The prevalence of childhood overweight and obesity (OW/OB) remains a public health crisis( Reference Ogden, Carroll and Kit 1 , Reference Dietz 2 ). National surveys indicate that children’s diets are lacking in fruits and vegetables and other nutrient- and fibre-rich foods( Reference Mun and Krebs-Smith 3 ), while as much as 40 % of children’s dietary intake comes in the form of high-fat, high-sugar foods( Reference Reedy and Krebs-Smith 4 ). To draw conclusions about the contribution of children’s diet to risk of obesity, valid and reliable dietary intake data are crucial. However, current dietary assessment methods, including 24 h recalls, FFQ, and manual and assisted diet records, are highly prone to recall bias and misreporting, making it difficult to obtain accurate estimates of children’s dietary intake( Reference Bingham 5 – Reference Basiotis, Welsh and Cronin 8 ).
In addition to the difficulties associated with dietary measurement instruments mentioned above, previous research has also illuminated systematic misreporting of dietary intake according to individual characteristics( Reference Bingham 5 ). Studies in adults have identified older age, higher BMI and female sex as predictors of under-reporting dietary intake( Reference Murakami and Livingstone 9 ), suggesting that specific person-level characteristics may be associated with accuracy of dietary recall. In children, developmental considerations pose an additional challenge. Studies have found that young (i.e. up to 12 years of age) children’s accuracy in independently reporting 24 h dietary intake is poor when compared with direct observation( Reference Diep, Hingle and Chen 10 ). Despite this, many studies have used 24 h recall methods to assess diet in children( Reference Livingstone and Robson 11 ), often enlisting the assistance of a proxy, or adult household member( 12 ). Although the use of 24 h recall in children under the age of 11 or 12 years is still generally regarded as having low reliability, employing parent assistance generally improves accuracy of children’s report( Reference Burrows, Martin and Collins 13 ). With regard to the accuracy of children’s report of dietary intake, several characteristics have been identified as contributing factors( Reference Sharman, Skouteris and Powell 14 ). A review of forty-five studies reporting on the accuracy of children’s (ages 6–12 years) dietary reporting by Sharman and colleagues( Reference Sharman, Skouteris and Powell 14 ) found that retention interval (i.e. shorter length of time between consumption and report) was the most important factor contributing to accuracy, as well as other person-level characteristics, including older age and lower social desirability. In contrast to the adult literature, that review found that other characteristics, such as child sex and race/ethnicity, had no association with accuracy of reporting dietary intake( Reference Sharman, Skouteris and Powell 14 ). For all these reasons, it has become widely recognized in the research community that new dietary assessment methods that can reduce or eliminate misreporting and recall bias are needed, especially when measuring diet in children.
In light of the limitations inherent to food frequency and 24 h recall methods, innovative techniques have emerged( Reference Illner, Freisling and Boeing 6 ), including ecological momentary assessment (EMA), a set of real-time data capture tools in which participants are repeatedly assessed during their daily lives( Reference Shiffman, Stone and Hufford 15 ). EMA is a novel method that allows for the real-time assessment of dietary intake( Reference Dunton 16 ) and which may provide a low-cost, feasible method of collecting dietary data. Using EMA to assess dietary intake in children may offer several advantages over traditional methods, including the reduction in time lapse between consumption and report from a full day to just a few hours and subsequent reduction of recall bias and improvement of recall accuracy, as well as reduced burden of reporting as compared with more time-intensive methods. In the effort to determine if EMA can be used to facilitate dietary recalls in children, it is important to compare EMA-reported dietary intake against 24 h recalls, the gold standard method of obtaining detailed, day-level dietary intake information( Reference Illner, Freisling and Boeing 6 ). Specifically, it is necessary to determine whether EMA-reported dietary intake is consistent with data produced by 24 h recalls. It is important to note that, although traditional dietary assessment methods such as the 24 h recall seek to determine what individuals are eating, when they are eating and how much (portion size) they are eating, current EMA methods may capture only some of these dimensions of dietary intake. However, depending on the research question, an understanding of whether specific types of foods were eaten within a given time window or not may be sufficient, and may be particularly amenable to EMA methods.
The primary goal of the present study was to provide preliminary evidence to support the use of EMA to collect dietary intake data in children, specifically to assess children’s report of consumption of target food categories. Specifically, we examined the overall concordance between children’s dietary intake data collected by EMA and 24 h diet recalls, by pre-specified food groups, during matched two-hour time windows over one weekend day and one weekday. Additionally, in cases of discordance (i.e. cases in which intake of a specific food type was reported in one source and not in another for a given time window), we examined whether reporting was more likely in EMA or 24 h recall, and whether this differed by food type. Finally, we determined whether the correspondence of reporting between EMA and 24 h recall differed by person-level factors previously shown to limit traditional methods of dietary assessment (i.e. sex, age, ethnicity and weight status).
Methods
Participants
Participants included mother and child dyads recruited from greater Los Angeles County, USA, in the Mothers’ and Their Children’s Health (MATCH) study. MATCH is a longitudinal investigation of the impact of maternal stress and behaviour on children’s obesity trajectories; detailed information on the study design and sample is published elsewhere( Reference Dunton, Liao and Dzubur 17 ). The current study uses cross-sectional data from the first wave of data collection. The study inclusion criteria for mother/child dyads were: (i) currently in 3rd–6th grade (child); (ii) reside together at least 50 % of time (mother and child); and (iii) ability to speak and read in English or Spanish (mother and child). Study exclusion criteria were: (i) use of medication for thyroid or psychological condition (mother); (ii) a health condition limiting ability to be physically active (mother or child); (iii) enrolled in a special education programme (child); (iv) currently using oral or inhalant corticosteroids for asthma (mother or child); (v) pregnancy (mother); (vi) underweight (BMI<5th percentile for age and sex; child); and (vii) working more than two evenings (between 17.00 and 21.00 hours) during the week or more than one eight-hour weekend shift (mother). The Institutional Review Boards at the University of Southern California and Northeastern University approved all aspects of this study. The current study focused solely on dietary data collected from the children in the MATCH study.
Procedures
Children, along with their mothers who were also participating in the MATCH study, came in for an initial in-person visit at a school or community centre. Anthropometric measurements were taken; height was measured in duplicate using a portable statiometer to the nearest 0·1 cm and weight was measured in duplicate to the nearest 0·1 kg using a Tanita scale. Mothers completed surveys reporting on their child’s race and ethnicity. Children reported their age and sex. Mothers and children were then trained on use of the study application, each on their own device. Participants who had their own Android-compatible smartphone were asked to download the study app on their own phone, and participants without a smartphone or with a non-Android smartphone borrowed a MotoG study smartphone (Motorola Mobility, USA) for use during the study period.
Dietary assessment measures
Ecological momentary assessment
Children were asked to carry the study smartphone with them at all times while they were awake, with the exception of time spent in non-compatible activities (i.e. showering, sleeping), for the following eight-day study period. Day 1 of the study protocol began in the evening and the protocol ended in the evening of day 8. Thus, days 1 and 8 were partial days. EMA surveys were prompted via the smartphone app in a stratified random sampling scheme; during weekdays, surveys were prompted up to three times during after-school hours (once at each of the following time windows: 15.30–16.00 hours, 17.30–18.00 hours and 19.30–20.00 hours) and, during weekend days, surveys were prompted up to seven times (once at each of the following time windows: 07.30–08.00 hours, 09.30–10.00 hours, 11.30–12.00 hours, 13.30–14.00 hours, 15.30–16.00 hours, 17.30–18.00 hours and 19.30–20.00 hours). Surveys took children approximately 2 min to complete, and children were trained to complete the surveys independently, without parental assistance. Through an audible notification, children were asked to pause their current activity to respond to the survey questions. At each EMA time point, children were presented with a ten-item survey containing the following target question: ‘In the past 2 HOURS, which of the following have you done? (Choose all that apply)’. At the first prompt of each day, children were instead asked: ‘Since you woke up this morning, which of the following have you done? (Choose all that apply)’, to capture the dietary intake that occurred over the entire day from the moment they woke up until the first EMA prompt. On the same screen, children were presented with a list of options, including ‘Eaten Fruits or Vegetables’, ‘Eaten Chips or Fries’, ‘Eaten Pastries or Sweets’, ‘Drank Soda or Energy Drinks (not counting diet)’ and ‘None’. The ‘Fruits/Vegetables’ option was selected to represent healthy foods, while ‘Chips/Fries’, ‘Pastries/Sweets’ and ‘Soda/Energy Drinks’ were selected as examples of frequently consumed high-fat, high-sugar foods and drinks, which are recommended to be limited by current My Plate guidelines( Reference Reedy and Krebs-Smith 4 ). Children could select as many of the food categories as applied and each of these response options was coded as binary, where a 0 response indicated the absence and a 1 response indicated the presence of past 2 h consumption of each food item at each prompt. These data were then aggregated to create an EMA-based, prompt-level food group consumption variable for each two-hour EMA prompt window (i.e. eaten ‘Fruits/Vegetables’, ‘Chips/Fries’, ‘Pastries/Sweets’, ‘Soda/Energy Drinks (not counting diet)’), which was coded as either 0=‘not consumed’ or 1=‘consumed’. The final EMA data set included participants and their corresponding EMA observations that were completed on days for which a participant also completed a 24 h dietary recall.
24 h Dietary recall
Children also completed up to two 24 h dietary recalls during the eight-day study period (on one weekday and one weekend day). Dietary recalls were conducted over the phone by trained staff and the data were analysed using Nutrition Data System for Research (NDSR) software, developed by the Nutrition Coordinating Center (NCC), University of Minnesota, Minneapolis, MN, USA( Reference Schakel 18 ). Whenever possible, mothers were asked to be nearby during the call and were consulted for additional information or clarification when deemed necessary by the interviewer or child. Children were first asked to freely recall what they ate during the previous calendar day; then in successive reviews of the previous-day’s food list, the interviewer probed for additional details using the multiple-pass method. Recall data were screened and checked for accuracy and plausibility of energy and nutrient-specific intakes, following a quality control process that included comparing daily total energy and key nutrient intakes against participants’ expected amounts for age and gender using reference values from the National Health and Nutrition Examination Survey, and cross-verifying data against source documentation when values were out of range, to correct any data entry errors. All 333 completed and screened recalls (mean energy=7227·4 (sd 1928·0) kJ (1727·4 (sd 460·8) kcal)) were eligible for inclusion in the present analysis. Because we aimed to assess presence or absence of foods reported across two sources (e.g. as opposed to the exact energy amount consumed on a given day), no additional exclusion criteria were applied. The meal-level food group serving count file from NDSR (File 03) was used to create a time-stamped, 24 h recall-based, meal-level food group serving count variable. For each eating occasion reported in the 24 h recall, we used NDSR food group codes to create groups of 24 h food categories to match each of the EMA categories (i.e. ‘Fruits/Vegetables’, ‘Chips/Fries’, ‘Pastries/Sweets’, ‘Soda/Energy Drinks (not counting diet)’) which were binary, in which 0=‘not consumed’ and 1=‘consumed’. For example, the NDSR codes ‘VEG0800 – Fried Potatoes’, ‘GRR0900 – Snack Chips – Refined Grain’ and ‘FMC0100 – Vegetable-based Savory Snack’ were combined to create a 24 h recall category that corresponded to the EMA category ‘Chips/Fries’. The full list of NDSR food codes included in each category, and examples of those that were excluded, are illustrated in the online supplementary material, Table S1. From this 24 h recall file, we selected days that could be linked to EMA days.
Ecological momentary assessment and 24 h recall data merging
EMA prompt windows, in which participants reported on dietary intake over the past 2 h, were used as anchors and matched (e.g. overlapping in time) 24 h dietary intake was merged into each EMA prompt window. For example, an EMA prompt completed at 17.45 hours asked about dietary intake from 15.45 to 17.45 hours. Thus, any eating occasion reported during 15.45–17.45 hours in the 24 h dietary recall data set was merged into this EMA prompt window. For each matched window, four new outcome variables of concordance were created, one for each of the four food categories (i.e. ‘Fruits/Vegetables’, ‘Chips/Fries’, ‘Pastries/Sweets’, ‘Soda/Energy Drinks (not counting diet)’). This variable was equal to 1 if an eating event for a given food category was identified as having either occurred or not occurred by both EMA and 24 h recall (e.g. both ‘Yes’ or both ‘No’) and equal to 0 if an eating event for a given food category was identified as having occurred in one source but not in the other source (e.g. ‘Yes’ in one and ‘No’ in the other). For the first prompt of the day on weekdays (which asked about intake ‘Since you woke up this morning’), the 24 h recall data for the corresponding period of time (i.e. everything reported by the participant from waking up until the EMA prompt time, a period of several hours) were merged to create the matched window. We additionally created expanding two-hour time windows by merging 24 h recall-reported intake by slightly expanded time windows, including ±2·5 min (i.e. 2 h and 5 min total) and ±5 min (i.e. 2 h and 10 min total) on either end, to examine differences in concordance rates by expanding time.
Data analysis
To examine the consistency, or concordance, with which foods within pre-specified food categories were reported by EMA and by 24 h recall, cross-tabulation analysis was used. Specifically, reports from each source were run separately for each food category and each exactly matched 2 h window. These analyses were repeated using the 2 h±2·5 min and 2 h±5 min windows to examine any differences in concordance rates by expanding time. To investigate person-level predictors of concordance, four separate multilevel logistic regression models were run; one for the concordance of reporting of each food category within a given matched two-hour time window. Multilevel modelling allows for the clustering of observations within individuals and can accommodate missing data and variable sampling windows( Reference Schwartz and Stone 19 ). The dependent variable in all models was the binary two-hour concordance variable where 1=‘concordant’ and 0=‘not concordant’. All predictors were entered into each of the models simultaneously. Person-level predictors included sex, age, ethnicity (Hispanic v. not Hispanic) and BMI category (OW/OB v. lean). Analyses were conducted in the statistical software package SAS version 9.4. Results were considered significant at P<0·05.
Results
Participant characteristics and data availability
Children were 53 % female, 51 % Hispanic, 34·0 % OW/OB, and had a mean age of 9·6 (sd 0·9) years. Table 1 displays the full demographic characteristics of the sample. Of the 202 dyads enrolled in the overall study, twenty-four children were excluded due to having no dietary recall data. An additional thirty-four children were excluded due to having no overlapping EMA and 24 h dietary recall data points (e.g. never reported dietary intake in both 24 h recall and EMA during the same window of 2 h±5 min), resulting in a sample of 144 children for cross-tabulation concordance analysis. An additional five children were excluded from the multilevel logistic regression analyses due to missing covariate data (i.e. no height and weight data for BMI category assignment). Thus, our analytical sample for logistic regression included 139 participants (Level 2) representing 249 unique days and 842 unique two-hour windows (Level 1).
Table 1 Demographic characteristics of participants: mother/child dyads recruited from greater Los Angeles County, USA, in the Mothers’ and Their Children’s Health (MATCH) study

Note: the sample comprised 144 children.
† Annual household income data were missing for one participant.
‡ Weight category was missing for five participants.
Dietary descriptive results
The mean number of matched windows per day was 4·6 (sd 1·9; range 1–7) and the mean number of matched windows per participant was 7·5 (sd 2·32; range 1–12). Across the matched windows, the proportion of EMA prompts in which children reported past 2 h consumption of each food category was as follows: ‘Fruits/Vegetables’ (24·3 %), ‘Pastries/Sweets’ (12·6 %), ‘Chips/Fries’ (10·8 %) and ‘Soda/Energy Drinks’ (9·3 %). Similarly, throughout the matched data set, the proportion of 24 h dietary recall two-hour windows in which children reported past 2 h consumption of each food category was as follows: ‘Fruits/Vegetables’ (32·0 %), ‘Pastries/Sweets’ (12·7 %), ‘Chips/Fries’ (5·0 %) and ‘Soda/Energy Drinks’ (3·8 %).
Concordance of ecological momentary assessment and 24 h dietary recall
The two-hour window reporting concordance rates for each food type are displayed in Fig. 1. Two-hour concordance was highest for ‘Soda/Energy Drinks’ (89·9 %), followed by ‘Chips/Fries’ (85·9 %), ‘Pastries/Sweets’ (78·6 %) and ‘Fruit/Vegetables’ (64·9 %). When the reports were discordant (i.e. reported in one source by not the other) the following foods were more likely to be reported in the two-hour EMA prompts v. two-hour 24 h recall windows: ‘Soda/Energy Drinks’ (7·7 % v. 2·5 %) and ‘Chips/Fries’ (9·2 % v. 4·9 %). Comparatively, the foods more likely to be reported in the two-hour 24 h recall windows v. the two-hour EMA prompts were as follows: ‘Pastries/Sweets’ (12·3 % v. 9·1 %) and ‘Fruits/Vegetables’ (24·6 % v. 10·6 %). Results also indicated that, across all food categories, concordance was highest for the exact 2 h time window. Concordance rates changed minimally (<0·5 %) when the exact time window was expanded by ±2·5 min and ±5 min (Fig. 1).
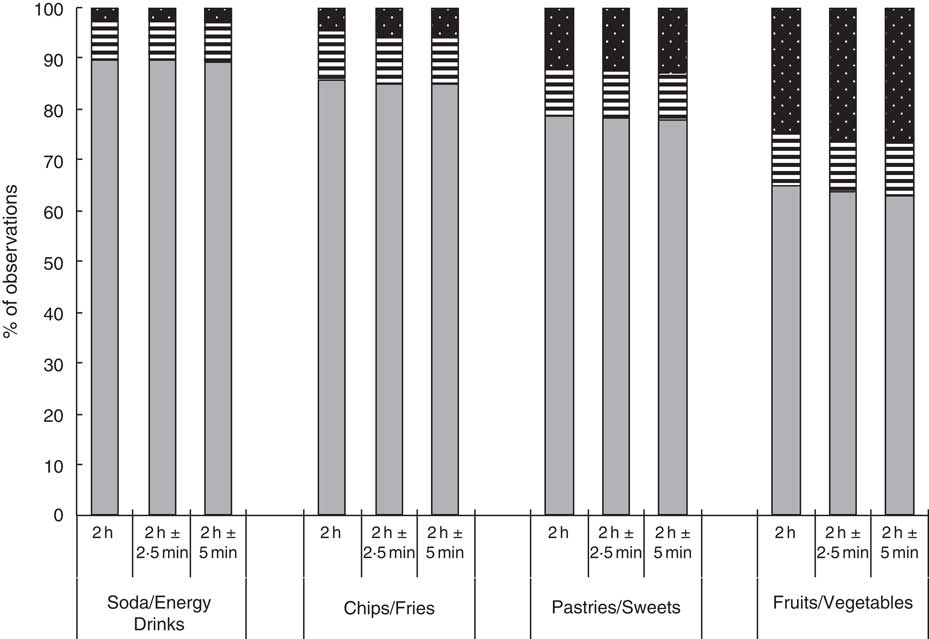
Fig. 1 Concordance of dietary intake reports across ecological momentary assessment (EMA) and 24 h recall, by food category, for matched two-hour time windows (exact 2 h time window, 2 h±2·5 min time window, 2 h±5 min time window; 879 observations), among 144 children (53 % female; mean age 9·6 (sd 0·9) years; 34·0 % overweight/obese), Los Angeles County, USA, Mothers’ and Their Children’s Health (MATCH) study. Concordance () is defined as an identical report in both report sources for a given time window (i.e. a given food item was either reported eaten or not eaten). Discordance (
, discordant, EMA only;
, discordant, 24 h recall only) is defined as a conflicting report across report sources for a given time window (i.e. a given food was reported eaten in one source but not the other)
Person-level predictors of concordance
Table 2 displays the results of the multilevel model examining predictors of concordant reporting. Several person-level characteristics were associated with the concordance of reporting across food category. OW/OB children were more likely to report concordant information across sources for a given two-hour window for ‘Soda/Energy Drinks’ (OR=2·01; 95 % CI 1·06, 4·04) and ‘Sweets/Pastries’ (OR=1·61; 95 % CI 1·03, 2·52). Results also revealed significant associations of child sex with the concordance of reporting such that girls (v. boys) were more likely to report concordant information across sources for a given two-hour window for ‘Fruits/Vegetables’ (OR=1·36; 95 % CI 1·01, 1·83). Additionally, Hispanic (v. non-Hispanic) children had greater odds of concordant reporting for ‘Pastries/Sweets’ (OR=1·55; 95 % CI 1·02, 2·36). Age was not associated with concordance of reporting for any outcome.
Table 2 Results of multilevel logistic regression predicting concordance of reporting by food category between two sources of dietary intake for matched time windows among 144 children, Los Angeles County, USA, Mothers’ and Their Children’s Health (MATCH) study
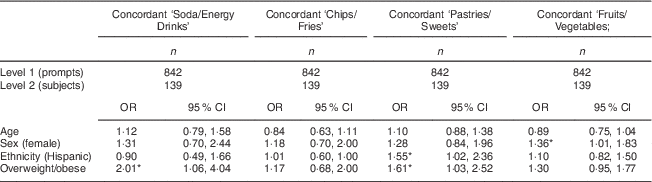
Note: Each column represents a different model, with each predicting the concordance of reporting of each of the four dietary category outcomes. All predictors were entered simultaneously into each model. Age is a centred, continuous variable. Child sex is coded as female (=1) v. male (=0). Overweight/obese (=1) as compared with lean (=0) is calculated based on the criteria of the Centers for Disease Control and Prevention (BMI at or above the 85th percentile for age and sex). Child ethnicity is coded as Hispanic (=1) v. non-Hispanic (=0).
* P<0·05.
Discussion
Overall, our results showed that concordance between dietary data collected by EMA and 24 h recall across selected food groups was high but that concordance differed by food type and by method of report. Specifically, the overall concordance rate was highest for the least frequently reported food group, ‘Soda/Energy Drinks’, and lowest for the most frequently reported food group, namely ‘Fruits/Vegetables’. By method of report, we found that ‘Soda/Energy Drinks’ and ‘Chips/Fries’ were more likely to be reported by EMA (v. 24 h recall); whereas ‘Pastries/Sweets’ and ‘Fruits/Vegetables’ were more likely to be reported by 24 h recall (v. EMA). Additionally, we found that concordance across methods in reporting ‘Soda/Energy Drinks’ and ‘Sweets/Pastries’ was more likely among OW/OB children v. lean children, that concordance of reporting ‘Fruits/Vegetables’ was more likely among girls v. boys, and that concordance of reporting ‘Pastries/Sweets’ was more likely for Hispanic children v. non-Hispanic children. Collectively, our findings show that using EMA to collect dietary data in children is comparable to 24 h recall data (at the food group level); that less frequently consumed foods are more likely to be reported by EMA, whereas more frequently consumed foods are more likely to be reported by 24 h recalls; and that concordance across methods varies significantly by important person-level characteristics and food groups.
The differing rates of concordance of reporting dietary intake across methods is notable. Children were more likely to report ‘Soda/Energy Drinks’ and ‘Chips/Fries’ by EMA than by 24 h recall, and more likely to report ‘Fruits/Vegetables’ by 24 h recall than by EMA. It may be more likely that consumption of fruits and vegetables is captured through 24 h recall, because the interviewer is able to capture instances of ‘Fruit/Vegetable’ intake that the child may have overlooked or been unaware of in the EMA report by probing the child for further information or by asking the child’s mother for meal preparation details. However, because we are comparing two imperfect sources (as compared with direct observation of actual intake), we are unable to know if discordance represents an intrusion (i.e. reporting in one source an item that was not actually eaten) or an omission (i.e. failing to report in one source an item that was actually eaten)( Reference Baxter, Hardin and Smith 20 ) in the given two-hour window. Our results also indicated that, across all food categories, concordance was highest for the exact 2 h time window, decreasing minimally (<0·5 %) when the exact time window was expanded by ±2·5 min and ±5 min. Additional post hoc analyses examined changes in concordance when expanding time windows by ±15 min and ±30 min and displayed similarly decreasing concordance with expanding time. These findings indicate that children consistently and precisely reported the presence or absence of consumption of target food items via EMA and 24 h recall at the matched time window.
Another important finding was that BMI category was positively associated with odds of concordant reporting for ‘Soda/Energy Drinks’ and ‘Pastries/Sweets’. In post hoc exploratory analyses stratifying the sample into OW/OB and lean groups, both OW/OB and lean children displayed the same rank-ordering pattern of concordance (e.g. highest for ‘Soda’, lowest for ‘Fruit’). However, although both OW/OB and lean children displayed the same rank-order pattern of concordance, post hoc ancillary analysis of the difference in concordance by weight status revealed consistently higher concordance levels across food categories for the OW/OB children as compared with the lean children. For example, across weight groups, concordance for ‘Pastries/Sweets’ was 78·6 %; however, when stratifying, concordance was 75·5 % for lean children and 83·6 % for OW/OB children. The finding that OW/OB children had greater concordance for some food types but not others is similar to what is seen in studies comparing self-report with direct observation (i.e. accuracy), where some studies observed higher( Reference Harrington, Kohler and McClure 21 ) and some lower( Reference Baxter, Smith and Nichols 22 ) accuracy for children with higher BMI, with no clear consensus overall( Reference Sharman, Skouteris and Powell 14 ). For example, in a study of changes in the reporting accuracy of children across multiple 24 h recalls, Baxter and colleagues found that overweight children had the greatest accuracy of recall (as defined by lowest intrusion and omission rates) as compared with normal-weight children. However, over the span of three recalls, each separated by a mean of approximately 40 d, overweight children’s reporting accuracy declined while the accuracy of lean children improved( Reference Baxter, Smith and Nichols 22 ). Results of the present study suggest that, when controlling for age, sex and ethnicity, OW/OB children are more likely to report consistent information across multiple sources for two out of four food categories.
Lastly, we observed greater concordance of reporting for girls (v. boys) for ‘Fruits/Vegetables’ as well as for Hispanic (v. non-Hispanic) children for ‘Pastries/Sweets’. Previous studies have largely found null differences in the accuracy of dietary recall by gender and ethnicity. In the review by Sharman et al.( Reference Sharman, Skouteris and Powell 14 ) of seventeen available studies, fifteen reported no differences in accuracy by sex, while two found greater accuracy in girls than in boys. That same review reported no differences by ethnicity in ten studies, and in one study found a tendency for Hispanic children to misreport serving sizes compared with children of other ethnicities. Compared with these studies, the present study found greater concordance of report, not accuracy, across two sources, suggesting that consistency of report might be slightly higher for girls and for Hispanic children for these food categories.
Our study has a number of strengths, including the use of two rich, time-linked dietary data sets which allowed us to capture real-time snapshots of recent dietary intake as well as detailed reports of full-day dietary intake. Additionally, the sample of children in our study is representative of the overall US population in terms of sex (53 % female) and rates of OW/OB (e.g. approximately one-third of the study population)( Reference Ogden, Carroll and Curtin 23 ). However, there were also some limitations to note. It is important to emphasize that our current analysis does not include a ground truth assessment; rather, we are comparing the concordance of report obtained from two imperfect measures. It is important to also consider the role of social desirability, which may lead children to report biased information on dietary intake based on perceptions of what they believe they should be eating( Reference Baxter, Smith and Litaker 24 ). Although social desirability is known to play a role in dietary reporting in general, we expect that any influence of social desirability would similarly influence children’s dietary report in both EMA and 24 h recall, therefore not affecting the within-person concordance rates presented here( Reference Baxter, Smith and Litaker 24 , Reference Sherwood 25 ). With regard to limitations inherent to our EMA measure of diet, because children reported on dietary intake via EMA at stratified random times, recalling dietary intake over the past 2 h, it is possible that children may have misjudged a given time window and either failed to report intake occurring just within the time window or mistakenly reported foods consumed prior to the start of the time window, leading to inaccurate report. There are also concerns regarding under- or over-reporting of dietary intake items due to EMA participant fatigue. In our study, all dietary items were presented on a single screen and children were instructed to select all that applied. Children may have advanced through this screen without selecting all appropriate items, in order to complete the surveys more quickly. Future studies may address this potential issue by presenting each of target dietary items on a separate screen with a ‘Yes’/’No’ response option. An additional limitation lies in the EMA prompting schedule; during weekdays, children are prompted to complete surveys only during after school times, with the first prompt occurring between 15.30 and 16.00 hours. Thus, to capture food intake occurring on weekdays prior to 15.30 hours, in the first prompt of the day on weekdays children are asked to report on dietary intake that occurred ‘Since you woke up this morning’, which included intake that occurred over several hours, in contrast to other EMA prompts that asked about ‘Past 2 HOURS’ intake. This could potentially lead to a difference in recall bias, due to the difference in recall length. However, in post hoc exploratory analyses limited to only these (ninety-six windows) prompts asking about intake ‘Since you woke up this morning’, the same rank-ordering pattern of concordance (e.g. highest for ‘Soda’, lowest for ‘Fruit’) was observed, suggesting that these EMA prompts did not affect our overall results. Our EMA item did not measure quantity or portion size, only absence or presence of item(s), which prevented us from studying the EMA-reported number of servings of separate eating events of a given food category within a given two-hour window. Additionally, it is unknown how children interpreted each of our EMA food categories or how they perceived what is a fruit/vegetable. For example, some children may have considered only whole fruit or vegetable intake (e.g. an apple or carrot sticks) when choosing to report having consumed ‘Fruits/Vegetables’, while other children may have been less strict in their interpretation by choosing to report having consumed fruits or vegetables that were part of a mixed meal. It is also not known if children misclassified fruit- or vegetable-flavoured snacks or candies as a fruit or vegetable. Finally, it is possible that using EMA in conjunction with 24 h recall may lead children to recall their previously reported EMA dietary intake at the time they complete the 24 h recall, potentially improving recall.
One limitation inherent in using 24 h recall to assess diet in children aged 8–12 years is the potential unsuitability of this method for assessing accurate dietary information( Reference Diep, Hingle and Chen 10 ); we have attempted to mitigate this weakness by enlisting parental assistance when available, a commonly employed technique( 12 ), which has been shown to improve overall accuracy of children’s dietary reporting( Reference Burrows, Martin and Collins 13 ). An additional limitation which may be present when merging the EMA with the 24 h dietary data is the inherent subjectivity involved in selecting the NDSR food codes to include in each food category. For example, we did not include NDSR code ‘FMC0100 – Vegetable-based Savory Snacks’ as a component of the ‘Fruits/Vegetables’ category; although food items of this type may contain vegetables, they do not necessarily represent the healthy type of food that the category intended to capture, nor is it clear that children, upon eating such an item, would report it was ‘Fruits/Vegetables’ within their subsequent EMA assessment. Another potential limitation inherent to the 24 h dietary intake data concerns the time stamping of eating occasions. Although interviews assess the time of each eating occasion, they do not assess duration or end time. This may be relevant in cases where meals, snacks or drinks are consumed over longer periods of time (i.e. drinking a bottle of soda over the course of a day), for which the 24 h dietary recall would record one eating occasion while the EMA would capture consumption that occurs over multiple prompts as separate eating events.
The present results indicate that EMA-collected dietary data and 24 h dietary recall data (at the food group level) are comparable, suggesting that EMA may be used alone or in combination with 24 h dietary recalls when assessing dietary intake in specified time intervals. Although the concept of concordance in the present study is not meant to represent accuracy, it may indicate consistency of subject reporting of dietary intake across sources. This may be of clear benefit to studies with limited resources to conduct costly and time-consuming 24 h dietary intake recall interviews for each participant. Furthermore, EMA methods may provide a useful tool for studies that are also interested in obtaining contextual information about when, where and with whom eating has occurred. Given the rise in use of mobile technologies to gather information from participants in real time, future research should continue to explore the use of EMA-facilitated dietary recalls in children.
Acknowledgements
Financial support: This research was supported by the National Heart, Lung, and Blood Institute (G.F.D., grant number R01HL119255) and the American Cancer Society (G.F.D., grant number 118283-MRSGT-10-012-01-CPPB); and partially supported by the University of Southern California Graduate School Provost Fellowship (S.G.O), the National Institutes of Health Cancer Control and Epidemiology Research Training Grant (S.G.O, grant number T32CA009492) and the National Heart, Lung, and Blood Institute (S.G.O., grant number F31HL137346). None of the study funders had a role in the design, analysis or writing of this article. Conflict of interest: None. Authorship: S.G.O. analysed the data and drafted the manuscript. S.S. assisted with analysis and manuscript revision. W.K. and E.D. assisted with data preparation, analysis and manuscript edits. G.F.D. designed the study, assisted with analytical strategy and manuscript preparation. Ethics of human subject participation: This study was conducted according to the guidelines laid down in the Declaration of Helsinki and all procedures involving human subjects were approved by the Institutional Review Boards at the University of Southern California and Northeastern University. Written informed consent was obtained from all subjects.
Supplementary Material
To view supplementary material for this article, please visit https://doi.org/10.1017/S1368980017003780