times higher in Asians than in Caucasians. Moreover, over 20 years ago, haemorrhagic strokes were more common among Asians, while among Caucasians, up to 80 % were likely to be ischaemic stroke events(Reference Simonetto, Sheth and Ziai6). However, the incidence of ischaemic stroke among Asians increased to about 76 % in 2004, and this level has been maintained. Damage caused by haemorrhagic stroke is more extensive than ischaemic stroke, and post-stroke complications in haemorrhagic stroke are severe(Reference Simonetto, Sheth and Ziai6).
Although hypertension, diabetes, smoking and obesity are widely acknowledged as risk factors for stroke, recent findings emphasise the substantial influence of genetic factors on an individual’s susceptibility to stroke(Reference Boehme, Esenwa and Elkind7). Genetic variations are pivotal in stroke risk by impacting various facets of vascular biology, including endothelial function, blood clotting mechanisms and overall vascular health(Reference Boehme, Esenwa and Elkind7). The genetic variants associated with stroke occurrence can exhibit variability between Asian and non-Asian populations. In a comprehensive genome-wide association study (GWAS) predominantly in individuals of European ancestry, specific genes such as G protein-coupled receptor kinase 5 (GRK5) and nitric oxide synthase 3 (NOS3) were identified in connection with stroke. Moreover, genetic variants of coagulation factor XI (F11), Kallikrein B1 (KLKB1), protein C, inactivator of coagulation factors Va and VIIIa (PROC), glycoprotein Ib platelet subunit alpha (GP1BA), laminin γ2 (LAMC2) and vascular cell adhesion molecule-1 (VCAM1) emerged as potential drug targets for stroke prevention(Reference Mishra, Malik and Hachiya8). The polygenic risk score (PRS) of the genetic variants of F11, KLKB1, PROC, GP1BA and LAMC2 demonstrated a robust predictive capacity for ischaemic stroke across diverse populations, including European (67 %), East Asian and African ancestries(Reference Mishra, Malik and Hachiya8). Additionally, two genetic loci, such as apolipoprotein E (APOE) and polyamine-modulated factor 1 (PMF1), have been identified as relevant to haemorrhagic stroke(Reference Chauhan and Debette9).
In addition to genetic factors, lifestyle factors, including diet, physical activity, smoking, alcohol consumption and stress, play a pivotal role in the pathogenesis of stroke. There is an interplay between lifestyle-related risk factors and genetic factors, leading to a stroke event(Reference Rutten-Jacobs, Larsson and Malik10). Genetic factors can influence an individual’s response to these lifestyle choices, potentially amplifying or mitigating their impact on stroke risk. Gene variants associated with metabolism, blood clotting and vascular health can interact with lifestyle factors to modulate an individual’s susceptibility to stroke(Reference Della-Morte, Pacifici and Rundek11). However, few studies have been conducted on identifying genetic variants linked to stroke and on the interaction of genetic and lifestyle factors influencing chances of stroke occurrence, especially in Asians. In the present study, we aimed to elucidate the intricate interplay between genetic predisposition and lifestyle-related factors on the chances of having a stroke. By exploring how genetic factors interact with lifestyle choices, we sought to elucidate the factors involved in the OR for stroke in individuals. This understanding can contribute to developing personalised strategies and targeted interventions to reduce stroke prevalence and improve stroke outcomes.
Methods
Study participants
Between 2010 and 2014, a voluntary enrollment process included 58 701 individuals aged 40–74 years in a city multicentred hospital-based cohort, part of the Korean Genome and Epidemiology Study (KoGES)(Reference Chauhan and Debette9). The age range was chosen to focus on health-related conditions and diseases, with a longitudinal investigation incorporating an extended follow-up period. The study adhered to the Declaration of Helsinki guidelines, obtaining Institutional Review Board (IRB) approvals from the Korea National Institute of Health (KBP-2015-055) and Hoseo University (1041231-150811-HR-034-01) for the KoGES protocol. All participants provided written informed consent, which is securely stored in the Korea Biobank.
Stroke definition, study design and sample size
Participants were included if they affirmed at least one physician-diagnosed stroke event, constituting the stroke group (case; n 672), while the remaining individuals formed the control or non-stroke group (control; n 58 029). The specific type of stroke was not specified. Exclusions were made for individuals with brain-related disorders such as Alzheimer’s and Parkinson’s diseases, except for stroke cases.
The sample size for logistic analysis was calculated using the Gpower calculator (Gpower Software, the University of Dusseldorf, Germany). To achieve significance at α = 0·05, β = 0·99, at an odds ratio of 1·05, and considering a stroke incidence of less than 1 %, a total sample size of 53 254 in the city hospital cohort was determined to be sufficient to meet the significance goal.
Participant characteristics and biometric measurements
Demographic, anthropometric and lifestyle data were collected from the participants during their initial visit. It included age, gender, education, income, smoking habits, alcohol consumption and physical activity(Reference Park12). The participants’ smoking habits were categorised into non-smokers, past smokers who had not smoked in the past 6 months and current smokers who had smoked at least twenty cigarettes in their lifetime(Reference Park12). Alcohol consumption was quantified by multiplying the alcohol content per occasion with the frequency of alcohol consumption. Coffee intake was measured like alcohol intake. Regular physical activity was assessed by inquiring whether the subjects engaged in 150 min or more of physical activity per week, with responses recorded as ‘yes’ or ‘no’.
At the initial interview, height and weight were measured as previously outlined(Reference Park12) to calculate the BMI by dividing body weight (in kilograms) by the square of height (in meters). Obesity was defined as a BMI of ≥ 25 kg/m2. Blood pressure was measured by a medical professional using a sphygmomanometer, while the participant was seated and at rest. Three readings were taken, and the average systolic blood pressure and diastolic blood pressure were determined. Blood samples were collected under fasting conditions to measure lipid profiles, alanine aminotransferase, aspartate aminotransferase and creatinine concentrations in the circulation using a Hitachi 7600 Automatic Analyzer (Hitachi). Plasma glucose and blood glycosylated Hb (HbA1c) levels and leucocyte counts were measured using an automatic analyser (ZEUS 9·9; Takeda). Serum high-sensitive C-reactive protein concentrations were measured using an enzyme-linked immunosorbent assay kit.
Assessment of typical food consumption through a semi-quantitative FFQ
Food intake over the last year was evaluated using a Semi-Quantitative FFQ (SQFFQ) administered by a skilled technician. Specifically designed to capture typical dietary patterns of Koreans, the SQFFQ’s accuracy and repeatability have been validated(Reference Park, Zhang and Lee13). The SQFFQ was comprised of 106 common food items in the Korean diet, and daily food intake was calculated by multiplying food frequencies per day by relative to the standard portion size in grams, aided by visual references such as photographs to reduce the bias. Participants provided food frequency responses categorised into nine options: never or rarely, once a month, two to three times a month, once or twice a week, three or four times a week, five or six times a week, daily, twice daily and ≥ 3 times daily. Daily food consumption was estimated by multiplying the median daily frequency of each food category by its respective portion size. Nutrient analysis for the obtained food intake data utilised the CAN-Pro 2·0 nutrient intake assessment software developed by the Korean Nutrition Society (Seoul, Korea). Nutrient intake was compared with the Korean Dietary Reference Intake, a set of dietary guidelines, to assess adherence to recommended nutritional standards(Reference Park12). The dietary inflammation index (DII), glycaemic index and glycaemic loading index were computed utilising previously reported equations(Reference Park12).
Analysis of dietary patterns using principal components analysis
We analysed thirty predefined food groups extracted from the original 106 food items in the SQFFQ to assess dietary patterns, as previously documented(Reference Ahn, Kwon and Shim14). The dietary patterns of these predefined food groups were derived through principal component analysis. The number of clusters was determined based on eigenvalues exceeding 1·5, generating four distinct dietary patterns(Reference Steel, Torrie and Dickey15). An orthogonal rotation procedure (varimax) was applied to ensure independence, which yielded four uncorrelated dietary patterns. Foods with factor-loading values of ≥ 0·40 were deemed to contribute substantially to the specific pattern(Reference Kim and Mueller16). These four dietary patterns were identified as the Korean-balanced diet, plant-based diet, Western-style diet and rice-main diet (refer to online Supplementary Table 1).
Genotyping and genome-wide association study quality assurance for assessing the chances of stroke occurrence
Genomic DNA genotyping for 58 701 participants was conducted by the Center for Genome Science at the Korea National Institute of Health. Genomic DNA extracted from whole blood was genotyped using the Affymetrix Genome-Wide Human SNP Array 5·0 (Affymetrix). Genotyping accuracy and quality were assessed utilising the genotyping algorithm, Bayesian robust linear model, with Mahalanobis distance classifier(Reference Hur, Yang and Kim17). The genotyping inclusion criteria were defined as follows: genotyping accuracy of ≥ 98 %, a genotyping call rate of < 4 % for missing genotypes, ≤ 3 % plate heterozygosity, absence of gender biases and a Hardy–Weinberg equilibrium P value greater than 0·05(Reference Hur, Yang and Kim17).
To investigate the genetic variant associated with stroke, a GWAS was conducted between stroke and non-stroke groups, adjusting for covariates such as age, gender, area of residence, income, BMI, energy and alcohol intake, physical exercise and smoking using PLINK (http://pngu.mgh.harvard.edu/∼purcell/plink). The distribution and suitability of genetic variants were examined through Manhattan and quantile–quantile plots. The lambda value of the quantile–quantile plot approached 1, indicating the appropriateness of the GWAS results. Genetic variants exhibiting high linkage disequilibrium (D’ < 0·2, r2 > 0·05) were excluded using Haploview 4·2 in PLINK, as they provided redundant genetic information for assessing the chance of stroke. Multi-marker Analysis of GenoMic Annotation gene-set analysis was performed for the curated gene sets and the gene ontology terms obtained from the Molecular Signatures Database using the FUMA GWAS (fuma.ctglab.nl/). The table displayed the significance level of 5 × 10–4.
Expression quantitative trait locus analysis
The expression quantitative trait locus analysis involves directly estimating the expression of candidate genes using genetic variants found at risk loci. In the context of stroke risk, this analysis identifies gene expressions associated with specific genetic variants through expression quantitative trait locus analysis, employing the genotype-tissue expression × expression quantitative trait locus calculator (https://gtexportal.org/home/testyourown, accessed on April 22, 2023).
Molecular docking of cytochrome P450 (CYP), family 1, subfamily a, polypeptide 1(1A1) (CYP1A1)_rs143070677 with food compounds
Among the genetic variants associated with stroke, CYP1A1_rs143070677 had only missense mutation among the identified genetic variants, and the food components were screened for CYP1A1_rs143070677 wild and mutated types. The sequences of the CYP1A1_rs143070677 were received from the ensemble genome browser website, and its RNA structure was generated using https://rnacomposer.cs.put.poznan.pl/. The wild and mutated protein data bank formats were converted into protein data bank, partial charge (Q) and atom type (T) (PDBQT) files using AutoDock Tools 1·5·6 (Molecular Graphics Laboratory, Scripps Research Institute, FL, USA)(Reference Yuan, Liu and Zhou18). The PDBQT format of the CYP1A1_rs143070677 was simulated with about 20 000 food components. The food components were selected when the binding energy between CYP1A1_rs143070677 and the food components was less than –10·0 kcal/mol(Reference Yang, Shi and Xie19). The lower the binding free energy, the tighter the binding and the greater the affinity.
Analysis of interactions between genetic variants using generalised multifactor dimensionality reduction
The generalised multifactor dimensionality reduction method is a flexible genetic model designed to identify and characterise complex interactions among discrete genetic features. We applied generalised multifactor dimensionality reduction to pinpoint the genetic variants that interact and were linked with the risk of stroke. We chose the best genetic model based on specific criteria: a significant P value (P < 0·05) from the sign test of trained balance accuracy and test balance accuracy, along with a strong cross-validation consistency score of 9 or 10 out of 10(Reference Hur, Wu and Yang20). We computed a PRS to quantify genetic risk by summing the risk alleles across the genetic variants within the selected optimal model.
Analysis of the association of stroke OR with lifestyle factors according to the polygenic risk score groups
We used adjusted logistic regression analysis to evaluate the association between chances of stroke and various factors, such as anthropometric measurements, biochemical data and genetic parameters. We utilised two models: the first adjusted for gender, age, area of residence, education level and BMI; the second included additional adjustments for energy intake, smoking and drinking habits and overall physical activity. OR and 95 % CI were calculated using logistic regression, considering the low-PRS group as the reference. Two models were considered: one adjusting for age, gender, education, residence area and BMI (model 1), and another adjusting for model 1 covariates plus smoking status, alcohol intake, physical activity and daily energy intake (model 2).
Assessing the interaction between the polygenic risk score and lifestyle factors influencing the chances of stroke
We conducted a multivariate generalised linear model analysis to investigate potential interactions between PRS and lifestyle factors affecting stroke chances. This analysis incorporated the main effects of PRS and lifestyle factors, including nutrient intakes, along with their interactions and relevant covariates. Participants were stratified into higher or lower lifestyle groups based on recommended values for each variable, such as dietary reference intake. After adjusting for covariates, we used a multivariate interaction model to assess the interplay between PRS and lifestyles, including dietary intake. An adjusted logistic regression analysis was also conducted, utilising cut-off values determined within each lifestyle group. These cut-offs were established considering dietary reference intake and the thirty-third percentiles for specific parameters.
Statistical analysis
Statistical analysis was conducted using the SAS software (version 9.3). We chose not to exclude observations with missing data in our analysis since there were missing values in some variables in individual participants while others were complete. Missing data were not imputed, and the analysis was conducted using the available data. The descriptive statistics of the categorical variables were computed based on gender and stroke incidence, and χ 2 tests were employed to analyse the frequency distributions of classification variables. Adjusted means and sd were assessed for continuous variables with consideration for gender and stroke incidence. Significant differences between groups were determined using a two-way ANOVA that accounted for covariates such as age, gender, residence area, BMI, education, smoking, alcohol intake, daily energy intake and physical activity. Multiple group comparisons were conducted using Tukey’s test. Usual food intake was estimated from the SQFFQ without imputation. The PRS derived from the selected model were categorised into low-, middle- and high-PRS groups. The distribution of categorical variables among these groups was examined using the χ 2 test. Additionally, a one-way ANOVA was applied to analyse continuous variables across PRS groups, adjusting for factors including age, sex, BMI, education, income, daily energy intake, alcohol consumption, smoking and physical activity. Statistically significant results were defined as those with P values < 0·05.
Results
Association of participants’ demographics and nutrient intake with stroke prevalence
The stroke prevalence was 1·20 % (n 672) among the participants (Table 1). Demographic variables like age, education and income did not vary significantly based on stroke occurrence or gender and were not linked to chances of stroke (Table 1). When assessing the daily nutrient intake using the SQFFQ, we observed no differences in the intake of carbohydrates (CHO), fat, protein, branched-chain amino acids (BCAA), fibre, Ca, Na, vitamin C, vitamin D, cholesterol and flavonoids between the stroke and non-stroke groups (online Supplementary Table S1). Only energy intake was positively associated with stroke. Similarly, indicators like the DII, glycaemic index and glycaemic load showed no association with the chances of stroke. Moreover, dietary patterns identified through PCA did not show variations regarding stroke prevalence or gender (online Supplementary Table S1). Additionally, lifestyle factors such as alcohol intake, exercise and smoking were not associated with stroke chances (Table 1).
Table 1. Demographic and lifestyle characteristics according to gender and stroke and their association with the occurrence of stroke
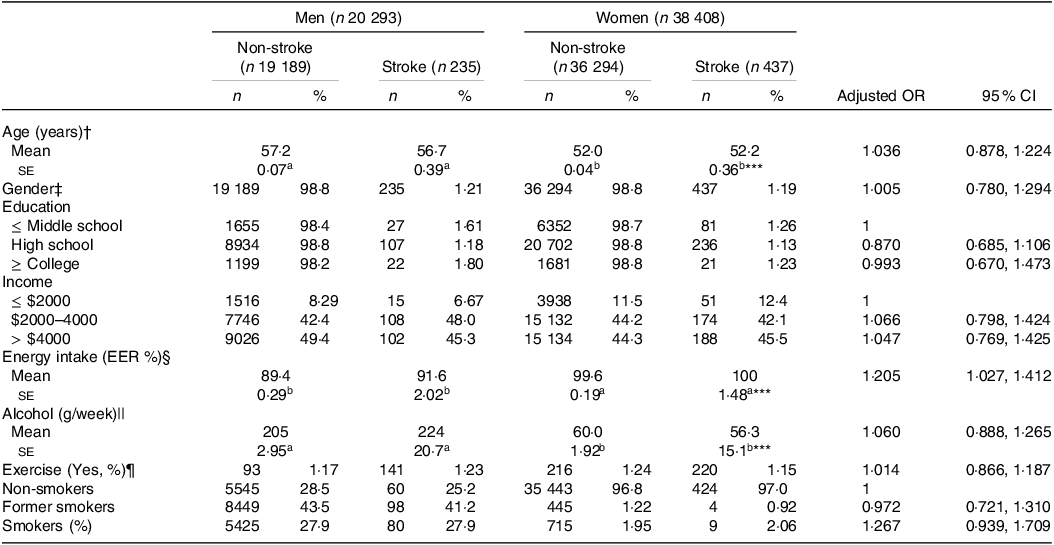
The values represent means ± se or number of the subjects (percentage of each group). Adjusted OR and 95 % CI with the covariates of adjusting for age, gender, BMI, physical activity, education, smoking and intake of alcohol and energy. The cut-off points of the reference were as follows:
a,b Different alphabets indicated significantly different among the groups in Tukey’s test at P < 0·05.
† < 55 years old for age.
‡ Men.
§ < Estimated energy requirement (EER).
|| < 20 g/d alcohol intake.
¶ < 150 min/week moderate-intensity exercise.
*** Significantly difference by gender at P < 0·001.
Association of anthropometric and biochemical parameters with stroke incidence
There was no significant difference in the prevalence of metabolic syndrome between the groups with and without stroke (online Supplementary Table S2). There were no significant variations in the various components of metabolic syndrome, such as waist circumference indicating abdominal obesity, hyperglycaemia, blood HbA1c levels, serum HDL-cholesterol and LDL-cholesterol levels and hypertension, between the groups with and without stroke. However, there were gender-based differences in these components (online Supplementary Table S2). Interestingly, serum TAG concentrations were notably higher in the stroke group compared with the non-stroke group, particularly among men (online Supplementary Table S2). On the other hand, indicators like the estimated glomerular filtration rate, serum alanine aminotransferase and aspartate aminotransferase concentration, serum C-reactive protein (CRP) concentration and leucocyte counts did not show significant variations between the stroke and non-stroke groups (online Supplementary Table S2). Furthermore, metabolic syndrome and metabolic parameters did not demonstrate an association with the chances of stroke (online Supplementary Table S2).
Interaction of genetic variants with each other using generalised multifactor dimensionality reduction
We visualised the statistical significance of genetic variants associated with stroke using a Manhattan plot (online Supplementary Fig. S1(a)). A Q-Q plot, which compares the observed data distribution to an expected theoretical distribution, is presented in online Supplementary Fig. S1(b). The lambda value (λ = 1·007), a measure of inflation, indicated that the GWAS results were not inflated (online Supplementary Fig. S1(b)). No genetic variants with a significance level below P < 5×10–8 were found for the chance of stroke. Therefore, we opted for a more liberal significance level (P < 5×10–5) to identify significant genetic variants.
Among the genetic variants associated with stroke risk, eleven showed interactions with each other through generalised multifactor dimensionality reduction. The optimal SNP–SNP interaction models encompassed 5–8 genetic variants (Table 2). The 5-, 6- and 7-SNP models demonstrated a P value < 0·001 for the sign test of trained balance accuracy and test balance accuracy after adjusting for age, gender, residence area, education and BMI for covariate set 1 and additionally considering energy intake, physical activity, alcohol intake and smoking status for covariate set 2 (Table 2). Both models exhibited a cross-validation consistency score of 10/10 (Table 2).
Table 2. The characteristics of the ten genetic variants of genes in the risk of stroke used for the generalised multifactor dimensionality reduction analysis
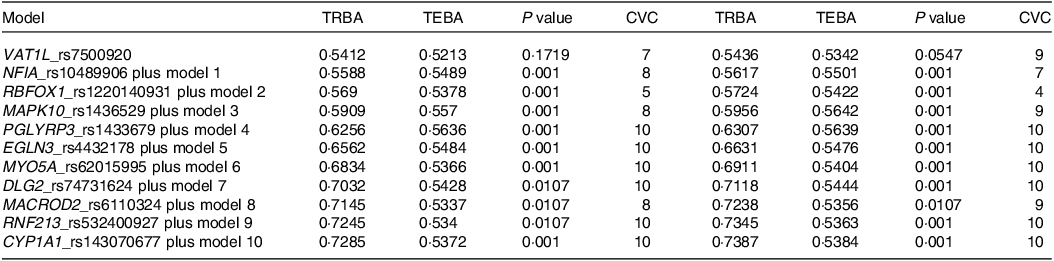
Adjusted for the covariates of adjusting for age, gender, BMI, physical activity, education, smoking and intake of alcohol and energy.
Specifically, the 5-SNP model comprised vesicle amine transport 1 like (VAT1L)_rs7500920, nuclear factor-IA (NFIA)_rs10489906, RNA-binding Fox-1 homolog 1 (RBFOX1)_rs1220140931, mitogen-activated protein kinase 10 (MAPK10)_rs1436529 and peptidoglycan recognition protein 3 (PGLYRP3)_rs1433679 (Table 2). The 6-SNP model included the SNP from the 5-SNP model and Egl-9 family hypoxia-inducible factor 3 (EGLN3)_rs4432178, while the 7-SNP model incorporated genetic variants from the 6-SNP model and myosin-Va (MYO5A)_rs62015995 (Table 2). The 5-, 6- and 7-SNP models with interactions between genetic variants were found to be associated with a 2·5-, 2·9- and 2·8-fold chances of stroke, respectively, after adjusting for confounders (Fig. 1).

Fig. 1. Adjusted OR and 95 % CI of stroke with polygenic chance score (PRS) of the 5-, 6- and 7-SNP models. PRS was calculated with the genetic variants associated with stroke chance selected by GWAS and GMDR. They were calculated using logistic regression analysis after adjusting with the covariates of age, area of residence, BMI, energy intake, smoking and drinking habits, overall physical activity and energy intake. GWAS, Genome-Wide Association Study; GMDR, generalised multifactor dimensionality reduction.
Genetic characteristics of variants linked to stroke risk identified via genome-wide association study
Table 3 presents the genetic attributes of the chosen variants (P < 5×10–5) associated with the chance of stroke. Among these, one SNP (MAPK10_rs1436529) showed an inverse association (0 < OR < 1), while ten SNP were positively associated with stroke risk (OR > 1). Their minor allele frequency exceeded 0·01, and the P value for Hardy–Weinberg equilibrium was greater than 0·05, meeting the inclusion criteria (Table 3). PGLYRP3_rs1433679 was located in the 3’untranslated regions (UTR), and CYP1A1_rs143070677 represented a missense mutation. Notably, NFIA_rs10489906, MAPK10_rs1436529, discs large MAGUK scaffold protein 2 (DLG2)_rs74731624 and phospholipase C beta 4 (PLCB4)_rs77987151 were situated in the nonsense-mediated RNA decay (NMD) transcript, while the rest were within the intronic regions (Table 3).
Table 3. The characteristics of the ten genetic variants related to stroke used for the generalised multifactor dimensionality reduction analysis

* Chromosome.
† SNP.
‡ Minor allele.
§ Major allele.
|| OR; se.
¶ P value for OR after adjusting for age, gender, BMI, residence area, physical activity, education, smoking and intake of alcohol, dietary fibre and energy.
** Minor allele frequency.
†† Hardy–Weinberg equilibrium. NFIA, nuclear factor-IA; PGLYRP3, peptidoglycan recognition protein-3; MAPK10, mitogen-activated protein kinase-10; DLG2, discs large MAGUK scaffold protein-2; CYP1A1, cytochrome P450 family-1 subfamily A member 1; RBFOX1, RNA-binding fox-1 homolog 1; VAT1L, vesicle amine transport 1-like protein; RNF213, ring finger protein 213; PLCB4, phospholipase C beta 4; MACROD2, MACRO domain containing 2; PDE9A, phosphodiesterase-9A.
Gene expression by expression quantitative trait locus according to genetic variants
Specific genetic variants associated with stroke risk demonstrated distinct gene expressions across various tissues. The risk allele of NFIA_rs10489906 exhibited elevated expression levels in adipose tissue (β = 0·28, P = 0·0029) and the tibial nerve (β = 0·2, P = 0·013). Similarly, the risk allele of MAPK10_rs1436529 showed increased expression in the tibial nerve (β = 0·077, P = 0·0083) and aorta artery (β = 0·11, P = 0·0034). On the other hand, PLCB4_rs77987151 (β = –0·42, P = 0·0023) and VAT1L_rs7500920 (β = –0·3, P = 0·0078) displayed altered expression in the tibial artery (online Supplementary Fig. S2).
Associations of stroke-related genes by multi-marker analysis of genomic annotation gene-set analysis
In Table 4, the outcomes of the multi-marker analysis of genomic annotation gene-set analysis are depicted, revealing the relationship between the curated gene sets, gene ontology terms and stroke. The analysis highlighted significant associations with gene ontology biological processes such as ‘regulation of IL-18 production’, ‘positive regulation of IL-18 production’ and ‘interferon-γ (IFN-γ) biosynthetic process’ (P < 5×10–5). The beta value represents the effect size or the regression coefficient associated with genetic variants related to stroke risk in the linear regression model and the pathways related to stroke risk per one-unit change in the genetic variants (Table 4). Since IL-18 is an IFN-γ-inducing factor, stroke risk is potentially involved in IFN-γ biosynthesis (Table 4). This finding suggests a noteworthy genetic connection between stroke risk and autoimmune or inflammatory diseases due to dysregulated innate and adaptive immunity.
Table 4. MAGMA gene-set analysis of stroke-associated genes in curated gene sets and gene ontology (GO) terms
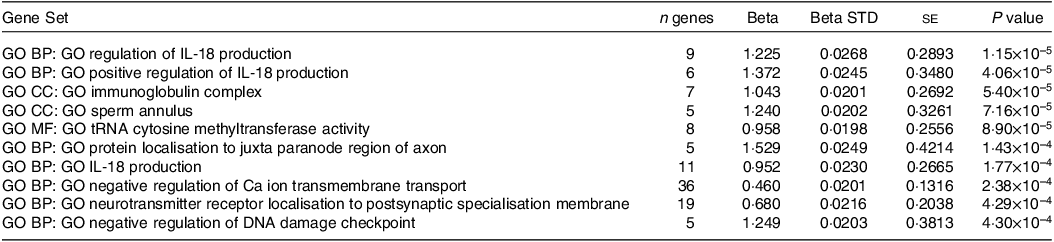
MAGMA, multi-marker analysis of genomic annotation; BP, biological process; CC, cellular component; MF, molecular function; n, number; STD, standardisation.
Molecular docking of CYP1A1_rs143070677 with food compounds
When the wild type (WT) and the mutated type (MT) of CYP1A1_rs143070677, a missense mutation of CYP1A1, bound to anthocyanins, flavonoids, hydrolyzable tannins and alkaloids, the binding free energy was lower than –10 kcal/mol (WT: 1352 bioactive compounds; MT: 1357 bioactive compounds; data not shown). The binding free energy of some bioactive compounds to the WT and the MT CYP1A1_rs143070677 was similarly lowered with polyphenols. However, the binding energy of other compounds to CYP1A1_rs143070677 was higher than –9 kcal/mol. Specifically, cyanidin 3-caffeoyl-sophoroside 5-glucoside, theaflavate A, artobiloxanthone, delphinidin 3,5-di(6”-malonyl glucoside), gambiriin B3, (9Z,9’Z)-7,7’, 8,8’-tetrahydro-lycopene and acrimarine had higher binding free energy with the CYP1A1_rs143070677 major allele (non-risk allele) than the minor allele (risk allele), and dimalonylawobanin, lepidine E, cis-geranylgeranyl bixin, zucchini factor B, isoscoparin 7-glucoside, epoxy fumitremorgin C and avenalumin II had higher binding free energy with the CYP1A1_rs143070677 minor allele (risk allele) than the major allele (non-risk allele) (online Supplementary Table S3).
Figures 2(a)–(f) illustrate the interactions between the WT CYP1A1_rs143070677 protein and isoscoparin 7-glucoside, a member of the class of compounds known as flavonoid-7-o-glycosides. It is found in barley leaves. Fig. 2(a) depicts the binding free energy, highlighting the hydrogen bond interactions with pink and green, indicating hydrogen donors and acceptors, respectively. The two-dimensional representation in Fig. 2(b) shows their specific binding positions and intermolecular forces. Furthermore, Fig. 2(c) displays the binding affinity and interactions between isoscoparin 7-glucoside and the WT CYP1A1_rs143070677. The binding free energies for isoscoparin 7-glucoside with WT and MT CYP1A1_rs143070677 were calculated to be -10·7 and -8·2 kcal/mol, respectively (Fig. 2(d)). In addition, Fig. 2(e) and Fig. 2(f) present the root mean square deviation (RMSD) and root mean square fluctuation (RMSF) for WT and MT CYP1A1_rs143070677 binding to punicafolin. The RMSD for WT CYP1A1_rs143070677 binding with punicafolin remained stable at around 3 Å over 100 nanoseconds (Fig. 2(e)). Similarly, the RMSF for WT CYP1A_1 rs143070677 binding with isoscoparin 7-glucoside indicated stability, not exceeding 3 nm, except at the 580-residue index in the RMSF (Fig. 2(f)). These findings strongly support the stable binding of isoscoparin 7-glucoside to the WT CYP1A1_rs143070677.

Fig. 2. Molecular docking and molecular dynamic simulation (MDS) of isoscoparin 7-glucoside on wild (WT) and mutated type (MT) CYP1A1_rs143070677. (a) Molecular docking of isoscoparin 7-glucoside on CYP1A1_rs143070677 WT. (b) The interaction force between isoscoparin 7-glucoside and CYP1A1_rs143070677 WT. (c) Molecular docking of isoscoparin 7-glucoside on CYP1A1_rs143070677 MT. (d) The interaction force between isoscoparin 7-glucoside and CYP1A1_rs143070677 MT. (e) The root-mean-square deviation (RMSD) of isoscoparin 7-glucoside on WT and MT of CYP1A1_rs143070677. (f) The root-mean-square fluctuation (RMSF) of isoscoparin 7-glucoside on WT and MT of CYP1A1_rs143070677. Isoscoparin 7-glucoside lowered the binding energy to cytochrome P450(CYP), family 1, subfamily A, polypeptide 1(1A1a)_rs143070677 WT and MT. Binding affinity with a hydrogen bond, carbon–hydrogen bond, pi-allyl and Van der Waal’s force was shown between the WT or MT CYP1A1_rs143070677 proteins and isoscoparin 7-glucoside with a hydrogen bond. RMSD and RMSF between isoscoparin 7-glucoside and WT and MT CYP1A1_rs143070677 were presented, and they were higher in the MT than in the WT.
Genetic interplay between lifestyle factors and the chances of stroke occurrence
The impact of energy, CHO, n-3 fatty acids and BCAA intake on chances of stroke was found to interact with the PRS (Table 5). Specifically, the genetic impact was more significant with a high energy intake than a low one. The stroke incidence in the participants with medium-PRS and high-PRS was much higher in the high-energy intake group than in the low-energy intake group (Fig. 3(a)). Similar patterns emerged for CHO and BCAA intake, wherein stroke prevalence was notably higher with high-CHO and high-BCAA intake within the high-PRS group than in the low-CHO and low-BCAA intake groups (Fig. 3(b) and (c)). Furthermore, stroke prevalence within the high-PRS group was higher in individuals with low-fat and low-n-3 fatty acid intake compared with those with high-fat and high-n-3 fatty acid intake (Fig. 3(d)).
Table 5. Adjusted OR for the chances of stroke by polygenic risk scores of the 6-SNP model (PRS) after covariate adjustments according to low- and high-lifestyle factors
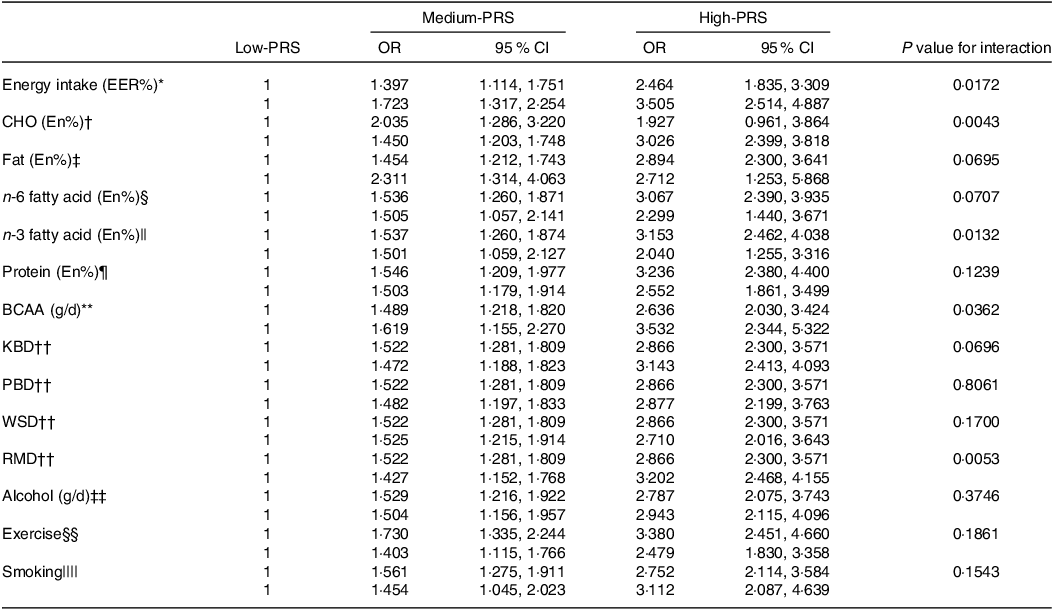
BCAA, branched-chain amino acids; KBD, Korean balanced diet; PBD, plant-based diet; WSD, Western-style diet; RMD, rice-main diet.
Multiple logistic regression models include the corresponding main effects, interaction terms of PRS and main effects (lifestyle factors) and potential confounders such as age, gender, BMI, residence area, physical activity, education, smoking and intake of alcohol, dietary fibre and energy. The reference was the low-PRS.
Values represent OR and 95 % CI. PRS with seven SNP were divided into three categories (1–5, 6–7 and ≥ 8) by tertiles as the low, medium and high groups of the best model of GMDR. The cut-off point was as follows:
* < EER.
† < 70 energy percent (En%).
‡ < 15 En%.
§ < 3·0 En%.
|| < 0·7 En%.
¶ < 13 En%.
** < 18·6 g/d.
†† < 33th percentiles.
‡‡ < 20 g/d alcohol intake.
§§ < 150 min/week moderate-intensity exercise.
|||| Non-smoking.
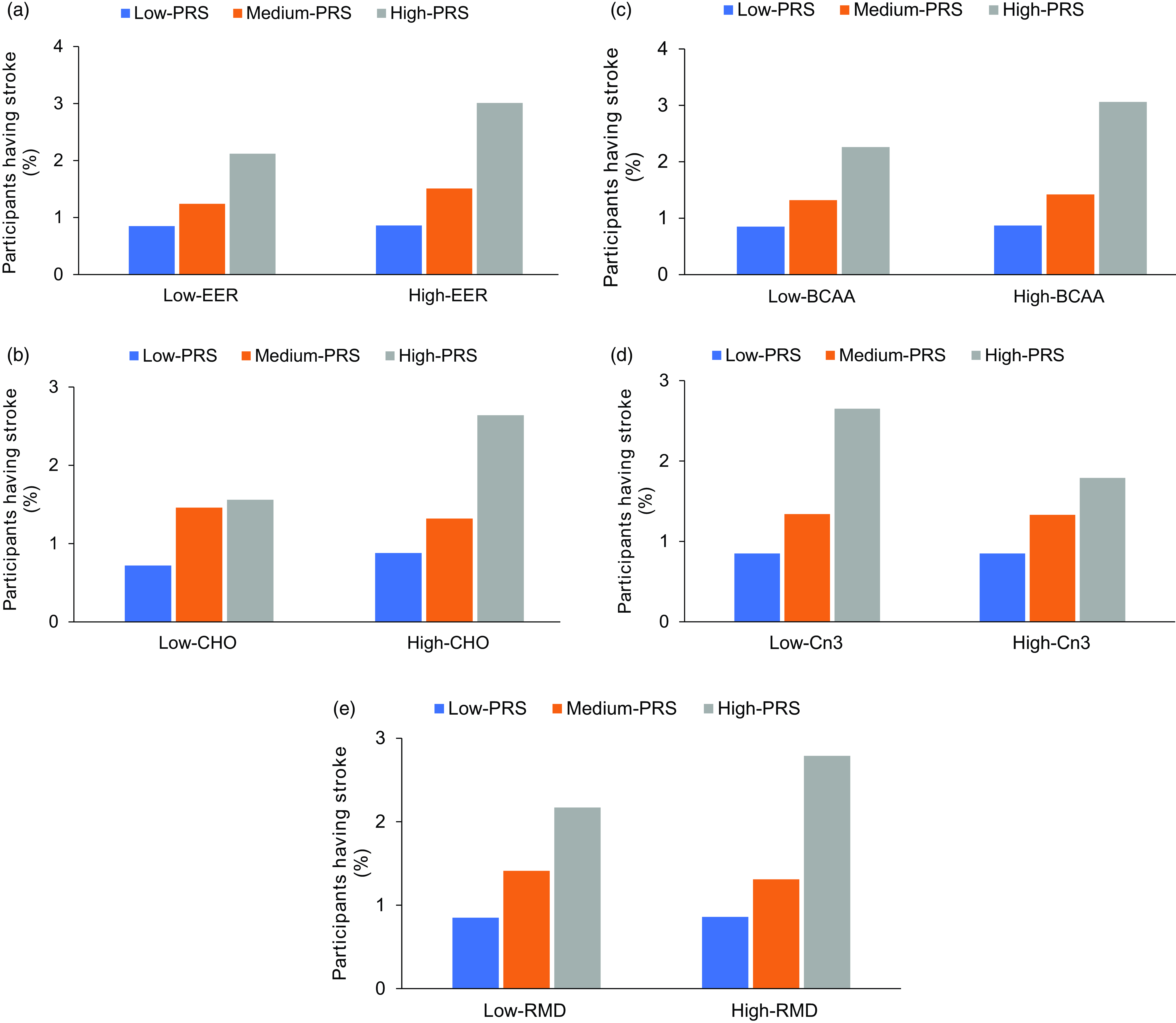
Fig. 3. Stroke incidence according to the parameters that interact with polygenic risk scores of the 6-SNP model. (a) Stroke incidence in the participants according to daily energy intake (cut-off value: estimated energy intake (EER)). (b) Stroke incidence in the participants according to carbohydrate intake (cut-off value: 70 energy percent). (c) Stroke incidence in the participants according to branched-chain amino acids (BCAA) intake (cut-off value: 18·6 g/d). (d) Stroke incidence in the participants according to n-3 fatty acid intake (cut-off value: 0·7 energy percent). (e) Stroke incidence in the participants according to the consumption of a rice-main diet (cut-off value: 33rd percentile). The P value indicates the interaction between PRS and designated parameters. PRS, polygenic risk score.
Among the four identified dietary patterns, the RMD exhibited a significant interaction with the PRS in influencing the chances of stroke (Table 5). Participants adhering to a high RMD displayed a more pronounced impact of the PRS on stroke prevalence than those on a low RMD (Fig. 3(e)). However, no significant interactions were observed between the PRS and alcohol intake, exercise or smoking status for chances of stroke.
Discussion
No studies have shown an interaction between the SNP in the PRS or PRS and environmental factors regarding stroke occurrence(Reference Hur, Wu and Yang20,Reference Heng, Kim and Yang21) . The present study investigated the intricate interplay between genetic predisposition and lifestyle-related risk factors in the context of stroke in a large hospital-based cohort. The results revealed that some genetic variants of PTPRD, DLG2 and GCLC were similar to those in a previous study(Reference Jo, Kwak and Kim22). However, genetic variants were mainly linked to the IL-18 and IFN-γ pathways. The PRS of the genetic variants linked to stroke chance interacted with energy, carbohydrates, n-3 fatty acids, BCAA intake and RMD. The results could be applied to personalised nutrition to prevent the prevalence of stroke.
The known non-modifiable risk factors for stroke are age, sex and race/ethnicity and the modifiable risk factors are obesity, hypertension, dyslipidaemia, hyperglycaemia, smoking, diet and physical inactivity. There is emerging evidence of the impact of genetic factors, which is a non-modifiable risk factor, on stroke chance and their interaction with lifestyle factors to influence the chance of stroke. Recent research has also revealed that inflammatory disorders, infection, pollution and cardiac atrial disorders may also trigger a stroke event(Reference Boehme, Esenwa and Elkind7). Nevertheless, the current study revealed no clear distinctions in established risk factors between the stroke and non-stroke groups. It suggests that individuals who experienced a stroke may have actively regulated their energy, glucose and lipid metabolism to forestall further stroke occurrences. Stroke patients included in this study exhibited no differences in the metabolic risk factors between the stroke and non-stroke groups, indicating effective management of dyslipidaemia, hyperglycaemia and hypertension in stroke patients.
A meta-analysis of the GWAS from 2000 onward presented several genetic variants for ischaemic stroke identified in various studies(Reference Huang, Yang and Shu23). These included angiotensin-converting enzyme (ACE), angiotensinogen (AGT) variant (M235T) and aldosterone synthase CYP11B2 (C344T) linked to renin-angiotensin, tissue plasminogen activator (tPA), plasminogen activator inhibitor 1 (PAI-1) and factor VII related to coagulation/fibrinolysis, GTP cyclohydrolase I (GCH1) and glutathione peroxidase 3 (GPx-3) linked to antioxidants, and apolipoprotein-E (APOE), apolipoprotein A5 (APOA5), and paraoxonase 1 (PON1) related to lipid metabolism, IL-1, IL-6, TNF-a and arachidonate 5-lipoxygenase activating protein (ALOX5AP) involved in inflammation(Reference Huang, Yang and Shu23). The present study showed that the genes of the variants for stroke chance were related to IL-18 and IFN-γ pathways, which are known to regulate the immune response of its target cells such as T-cells, natural killer cells, macrophages and other immune cells through the Janus kinase/signal transducers and activators of transcription (JAK-STAT) signalling pathway. They are involved in innate and adaptive immunity against viruses, some bacteria and protozoan infections. Overexpression of IFN-γ is involved in autoimmune diseases(Reference Matta, Song and Li24). Therefore, a stroke event may be linked to immune disorders and potentially autoimmune diseases(Reference Parikh, Merkler and Iadecola25). Consistently, the present study showed the genetic relationship between immune disorders, autoimmune diseases and stroke.
The genetic variants selected to evaluate stroke chance in the present study included VAT1L, NFIA, RBFOX1, MAPK10, PGLYRP3, EGLN3, MYO5A and DLG2, and they interacted with each other to elevate stroke chance. The chance of stroke due to the genetic variants was 0·796 ∼ 1·786-fold in the 5-, 6- and 7-SNP models. However, their PRS was positively associated 2·8 times with stroke chance, indicating that the genetic variants interacted to elevate stroke chance. The VAT1L protein has an oxidoreductase activity and zinc ion-binding activity. It is overexpressed in the brain-hypothalamus (×9·5), nucleus accumbens (basal ganglia) (×6·2), cerebellar hemisphere (×4·8), caudate (basal ganglia) (×4·8), cerebellum (×4·7) and putamen (basal ganglia) (×4·0). However, in the tibial artery, the GG allele of VAT1L_rs7500920 expression was higher than the CC allele. NFIA is a critical transcriptional regulator of astrocyte development, and it is highly expressed in reactive astrocytes in human neurological injury. NFIA-deficient astrocytes exhibit defects in remodelling of the blood–brain barrier after white matter injury, which correlates with suppression of remyelination(Reference Laug, Huang and Huerta26). Moreover, after cerebral ischaemia/reperfusion, microRNA 424 (MiR-424) prevents astrogliosis by enhancing trimethylation of histone H3 lysine 27 (H3K27me3) via NFIA/DNA methyltransferase-1 signaling in elderly mice(Reference Zhao, Li and Wang27). RBFOX1 is a splicing factor of Ca/calmodulin-dependent protein kinase IIγ(CAMK2G), and its expression is reduced in patients with post-myocardial infarction(Reference Williams, Walton and Pinell28). Monocyte locomotion inhibitory factor (MLIF) protects against ischaemic stroke by lowering MAPK10 (JNK3) and decreasing proinflammatory cytokines(Reference Lv, Zhu and Li29). The DLG2 gene encoding postsynaptic density protein-93 (PSD-93) and its inhibition activates nuclear factor erythroid 2-related factor 2 (Nrf2)/antioxidant response element (ARE) antioxidant signalling in oxygen-glucose deprivation and reoxygenation (OGD/R)-exposed neurons(Reference Zhang, Qian and Qian30). DLG2 can be an essential regulator of neuroprotection through the antioxidant system during an ischaemic stroke(Reference Zhang, Qian and Qian30). Although CYP1A1_rs143070677 was not included in 6- and 7-SNP models, it was only a missense mutation in selected ten SNP. The food components were screened CYP1A1_rs143070677 wild and mutated types. The activity of both wild and mutated types could be modulated with food components. CYP1A1 is an extrahepatic monooxygenase involved in the metabolism of endogenous substrates and drugs and activating certain toxins and environmental pollutants, suggesting the elimination of toxins plays a pivotal role in stroke incidence. Therefore, the genes and the associated proteins identified for this study are linked to brain and nervous system regulation, inflammation, toxin elimination and oxidative stress and their expressions might be involved in stroke risk.
Although obesity, hypertension, diabetes, dyslipidaemia and smoking are well-known risk factors for stroke, the prevalence of these conditions did not vary significantly between the stroke and non-stroke groups. Few studies have reported a significant association between genetic variants and lifestyle factors in stroke prevalence. In a Chinese study, the aldehyde dehydrogenase 2 (ALDH2)_rs671 variant was found to interact with alcohol consumption, influencing stroke chance (Meta-P = 3·39 × 10–17)(Reference Shi, Kelly and Zhu31). In the present study, the PRS indicated that the interaction between genetics and several lifestyle factors may modulate stroke chance. Energy, CHO, n-3 fatty acids and BCAA intake influenced the chances of stroke occurrence. Among the dietary patterns, RMD interacted with genetic risk factors for stroke. The genetic impact was more significant with a high energy intake than a low one. The stroke prevalence in the participants with medium-PRS and high-PRS was much higher in the high-energy intake than in the low-energy intake group. Moreover, stroke prevalence was much higher in the participants with a high PRS having a high CHO intake than those having a low CHO intake. Since the participants in the RMD group had a high CHO intake, the RMD group showed a trend similar to that of a high CHO diet. The results suggested that although dietary patterns and nutrient intake did not impact stroke prevalence, they influenced it, according to the PRS.
The strength of this study was the multifaceted approach to investigating metabolic and dietary factors, thus providing a comprehensive assessment of their relationships with the chances of stroke occurrence in a large hospital-based cohort. The study also included various lifestyle factors, such as alcohol consumption, exercise and smoking, providing a holistic view of their potential associations with the chances of stroke. The study explored the interaction between genetic predisposition and specific dietary components, offering valuable insights into how lifestyle factors can modify the genetic chance of stroke. The study also had several limitations as follows: the study could not present a cross-sectional analysis, which limits the ability to establish causality or infer the temporal relationship between variables. Further, the application of the study findings could be limited to Asians. The type of stroke among the participants was not specified as ischaemic or haemorrhagic stroke. However, considering Korean stroke prevalence and post-stroke complication severity, the cohort was believed to include ischaemic stroke patients primarily.
In conclusion, this study investigated the intricate interplay between genetic predisposition and lifestyle-related risk factors on the likelihood of stroke occurrence. We identified the specific SNP associated with stroke chance through a comprehensive GWAS and subsequent analyses. Our findings suggest that a combination of genetic factors, particularly those related to IL-18 and the IFN-γ pathway, significantly influences an individual’s susceptibility to stroke. Interestingly, we observed that lifestyle choices, particularly dietary components, can modulate the impact of genetic predisposition to stroke. The interaction between genetic variants and specific dietary elements, including energy, carbohydrates, n-3 fatty acids and BCAA, is crucial in determining the chances of stroke. Tailoring dietary patterns based on these interactions may provide an effective stroke prevention and management strategy. Understanding these genetic and lifestyle interactions is paramount for devising personalised prevention and intervention strategies, thereby improving outcomes and reducing the burden of stroke on individuals and the healthcare systems.
Acknowledgements
We appreciate the participants involved in a city hospital-based study of KoGES.
This study was supported by a grant from the National Research Foundation of Korea (NRF) funded by the Ministry of Science and ICT (RS-2023-00208567).
S. P.: conceptualisation, formal analysis and writing – original draft preparation. S.P.: resources, methodology, formal analysis, writing – review and editing and funding acquisition. S.P has read and agreed to the published version of the manuscript.
The authors declare that the research was conducted without any commercial or financial relationships that could be construed as a potential conflict of interest.
Supplementary material
For supplementary material/s referred to in this article, please visit https://doi.org/10.1017/S0007114524000394