Introduction
Stressful experiences during childhood are common; more than half of youth report having been exposed to at least one form of adversity (e.g., parental loss, maltreatment, or economic adversity) (McLaughlin et al., Reference McLaughlin, Greif Green, Gruber, Sampson, Zaslavsky and Kessler2012). While adolescence is characterized by normative increases in symptoms of internalizing disorders (e.g., depression and anxiety) (Bagalman & Cornell, Reference Bagalman and Cornell2018), youth who have been exposed to adversity are at a two-fold risk for developing mental health difficulties before adulthood (LeMoult et al., Reference LeMoult, Humphreys, Tracy, Hoffmeister, Ip and Gotlib2019). Importantly, LeMoult et al. (Reference LeMoult, Humphreys, Tracy, Hoffmeister, Ip and Gotlib2019) found that some forms of adversity (e.g., emotional abuse) are more strongly associated with emotional difficulties than are other stressful experiences (e.g., economic adversity).
Examining distinct forms of childhood adversity and their developmental consequences is consistent with the framework outlined in the Dimensional Model of Adversity and Psychopathology (DMAP; McLaughlin & Sheridan, Reference McLaughlin and Sheridan2016), which posits that different dimensions of adversity affect mental health through distinct neurobiological mechanisms. Adolescence is a period of continued maturation of brain circuitry that supports cognitive and emotion processing; further, findings from twin studies suggest that the environment is estimated to explain ∼30% of variation in the development of brain networks (Teeuw et al., Reference Teeuw, Brouwer, Guimarães, Brandner, Koenis, Swagerman, Verwoert, Boomsma and Hulshoff Pol2019). Different dimensions of adversity likely affect brain development in heterogeneous ways. These variations, combined with genetic or other environmental sources of neurodevelopmental variability, might moderate associations between adversity and the emergence and maintenance of internalizing symptoms throughout adolescence (Miller et al., Reference Miller, Chahal and Gotlib2021).
Two main dimensions of the DMAP – threat (the presence of potentially harmful experiences, such as physical or emotional abuse) and deprivation (the absence of expected social and cognitive input, such as physical and emotional neglect or poverty) – have been shown to have distinguishable neural consequences (McLaughlin et al., Reference McLaughlin, Weissman and Bitrán2019; Sheridan & McLaughlin, Reference Sheridan and McLaughlin2014). In a recent review, McLaughlin et al. (Reference McLaughlin, Weissman and Bitrán2019) described the brain-based alterations typically found in children and adolescents with a history of deprivation and in those exposed to threat. Children exposed to threat-related adversity (e.g., abuse, violence) were characterized by a neural signature of enhanced threat processing, including greater amygdala activation to threat conditions, greater insula reactivity to threat, and reduced amygdala volume. In contrast, children and adolescents who experienced deprivation (e.g., physical or emotional neglect or poverty) exhibited variations in fronto-parietal regions underlying cognitive control (e.g., reduced thickness and volume of the dorsolateral prefrontal cortex and superior parietal cortex). Investigators have demonstrated further that these neural alterations are associated with specific behavioral characteristics; for example, children exposed to physical and emotional neglect by being raised in institutionalized settings have been shown to exhibit neuropsychological deficits in tasks that require cognitive control (Pollak et al., Reference Pollak, Nelson, Schlaak, Roeber, Wewerka, Wiik, Frenn, Loman and Gunnar2010).
In addition to threat and deprivation, experiences of unpredictability in childhood (e.g., inconsistent caregiver presence, unstable housing) have been found to result in prolonged internalizing symptoms of depression, anxiety, and anhedonia (Glynn et al., Reference Glynn, Stern, Howland, Risbrough, Baker, Nievergelt, Baram and Davis2019). Conceptually, unpredictability has been defined as the rates at which environmental stressors vary over time, and prior work provides examples of unpredictability in parental inconsistency, residential changes, paternal transitions, and parental job changes (Belsky et al., Reference Belsky, Schlomer and Ellis2012). Further, unpredictability can include any variations in an individual’s life that could be considered stressful and unforeseen (e.g., parental divorce, death of someone close, sudden financial hardships). We know less, however, about the relation between childhood unpredictability and brain development. Although inconsistent maternal behaviors have been associated cross-sectionally with hyperconnected fronto-limbic circuitry in mice (Johnson et al., Reference Johnson, Delpech, Thompson, Wei, Hao, Herman, Hyder and Kaffman2018) and with aberrant microstructural properties in corticolimbic white matter tracts in humans (Granger et al., Reference Granger, Glynn, Sandman, Small, Obenaus, Keator, Baram, Stern, Yassa and Davis2021), the effects of unpredictability on the development of neurocircuitry and of subsequent problematic behaviors are not clear.
In general, previous studies examining associations between dimensions of childhood adversity and brain metrics have focused on differences in structural and localized functional activation. Less research has examined how dimensions of adversity affect resting-state (i.e., without task) functional connectivity within or between intrinsic networks (i.e., coactivated spatially remote areas). Elucidating functional connectivity, both within and between networks, provides a larger-scale characterization of the brain than do methods that examine single brain regions. Given the behavioral and cognitive heterogeneity of internalizing symptoms (and of psychopathology more broadly), resting-state network analysis offers a more comprehensive mapping of psychological processes to whole-brain neural functioning. In addition, test-retest reliability is higher, and signal-to-noise is stronger, in resting-state studies than they are in task-based studies (Reza Daliri, Reference Reza Daliri2014). Finally, resting-state scans can be used in most samples, including younger and older participants and those with psychiatric or cognitive differences, given that understanding and carrying out a task is not a limitation.
The Triple Network Model of Psychopathology (Menon, Reference Menon2011) posits that aberrant connectivity within and between the fronto-parietal (FPN), default mode (DMN), and salience (SAL) networks underlies a range of psychopathologies. The FPN, composed of dorsolateral prefrontal and posterior parietal cortices, is a control network involved in coordinating behavior in a goal-directed manner (Marek & Dosenbach, Reference Marek and Dosenbach2018). The DMN is a set of mostly cortical midline regions (e.g., anterior medial prefrontal cortex and posterior cingulate cortex) that are particularly active when a person is not engaged in tasks, but instead, is engaged in self-directed thought or introspection (Raichle, Reference Raichle2015). The SAL encompasses anterior cingulate and ventral anterior insular regions and is a broad network with proposed roles in awareness, salience detection, and autonomic processing (Seeley, Reference Seeley2019). Several studies have found that internalizing symptoms in adolescents are associated with altered connectivity in the FPN (Pan et al., Reference Pan, Xu, Zhou, Chen, Wei, Lu, Shang, Wang and Huang2020), DMN (Kaiser & Pizzagalli, Reference Kaiser and Pizzagalli2015; Karcher et al., Reference Karcher, Michelini, Kotov and Barch2020), and SAL (Ordaz et al., Reference Ordaz, LeMoult, Colich, Prasad, Pollak and Popolizio2016), as well as between these networks; for example, DMN-FPN connectivity has been found to be higher, and FPN-SAL connectivity lower, in youth with more severe internalizing symptoms (see review by Chahal et al., Reference Chahal, Gotlib and Guyer2020 for further information about within- and between-network connectivity differences). However, most studies examining associations between adversity and neurocircuitry have focused on fronto-limbic (mainly prefrontal-amygdala) functional connectivity. These studies have documented cross-sectionally that greater exposure to adversity is related to stronger age-related changes in prefrontal-amygdala connectivity (see reviews by Herzberg & Gunnar, Reference Herzberg and Gunnar2020; Miller et al., Reference Miller, Chahal and Gotlib2021), findings that support the Stress Acceleration Hypothesis (Callaghan & Tottenham, Reference Callaghan and Tottenham2016). To date, however, no longitudinal evidence spanning the course of adolescence has been published to address this theory from the Triple Network perspective. In the only study thus far to have examined the effects of different dimensions of adversity (i.e., abuse and neglect) on the changes in network connectivity across two timepoints of mid to late adolescence (age 16–19), Rakesh et al. (Reference Rakesh, Allen and Whittle2021) found that although within-SAL connectivity did not change on average, SAL increased in youth who experienced higher neglect and abuse; further, increases in SAL mediated the association between neglect/abuse and depressive symptoms at age 19.
We do not yet know whether different dimensions of adversity are related to the development of connectivity within and between the SAL, DMN, and FPN from early to late adolescence. Further, no studies have directly compared neurodevelopmental correlates of more than two dimensions of adversity within the same sample. In the current longitudinal study, we tested whether three dimensions of adversity (unpredictability, threat, and deprivation) are differentially associated with the development of network connectivity over three timepoints of adolescence, spanning ages 9–19 years, in a community sample of youth. Given the literature identifying altered SAL activation and connectivity in youth who have experienced stress in the form of potential harm (McLaughlin et al., Reference McLaughlin, Weissman and Bitrán2019; Rakesh et al., Reference Rakesh, Allen and Whittle2021), we hypothesized that youth with greater exposure to threat and unpredictability would be characterized by alterations in trajectories of SAL. Further, given that unpredictability constitutes random variation in salient events (Ellis et al., Reference Ellis, Figueredo, Brumbach and Schlomer2009), we hypothesized that exposure to unpredictability would also be associated with altered trajectories of SAL. Finally, based on previous findings of altered fronto-parietal activation and connectivity in youth who experienced neglect or poverty (McLaughlin et al., Reference McLaughlin, Weissman and Bitrán2019), and of altered DMN in adolescents who were exposed to poverty (D. G. Weissman et al., Reference Weissman, Conger, Robins, Hastings and Guyer2018), we hypothesized that trajectories of FPN and DMN connectivity would vary depending on levels of exposure to deprivation. Given the lack of research examining the effects of these different dimensions of adversity on network trajectories, we did not generate specific hypotheses about the directions of these effects.
Method
Sample
The sample included 225 adolescents (132 females) who were recruited in 2013–2016 from the San Francisco Bay Area to participate in a study assessing the effects of early life stress on psychobiology across puberty. Participants were 9.11–13.98 years (M = 11.33 ± 1.05) of age at the first timepoint (T1), 11.15–16.26 (M = 13.40 ± 1.06) years at the second timepoint (T2), and 13.10–19.23 (M = 15.60 ± 1.20) years at the third timepoint (T3) (Figure 1). Given the original aims of the study, participants were recruited to be in early stages of puberty at T1 (Tanner Stage M = 2.02 ± 0.74). Males and females were matched on pubertal status; thus, males (M = 11.80 ± 0.93) were older than females (M = 11.01 ± 1.01) (F(1, 223) = 36.23, p < .001). Exclusion criteria included an inability to participate in neuroimaging scans (e.g., non-removable metal), intellectual delay, current or parent neurological disorders, non-fluency in English, and self-reported onset of menses for females. 8% of the sample was Black, 9% Hispanic, 11% Asian American, 20% two or more races, 44% White, and 8% other than what was listed. Early adversity was assessed at the first timepoint, and scan data and reports of internalizing symptoms were obtained at all timepoints. Age, sex, internalizing symptom severity at T1, average connectivity value, unpredictability, and deprivation were not associated with study attrition; however, participants with higher threat-related adversity had more neuroimaging timepoints (F = 5.04, p = .025). All adolescents and their parent(s)/legal guardian(s) provided informed assent and consent, respectively. This study was approved by the Stanford University Institutional Review Board.

Figure 1. Ages of participants across timepoints. Participant ages across timepoints (T1–T3) are shown. T1 = green; T2 = purple; T3 = orange.
Dimensions of adversity (T1)
Early adversity interview (T1)
At T1, a modified version of the Traumatic Events Screening Inventory for Children (TESI-C; Ribbe, Reference Ribbe1996) was used to assess more than 30 types of stressful life experiences (e.g., domestic violence, abuse, parental separation). Participants provided details about stressful events including their own subjective severity rating. A panel of three to four coders blind to the adolescents’ subjective severity ratings then rated the objective severity of each event on a scale ranging from 0 (non-event or non-impactful) to 4 (extremely severe impact), with half-point increments, using a modified version of the UCLA Life Stress Interview coding system (Rudolph et al., Reference Rudolph, Hammen, Burge, Lindberg, Herzberg and Daley2000, ICC = 0.99). Cumulative adversity severity scores were computed by summing the maximum objective severity scores for each type of endorsed stressor (Chahal et al., Reference Chahal, Kirshenbaum, Ho, Mastrovito and Gotlib2021; King et al., Reference King, Graber, Colich and Gotlib2020) that could be characterized as either unpredictable or threatening. 223 of the 225 participants recruited for the study completed the TESI-C interview.
Unpredictability (T1)
To assess unpredictability, we summed the severity rating of the following events from the TESI-C interview: moved households, death of someone close, parental divorce, separation from family related to legal issues or travel, family legal problems, experience a disaster, witness illness/injury, experience illness/injury, witness accident, experience accident, and family financial insecurity (M = 4.06 ± 3.18).
Threat (T1)
To assess threat, we summed the maximum objective severity ratings from the TESI-C interview for the following events: family verbal conflict (including verbal threats to the participant and/or family members), bullying involving threat or experience of physical victimization, community violence, community instability (community-level threats such as hearing gunshots or bomb and active shooter threats in one’s school or neighborhood), domestic violence, community verbal conflict (including verbal threats to the community members while the participant was nearby), emotional abuse, physical abuse, mugging/robbery, war/terrorism, sexual abuse, threats of domestic violence, kidnapping, threats of physical abuse, and witnessing sexual abuse (M=2.07 ± 2.29). This measure of threat is consistent with prior work using the TESI-C that includes threat instances of exposure and threat of exposure to domestic and community violence (King et al., Reference King, Graber, Colich and Gotlib2020).
Deprivation (T1)
Although the TESI-C also assesses neglect, events related to this dimension of adversity were rarely endorsed in the current sample. Therefore, we indexed deprivation with a composite score based on continuous measures of each participant’s environment. Measures included family and neighborhood socioeconomic status (SES) indicators and (in a subset of the sample) child-reported neglectful behaviors. The composite was derived by computing the average of the Z-scored measures. Thus, our measure of deprivation indexed material deprivation to a larger degree, and emotional or psychosocial deprivation to a lesser degree.
Family-level indicators of SES (n = 193) were based on parent-reported highest education attainment and annual family income. Parent-reported highest education was coded as an ordinal variable (1 = no GED/high school diploma (.47%); 2 = GED/high school diploma (1.42%); 3 = some college (18.96%); 4 = 2-year college degree (8.53%); 5 = 4-year college degree (37.44%); 6=master’s degree (26.54%); 7=professional degree (MD, JD, DDS)/doctorate; 4.74%), and 1.9% declined to answer. We computed income-to-needs ratios (M = 1.28 ± 0.56) for each participant by dividing the caregiver-reported total family income over the previous 12 months by the 2017 low-income limit for the county in which the participants live (80% of the median income and based on number of people in the household as determined by the Department of Housing and Urban Development; https://www.huduser.gov/portal/datasets/il/il2017/2017summary.odn) (King et al., Reference King, Humphreys, Camacho and Gotlib2019). Z-scores of parental education and income-to-needs ratio were reversed, such that higher scores reflect higher deprivation.
Neighborhood-level indicators of SES (n = 214) were based on census tract information provided by the California Communities Environmental Health Screening Tool 3.0 (CalEnviroScreen; https://oehha.ca.gov/calenviroscreen), an online tool created by the Office of Environmental Health Hazard Assessment on behalf of the California Environmental Protection Agency (Faust et al., Reference Faust, August, Bangia, Galaviz, Leichty, Prasad and Zeise2017). CalEnviroScreen converts census tract information into percentiles representing disadvantage relative to other census tracts in California. Using each participant’s address, we extracted neighborhood-level percentiles of poverty (i.e., the percentage of the population living below two times the federal poverty level from 2011-2015; M = 25.04 ± 21.42), unemployment (i.e., the percentage of the eligible labor force that was unemployed during this time; M = 31.20 ± 23.90), and housing burden (i.e., the percentage of households that both make less than 80% of the HUD Area Median Family Income and pay greater than 50% of their income to housing costs from 2009-2013; M = 33.04 ± 23.82).
A subsample of the participants (n = 98) completed the Multidimensional Neglectful Behavior Scale (MNBS; Kantor et al., Reference Kantor, Holt, Mebert, Straus, Drach and Ricci2004), an 8-item self-report scale on which participants were asked to rate the degree to which they experienced psychosocial (e.g., “My parents comfort me when I am upset”) and material (e.g., “My parents give me enough clothes to keep me warm”) neglect on a scale of 1 (strongly disagree) to 4 (strongly agree). In our sample, Cronbach’s α was 0.79 for the MNBS (M = 10.96 ± 3.94), indicating acceptable reliability. Only a subsample of participants completed this measure because it was added to the battery of self-report instruments halfway through the first timepoint of study data collection.
Internalizing symptoms (T1–T3)
Participants completed the Youth Self-Report (Achenbach, Reference Achenbach1991), a reliable measure of children’s and adolescent’s emotional and behavioral problems (Ebesutani et al., Reference Ebesutani, Bernstein, Martinez, Chorpita and Weisz2011), at all timepoints (ns = 198, 161, and 156 at T1–T3, respectively). We used the validated subscale for internalizing symptoms (Cronbach’s αs = 0.92, 95% CI [0.89–0.94] at T1, 0.93 [0.91–0.95] at T2, 0.92 [0.90–0.93] at T3). Although all analyses used normalized raw scores, based on their T scores 10% of the sample had borderline or clinical levels of internalizing symptoms at T1, 8% at T2, and 15% at T3. This relatively mild-to-moderate range of symptoms was expected given that our normative sample was recruited to be in early adolescence, before the peak incidence of these symptoms is expected to occur.
Neuroimaging data (T1–T3)
Scan data acquisition and preprocessing
Detailed scan parameters, and our approach to preprocessing and postprocessing the neuroimaging data, are presented in the Supplement. Results included in this manuscript come from preprocessing performed using the automated fMRIPrep pipeline v20.2.1 (Esteban et al., Reference Esteban, Markiewicz, Blair, Moodie, Isik, Erramuzpe, Kent, Goncalves, DuPre, Snyder, Oya, Ghosh, Wright, Durnez, Poldrack and Gorgolewski2019; RRID:SCR_016216), which is based on Nipype 1.5.1 (Gorgolewski et al., Reference Gorgolewski, Burns, Madison, Clark, Halchenko, Waskom and Ghosh2011; RRID:SCR_002502). Of the 458 available resting-state scans across timepoints, 40 were excluded due to image quality and motion constraints (functional data that exceeded .25 mm framewise displacement (FD) on greater than 20 volumes or mean FD values that were greater than two standard deviations from the sample mean). Of the 418 remaining scans, 13 were excluded based on having outlier connectivity values (±1.5 × the interquartile range), resulting in a final sample of 405 samples of imaging data (ns = 169, 124, and 112 at T1–T3, respectively; ns = 67, 73, and 57 with 3, 2, or 1 timepoint(s) of neuroimaging).
ROI definitions
Regions of interest (ROIs; Figure 2) were selected from the functionally defined Seitzman 300-ROI parcellation (Seitzman et al., Reference Seitzman, Gratton, Marek, Raut, Dosenbach and Schlaggar2020), which includes both cortical and non-cortical structures. These ROIs have been validated using multiple data sets and each region is assigned functional network labels. We selected all regions (4–5 mm spheres depending on region) included in the DMN (67 regions), SAL (9 regions), and FPN (36 regions) according to the Seitzman network labels (MNI coordinates, anatomical label, and network assignments are presented in Table S1).

Figure 2. Regions of interest in examined networks. Regions of interest and network assignments were derived from the Seitzman et al. (Reference Seitzman, Gratton, Marek, Raut, Dosenbach and Schlaggar2020) atlas. DMN = default mode network (67 regions); FPN = fronto-parietal network (36 regions); SAL = salience network (9 regions).
Measures of functional connectivity
We extracted the mean time series from each ROI. We computed Fisher Z-transformed Pearson’s correlations between each region’s time series to create connectivity matrices for each participant. Within-network connectivity values were calculated by averaging across all ROI pairs in the network (e.g., SAL, FPN, and DMN). Between-network connectivity values were calculated by averaging connectivity between ROIs of a network and others (e.g., DMN-FPN, DMN-SAL, and FPN-SAL).
Data analyses
Testing associations between dimensions of adversity and trajectories of network connectivity
We used Generalized Additive Mixed Models (GAMM) in R v.4.1.0 (R Core Team, 2019) to test whether dimensions of adversity were associated with trajectories of network connectivity within and between the SAL, FPN, and DMN. Models included main effects of unpredictability, threat, and deprivation (only on dimension at a time per model), and interaction terms of the adversity dimension and age; covariates of no interest were head motion (mean FD) and scan fieldmap group status (fieldmaps were only applied during scan data preprocessing if they were available and improved image quality). Age was centered such that 9 years old was the intercept (i.e., late childhood; the youngest age in our sample) to ensure that extracted model parameters estimated change in connectivity from early through late adolescence, rather than relative to mid-adolescence. The dependent variables in these models were SAL, FPN, DMN, DMN-FPN, DMN-SAL, and FPN-SAL connectivity. In supplementary analyses, we also tested combined adversity models to determine whether any dimension of adversity was uniquely associated with trajectories of network connectivity after controlling for other dimensions. In addition, we conducted follow-up exploratory analyses to test whether family (income-to-needs ratio and parental education level) and neighborhood (CalEnviroScreen measures of neighborhood poverty, housing burden, and unemployment) SES indicators of deprivation were differentially related to trajectories of network connectivity. Given the exploratory nature of the study and the small-to-moderate neuroimaging sample size (Marek et al., Reference Marek, Tervo-Clemmens, Calabro, Montez, Kay and Hatoum2020), we did not correct the resulting p values for multiple comparisons.
Testing associations between dimensions of adversity and trajectories of internalizing symptoms
We tested whether dimensions of adversity were associated with trajectories of internalizing symptoms using separate GAMM models in which unpredictability, threat, or deprivation were included as main effects and moderators of age-related changes in symptoms. We included sex as a covariate of no interest given that females have been found to have greater increases in internalizing symptoms during adolescence than do males (Leve et al., Reference Leve, Kim and Pears2005). We also tested a combined adversity model, presented in the Supplement.
Testing initial level and trajectory of average network connectivity as moderators of the associations between dimensions of adversity and trajectories of internalizing symptoms
To simplify our analyses examining whether trajectories of network connectivity moderate associations between dimensions of adversity and trajectories of internalizing symptoms, we computed the intercepts and slopes of change in all networks (i.e., within-SAL, within-DMN, within-FPN, DMN-FPN, DMN-SAL, and FPN-SAL connectivity) by entering these values into longitudinal multilevel models that included age as the predictor of interest, and head motion and fieldmap group status as covariates of no interest. We then computed the mean connectivity intercept and slope for each participant by averaging the six network intercepts and slopes values. We similarly extracted intercepts and slopes of internalizing symptoms using age as the predictor in longitudinal multilevel models. We then used two linear regression models to test whether 1) initial levels of connectivity (intercepts) moderate associations between dimensions of adversity and initial levels of internalizing symptoms; and 2) initial levels and slopes of connectivity moderate associations between dimensions of adversity and slopes of internalizing symptoms (controlling for initial levels of internalizing symptoms).
Results
Participants and sample characteristics
Sample characteristics and correlations among age, dimensions of adversity, and internalizing symptoms are presented in Table 1. Unpredictability, threat, and deprivation were positively correlated (rs = .18–.45, ps < .005). At T1 and T2, participants with higher deprivation tended to be younger (rs = −.20 to −.26, ps < .005); neither threat nor unpredictability was significantly related to age, nor were there sex differences in any of the three dimensions of adversity (all ps > .05).
Table 1. Sample characteristics

Note. M and SD are used to represent mean and standard deviation, respectively. Values in square brackets indicate the 95% confidence interval for each correlation. The confidence interval is a plausible range of population correlations that could have caused the sample correlation (Cumming, Reference Cumming2014). Variables contributing to the deprivation score are described in the Supplement.
* Indicates p < .05.
** Indicates p < .01.
Associations between dimensions of adversity and trajectories of network connectivity
Associations between connectivity measures and across timepoints are presented in Figure S1. Connectivity values were more strongly correlated within than across timepoints. In addition, within-network connectivity (e.g., within-FPN) was higher than between-network connectivity (e.g., FPN-SAL) across timepoints (ts(399) = 11.66–52.73, ps < .0001).
Associations between dimensions of adversity and changes in network connectivity are presented in Table 2 and visualized in Figures 3–5. On average, SAL, DMN, DMN-FPN, and FPN-SAL connectivity decreased with age as indicated by negative slopes (ßs = −0.10 to −0.05, ps = <.001–.040), though these changes differed depending on dimensions of adversity. Higher unpredictability was associated with higher/flatter slopes of change (i.e., less decreases over time) in SAL, DMN-SAL, and FPN-SAL (ßs = 0.05–0.08, ps = .002–.036). Higher unpredictability was also associated with lower initial levels of SAL connectivity (ß = −0.22, p = .049). Higher threat was associated with higher/flatter slopes of change in FPN (ß = 0.05, p = .043) and DMN-SAL connectivity (ß = 0.05, p = .040). Finally, higher deprivation was associated with higher/flatter slopes in FPN (ß = 0.06, p = .011) and DMN connectivity (ß = 0.05, p = .024).

Figure 3. Associations between unpredictability and network connectivity trajectories. Participants who experienced higher unpredictability had higher initial levels of connectivity within the salience network (SAL). Participants with higher unpredictability also had smaller decreases (i.e., more stability) in within-SAL, between default mode (DMN) and SAL, and between fronto-parietal (FPN) and SAL connectivity throughout adolescence. Unpredictability is grouped only for visualization (mean ± 1 standard deviation).
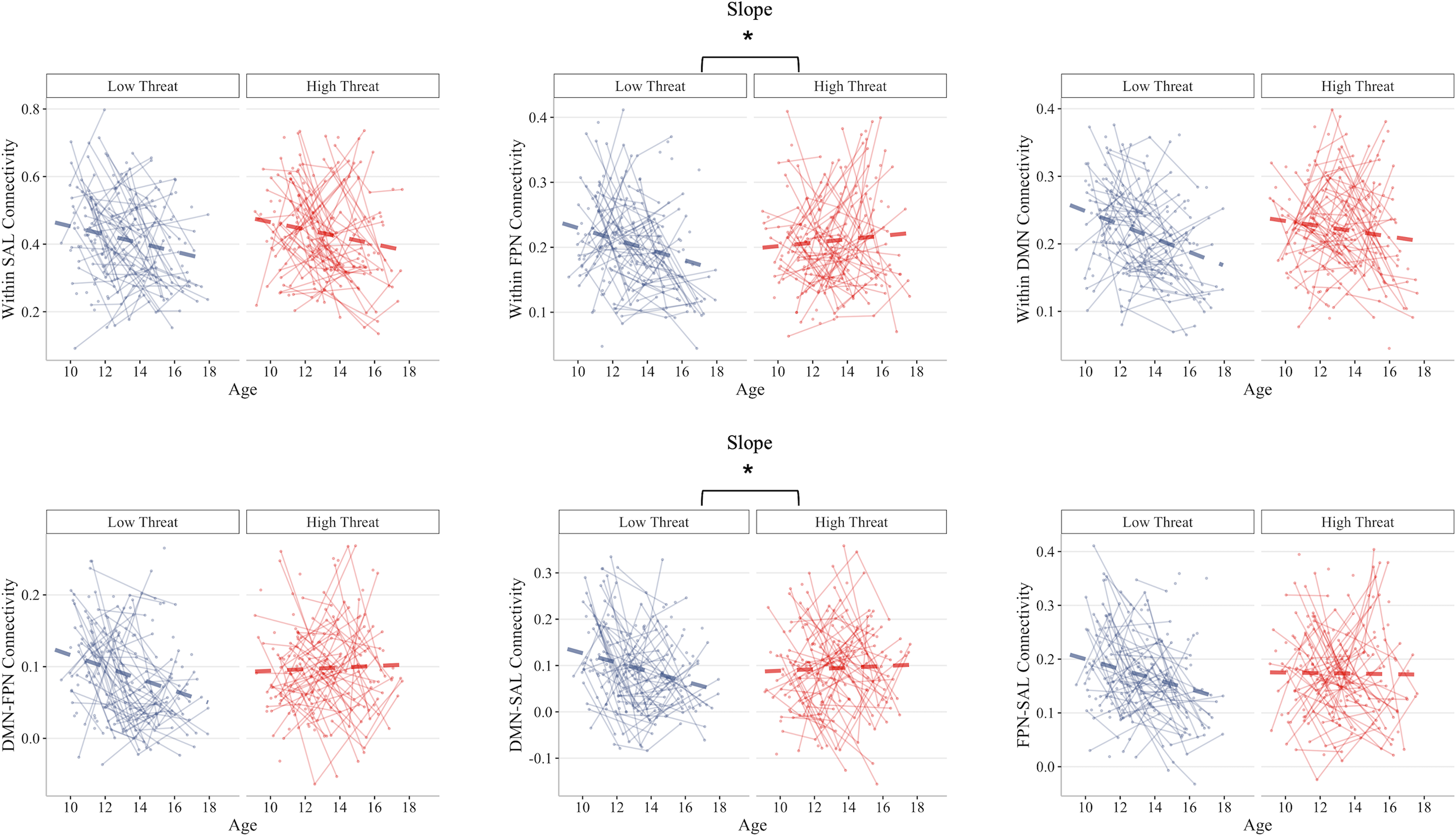
Figure 4. Associations between threat and network connectivity trajectories. Participants who experienced higher threat had smaller decreases (i.e., more stability) in FPN and DMN-SAL connectivity throughout adolescence. Threat is grouped only for visualization (mean ± 1 standard deviation).

Figure 5. Associations between deprivation and network connectivity trajectories. Participants who experienced higher deprivation had smaller decreases (i.e., more stability) in FPN and DMN connectivity throughout adolescence. Deprivation is grouped only for visualization (mean ± 1 standard deviation).
Table 2. Associations between dimensions of adversity and network connectivity development

Note: Generalized Additive Mixed Models were used to test associations between dimensions of adversity and trajectories of network connectivity across adolescence. SAL = salience network; FPN = fronto-parietal network; DMN = default mode network. Motion was based on average framewise displacement across the resting-state scan. p <.05 is indicated in bold.
Interestingly, these associations appeared to be driven by family deprivation (i.e., lower SES) rather than by neighborhood deprivation. Specifically, participants with higher family deprivation had lower initial levels and higher/flatter slopes (i.e., lower decreases) in FPN, DMN, DMN-FPN, DMN-SAL, and FPN-SAL connectivity (ps = .001–.045). In contrast, neighborhood deprivation was not associated with either initial levels or slopes in any network (ps > .05) (Table S2; Figure S2–3). Although combined adversity models yielded similar findings, either only one or no dimension of adversity was associated with connectivity development for each network (i.e., deprivation with FPN and DMN, and neither unpredictability nor threat with DMN-SAL) (Table S3).
Associations between dimensions of adversity and trajectories of internalizing symptoms
Associations between dimensions of adversity and trajectories of internalizing symptoms are presented in Table S4. Higher unpredictability was associated with higher initial levels of internalizing symptoms (ß = 0.40, p = .001), but also with lower slopes (i.e., less increases/ more stability in symptoms over time) (ß = −0.05, p = .039). Higher threat was similarly associated with higher initial levels of internalizing symptoms (ß = 0.34, p = .001), but not with changes in symptoms (p > .05). Finally, deprivation was not associated with either initial levels or changes in internalizing symptoms (ps > .05). The combined adversity model yielded similar results for associations between dimensions of adversity and initial levels of symptoms, although none of the dimensions of adversity were associated with changes in symptoms.
Initial level and trajectory of average network connectivity as moderators of associations between dimensions of adversity and trajectories of internalizing symptoms
Models testing longitudinal parameters of network connectivity as moderators of the associations between dimensions of adversity and trajectories of internalizing symptoms are presented in Table 3 and visualized in Figure 6. Initial levels of average network connectivity did not moderate the associations between unpredictability and threat and initial levels of internalizing symptoms (ps > .05). However, associations between dimensions of adversity and changes in internalizing symptoms over time were moderated by both initial levels (ßs = 0.40–0.44, ps < .004) and slopes of average network connectivity (ßs = −0.54 to −0.27, ps = .001–.047). Follow-up analyses indicated that when initial levels of average network connectivity were higher, greater unpredictability (ß = 0.26, p = .044) and greater threat (ß = 0.27, p = .026) were associated with steeper increases in internalizing symptoms. In contrast, when initial levels of connectivity were lower, greater unpredictability (ß = −0.39, p = .001) and greater threat (ß = −0.33, p = .005) were associated with shallower increases in symptoms. In addition, when connectivity slopes were lower (i.e., when there were steeper decreases in connectivity values over time), greater unpredictability (ß = 0.34, p = .010) and greater threat (ß = 0.30, p = .016) were associated with steeper increases in symptoms; however, when connectivity slopes were higher (i.e., when there were smaller decreases in connectivity values over time), there were shallower increases in symptoms (unpredictability: ß = −0.47, p < .001; threat: ß = −0.36, p = .002). Similarly, greater deprivation was associated with steeper increases in internalizing symptoms when connectivity slopes were lower (ß = 0.24, p = .034), but not when connectivity slopes were higher (ß = −0.16, p = .137). In a combined adversity model that controlled for each dimension of adversity, only the association between unpredictability and trajectories of internalizing symptoms was moderated by initial levels and slopes of network connectivity (Table S5).

Figure 6. Network connectivity trajectories moderate associations between dimensions of adversity and trajectories of internalizing symptoms. (a) Higher levels of unpredictability and threat were associated with higher initial levels (i.e., intercepts) of internalizing symptoms; initial levels of average connectivity across networks (FPN, DMN, SAL, and between these networks) did not moderate these associations. (b) Higher unpredictability was associated with increases in internalizing symptoms over time when initial connectivity was higher; a negative association between unpredictability and internalizing symptom slopes was found when initial connectivity was lower. Similarly, threat was positively associated with internalizing slopes when initial connectivity was higher; a negative association between threat and internalizing slopes was found when initial connectivity was lower. There was no association between deprivation and internalizing symptom severity slopes, and connectivity intercepts did not moderate this non-association. (c) All dimensions of adversity were associated with increases in internalizing severity when connectivity slopes were lower (i.e., greater decreases); however, in participants with higher connectivity slopes (i.e., less decreases), greater adversity was related to decreases in internalizing symptoms over time. All adversity dimensions are grouped only for visualization (mean ± 1 standard deviation). All models included sex, head motion (average framewise displacement), and fieldmap correction group as covariates of no interest. The model measuring the interactions of adversity and connectivity intercept and slope on slopes of internalizing symptoms included the internalizing intercept as a covariate.
Table 3. Initial levels and slopes of average network connectivity as moderators of the associations between dimensions of adversity and initial levels and slopes of internalizing symptoms

Note: Connectivity = average connectivity across all examined networks. p < .05 is indicated in bold.
Follow-up exploratory analyses showed that our findings of initial levels and slopes of connectivity moderating associations between dimensions of adversity and trajectories of internalizing symptoms were not specific to certain networks, but were widespread across SAL, FPN, DMN, DMN-FPN, DMN-SAL, and FPN-SAL connections (Table S6).
Discussion
In a community sample of adolescents, we found that the functional architecture of brain networks that integrate sensory, emotional, and cognitive information matures differently depending on the dimension of adversity experienced. While connectivity within and between most brain networks decreased over adolescence, youth exposed to adversity generally had stable connectivity over time. Whereas the adversity-related differences in network development were widespread for family-level deprivation, they were confined to SAL-related connectivity measures for unpredictability, and specific to FPN and DMN-SAL connectivity measures for threat. In addition, adolescents who experienced higher family-level deprivation had lower initial levels of connectivity in most networks, a pattern also observed in the SAL for adolescents who experienced more unpredictability. These findings suggest that different dimensions of adversity are related to the development of distinct functional brain networks in similar ways (i.e., flatter slopes); however, whereas some dimensions (family-level socioeconomic disadvantage) have more widespread neural relations, others (threat and unpredictability) appear to be more focal.
The present findings also suggest that in youth who were exposed to higher levels of unpredictability, threat, or deprivation, changes (or lack of changes) in network connectivity throughout adolescence are generally adaptive, as they are associated with smaller increases, and in some cases, with improvements, in internalizing symptoms. However, because youth with higher unpredictability and more threat-related experiences of adversity enter adolescence with elevated levels of internalizing symptoms, the lack of normative increases in symptoms throughout adolescence may not necessarily be a favorable outcome; rather, stability in functional brain networks may reflect, or contribute to, the maintenance of internalizing symptoms throughout adolescence and, perhaps, into adulthood. Longitudinal studies that extend into adulthood are needed to understand whether the current findings reflect an earlier emergence and maintenance of internalizing syndromes in youth who were exposed to early adversity or an adaptive neurodevelopmental pattern that attenuates increases in symptoms in adversity-exposed youth during adolescence and adulthood. Researchers should also examine relations with other symptom domains (e.g., externalizing, social, cognitive) to assess whether these patterns of network connectivity contribute to other types of socio-emotional difficulties.
The Triple Network Model posits that different forms of psychopathology are characterized by aberrant functional organization of the SAL, FPN, and DMN and their cross-network interactions (Menon, Reference Menon2011). Consistent with our results, Sylvester et al. (Reference Sylvester, Whalen, Belden, Sanchez, Luby and Barch2018) found that DMN connectivity decreased with age during adolescence; however, the negative slope of DMN connectivity was flatter in adolescents with higher levels of shyness. These researchers posited that by the end of adolescence, shy youth have intra-DMN hyper-connectivity, potentially contributing to heightened self-referential thinking from which it is difficult to disengage. We found similarly in this study, that youth who experienced more deprivation had flatter slopes of DMN change than did their lower-deprivation peers; however, this connectivity pattern was associated with smaller increases in internalizing symptoms during adolescence. As we noted above, it is possible that a flat trajectory of DMN connectivity reflects the maintenance of stable, elevated internalizing symptoms over adolescence.
As we found within the DMN, DMN-FPN connectivity remained stable over adolescence and, given the general age-related decrease in network connectivity, DMN-FPN connectivity may be relatively elevated in late adolescence in youth exposed to higher family-level deprivation. The DMN and FPN have been shown to become more anticorrelated in adolescence (Chai et al., Reference Chai, Ofen, Gabrieli and Whitfield-Gabrieli2014), a neurodevelopmental pattern posited to facilitate the inhibition of internally directed self-referential thoughts during cognitively demanding tasks (D. H. Weissman et al., Reference Weissman, Roberts, Visscher and Woldorff2006). However, one large study found that while lower DMN-FPN connectivity was related to higher cognitive test scores in above-poverty youth, in below-poverty youth higher cognitive tests scores were related to higher DMN-FPN connectivity (Ellwood-Lowe et al., Reference Ellwood-Lowe, Whitfield-Gabrieli and Bunge2020). Thus, an “aberrant” pattern of network development for some youth may be an adaptive pattern for others (e.g., those exposed to high family-level deprivation).
Participants who experienced greater deprivation had more stable FPN connectivity throughout adolescence, whereas youth who experienced less deprivation showed small decreases in FPN connectivity. This pattern of findings is consistent with results reported in a recent review of altered function in fronto-parietal regions in children exposed to deprivation (McLaughlin et al., Reference McLaughlin, Weissman and Bitrán2019). Task-based studies have found that children from lower- versus higher-income families differentially engage prefrontal and parietal brain regions during cognitive tasks, likely reflecting differences in neural mechanisms needed to engage in executive processes and performance on these tasks (Sheridan et al., Reference Sheridan, Peverill, Finn and McLaughlin2017; Sheridan et al., Reference Sheridan, Sarsour, Jutte, D’Esposito and Boyce2012). We did not separate prefrontal and parietal regions in our FPN network; however, researchers have shown that during adolescence, prefrontal connections increase while parietal interconnectivity decreases, a pattern that coincides with developmental improvements in inhibitory control (Hwang et al., Reference Hwang, Velanova and Luna2010). Youth who experience greater deprivation may require greater co-activation between FPN regions throughout adolescence to engage similar levels of cognitive control as their non-exposed peers. Because we did not measure cognitive control explicitly in our study, this formulation is speculative; again, however, this neurodevelopmental pattern may be adaptive for youth exposed to higher family-level deprivation.
We found that youth exposed to greater unpredictability had flatter slopes of change in within and between SAL network connectivity. The SAL is involved in attentional capture of salient external stimuli and is necessary for dynamic network interactions between the FPN and DMN (Menon, Reference Menon2011). Damage to white matter tracts connecting SAL regions, particularly with the DMN, has been shown to impair cognitive control abilities in the context of unexpected stimuli (Jilka et al., Reference Jilka, Scott, Ham, Pickering, Bonnelle and Braga2014). Thus, the SAL plays an important role in inhibition, performance monitoring, attention, and working memory during unexpected events (Wessel & Aron, Reference Wessel and Aron2017).
Functional connections within the SAL increase in response to stressors, typically followed by decreases in connectivity when the stressor is no longer present (van Leeuwen et al., Reference van Leeuwen, Vinkers, Vink, Kahn, Joëls and Hermans2021). Following unpredictable adversity, stable and elevated SAL connectivity may prepare individuals for unexpected stressors and cognitive demands from the environment. For example, elevated within-SAL and DMN-SAL connectivity has been found following induced stress and has been referred to as "alerted default mode" (Clemens et al., Reference Clemens, Wagels, Bauchmüller, Bergs, Habel and Kohn2017). When the stressor is no longer present, however, this elevated SAL connectivity pattern could contribute to unnecessarily elevated physiological arousal; for example, within-SAL connectivity has been shown to be associated with heart rate (C. B. Young et al., Reference Young, Raz, Everaerd, Beckmann, Tendolkar, Hendler, Fernández and Hermans2017). Sustained hyper-connectivity of the SAL may also underlie symptoms of psychopathology. Indeed, heightened DMN-SAL connectivity has been found in individuals with less accurate self-appraisal and higher levels of internalizing symptoms (Hogeveen et al., Reference Hogeveen, Krug, Elliott and Solomon2018). In addition, Rakesh et al. (Reference Rakesh, Allen and Whittle2021) found that abuse and neglect were related to increases in SAL connectivity from mid-adolescence to late adolescence, a pattern that mediated the link between abuse/neglect and depressive symptoms at age 19 (Rakesh et al., Reference Rakesh, Allen and Whittle2021). We similarly found higher SAL slopes in youth exposed to higher unpredictability; however, we also found decreases in SAL connectivity in low unpredictability contexts and stable SAL connectivity in high unpredictability contexts. These trajectory differences may be due to participants’ ages in the two samples: whereas Rakesh et al.’s sample spanned mid-adolescence to late adolescence at two timepoints (ages 16 and 19), our sample spanned early to late adolescence and was heavily weighted in ages 12–16 (Figure 2).
Compared to unpredictability and deprivation, we found that threat-related adversity was related specifically to variations in the maturation of FPN and DMN-SAL networks. Previous studies examining the impact of threat-related adversity (e.g., abuse) on functional connectivity focused primarily on interactions between the amygdala and other regions (e.g., Cheng et al., Reference Cheng, Mills, Miranda Dominguez, Zeithamova, Perrone, Sturgeon, Feldstein Ewing, Fisher, Pfeifer, Fair and Mackiewicz Seghete2021). Although the networks we examined did not include the amygdala, our findings provide novel evidence about the role of threat in FPN development. In their review, McLaughlin et al. (Reference McLaughlin, Weissman and Bitrán2019) reported that threat is not consistently associated with alterations in activation or structure of fronto-parietal regions; in contrast, we found that functional connectivity within these regions follows a different trajectory for threat-exposed youth. Similar to deprivation- and unpredictability-exposed youth, an altered trajectory of FPN and DMN-SAL connectivity during adolescence may be related to levels of neurocognitive and sensory integration that underlie stable and/or elevated internalizing symptom levels throughout adolescence.
We did not find many main effects of threat or unpredictability on connectivity development, although we did find interactions with age. These findings suggest that in this study, initial levels of connectivity in early adolescence are not significantly related to threat or unpredictability, although changes in connectivity throughout adolescence are related to these dimensions; however, we did find that both initial levels and changes in connectivity are associated with levels of familial deprivation. These dimension-level differences in connectivity trajectories might be attributed to variability in our adversity measures, where threat was more positively skewed than was deprivation, which was more normally distributed. Greater variability in the familial deprivation measure, in addition to more consistent differences in resource availability throughout life, might have contributed to our finding that both initial levels and changes in connectivity were related to familial deprivation. Instances of unpredictability and threat were less endorsed and likely infrequent throughout the participants’ lives; thus, in this unselected community sample, these dimensions of adversity were associated only with changes in, but not with initial levels of, connectivity. Unpredictability and threat might have been associated in early adolescence with differences in other networks not measured in this study.
In our study, we tested whether brain development moderates the link between adverse experiences and internalizing symptoms. Other factors, including early experiences (e.g., during infancy), environmental and individual cognitive sources of resilience (e.g., parental and peer support; cognitive control), and genetic variations have been shown to contribute to heterogeneity in brain development (Graham et al., Reference Graham, Fisher and Pfeifer2013; Holz et al., Reference Holz, Tost and Meyer-Lindenberg2020; Teeuw et al., Reference Teeuw, Brouwer, Guimarães, Brandner, Koenis, Swagerman, Verwoert, Boomsma and Hulshoff Pol2019). Thus, while we demonstrated in the current study that adverse experiences in childhood and early adolescence are related to differences in the development of functional connectivity, other sources of variability likely interact to influence associations among adversity, neurodevelopment, and symptom changes.
We should note that our findings do not support nor refute the Stress Acceleration Hypothesis (Callaghan & Tottenham, Reference Callaghan and Tottenham2016), which posits that early adversity leads to accelerated development of emotion circuits in the brain. We did find that initial levels of functional connectivity were lower in the SAL in youth with higher unpredictability, and that initial levels of all other networks were lower in youth who were exposed to family-level deprivation. Because the normative trajectory of change in this sample was one of decreasing connectivity, we might conclude from the initial level data that in early adolescence, youth exposed to greater adversity exhibit more "mature" neural architecture at a younger age. However, when we examined longitudinal changes in these networks, we found different trajectories of change in network connectivity for low- (decreasing connectivity) versus high- (stable connectivity) adversity-exposed youth. While amygdala-prefrontal circuitry, in particular, might exhibit accelerated development, our network-level findings suggest neither an acceleration nor a delay (i.e., a temporal shift in the same slope shape) in the development of FPN, SAL, or DMN connectivity during adolescence in youth exposed to unpredictability, threat, or deprivation.
In our main models (i.e., adversity dimensions tested separately), we found evidence for relations of two different dimensions to development of connectivity in several networks (e.g., deprivation and threat for the FPN and unpredictability and threat for the DMN-SAL). However, in combined models that controlled for co-occurring dimensions of adversity, fewer dimensions of adversity were related to network development. Dimensions of adversity often co-occur, and they may have shared neurocognitive mechanisms of psychopathology (e.g., aberrant FPN development following deprivation and threat). Indeed, all three dimensions in the current study were correlated with each other, and combining dimensions did not distinguish phenotypes; that is, many of the findings relating adversity dimensions to connectivity development were no longer significant when including threat, unpredictability, and deprivation in the same model. Multicollinearity increases the standard errors of coefficients in a linear regression model, leading to coefficients of independent variables not being significantly different from zero (Kim, Reference Kim2019). In separate models, we found evidence for unique neurodevelopmental consequences of specific dimensions of adversity (e.g., atypical SAL development following unpredictability), providing support for the formulation of DMAP model that adverse experiences do not have equivalent developmental sequelae.
It is noteworthy that we found decreases in connectivity within and between most networks. Several resting-state fMRI studies previously reported that adolescence is characterized by increases in long-range connections in cortical areas and decreases in short-range connections (e.g., Fair et al., Reference Fair, Dosenbach, Church, Cohen, Brahmbhatt, Miezin, Barch, Raichle, Petersen and Schlaggar2007; Supekar et al., Reference Supekar, Musen and Menon2009). However, these findings were later shown to be confounded by head motion during the scan (Power et al., Reference Power, Barnes, Snyder, Schlaggar and Petersen2012; Satterthwaite et al., Reference Satterthwaite, Wolf, Ruparel, Erus, Elliott, Eickhoff, Gennatas, Jackson, Prabhakaran, Smith, Hakonarson, Verma, Davatzikos, Gur and Gur2013). We applied stringent quality-control criteria to the functional neuroimaging data by excluding participants based on individual- and sample-level motion thresholds, regressing motion-related artifacts (i.e., framewise displacement, head motion parameters and their first- and second-order derivatives), censoring high motion frames, and including mean framewise displacement as a covariate of no interest in statistical models. Nevertheless, head motion is inevitably a source of fMRI signal artifact in neuroimaging studies, particularly in studies involving children and adolescents. In the current study, even after our quality-control steps, head motion was positively associated with nearly all connectivity measures, but also decreased with age. Our results are consistent with more recent studies using similar levels of motion control that also found that, on average, that connectivity within and between the FPN, SAL, and DMN either decreases with age or does not change (e.g., Rakesh et al., Reference Rakesh, Allen and Whittle2021; Sylvester et al., Reference Sylvester, Whalen, Belden, Sanchez, Luby and Barch2018) over time.
Limitations
We should note three limitations of this study. First, we did not correct results for multiple comparisons. We selected our networks of interest based on the Triple Network Model and because they are frequently implicated in psychopathology. However, very few studies have tested associations between different dimensions of adversity and longitudinal changes in these networks during adolescence. Consequently, our statistical analyses were exploratory in nature, and we did not correct for multiple comparisons. Second, our networks of interest have been validated primarily in samples of young adults (e.g., Seitzman et al., Reference Seitzman, Gratton, Marek, Raut, Dosenbach and Schlaggar2020). It is possible that these networks contain different regional components at younger ages. We did select a predefined network parcellation rather than a data-driven and sample-specific network definition so that future studies could replicate our methods. Third, our sample size was moderate for a neuroimaging study. We had 405 total datapoints, but still had slightly less than the 180 participants recommended to detect age-related changes in functional network connections after accounting for head motion (Satterthwaite et al., Reference Satterthwaite, Wolf, Ruparel, Erus, Elliott, Eickhoff, Gennatas, Jackson, Prabhakaran, Smith, Hakonarson, Verma, Davatzikos, Gur and Gur2013). Analyses of large multi-site developmental cohort study data (e.g., the Adolescent Brain Cognitive Development Study) are needed to confirm our findings of relations between dimensions of adversity and changes in network connectivity and trajectories of internalizing symptoms. Fourth, we measured adversity only at the first timepoint in order to examine the impact of stressful experiences from early childhood to early adolescence, rather than throughout adolescence. However, there may have been later experiences that were unassessed at later follow-up points that could contribute to the development of network connectivity. Although we closely followed definitions of different adversities based on prior work, we did use “best-guess” heuristics to parse events into different dimensions of adversity. For example, we included "experiencing a disaster" in our unpredictability measure, while "witnessing war/terrorism" was selected for threat. In another study conducted by different investigators, these events might be transposed. Therefore, it is important that future work examine whether there are clear and statistically significant distinctions among adverse experiences, or whether it is sound practice to include one type of event in different dimensions of adversity. Similarly, although our measure of unpredictability is aligned with that used in previous work, researchers have discussed the importance of measuring unpredictability more precisely as statistical variation in the environment (E. S. Young et al., Reference Young, Frankenhuis and Ellis2020). Conceptualizing and measuring dimensions of adversity more strongly and consistently across studies should increase the generalizability of findings. Finally, exposure to threat, deprivation, and unpredictability in our sample may be relatively low compared to what one might see in a sample that had experienced more severe forms of trauma (e.g., institutionalized youth) or from a more socioeconomically disadvantaged community. Thus, it is not clear whether our findings are generalizable to samples of youth with higher levels of adversity. Nevertheless, it is noteworthy that even in an unselected community sample of youth, variations in levels of adversity were meaningfully related to neural heterogeneity throughout adolescence.
Conclusions
Several studies have provided foundational knowledge about relations between adversity and functional connections in the brain; however, past work has been primarily cross-sectional and has focused on the amygdala (e.g., Cheng et al., Reference Cheng, Mills, Miranda Dominguez, Zeithamova, Perrone, Sturgeon, Feldstein Ewing, Fisher, Pfeifer, Fair and Mackiewicz Seghete2021; see review by Herzberg & Gunnar, Reference Herzberg and Gunnar2020). The current study investigated the roles of three dimensions of adversity (unpredictability, threat, and deprivation) in the development of three broad functional networks that have been implicated in psychopathology (the SAL, DMN, and FPN); further, we examined whether trajectories of network connectivity explain differences in trajectories of internalizing symptoms following exposure to adversity. This study is among the first to show that exposure to different dimensions of adversity is associated with alterations in the development of intrinsic brain networks. Alterations in trajectories of network connectivity were widespread for youth who experienced greater family-level deprivation, focal to SAL-related networks for youth who experienced more unpredictable forms of adversity, and specific to FPN and DMN-SAL networks for youth who experienced threat-related adversity. The trajectories of network connectivity that we observed in youth who were exposed to adversity were accompanied by smaller increases and, in some cases, decreases in internalizing symptoms throughout adolescence. Longitudinal studies with wider developmental windows are needed to examine whether the patterns of network maturation observed in the current study are adaptive or, alternatively, reflect the maintenance of internalizing symptoms throughout adolescence and into adulthood. Our findings suggest that patterns of functional brain development during adolescence are related to childhood adversity, particularly in networks integrating sensory, cognitive, and emotional information, and underscore the importance of distinguishing among different dimensions of adversity and their relations to brain development.
Supplementary material
The supplementary material for this article can be found at https://doi.org/10.1017/S0954579421001814
Acknowledgments
We thank the participants and their families for participating in this research.
Funding statement
This research was supported by the National Institutes of Health (NIH; R37MH101495 to IHG and F32MH120975 to RC), the Klingenstein Third Generation Foundation to RC, and the Stanford University Precision Health and Integrated Diagnostics Center to IHG.
Conflicts of interest
None.