Introduction
RNA’s biological relevance has traditionally been ascribed to its role as an intermediate in the flow of genetic information from DNA to the production of functional proteins. However, it has become increasingly evident that RNA has many functional and regulatory roles in all domains of life. For instance, RNA molecules can regulate gene expression directly or by interacting with small organic molecules (miRNA, Pasquinelli et al., Reference Pasquinelli, Hunter and Bracht2005; riboswitches, Serganov and Nudler, Reference Serganov and Nudler2013, respectively), and can exert enzymatic activity (ribozymes, Doudna and Cech, Reference Doudna and Cech2002). Therefore, being involved in many cellular pathways, RNA molecules offer opportunities as targets for the development of therapeutic strategies (Shortridge and Varani, Reference Shortridge and Varani2015; Connelly et al., Reference Connelly, Moon and Schneekloth2016; Matsui and Corey, Reference Matsui and Corey2017; Warner et al., Reference Warner, Hajdin and Weeks2018; Falese et al., Reference Falese, Donlic and Hargrove2021). However, most of the drug discovery efforts have focused on proteins, which have long been known to modulate cellular activity. Nevertheless, in the human genome only a small fraction of the transcribed RNA is translated into proteins (Fig. 1) (Warner et al., Reference Warner, Hajdin and Weeks2018; ENCODE Project Consortium, 2012; Oliver et al., Reference Oliver, Mallet, Gendron, Reinharz, Hamilton, Moitessier and Waldispühl2020), and only a small portion of these proteins has been successfully targeted (Warner et al., Reference Warner, Hajdin and Weeks2018). The growing characterisation of noncoding RNA, therefore, offers new opportunities for novel therapeutic approaches, which may be particularly valuable when seeking alternatives in cases of drug resistance or when traditionally undruggable protein targets are encountered.

Fig. 1. The targetable portions of the human genome. More than 70% of the human genome is transcribed into RNA, but only a small portion of this encodes for, and is thus translated into, proteins (red slice) (ENCODE Project Consortium, 2012; Oliver et al., Reference Oliver, Mallet, Gendron, Reinharz, Hamilton, Moitessier and Waldispühl2020), of which, only a small fraction has been successfully targeted with drugs (Warner et al., Reference Warner, Hajdin and Weeks2018). The possibility of targeting non-coding functional RNA molecules (green slice) could significantly increase the number of drug discovery strategies.
Computational approaches have reached the status of standard tools in drug discovery campaigns (Macalino et al., Reference Macalino, Gosu, Hong and Choi2015). This is particularly true for structure-based drug design (Jorgensen, Reference Jorgensen2004), where the macromolecule’s structural information is used to find small molecules able to bind and modulate its activity. These computer-aided approaches have mostly been used to identify drugs for protein targets, as RNA has only recently been fully recognised as a relevant pharmaceutical target. Moreover, RNA targets are particularly challenging for standard computational approaches due to their complex structural dynamics and high charge density. Indeed, the applicability of standard approaches to RNA targets is still being debated and is a hot topic for research (Fedorova et al., Reference Fedorova, Jagdmann, Adams, Yuan, Van Zandt and Pyle2018; Warner et al., Reference Warner, Hajdin and Weeks2018; Juru and Hargrove, Reference Juru and Hargrove2021; Manigrasso et al., Reference Manigrasso, Marcia and De Vivo2021).
In this Perspective article, we put ourselves in the shoes of computational medicinal chemists who have experience in targeting proteins and who wish to extend their expertise to RNA to find potent and selective small-molecule ligands. We discuss critical aspects associated with this transition, which may require additional operations or some rethinking of standard procedures. The Perspective is divided into three sections that reflect the main challenges of computational RNA-targeted drug discovery (Fig. 2). First, we address RNA-small molecule recognition, particularly how RNA dynamics should be treated and how to identify potential binders. Second, we address small-molecule selectivity for RNA targets. Third, we address RNA binders and their expected physicochemical properties. We conclude with an outlook on future opportunities for computational medicinal chemists on the way to a wider playground, where to apply and expand their expertise.

Fig. 2. RNA-targeted computational drug discovery. A schematic representation of the Perspective’s three main sections: structural dynamics of the target in RNA-ligand recognition (top), target selectivity (left) and physicochemical properties of RNA binders (right).
RNA-small molecule recognition
Rational drug discovery campaigns typically start with a biomolecular target that has been pre-clinically validated. Validated targets play a critical role in a physio-pathological process and their modulation is likely to produce a therapeutic effect, hopefully within an acceptable safety window. Drug discovery pipelines, therefore, begin by identifying small-molecule hits for a validated target (Hughes et al., Reference Hughes, Stephen Rees and Philpott2011). In a structure-based context, computational strategies for this stage include fragment-based approaches (Erlanson et al., Reference Erlanson, Fesik, Hubbard, Jahnke and Jhoti2016), de novo design (Schneider and Fechner, Reference Schneider and Fechner2005), and, most importantly, virtual screening of small-molecule libraries (Maia et al., Reference Maia, Assis, De Oliveira, Da Silva and Taranto2020). In a virtual screening campaign, a small-molecule library undergoes molecular docking, a procedure that aims at predicting how the ligands bind to the target. This computational approach is rapid and can be used to roughly discriminate between binders and non-binders. As such, it has become a well-established strategy for the identification of small-molecule hits.
There are two particularly critical aspects involved in extending docking protocols, that have long been refined over proteins, to RNA targets: i) how to describe the target’s structure, and in particular how to account for its intrinsic flexibility and structural dynamics, and ii) how to assess quantitatively the binding poses. These aspects are already critical in the context of protein targets but may be even more crucial for RNA targets. Concerning the former aspect, any docking campaign requires the target’s structure as the starting point. Experimental methods to reconstruct the 3D structures at the atomistic level include X-ray crystallography, nuclear magnetic resonance (NMR), and cryo-electron microscopy (Palamini et al., Reference Palamini, Canciani and Forneris2016). In the absence of experimental data, modelling approaches can be taken advantage of to reconstruct the target’s structure with varying degrees of accuracy, depending on the available information (Hameduh et al., Reference Hameduh, Haddad, Adam and Heger2020). In this respect, the machine-learning based approach AlphaFold (Jumper et al., Reference Jumper, Evans, Pritzel, Green, Figurnov, Ronneberger, Tunyasuvunakool, Bates, Žídek and Potapenko2021) is achieving impressive results in protein 3D-structure prediction, however, an equivalent for RNA does not exist yet. For protein targets, X-ray crystallography experiments have long been established as an efficient method to produce high-resolution structures. Therefore, a docking effort on a protein target typically begins with a crystal structure. In contrast, a significant fraction (about 40%) of RNA structures is solved via NMR (Barnwal et al., Reference Barnwal, Yang and Varani2017), while crystal structures can be often found in the cases of larger and structurally complex RNA molecules.
While a reliable structure is certainly a great starting point, it may however not suffice for a comprehensive description of the target of interest. Indeed, biomolecules are not frozen entities, but rather present in solution various degrees of structural flexibility. This dynamic nature not only can influence the binding of molecular partners, but structural modifications can also be triggered upon the binding of smaller molecules. Therefore, including information about the dynamics of the target during a docking procedure can depict more realistically what really occurs at the molecular level and potentially improve results. This is a long-standing issue in computational drug discovery (Feixas et al., Reference Feixas, Lindert, Sinko and McCammon2014). Indeed, protein flexibility is typically addressed in docking protocols through different approaches (Buonfiglio et al., Reference Buonfiglio, Recanatini and Masetti2015), such as soft-docking (Ferrari et al., Reference Ferrari, Wei, Costantino and Shoichet2004) and induced-fit docking (Sherman et al., Reference Sherman, Day, Jacobson, Friesner and Farid2006). These strategies can be considered an integral part of the docking protocol. While they do not usually affect the performance of the docking calculation much, they do account for a rather limited structural flexibility of the target. An alternative approach is to include the receptor dynamics as an ensemble of multiple conformations (Huang and Zou, Reference Huang and Zou2007; Amaro et al., Reference Amaro, Baudry, Chodera, Demir, McCammon, Miao and Smith2018). This strategy allows greater conformational changes of the target, but the docking calculation scales up linearly as the procedure must be iterated for each of the structures in the ensemble (Fig. 3).

Fig. 3. Ensemble docking. An ensemble comprising multiple conformations of the target is included to take into account its structural dynamics. The docking calculation (virtual screening for large libraries) is repeated for each structure in the ensemble. The RNA structures here belong to the conformational ensemble of the transactivation response element (TAR) RNA from human immunodeficiency virus type-1 reconstructed in Salmon et al. (Reference Salmon, Bascom, Andricioaei and Al-Hashimi2013).
From a practical standpoint, the ensemble is generated separately from the docking calculation using other computational methods. This ensemble docking approach has been used for protein targets (Amaro et al., Reference Amaro, Baudry, Chodera, Demir, McCammon, Miao and Smith2018). Compared to common protein targets, RNA molecules display marked and complex structural dynamics (Ganser et al., Reference Ganser, Kelly, Herschlag and Al-Hashimi2019). Therefore, the ensemble docking approach appears as the natural choice and should indeed be preferred for RNA targets. The literature contains some successful examples in this direction (Stelzer et al., Reference Stelzer, Frank, Kratz, Swanson, Gonzalez-Hernandez, Lee, Andricioaei, Markovitz and Al-Hashimi2011; Ganser et al., Reference Ganser, Lee, Rangadurai, Merriman, Kelly, Kansal, Sathyamoorthy and Al-Hashimi2018).
A diverse set of computational approaches can be used to generate RNA conformational ensembles. In particular, static frameworks could be employed to generate a pool of RNA structures, as it is done through the popular Fragment Assembly of RNA with Full-Atom Refinement (FARFAR) algorithm in the Rosetta software suite (Watkins et al., Reference Watkins, Rangan and Das2020). In contrast, methods that mimic the dynamics, such as molecular dynamics (MD) simulations, can be employed in the generation of a conformational ensemble (Sponer et al., Reference Sponer, Bussi, Krepl, Banáš, Bottaro, Cunha, Gil-Ley, Pinamonti, Poblete and Jurečka2018). MD explores the conformational dynamics of biomolecules under realistic conditions (e.g., explicit solvent, quasi-physiological ionic concentrations) and has become an indispensable tool for investigating mechanistic features at the atomistic level (De Vivo et al., Reference De Vivo, Masetti, Bottegoni and Cavalli2016; Decherchi and Cavalli, Reference Decherchi and Cavalli2020). Notably, the results of MD simulations strongly depend on the ability of the underlying model (i.e., the force field) to capture the physics of the interactions in molecular systems. Since structural biology and drug discovery have long been focused on proteins, force fields for RNA have developed at a much slower pace (Table 1).
Table 1. Main classes of RNA force fields and their major variants
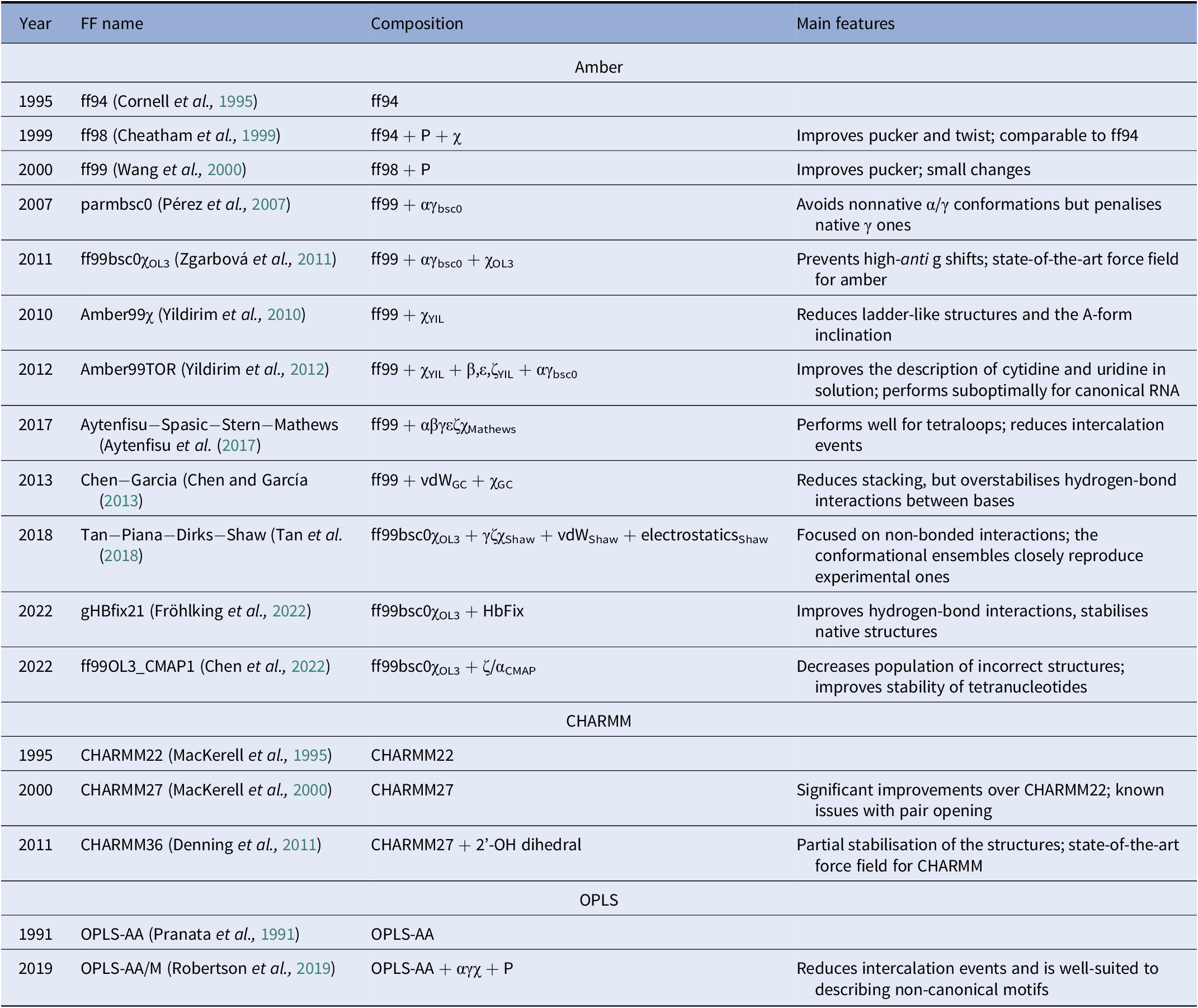
Among the Amber family of force fields, the ff99 force field combined with the bsc0 and χOL3 refinements is considered state-of-the-art, as it is the most validated and widely used (Wang et al., Reference Wang, Cieplak and Kollman2000; Pérez et al., Reference Pérez, Marchán, Svozil, Sponer, Cheatham, Laughton and Orozco2007; Zgarbová et al., Reference Zgarbová, Otyepka, Šponer, Mládek, Banáš, Cheatham and Jurecka2011). In particular, ff99 (Wang et al., Reference Wang, Cieplak and Kollman2000) is a major branch of Amber force fields that includes parameters for both proteins and nucleic acids and was based on the previous ff94 version (Cornell et al., Reference Cornell, Cieplak, Bayly, Gould, Merz, Ferguson, Spellmeyer, Fox, Caldwell and Kollman1995). For DNA and RNA, the main difference between ff94 and ff99 is the refinement of the sugar puckering and χ dihedral parameters. In 2007, Orozco and coworkers introduced a major correction, known as bsc0, where the α and γ dihedral angles of the nucleic acid backbone were modified to avoid the formation of nonnative γ-trans backbone dihedral states, thus reducing unrealistic helical twists in A-RNA (Pérez et al., Reference Pérez, Marchán, Svozil, Sponer, Cheatham, Laughton and Orozco2007). In 2011, the χOL3 refinement involved a reparameterisation of the χ dihedral to prevent high-anti γ shifts in RNA, which led to entirely untwisted and ladder-like structures (Zgarbová et al., Reference Zgarbová, Otyepka, Šponer, Mládek, Banáš, Cheatham and Jurecka2011). In more recent attempts to improve the force field, dihedral reparameterisation was conducted in the Mathews group (Aytenfisu et al., Reference Aytenfisu, Spasic, Grossfield, Stern and Mathews2017), while dihedral, electrostatic and van der Waals parameters were considered by the Shaw group (Tan et al., Reference Tan, Piana, Dirks and Shaw2018). Recently, different schemes were also proposed, which used an additional term to better describe hydrogen-bond interactions (Fröhlking et al., Reference Fröhlking, Mlýnský, Janeček, Kührová, Krepl, Banáš, Šponer and Bussi2022) or introduced the grid-based energy correction map (CMAP) term in the context of RNA force fields (Chen et al., Reference Chen, Liu, Cui, Li and Chen2022). The ability of the CHARMM and OPLS families of force fields to describe RNA structures is also gradually being improved (see Table 1) (Denning et al., Reference Denning, Priyakumar, Nilsson and Mackerell2011; Robertson et al., Reference Robertson, Qian, Robinson, Tirado-Rives and Jorgensen2019). In addition to the force field, the thoroughness of conformational sampling is a common issue for both protein and RNA modelling. This is because the timescales that are accessible via conventional (or “plain”) MD simulations are still limited (in the order of tens of microseconds for most of the research projects). Nevertheless, outstanding results have been achieved thanks to hardware advances (Pande et al., Reference Pande, Baker, Chapman, Elmer, Khaliq, Larson, Rhee, Shirts, Snow and Sorin2003; Shaw et al., Reference Shaw, Grossman, Bank, Batson, Butts, Chao, Deneroff, Dror, Even and Fenton2014) and sophisticated enhanced sampling approaches (Abrams and Bussi, Reference Abrams and Bussi2013; Mlýnský and Bussi, Reference Mlýnský and Bussi2018) being implemented in popular instruments such as PLUMED (Tribello et al., Reference Tribello, Bonomi, Branduardi, Camilloni and Bussi2014). In the context of proteins, particularly challenging systems in terms of conformational sampling are intrinsically disordered proteins (Habchi et al., Reference Habchi, Tompa, Longhi and Uversky2014). In this respect, enhanced sampling methods were successfully employed (Granata et al., Reference Granata, Baftizadeh, Habchi, Galvagnion, De Simone, Camilloni, Laio and Vendruscolo2015; Palazzesi et al., Reference Palazzesi, Prakash, Bonomi and Barducci2015; Bernetti et al., Reference Bernetti, Masetti, Pietrucci, Blackledge, Jensen, Recanatini, Mollica and Cavalli2017; Masetti et al., Reference Masetti, Bernetti and Cavalli2020), and we thus envision that they will find increasing application also on RNA molecules.
Despite recent improvements, the current force fields still bear some limits. Combined with the shortcomings linked to the sampling, they can generate RNA conformational ensembles that do not entirely agree with experiments. While this is problematic in general, it may become particularly critical when using the generated structural ensembles for docking. However, experimental information can be used to generate more reliable ensembles (Pitera and Chodera, Reference Pitera and Chodera2012; Hummer and Köfinger, Reference Hummer and Köfinger2015; Bonomi et al., Reference Bonomi, Camilloni, Cavalli and Vendruscolo2016, Reference Bonomi, Heller, Camilloni and Vendruscolo2017; Cesari et al., Reference Cesari, Reißer and Bussi2018; Orioli et al., Reference Orioli, Larsen, Bottaro and Lindorff-Larsen2020) in both static (Shi et al., Reference Shi, Rangadurai, Assi, Roy, Case, Herschlag, Yesselman and Al-Hashimi2020) and dynamic frameworks (Bottaro et al., Reference Bottaro, Bussi, Kennedy, Turner and Lindorff-Larsen2018). During MD simulations, for example, experimental data can be included on the fly to guide the sampling towards regions of the conformational space that are supported by experiments. Alternatively, reweighting approaches can be applied after the MD simulation to identify those conformations that better agreed with the experimental data. Both strategies have been successfully applied to reconstruct reliable conformational ensembles of RNA molecules (Borkar et al., Reference Borkar, De Simone, Montalvao and Vendruscolo2013; Bottaro et al., Reference Bottaro, Bussi, Kennedy, Turner and Lindorff-Larsen2018). Notably, the experimental data here can come from a broader range of sources than the data on the initial atomistic structures. Indeed, any experimental information that can be related to an observable computed from the biomolecule coordinates in the MD trajectory can be exploited. Thus, experimental methods that provide coarser structural information (e.g., small-angle X-ray scattering, SAXS) are extremely valuable and have been used for this purpose (Bernetti et al., Reference Bernetti, Hall and Bussi2021). Finally, clustering algorithms that have become of routine use in the context of MD simulations (Bernetti et al., Reference Bernetti, Bertazzo and Masetti2020) can be of remarkable support to select representative structures from MD-generated ensembles for the subsequent docking/virtual screening stage.
Given a biomolecular target’s structure or ensemble of structures, the docking procedure attempts to find plausible ligand-target bound configurations, that is, binding modes (or “poses”) with overall favourable interactions between the two binding partners. We here briefly outline some popular docking software packages and refer the interested reader to a recent comprehensive overview (Zhou et al., Reference Zhou, Jiang and Chen2021). Glide (Friesner et al., Reference Friesner, Banks, Murphy, Halgren, Klicic, Mainz, Repasky, Knoll, Shelley and Perry2004), GOLD (Jones et al., Reference Jones, Willett, Glen, Leach and Taylor1997) and AutoDock Vina (Trott and Olson, Reference Trott and Olson2010) are software packages that were devised for protein targets and that can also be used for RNA when needed. Conversely, AutoDock (Morris et al., Reference Morris, Goodsell, Halliday, Huey, Hart, Belew and Olson1998), DOCK 6 (Lang et al., Reference Lang, Brozell, Mukherjee, Pettersen, Meng, Thomas, Rizzo, Case, James and Kuntz2009) and ICM (Abagyan et al., Reference Abagyan, Totrov and Kuznetsov1994) have been improved to be used with RNA through dedicated docking protocols, optimised ligand-sampling algorithms, or the inclusion of solvation effects either in the generation of poses or in the scoring functions. Finally, the software packages MORDOR (Guilbert and James, Reference Guilbert and James2008), rDOCK (Ruiz-Carmona et al., Reference Ruiz-Carmona, Alvarez-Garcia, Nicolas Foloppe, Juhos, Schmidtke, Barril, Hubbard and Morley2014), and the very recent RLDOCK (Sun et al., Reference Sun, Jiang, Zhou and Chen2020) and NLDock (Feng et al., Reference Feng, Zhang, Wu and Huang2021) were specifically developed for RNA docking, reflecting the growing interest in RNA-oriented drug discovery. The trend that emerges from the validation of the latter approaches on diverse datasets of experimental RNA-ligand complexes, usually evaluated as the success rate in reproducing experimental binding poses, is that RNA-specific methods outperform the tools developed for proteins or generic macromolecules (Feng et al., Reference Feng, Zhang, Wu and Huang2021; Zhou et al., Reference Zhou, Jiang and Chen2021). Notably, such trend highlights the relevance of specifically considering interaction and structural features that are peculiar of RNA-ligand binding.
The quality of the poses identified by the docking procedure can be assessed through a variety of strategies that fall under the term “scoring functions”. These can be broadly classified as the follows: i) knowledge-based, when the scoring is built upon information extracted from known three-dimensional structures of target-ligand complexes; ii) physics-based, when the scoring is based on force fields or simplified empirical functions of the target-ligand interactions and iii) machine learning (ML)-based, when the scoring is evaluated through ML models trained on available experimental data (Zhou et al., Reference Zhou, Jiang and Chen2021). Scoring functions are typically included in docking software. However, there has been a rapid growth in standalone options, including the knowledge-based ITScore-NL scoring function (Feng and Huang, Reference Feng and Huang2020) and the ML-based RNAPosers (Chhabra et al., Reference Chhabra, Xie and Frank2020), RNAmigos (Oliver et al., Reference Oliver, Mallet, Gendron, Reinharz, Hamilton, Moitessier and Waldispühl2020) and AnnapuRNA (Stefaniak and Bujnicki, Reference Stefaniak and Bujnicki2021) scoring functions. Notably, MD simulations can also be used for scoring (Menchon et al., Reference Menchon, Maveyraud and Czaplicki2018). Indeed, binding pose stability can be assessed with plain MD runs or, alternatively, binding affinities can be estimated with more computationally intensive MD-based free energy calculations (Decherchi and Cavalli, Reference Decherchi and Cavalli2020). These procedures can straightforwardly be applied to RNA targets. The only caveats are the accuracy of the force field and, most importantly, the limited number of RNA-ligand complexes that can typically be managed via these approaches, which hinders their use for large-scale virtual screenings.
Selectively targeting RNA structures
Most functional proteins targeted in drug discovery campaigns fold in a well-defined native state under physiological conditions. The functional activity of proteins usually takes place in surface cavities. In structure-based approaches, structural information about these cavities is directly used to design ligands that might bind there (Pérot et al., Reference Pérot, Sperandio, Miteva, Camproux and Villoutreix2010). If the target of interest is well-characterised, relevant binding sites may already be known. Often, however, either the binding site is unknown or alternative binding sites may be sought (e.g., for allosteric modulation) (Kuzmanic et al., Reference Kuzmanic, Bowman, Juarez-Jimenez, Michel and Gervasio2020). The possibility of identifying binding pockets by computational means is thus integral to modern structure-based drug design. The SiteMap tool of the Schrödinger suite (Halgren, Reference Halgren2009), the ICM PocketFinder (An et al., Reference An, Totrov and Abagyan2005), the NanoShaper software suite (Decherchi and Rocchia, Reference Decherchi and Rocchia2013) and its dynamic extension Pocketron (La Sala et al., Reference La Sala, Decherchi, De Vivo and Rocchia2017) are popular options in this regard (Pérot et al., Reference Pérot, Sperandio, Miteva, Camproux and Villoutreix2010). These tools have been widely employed to detect pockets in proteins. Although some of them have also been used to identify pockets in RNA molecules (Ganser et al., Reference Ganser, Lee, Rangadurai, Merriman, Kelly, Kansal, Sathyamoorthy and Al-Hashimi2018; Hewitt et al., Reference Hewitt, Calabrese and Schneekloth2019; Panei et al., Reference Panei, Torchet, Menager, Gkeka and Bonomi2022), an extensive exploration of their applicability is still missing. In proteins, suitable binding pockets have a well-defined 3D organisation of the amino acids comprised therein, which, alongside their composition and variability, display a wealth of physicochemical features (e.g., balance between hydrophobic/hydrophilic regions, solvent exposure and shape) that make the pockets rather distinctive. Taken together, these aspects encode, to a certain extent, the target selectivity that can potentially be achieved by addressing that site (Ehrt et al., Reference Ehrt, Brinkjost and Koch2016; Smilova et al., Reference Smilova, Curran, Radoux, von Delft, Cole, Bradley and Marsden2022). Usually, however, this information is not extensively exploited in the early stages of the drug discovery pipeline, where most of the effort is directed towards the identification of potential hits. Conversely, it is only during later phases of lead optimisation that target selectivity is fully explored, together with chemical modifications that can improve the affinity and ADMET (absorption, distribution, metabolism, excretion and toxicity) properties.
Moving into the context of RNA, molecules with functional roles in cellular pathways display a markedly heterogeneous structural complexity. Indeed, functional RNA molecules vary from short hairpin loops and miRNA, to tRNA, riboswitches and larger ribozymes, up to the scale of the ribosome (Ganser et al., Reference Ganser, Kelly, Herschlag and Al-Hashimi2019). From a structure-based drug discovery perspective, we can broadly distinguish two main scenarios depending on the complexity of the RNA target’s molecular structure.
In the first scenario, relatively simple secondary structure elements can be identified as hot spots for small-molecule binding. These short stem-loop motifs include for instance apical loops, bulges and internal loops, and have been extensively studied in this regard (Liu et al., Reference Liu, Thomas and Hergenrother2004; Disney and Childs-Disney, Reference Disney and Childs-Disney2007; Meyer and Hergenrother, Reference Meyer and Hergenrother2009). Here, the structure-based strategy is made possible by the unpaired nucleobases, which can arrange in characteristic structures and thus offer the possibility of small-molecule binding (Juru and Hargrove, Reference Juru and Hargrove2021). However, their pockets are usually shallow or relatively small, which makes it challenging to find high-affinity binders that produce specific interactions (Fig. 4) (Warner et al., Reference Warner, Hajdin and Weeks2018; Juru and Hargrove, Reference Juru and Hargrove2021). Indeed, shallow pockets pose a biophysical limit to how potent non-irreversible binders can be. Furthermore, this strategy may prove particularly arduous in terms of selectivity because similar secondary structure elements can be found across diverse RNA molecules. Indeed, only modest potency and selectivity have been achieved in reported studies focused on these types of RNA molecules (Warner et al., Reference Warner, Hajdin and Weeks2018). However, this strategy may be particularly valuable and viable in the absence of a more complex tertiary structure, which offers more characteristic architectures for potential drug binding (Juru and Hargrove, Reference Juru and Hargrove2021).

Fig. 4. Protein and RNA binding pockets. Binding pockets in proteins (left, riboflavin kinase, PDBID: 1NB9) are typically highly structured. In RNAs, structured pockets like those in proteins are found in highly folded structures (right, FMN riboswitch, PDBID: 3F4G). In contrast, relatively simple RNA structures (middle, HIV TAR, PDBID: 1QD3) usually offer shallow or relatively small pockets, which are more challenging to target with small molecules. The pockets shown herein (in violet) were identified with the NanoShaper software (Decherchi and Rocchia, Reference Decherchi and Rocchia2013). The two RNA structures were chosen as representatives of good and intermediate quality pockets from the examples reported by Warner et al. (Reference Warner, Hajdin and Weeks2018): in this work, pocket quality was estimated using the ICM tool PocketFinder (An et al., Reference An, Totrov and Abagyan2005), where pockets of larger size and buriedness resulted in higher quality.
The second scenario involves RNA molecules achieving a higher level of folding and thus complex 3D structures. Although RNA molecules have less chemical variety than proteins (4 nucleotides vs 21 amino acids), this complex folding can nevertheless produce distinctive cavities reminiscent of protein-like binding pockets (Warner et al., Reference Warner, Hajdin and Weeks2018; Hewitt et al., Reference Hewitt, Calabrese and Schneekloth2019). These cavities can be suitable for computational approaches already established in the context of protein targets. In such cases, the determinants of RNA-ligand recognition are likely to be similar to those of protein-ligands, with no need to develop alternative RNA-centric approaches (Fedorova et al., Reference Fedorova, Jagdmann, Adams, Yuan, Van Zandt and Pyle2018). Riboswitches and ribozymes are remarkable examples of highly folded RNA molecules with complex tertiary structures (Zafferani and Hargrove, Reference Zafferani and Hargrove2021). Since those interact with metabolites or substrates, they are already prone to ligand recognition in pre-formed binding pockets. Multi-junctions and pseudoknots, in general, have also been suggested as promising RNA species for RNA-targeted drug discovery because their great structural complexity is suitable for pocket formation (Warner et al., Reference Warner, Hajdin and Weeks2018). Despite this, to the best of our knowledge, the literature contains just one report of a successful campaign of rational drug design using classical, established medicinal chemistry protocols (Fedorova et al., Reference Fedorova, Jagdmann, Adams, Yuan, Van Zandt and Pyle2018). This work used high-throughput experimental assays to identify hits. However, computational approaches could also be employed for hit identification of these RNA molecules, so compound libraries could potentially be investigated on a much larger scale.
Given this overall picture, at variance with protein targets, the discourse around selectivity becomes more urgent already at an earlier stage when targeting RNA molecules. This is because the choice of a certain class of RNA targets can greatly impact the level of selectivity that can be achieved. While the second scenario discussed for RNA appears to hold more promise for identifying selective binders to modulate RNA activity, this may however preclude opportunities to develop effective drugs for pathological conditions mediated by structurally simpler RNAs (Juru and Hargrove, Reference Juru and Hargrove2021). We, therefore, encourage researchers to be open to both scenarios while considering their respective implications for selectivity.
Properties of RNA ligands
To identify drug candidates, it is essential to know the physicochemical features that ligands should possess in order to bind to a particular class of biomolecular targets. In computational drug discovery, this knowledge can be instrumental to design libraries for virtual screening or to guide the lead optimisation stage of a candidate. For proteins, drug discovery usually aims to identify small organic molecules with physicochemical profiles that meet the criteria of oral drugs, including solubility, bioavailability, cell and tissue permeability, chemical stability and absence of toxicity. In this respect, Lipinski’s rule of five is the established guiding principle for rational drug design (Lipinski, Reference Lipinski2004). Indeed, through a retrospective analysis of approved drugs and drug candidates, Lipinski’s rule of five empirically set the drug-likeness boundaries for physicochemical parameters including molecular weight, lipophilicity and number of hydrogen-bond donors and acceptors. This, in conjunction with the wealth of knowledge accumulated through years of experience in the field, has led to the identification of a rather defined chemical space that is characteristic of protein-targeting small organic molecules. Similarly, the knowledge gained in decades of successes and failures in drug discovery campaigns has allowed to compile a list of undesirable chemical features that for several reasons (mostly non-specific interference with biological assays) should not be possessed by drugs, the so-called PAINS (Baell and Holloway, Reference Baell and Holloway2010). In structure-based virtual screening, Lipinski’s rule of five and PAINS filters are typically applied before the molecular docking. This is to avoid wasting time on performing docking calculations of molecules that will likely be discarded anyway, regardless of their ability to bind to the target.
For RNA targets, the chemical space of small-molecule binders has not been fully characterised yet. Since relatively few small organic molecule binders of RNA targets are known, their expected properties are not yet established and are a hot research topic (Warner et al., Reference Warner, Hajdin and Weeks2018; Juru and Hargrove, Reference Juru and Hargrove2021). Early identified ligands that acted by binding RNA had a positive net charge and were able to intercalate between RNA bases (Thomas and Hergenrother, Reference Thomas and Hergenrother2008; Guan and Disney, Reference Guan and Disney2012). However, such physicochemical properties cause non-specific binding on the negatively charged RNA backbone, yielding low selectivity. For this reason, ligands may display a relatively high level of toxicity, therefore often resulting non-viable. Recently, research efforts based on the analysis of ligands with activities towards RNA were directed to the characterisation of the physicochemical space of RNA small-molecule binders (Morgan et al., Reference Morgan, Forte, Culver, Zhang and Hargrove2017, Reference Morgan, Sanaba, Donlic, Karloff, Forte, Zhang and Hargrove2019; Haniff et al., Reference Haniff, Knerr, Liu, Crynen, Boström, Abegg, Adibekian, Lekah, Wang and Cameron2020; Rizvi et al., Reference Rizvi, Maria, Nahvi, Klappenbach, Klein, Curran, Richards, Chamberlin, Saradjian and Burchard2020). The overall picture that is gradually emerging points to an RNA-privileged chemical space. The most peculiar structural features identified in RNA-targeting ligands appear to be a higher nitrogen count, a lower oxygen count, an enrichment in aromatic rings, fewer stereocenters and fewer sp3-hybridised carbon atoms (Morgan et al., Reference Morgan, Sanaba, Donlic, Karloff, Forte, Zhang and Hargrove2019; Haniff et al., Reference Haniff, Knerr, Liu, Crynen, Boström, Abegg, Adibekian, Lekah, Wang and Cameron2020). Additionally, in contrast to the more heterogeneous spatial arrangements of the approved drugs, a prevalence of rod- and planar-like shapes (Wirth and Sauer, Reference Wirth and Sauer2011) has also been observed. Interestingly, we note how, as a whole, these features are reminiscent of the nucleobases. Most remarkably, this RNA-privileged chemical space appears to be a subset of the space traditionally occupied by protein-binding ligands and, more generally, by orally administered drugs (Fig. 5) (Juru and Hargrove, Reference Juru and Hargrove2021). An interesting implication of this latter aspect is that there is a real potential for these molecules to be RNA-targeting therapeutics. From a more practical perspective, the identified characteristics represent indispensable instruments for computational medicinal chemists to refine screening libraries and to guide the optimisation of promising binders.

Fig. 5. The chemical space of RNA-binding ligands. Bioactive RNA-targeted compounds populate a region in chemical space (here projected along two hypothetical principal components of cheminformatic parameters) occupied by FDA-approved drugs, which mostly target proteins (Juru and Hargrove, Reference Juru and Hargrove2021). Therefore, while RNA ligands have particular structural and shape properties, they can also possess the typical drug-like properties.
While it is essential to take advantage of this knowledge, the number of known RNA binders is still limited, so the exploration and definition of the chemical space of RNA-binding ligands has only just begun. Therefore, further advances may come into play and refine the current picture in the (possibly near) future. Indeed, as a further level of complexity, recently discovered potent and selective ligands of an RNA ribozyme contained chemical groups that would usually be classified as PAINS (Fedorova et al., Reference Fedorova, Jagdmann, Adams, Yuan, Van Zandt and Pyle2018). This highlights how critical it can be to apply rules of thumb, which were developed to target proteins, for RNA targeting, since the ligands’ chemical space is still under construction. Given our limited knowledge, it is therefore important to build screening libraries by taking a non-RNA-biased approach, increasing chemical diversity and maintaining drug-likeness.
In conclusion, we are still in the process of comprehensively characterising the physicochemical properties of RNA binders. Therefore, while the boundaries of their features are gradually becoming clearer, room for potential expansion in this respect should nevertheless be contemplated.
Outlook and concluding remarks
The growing recognition of RNAs as promising pharmaceutical targets requires a mindset change in drug discovery. In this Perspective, we discussed the three main challenges of computational RNA-targeted drug discovery: i) the prominent role of target flexibility in predicting small-molecule binding; ii) the importance of achieving binding selectivity; and iii) the knowledge of the chemical space expected for RNA-binding drugs.
We have discussed how the currently available computational procedures, which have been optimised and refined over decades of efforts directed to protein targets, can be used in the novel and partially unexplored context of RNA targets, and how to take appropriate precautions. For example, recent scoring functions were specifically developed to describe the interaction of small molecules with RNA, which means that well-established docking protocols can be easily adapted to RNA targets. Moreover, we discussed how MD simulations, which are extensively used for lead optimisation in protein-based drug discovery, will become an essential tool for considering RNA target flexibility in the earlier stages of screening campaigns. Furthermore, experimental data can increase the reliability of RNA conformational ensembles reconstructed via MD. Interestingly, we note how experimental information often has a dual function when targeting RNA, since the experimental data can be used as the source of starting structures for the simulations and as a guide to refine the conformational ensembles. Moreover, MD simulations (possibly combined with enhanced sampling methods and appropriate analysis tools) may help identify suitable pockets to focus on in the search for specific interactions. In this respect, by showing a greater amount of structural complexity, RNA motifs such as riboswitches, ribozymes, multi-junctions and pseudoknots are intrinsically more inclined to pocket formation, and thus they are better suited for RNA-targeted discovery of small molecule drugs. Given the above and relative to traditional protein-based drug discovery, computational medicinal chemists may need a more skilled background in statistical mechanics and simulative methods in order to take full advantage of these approaches.
Once the chemical space of RNA-targeting drugs has been defined, machine learning and artificial intelligence, which are revolutionising several aspects of conventional drug discovery (Vamathevan et al., Reference Vamathevan, Clark, Czodrowski, Dunham, Ferran, Lee, Li, Madabhushi, Shah and Spitzer2019), will be critical to developing novel active compounds. Indeed, the most recently developed scoring functions for docking tend to be based on machine learning approaches, and artificial intelligence is already being leveraged to advance the area of force field improvement.
The landscape of currently available small-molecule drugs targeting RNA is somewhat limited. Current drugs are antibiotics targeting ribosomal RNA (rRNA), such as the synthetic oxazolidinone linezolid that acts by binding a highly structured pocket, and the very recent Roche and PTC Therapeutics’ risdiplam, used in the treatment of spinal muscular atrophy (SMA), which acts by stabilising the interaction between an RNA splice site and a small nuclear ribonucleoprotein (snRNP) (Sheridan, Reference Sheridan2021). Furthermore, Merck’s ribocil inhibits bacterial growth by binding to a bacterial riboswitch, however, this small molecule is on hold at the preclinical stage due to the rapid development of bacterial resistance and unlikely will be pursued further (Warner et al., Reference Warner, Hajdin and Weeks2018). Despite being limited in number, all these examples support the idea that RNA is a legitimate target of small molecules and highlights the potential of focusing on RNA molecules with high structural complexity to achieve high affinity and selectivity.
Finally, a mention is here required on other types of RNA molecules (not extensively covered in this contribution) as pharmaceutical targets, which are currently in the market/clinical trials for major unmet medical needs. Remarkable examples thereof are the recently approved risdiplam for SMA and the Novartis’s branaplam, under clinical trial for both SMA and Huntington disease, which act as splicing modulators by binding to pre-mRNA (Childs-Disney et al., Reference Childs-Disney, Yang, Gibaut, Tong, Batey and Disney2022).
In conclusion, time has come for computational drug discovery to embrace the potential of RNA to become an established drug target shortly. Indeed, thanks to the growing interest in discovering small molecules that target RNA, the field is gradually producing useful resources, such as the recent HARIBOSS database of RNA-small molecule structures (Panei et al., Reference Panei, Torchet, Menager, Gkeka and Bonomi2022). In the same spirit, efforts by the computational community in sharing simulation inputs via dedicated resources (e.g., the PLUMED-NEST initiative (Bonomi et al., Reference Bonomi, Bussi, Camilloni, Tribello, Banáš, Barducci, Bernetti, Bolhuis, Bottaro and Branduardi2019)), and sharing computational practises via Jupyter Notebooks (Kluyver et al., Reference Kluyver, Ragan-Kelley, Pérez, Granger, Bussonnier, Frederic, Kelley, Hamrick, Grout, Corlay, Ivanov, Avila, Abdalla, Willing, Loizides and Scmidt2016), are likely to accelerate the expansion of more complex and sectorial computational skills to successful drug discovery.
Open peer review
To view the open peer review materials for this article, please visit http://doi.org/10.1017/qrd.2022.20.
Acknowledgements
Giovanni Bussi, Angelo Favia and Thorben Fröhlking are gratefully acknowledged for carefully reading the manuscript and providing useful suggestions.
Comments
Comments to Author: I enjoyed the perspective by Bernetti et al, and consider this topic to be a useful area for discussion by our community. I would recommend that the authors discuss what RNA targeting drugs are currently in use (I think this is only antibiotics which bind in the ribosome), and what new targets might be of interest for the future, and why these are particularly promising, given the very limited number of RNA targeting therapies compared to DNA and proteins. I was also curious as to whether the RNA specific docking tools perform “better” for RNA compared to generic methods, and how this might be quantified. The authors may consider expanding their review to cover these points. There are a couple of minor language and typographical errors, so careful proof-reading is also needed before resubmission. Please could they define their “ADMET” abbreviation in the text for non-medicinal chemists.