The burden associated with common human pathogens becoming resistant to the majority of prescribed antimicrobials has been defined by the World Health Organization (WHO) as one of the current greatest threats to humanity.1 Multidrug-resistant organisms (MDROs) and extensively drug-resistant organisms (XDROs),Reference Magiorakos, Srinivasan and Carey2 are prevalent in hospitals, in non–acute-care settings, and even in the community.Reference Marchaim, Chopra and Bogan3 Infections with MDROs and XDROs result in significantly worse outcomes, partially due to delay in initiation of appropriate antimicrobial therapy (DAAT).Reference Paul, Shani, Muchtar, Kariv, Robenshtok and Leibovici4 In severe sepsis, DAAT is the strongest modifiable independent predictor for mortality.Reference Paul, Shani, Muchtar, Kariv, Robenshtok and Leibovici4 However, the widespread use of empiric, broad-spectrum therapy by providers further increases the burden of resistance.Reference Bogan and Marchaim5 Moreover, when the infection results from a susceptible strain, some of the broad-spectrum agents (eg, vancomycin, colistin), are less efficacious and more toxic than narrower-spectrum agents.Reference Wong, Wong, Romney and Leung6 This “double-edged sword” situation is harmful both to individual patients and to the ecology in general.Reference Pogue, Kaye, Cohen and Marchaim7
Previous studies have used various definitions for healthcare-associated infection in an attempt to predict resistance phenotypes.Reference Friedman, Kaye and Stout8 These definitions have also been incorporated into official treatment guidelines,9 such as the Duke-2002 criteriaReference Friedman, Kaye and Stout8 or the modified Duke-2002 criteria, to guide the empiric initiation of broad-spectrum agents.Reference Cardoso, Almeida and Friedman10 Further work has shown that these definitions perform poorly in predicting resistance.Reference Friedman, Levit and Taleb11 Some prescribers use scores composed of acute illness indices, such as the APACHE-II for septic shockReference Lee, Lim and Hong12 or the CURB-65 for community-onset pneumonia,Reference Liu, Xu and Zhou13 to guide empiric coverage for MDROs, even though those scores were developed and validated for other purposes. Therefore, prescribers need directive and reliable tools to improve appropriate targeting of antimicrobials to populations at high risk of MDROs or XDROs and to reduce the misuse of broad-spectrum therapeutics.Reference Pogue, Kaye, Cohen and Marchaim7
The management at the initial acute phase of sepsis is crucial to patient outcomes.Reference Kumar, Roberts and Wood14 There are 2 common time points at which decisions are made pertaining to initial management of acute sepsis, and errors frequently occur.Reference Pogue, Kaye, Cohen and Marchaim7 The first is upon admission (UA), with regard to MDROs such as extended-spectrum β-lactamase–producing Enterobacterales (ESBL),Reference Marchaim, Gottesman and Schwartz15 methicillin-resistant Staphylococcus aureus (MRSA),Reference Marchaim, Kaye and Fowler16 and Pseudomonas aeruginosa. The second is in cases of nosocomial infection, with regard to XDROs, such as vancomycin-resistant Enterococcus (VRE),Reference Hayakawa, Martin and Gudur17 and carbapenem-nonsusceptible gram-negative bacteria, such as Enterobacterales (CRE),Reference Marchaim, Chopra and Bhargava18 Acinetobacter baumannii (CRAB),Reference Tal-Jasper, Katz and Amrami19 and P. aeruginosa (CRPA).Reference Aviv, Lazarovitch and Katz20
Prior attempts to develop tools to predict resistance have been limited by several factors. Some scores lacked comprehensiveness and were either focused on a specific pathogen (eg, differentiating ESBL from CRE),Reference Martin, Tansek and Collins21 on a specific drug (eg, predicting resistances to piperacillin-tazobactam, cefepime, or meropenem in the ICU),Reference Vazquez-Guillamet, Vazquez, Micek and Kollef22 or were limited to patients with a single infectious syndrome (eg, pneumonia or UTI).Reference Webb, Dascomb and Stenehjem23,Reference Hebert, Gao and Rahman24 These studies addressed certain aspects of empiric prescription, but they did not address the whole process of decision making that prescribers face, particularly among cognitively impaired patients, in which the infectious syndrome (and naturally the offending pathogen) at the initial acute phase are difficult to determine.Reference Pogue, Kaye, Cohen and Marchaim7 Moreover, prior scores were limited by low performance,Reference Vazquez-Guillamet, Vazquez, Micek and Kollef22 small sample size,Reference Tumbarello, Trecarichi and Bassetti25 the absence of validation data sets,Reference Vazquez-Guillamet, Vazquez, Micek and Kollef22 requiring parameters that were unpractical to extract bedside (eg, Charlson scores),Reference Tumbarello, Trecarichi and Bassetti25,Reference Charlson, Pompei, Ales and MacKenzie26 or reliance on additional non–standard-of-care testing (eg, arterial blood gases to calculate APACHE for patients with mild sepsis).Reference Vazquez-Guillamet, Vazquez, Micek and Kollef22 In addition, prescribers in general are reluctant to memorize scores.Reference Pogue, Kaye, Cohen and Marchaim7
We aimed to generate a simple electronic calculator to predict both MDRO UA and nosocomial XDRO infections, and only require parameters to be entered which are readily available at the bedside.
Methods
We conducted a retrospective cohort study at Shamir Medical Center (SMC), Israel. The derivation data set for the MDRO UA score consisted of patients with sepsis admitted to the emergency room from August 28, 2016, to December 12, 2016 (overall, 106 consecutive days, a convenience sample based on a-priori power calculations). The derivation data set for the nosocomial XDRO score consisted of hospitalized septic patients from the entire calendar year of 2016. The institution’s ethics committee approved the study prior to initiation.
We applied the following inclusion criteria: patients aged >18 years, with presence of sepsis,Reference Singer, Deutschman and Seymour27 from whom blood cultures were obtained. According to hospital regulations, blood cultures are ordered from every hospitalized patient with sepsis, disregarding its severity or its origin. Data used for the derivation of the MDRO UA score data set were restricted to patients with sepsis from whom blood cultures were obtained during the first 2 calendar days of hospitalization (ie, the day of ER admission was considered day 1). The nosocomial XDRO score was based on a data set of septic patients from whom blood cultures were obtained on day 4 of hospitalization and thereafter. Patients from whom blood cultures were drawn on day 3 of hospitalization were not included in either data set. Patients with monomicrobial, microbiologically confirmed, anaerobic or fungal infection were excluded because these infections are subjected to a different set of prediction rules.Reference Marcus, Levy and Salhab28
Multidrug-resistant organisms (MDROs) and extensively drug-resistant organisms (XDROs)
We utilized broad definitions of MDROs and XDROs as determined by an international consortium in 2012, Reference Magiorakos, Srinivasan and Carey2 and we modified them to include pathogens resistant to the initial first line of therapy recommended by official guidelines.29 MDROs were defined as follows: (1) MRSA, (2) ampicillin-nonsusceptible enterococci, (3) penicillin- or ceftriaxone-nonsusceptible Streptococcus pneumoniae, (4) P. aeruginosa, (5) A. baumannii, or (6) Enterobacterales nonsusceptible to 1 or more third-generation cephalosporin (eg, ceftriaxone, ceftazidime).
XDROs were defined as follows: (1) VRE, (2) heterogeneous vancomycin-intermediate S. aureus (hVISA) or S. aureus with MIC≥2 to vancomycin,Reference Liu, Bayer and Cosgrove30 (3) CRE (Enterobacterales with evidence of carbapenemase production and/or with meropenem MIC≥2),31 (4) CRAB, (5) CRPA, and (6) intrinsically carbapenem-nonsusceptible gram-negative bacteria (eg, Stenotrophomonas maltophilia and Burkholderia cepacia).
Data collection derivation data set
Clinical data were retrieved from all records. Mortality data were retrieved from a national registry governed by the Israeli Ministry of the Interior. More than 200 potential score components were captured (Supplementary Table 1 online), including demographics, healthcare exposures, comorbidities, acute illness indices, microbiological data, antimicrobial treatments, and outcomes. The infectious clinical syndrome was determined based on established criteria.32 The microbiological diagnosis was determined based on blood culture results or culture results from nonsterile sites that were coupled to the appropriate infectious syndrome (eg, respiratory culture for patients with pneumonia, urine culture for patients with urinary-tract infection and so on). Only parameters that were readily available at the bedside to a clinician equipped with an electronic chart were incorporated into the models.
Table 1. Univariable Analyses of Features Associated With Septic Patients with Multidrug-Resistant Organism (MDRO) Infection Upon Admission (UA) Versus Those With Non-MDRO UA infection, and of Patients With Nosocomial Extensively Drug-Resistant Organism (XDRO) Infection Versus Patients With Non-XDRO Nosocomial Infection (Derivation Data Sets)
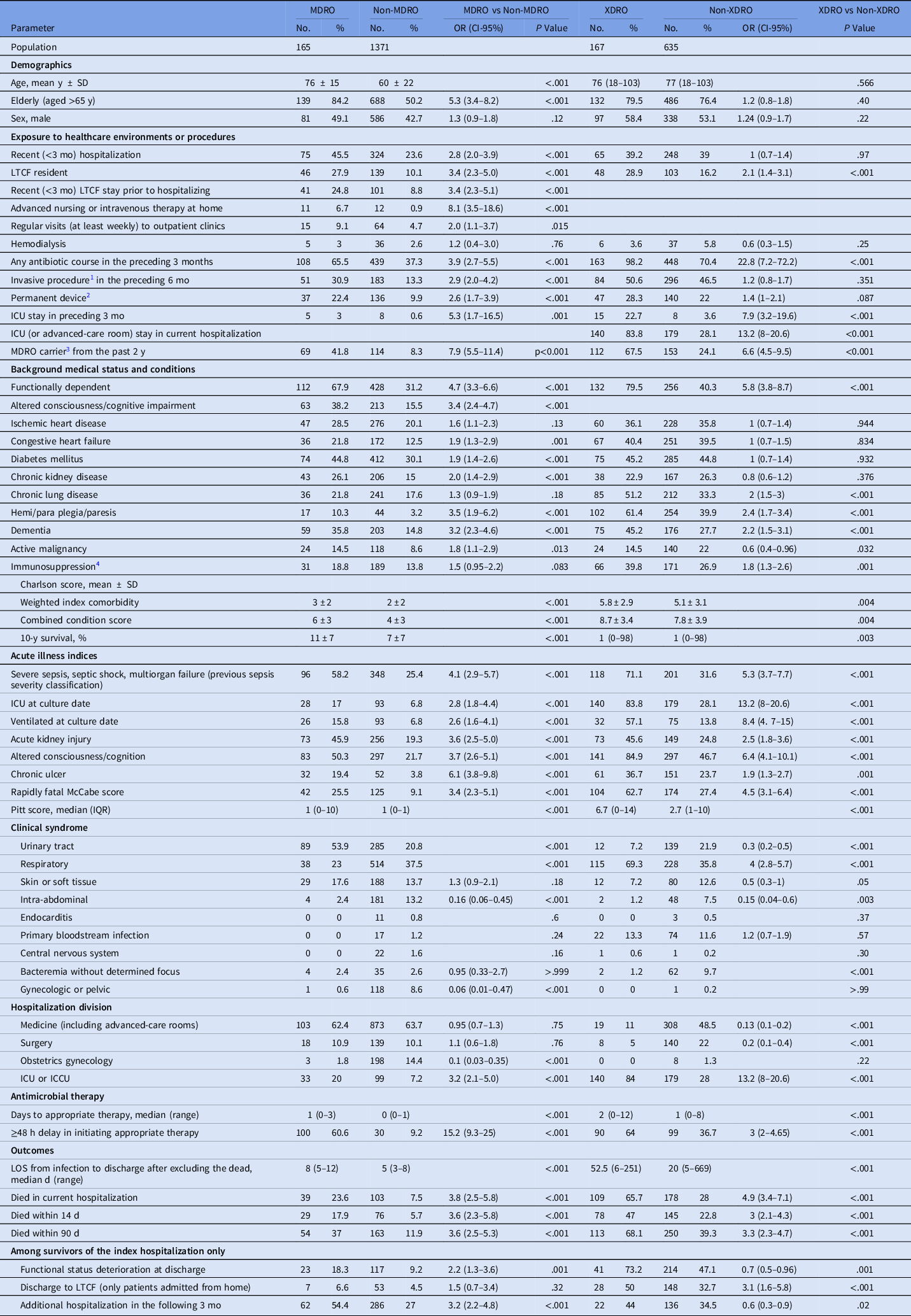
Note. MDRO, multidrug-resistant organism; OR, odds ratio; CI, confidence interval; LTFC, long-term care facility; S/P, stats post; CABG, coronary artery bypass graft; CVA, cerebrovascular accident; TIA,- transient ischemic attack; AIDS, acquired immune deficiency syndrome; SIRS, systemic inflammatory response syndrome; ICU, intensive care unit ICCU, intensive cardiac care unit; LOS, length of stay; SD, standard deviation; IQR, interquartile range; LOS, length of stay.
Validation data sets
The score performances were tested on different historical validation data sets. Among them, 6 were from SMC and 1 was from Detroit Medical Center. The same inclusion, exclusion, and stratification criteria were applied to all data sets. In a few of the validation data sets, only patients with positive blood culture results were included. The MDRO UA score was validated for 3 data sets, and the nosocomial XDRO score was validated for 4 data sets (Supplementary Table 2 online).
Table 2. Multivariable Model of Risk Factors for MDROa Upon Admission (Derivation Data Set)
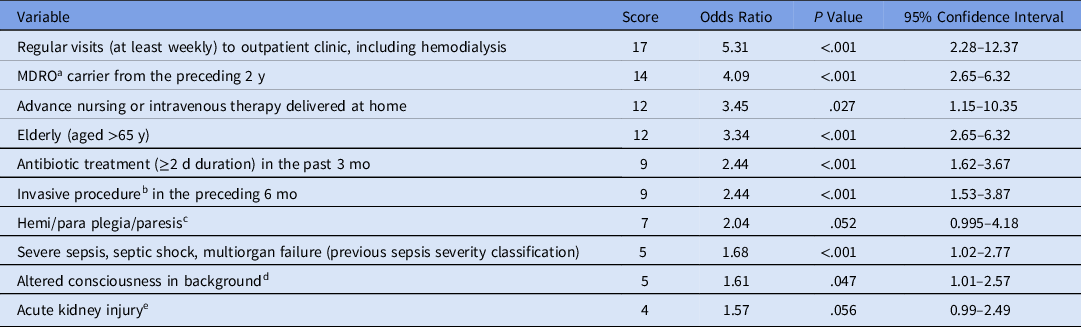
Note. MDRO, multidrug-resistant organism.
a MDRO include (1) Methicillin-resistant staphylococcus aureus (MRSA), (2) ampicillin non-susceptible enterococci, (3) penicillin or ceftriaxone nonsusceptible Streptococcus pneumoniae, (4) Pseudomonas aeruginosa, (5) Acinetobacter baumannii, and (6) Enterobacterales nonsusceptible to 1 or more third-generation cephalosporin (eg, ceftriaxone, ceftazidime).
b This includes any type of invasive procedure, such as surgery, endoscopy, central-line placement, any percutaneous procedure (eg, coronary angiography and paracentesis).
c This refers to the status of the patient prior to infection onset (include bed-ridden).
d This includes dementia, disorientation, confusion, or coma.
e An acute rise in serum creatinine level: ie, >0.3 mg/dL, by >50% of baseline, or a decrease in urine output for >6 h.
Statistical analyses
All analyses were performed using Stata version 13.1 software (StataCorp, College Station, TX). Regarding model validation, all variables of interest were tested using a forward-stepwise logistic regression model requiring a P value <.15 by the likelihood ratio test for inclusion. After the addition of significant variables, variables with a P value ≥.20 were excluded. Variables with a negative coefficient in the model were then excluded for the utility of the prediction score. Each remaining parameter was assigned a score equal to 10 times its coefficient, and scores for individual parameters were summed to determine a total score. A receiver operating characteristic (ROC) curve was generated and the area under the curve (AUC) was calculated to assess model performance. Sensitivity, specificity, positive predictive values (PPV) and negative predictive value (NPV) were calculated following the assignment of a cutoff score. A free web-based calculator was created that responds to all platforms of stationary and mobile devices.
Results
Overall, 4,114 patients were included: 2,472 patients in the derivation (n = 1,536) and validation (n = 936) data sets of the MDRO UA score and 1,642 patients in the derivation (n = 802) and validation (n = 840) data sets of the nosocomial XDRO score.
Descriptive characteristics of individuals in the derivation data sets
We included 1,536 consecutive adults with sepsis UA and 802 with nosocomial sepsis. Most patients were elderly (53.8% of the UA data set and 77.1% of the nosocomial data set), with a mean and median age of 62 ± 22 years for the UA data set and 77 years (range, 18–103) for the nosocomial data set. Individuals in both data sets had frequent interaction with healthcare settings and invasive procedures prior to the index hospitalization, including permanent residency at long-term care facilities (11% of the UA data set and 19% of the nosocomial data set), recent (<6 months) invasive procedure (15% of the UA data set and 47% of the nosocomial data set), and previous recent (<3 month) hospitalizations (25% of the UA data set and 39% of the nosocomial data set). The mean Charlson combined condition scores were 4.2 ± 3.5 for the UA data set and 8 ± 3.8 for the nosocomial data set. Reference Charlson, Pompei, Ales and MacKenzie26 Past MDRO carriage was evident among 12% of the UA data set and 33% of the nosocomial data set, and previous recent (<3 months) antibiotic therapy was documented among 41% of the UA data set and 76% of the nosocomial data set. Respiratory infections were the most common infectious syndrome among patients with sepsis UA (36%) and among patients with nosocomial sepsis (43%). The complete descriptive analysis of both populations is provided in Supplementary Table 1 (online).
Microbiological distribution of offending pathogens (derivation data sets)
Definitive microbiological diagnosis of the causative offending pathogen(s) was achieved in 33% (501 of 1,536) of patients with sepsis UA (19% of them had bacteremia) and among 56% (448 of 802) of patients with nosocomial sepsis (20% had bacteremia). Of the pathogens associated with sepsis UA, 165 (11%) were MDROs, most commonly ESBLs (6%), P. aeruginosa (3%), and MRSA (1%). Of patients with nosocomial sepsis, 21% were XDROs, mainly CRAB (12.5%), CRPA (2.4%), and CRE (2.4%). The complete microbiological descriptive data are provided in Supplementary Table 3 (online).
The MDRO upon admission score
Patients with MDRO infection were significantly older and had higher rates of recent exposure to healthcare and to antibiotics (Table 1). Multiple background conditions were associated with MDROs, including impaired functional status and cognition at baseline as well as enhanced acute illness indices. As expected, DAAT was more common in patients with MDROs, and clinical outcomes were worse (Table 1).
Multivariable analysis of risk factors was performed, and the final score components are depicted in Table 2. A cutoff of ≥22 points yielded 86% sensitivity, 67% specificity, 24% PPV, 98% NPV, and an ROC AUC of 0.85 (95% confidence interval [CI], 0.82–0.88) (Fig. 1). The application of the Duke-2002 criteria Reference Friedman, Kaye and Stout8 to this data set yielded an ROC AUC of 0.62 (70% sensitivity, 61% specificity, 18% PPV, 94% NPV), and the modified Duke-2002 criteria Reference Cardoso, Almeida and Friedman10 yielded an ROC AUC of 0.69 (68% sensitivity; 61% specificity; 17% PPV; 94% NPV). The APACHE-II Reference Lee, Lim and Hong12 and the CURB-65 (only among patients with pneumonia) Reference Liu, Xu and Zhou13 yielded ROC AUCs of 0.8 and 0.75, respectively.
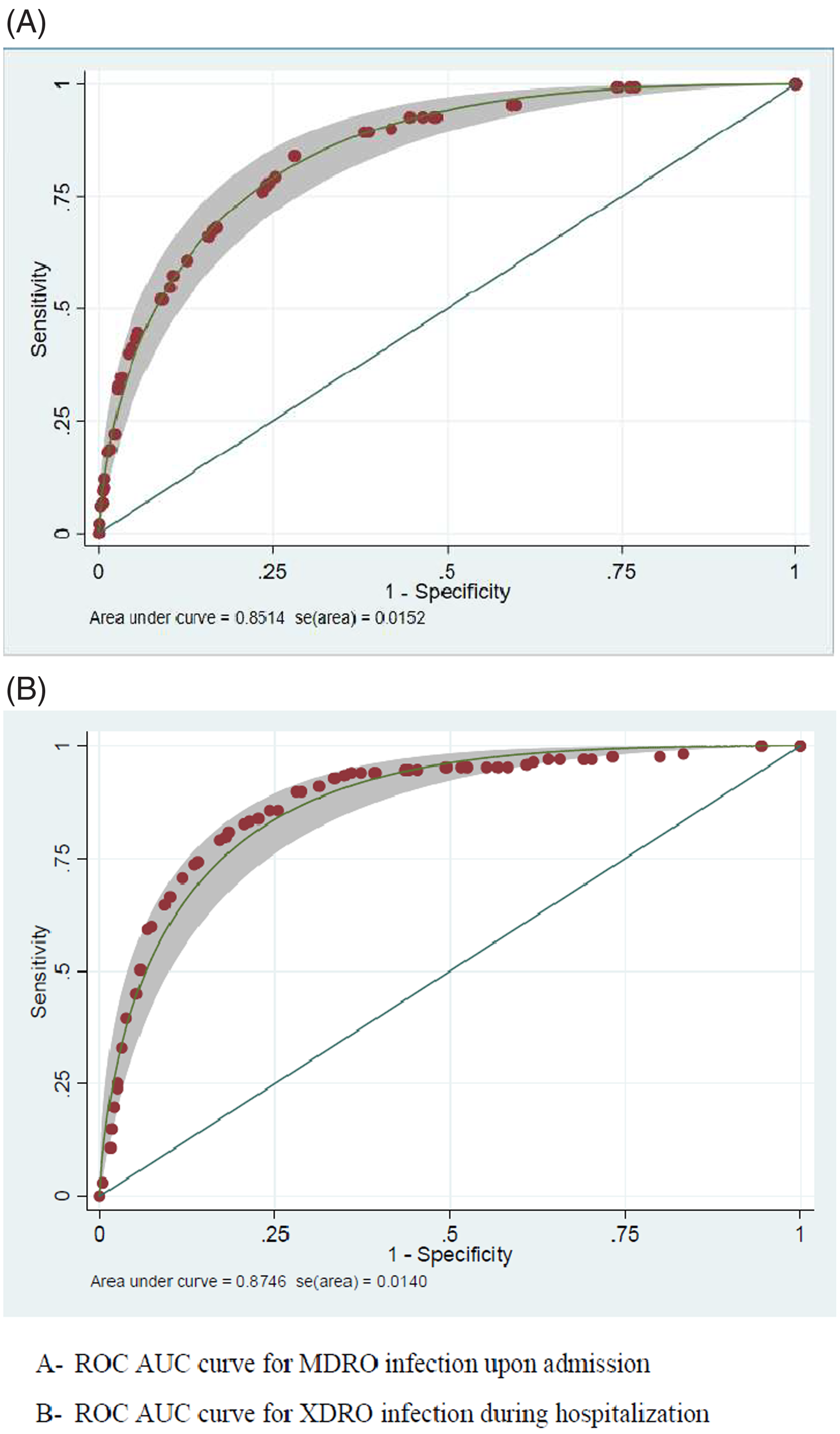
Fig. 1. ROC AUC curve for MDRO-scoring system and XDRO-scoring system. (A) ROC AUC curve for MDRO infection upon admission. (B) ROC AUC curve for XDRO infection during hospitalization. Note. ROC AUC, area under the receiver operating characteristic curve; MDRO, multidrug-resistant organisms; XDRO, extensively drug-resistant organisms.
The nosocomial XDRO score
Among patients with nosocomial sepsis, prior exposures to healthcare settings were much more substantial among patients with XDROs including recent long-term care facility (LTCF) stays, exposure to antibiotics, or past carriage of MDRO/XDRO. Indexes of comorbidities Reference Charlson, Pompei, Ales and MacKenzie26 and acute illness indices (ie, severity of sepsis, Reference Singer, Deutschman and Seymour27 Pitt bacteremia score, Reference Paterson, Ko and Von Gottberg33 and McCabe score Reference Bion, Edlin, Ramsay, McCabe and Ledingham34 ) were also elevated among XDRO patients. Patients with nosocomial XDRO infections had frequent DAAT, and in nearly half of these patients, appropriate initiation of therapy was delayed for >2 days. This delay was reflected in significantly worse outcomes (Table 1). The final nosocomial XDRO score included 7 parameters as depicted in Table 3. Using 36 points as the cutoff yielded 90% sensitivity, 72% specificity, 46% PPV, 97% NPV, and an ROC AUC of 0.87 (95% CI, 0.84–0.91) (Fig. 1).
Table 3. Multivariable Model of Risk Factors for Nosocomial XDRO Infection (derivation data set)

Note. XDRO, extensively drug-resistant organism; ICU, intensive-care unit; MDRO, multidrug-resistant organism.
a MDRO include (1) Methicillin-resistant staphylococcus aureus (MRSA), (2) ampicillin non-susceptible enterococci, (3) penicillin or ceftriaxone nonsusceptible Streptococcus pneumoniae, (4) Pseudomonas aeruginosa, (5) Acinetobacter baumannii, and (6) Enterobacterales nonsusceptible to 1 or more third-generation cephalosporin (eg, ceftriaxone, ceftazidime).
b This refers to the status of the patient prior to infection onset (include bed-ridden).
c This refers to any type of invasive procedure, including any type of surgery (from minor to major, ie, the whole spectrum), endoscopies, permanent central line insertions, any percutaneous procedure (eg, coronary angiography), ascites paracentesis, and more.
The validation data sets
Table 4 reflects the performances of the 7 different validation historical data sets. The ROC AUC range was 0.7–0.88 among the validation data sets of the MDRO UA score, and the ROC AUC range was 0.66–0.75 among the validation data sets of the nosocomial XDRO score.
Table 4. Score Performance Characteristics (Validation Data Sets)
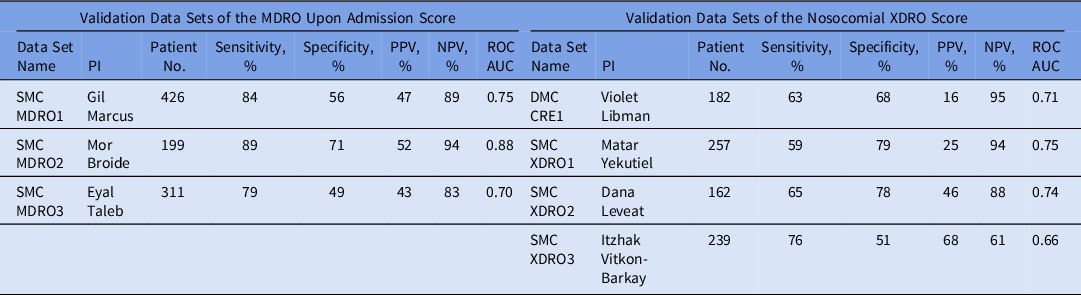
PI, primary investigator; MDRO, multidrug-resistant organism; XDRO, extensively drug-resistant organism; ROC-AUC, the area under the receiver operating characteristic curve; SMC, Shamir Medical Center; PPV, positive predictive value; NPV, negative predictive value.
Web calculator
We created a free web calculator (https://assafharofe.azurewebsites.net).
Discussion
In this study, we present a simple, bedside tool to assist in empiric prescription of antibiotics during the first encounter with a septic patient. This tool could be implemented as a stewardship intervention in hospitals. For the past 80 years, antibiotics have been an efficacious and safe therapeutics, but there are concerns of it becoming a ‘wasted resource,’ Reference Lopez-Hernandez, Alarcon and Lopez-Brea35 due to frequent errors in empiric prescription of broad-spectrum agents. Reference Namdari, Tan and Dowzicky36 Practitioners need reliable tools to guide empiric decisions and to avoid errors, which can be harmful to patients and to facilities. To our knowledge, this tool is among the largest score creation efforts for MDROs and XDROs conducted to date. In comparison with previous studies, Reference Martin, Tansek and Collins21,Reference Vazquez-Guillamet, Vazquez, Micek and Kollef22,Reference Hebert, Gao and Rahman24 the performance of our calculator was better. Moreover, this calculator addresses a wider range of situations, reflecting the broader perspective anticipated from a clinician, while managing a patient with acute sepsis. The high NPVs in both scores, of 98% and 96%, could assist prescribers in safely avoiding overuse of broad-spectrum agents. This tool could be a simple and effective stewardship intervention; broad-spectrum agents, on top of their detrimental ecological impact and the fiscal burden they impose, are also frequently less efficacious and more toxic to the patient. Reference Wong, Wong, Romney and Leung6 Prescribers are already using “prediction tools” that are less established were not originally developed to predict resistances and displayed much lower performances (both in the original studies and herein): the Duke-2002, Reference Friedman, Kaye and Stout8 modified Duke-2002, Reference Cardoso, Almeida and Friedman10 APACHE-II, Reference Lee, Lim and Hong12 and CURB-65 (for patients with community-onset pneumonia). Reference Liu, Xu and Zhou13
Mobile devices are used nowadays by nearly every prescriber worldwide, including in developing countries. The calculator is responsive with all devices and is compatible with network security settings. A shortcut to this calculator could be pasted to home screens of private devices, and to organizational computers located at ERs, regular floors, and in ICUs. The calculator is anonymous; it is designed to assist hospitalists, internists, surgeons, intensivists, clinical pharmacists, and every adult prescriber. However, the calculator should not be used in lieu of a comprehensive medical evaluation, and it should not replace infectious diseases (ID) consultation when appropriate. ID experts will always be needed to tailor management, and in many facilities, they are responsible for prescription of broad-spectrum antibiotics. Therefore, the calculator may be able also to assist prescribers in determining when a formal ID consultation is needed. Sometimes a unique clinical or epidemiological finding is evident: a pathognomonic rash, travel to a certain area, acknowledgement of current epidemics. These unique scenarios necessitate special management considerations and usually the direct involvement of an ID specialist. When no unique exposure or finding is evident, prescribers frequently think broadly of 4 categories of pathogens among septic adults: gram-positive organisms (sometimes with special focus on Enterococcus), gram-negative organisms, anaerobes, and fungi. Anaerobes and fungi (mainly Candida spp) are relevant in certain syndromes (eg, intra-abdominal infections, catheter-related bloodstream infections) or other scenarios (eg, following a gastrointestinal endoscopic procedure); therefore, they should be managed based on a different set of prediction rules. Reference Marcus, Levy and Salhab28 The purpose of this calculator was to assist clinicians, at the bedside, with prescribing anti–gram-positive agents and anti–gram-negative empiric regimens.
Our study has several limitations. The tool is based on broadly applicable determinations, both in terms of the definitions we used for stratifying the level of resistance (ie, MDRO and XDRO), and our extrapolations to the spectrum of antibacterial coverage by agents. The calculator should also be validated in other centers, to determine its generalizability. On the validation data sets that it was tested, with all historical cohorts with data limited to specific subgroups, we observed a wide range of performances, some unfavorable. The performances with the validation data sets derive the overall performance of the tool. However, in 6 of the validation data sets, only patients with positive blood cultures were included, as opposed to the derivation data set, which included the entire background population from which the cases (ie, patients with MDRO or XDRO) arose. If MDROs or XDROs are not prevalent in a facility, the tool is irrelevant, though this situation is uncommon nowadays. In some hospitals, XDRO infections may be prevalent upon admission, but this situation is rare. Reference Adler, Friedman and Marchaim37 The performance of the calculator might differ according to the percentages of MDROs and XDROs in different populations and might change over time, in accordance to temporal changes in MDROs and XDROs epidemiology. An additional limitation of our study is that the calculator does not address certain possible additional empiric prescribing dilemmas (eg, enterococcal, anaerobe, and fungal infections). Reference Marcus, Levy and Salhab28 However, it addresses the ‘backbone’ of empiric regimens for the majority of situations.
To conclude, we developed a tool to assist prescribers confronted with septic patients, which is free, anonymous, and accessible at the bedside, though it lacks additional validated data. Our future goal is to test the tool in a prospective interventional study on several outcomes, considering DAAT, mortality rates, and outcomes that measure the ecological burden of antimicrobials.
Supplementary material
To view supplementary material for this article, please visit https://doi.org/10.1017/ice.2020.1372
Acknowledgments
Financial support
No financial support was provided relevant to this article.
Conflicts of interest
All authors report no conflicts of interest relevant to this article.