1. Introduction
Speech signal unfolds rapidly over time. To arrive at a proper understanding of the message, listeners need to process multiple levels of information (e.g. acoustic encoding, lexical access, syntactic processing, and semantic integration). Such processing needs to be accomplished efficiently and quickly within a limited time, which provides a big challenge to the human brain. Predictive language processing has been proposed to reduce this burden (Friston, Reference Friston2010) by allowing listeners to use prior knowledge and current contextual information to predict upcoming words before they are actually spoken (Kuperberg & Jaeger, Reference Kuperberg and Jaeger2016). In this way, top-down semantic constraints can assist the bottom-up sensory processing of the rapidly unfolding speech signal. The goal of this study was to further understand whether and how listeners use semantic constraints to predict the phonological features of upcoming words in real-time speech processing.
1.1. Phonological prediction in language comprehension
An increasing number of studies have used the event-related potential (ERP) or eye-tracking method to examine the likelihood of predicting phonological information before it appears in language inputs. One particularly influential ERP reading comprehension study was conducted by DeLong et al. (Reference DeLong, Urbach and Kutas2005), in which the congruency between the target nouns’ phonological forms (e.g. kite versus airplane) and their preceding indefinite articles (a versus an) was manipulated. Their results showed a reduced N400 ERP as a function of high cloze probability at both nouns (e.g. kite) and articles (e.g. a), suggesting pre-activation of the phonological aspects of highly predictable nouns.
Subsequent studies combined the eye-tracking technique with the visual world paradigm (VWP) to further examine the robustness of phonological prediction in speech processing (e.g. Ito, Reference Ito2019; Ito et al., Reference Ito, Pickering and Corley2018; Li et al., Reference Li, Li and Qu2022; Shen et al., Reference Shen, Hyönä, Wang, Hou and Zhao2021). In this paradigm, listeners are presented with words or pictures on the screen while they listen to incoming stimuli. Their fixations on printed words or pictures are driven by lexical activation (Tanenhaus et al., Reference Tanenhaus, Magnuson, Dahan and Chambers2000), which provides a means to examine the pre-activation of phonological information before their acoustic onset. In the eye-tracking study by Ito et al. (Reference Ito, Pickering and Corley2018), native English speakers listened to sentences containing a highly predictable word and viewed four objects. One of the objects corresponded to the predictable word and one to a phonological competitor whose initial phoneme shares with the target word, in addition to two pictures for unrelated words. Their participants fixated more on the target object, and the phonological competitor before the target word was presented in the sentence, suggesting the pre-activation of phonological information. This phonological pre-activation started around 500 ms before the target word onset and lasted for about 150 ms. Similarly, Shen et al. (Reference Shen, Hyönä, Wang, Hou and Zhao2021) found that speakers of Standard Chinese allocated more fixations to the phonological competitor (with an onset overlap to the predicted target), compared with the unrelated distractors. The phonological pre-activation lasted for about 100 ms. In short, the above studies indicate that during on-line language comprehension, the human brain can predict the phonological information of an upcoming word before it appears as the bottom-up speech input, and the phonological pre-activation effect in the above VWP studies is usually short-lasting and therefore only detectable for a short period of time.
Phonological prediction in language comprehension not only appears to be brief but also may be weak and inconsistent. The phonological pre-activation effect found in Shen et al. (Reference Shen, Hyönä, Wang, Hou and Zhao2021) was only marginally significant. Regarding ERP studies, attempts to replicate the phonological form consistency effect observed by DeLong et al. (Reference DeLong, Urbach and Kutas2005) have also been unsuccessful (Ito et al., Reference Ito, Martin and Nieuwland2017; Nieuwland et al., Reference Nieuwland, Politzer-Ahles, Heyselaar, Segaert, Darley, Kazanina and Huettig2018). In addition, Ito et al. (Reference Ito, Pickering and Corley2018) found that native English speakers were able to predict phonological information in their native language, but this effect was not observed in L2 listeners whose native language was Japanese. A recent study by Ito and Sakai (Reference Ito and Sakai2021) also found no evidence of phonological prediction when native Japanese speakers listened to Japanese sentences. These observations suggest that the human brain does not always predict the phonological forms of upcoming words, even in highly predictive context. Further research is therefore needed to understand the extent to which phonological forms are pre-activated during language comprehension and the factors that influence this predictive process.
1.2. Mechanisms underlying flexible prediction
The inconsistent findings regarding the presence (or absence) of phonological prediction may be due to a variety of factors, such as processors’ prior knowledge, the level of context constraint, and the usefulness of phonological prediction in the current processing situation. Some of the factors have been shown to affect the likelihood or extent of phonological prediction (e.g. Li et al., Reference Li, Ren, Zheng and Chen2020, for long-term tonal experience; Linderholm, Reference Linderholm2002, for text constraints; and Zheng et al., Reference Zheng, Zhao, Yang and Li2021, for the difficulty of speech processing). These modulating factors, particularly the utility of prediction for the task at hand, are closely related to the two cognitive systems proposed to support predictive language processing.
According to Huettig (Reference Huettig2015), predictive language processing relies on two non-contradictory systems. According to ‘dumb’ System 1, a specific word can be pre-activated due to automatic activation spreading from its semantically associated words in the context, and this lexical pre-activation, in turn, is likely to automatically spread to its associated phonological level of representation in the mental lexicon, with no possibility of flexible adaptation (Huettig, Reference Huettig2015; Kukona, Reference Kukona2020). In contrast, System 2 suggests that language prediction can be flexibly and strategically generated through top-down processing mechanisms. This type of prediction is considered cognitively demanding and associated with a generative architecture of comprehension (Huettig, Reference Huettig2015; Kuperberg & Jaeger, Reference Kuperberg and Jaeger2016). For example, the higher message-level information within our internal representation of a sentence or discourse context can be used to pre-activate an upcoming word. This already pre-activated lexical representation, when held with a high degree of certainty, can be used strategically by the processor to pre-activate the lower-level(s) phonological information. In real-time speech understanding, the balance between the benefit and cost of top-down prediction is important for efficient processing. Even in a highly constraining context, an efficient processor is expected not to be engaged in phonological prediction if it is not useful for the task at hand. Thus, according to System 2, the likelihood or strength of phonological prediction can be flexibly adjusted based on factors such as the utility of prediction and the credibility of contextual constraint.
There is evidence that lexical or semantic prediction in language comprehension is flexible (Brothers et al., Reference Brothers, Swaab and Traxler2017, Reference Brothers, Dave, Hoversten, Traxler and Swaab2019). For example, the benefit of predictive context, as indicated by reduced N400 effects for predicted target words, was larger and occurred earlier when sentences were spoken by reliable speakers (who tended to complete sentences with predictable words) compared with those produced by unreliable speakers (who tended to complete sentences with plausible but unpredictable words) (Brothers et al., Reference Brothers, Dave, Hoversten, Traxler and Swaab2019). A recent listening comprehension study demonstrated further that the context-based lexical prediction effect could be strategically enhanced for musician listeners in non-ideal listening situations where top-down lexical prediction was more helpful (Zheng et al., Reference Zheng, Zhao, Yang and Li2021). In short, the above studies suggest that the human brain can flexibly adjust its lexical or semantic level of prediction to comprehend language. What needs to be further clarified is the extent to which such a mechanism of flexible prediction can generalize to prediction at the phonological level.
1.3. This study
This study aimed to investigate further the extent to which listeners predict the phonological forms of upcoming words during on-line speech comprehension. Furthermore, we were interested in whether phonological prediction can be flexibly adjusted based on factors such as its usefulness in speech processing.
To this end, the eye-tracking technique and a printed-word version of the VWP were employed. This printed-word version of VWP has been found by previous studies to be sensitive to phonological manipulations during spoken sentence processing (Huettig & McQueen, Reference Huettig and McQueen2007; Shen et al., Reference Shen, Hyönä, Wang, Hou and Zhao2021; but see Yang & Chen, Reference Yang and Chen2022). In this study, participants listened to their native Mandarin Chinese spoken sentences while viewing a scene consisting of two single characters printed on the screen, one of which was the critical word and the other was a distractor word. The spoken sentences contained a highly predictable target word (e.g. bamboo: ‘In order to feed the panda, Mr. Wang brought here some bamboo by car’). The phonological relationship between the target word in the spoken sentence and the printed critical word on the screen was manipulated, and the fixation ratio over the critical word was calculated to estimate phonological activation.
One innovative aspect of our study was to investigate whether phonological information is pre-activated during on-line speech processing and what the representational nature of such prediction is. In particular, we were interested in whether suprasegmental lexical tone can be separately pre-activated, as tonal information plays a critical role in distinguishing lexical-semantic meanings in Mandarin Chinese (Yang & Chen, Reference Yang and Chen2022, and references therein). Shen et al.’s (Reference Shen, Hyönä, Wang, Hou and Zhao2021) study on Chinese has shown that while listening to a highly predictable sentence, participants allocated more fixations towards competitors (which share both segmental and tonal information with the spoken target word), compared with distractors. Although their study suggested that lexical tone can be pre-activated together with the tone-carrying segmental syllable, it remains unknown whether lexical tone can be independently pre-activated without its accompanying segmental information. This issue was addressed in this study.
We manipulated the critical word printed on the screen as (1) the highly predicted target word itself, (2) a homophone competitor (sharing all segmental and tonal information with the target word), (3) a tonal competitor (sharing only the same lexical tone with the target word), or (4) an unrelated word.
Another innovative aspect of the study was to tap directly into the role of task in phonological predictive processing. One group of participants (Experiment 1a) completed a ‘word judgement’ task, in which listeners were asked to determine whether the spoken sentence mentions any of the words in the visual array. Another group (Experiment 1b) completed a ‘pronunciation judgement’ task, in which listeners determined whether words shown on the screen overlapped phonologically with any of the words in the oral sentence they had just heard. If listeners could predict the phonological aspects of an upcoming target word during real-time language comprehension, the fixation proportion on the phonological competitor (i.e. homophone competitor or tonal competitor) should be significantly higher than that of the unrelated words.
Experiment 2 adapted the design in Experiment 1 and opted for a within-subject design to further verify the flexibility of phonological prediction. A new group of participants were recruited, and all completed both the ‘word judgement’ task (in one block) and the ‘pronunciation judgement’ task (in another block). In this way, we aimed to eliminate any potential confounding factors such as individual difference (e.g. cognitive abilities or prior knowledge) and enhance the awareness of the different goals of the two different tasks. We expected that the effect of phonological prediction would be more pronounced in the ‘pronunciation judgement’ task than in the ‘word judgement’ task, if listeners’ ability to generate phonological predictions could indeed be flexibly adjusted based on their usefulness for a task.
2. Experiment 1a
Experiment 1a sought to determine whether the phonological information of a highly predictable word can be pre-activated during on-line speech comprehension. The task of the participants was to indicate whether the spoken sentence they just heard contained any of the words in the visual array.
2.1. Methods
2.1.1. Participants
Fifty participants were recruited for this experiment. This decision was made by taking into consideration two things. One is the results of a power analysis by Li et al. (Reference Li, Li and Qu2022), which reported that at least 30 participants are needed to achieve a statistical power of over 0.9 based on the effect size in Ito (Reference Ito2019, Experiment 2), which has a similar design to ours. The second is the typical number of participants (40–50) recruited in recent studies, which examined phonological prediction with VWP (Ito, Reference Ito2019; Ito & Sakai, Reference Ito and Sakai2021; Li et al., Reference Li, Li and Qu2022; Shen et al., Reference Shen, Hyönä, Wang, Hou and Zhao2021).
In Experiment 1a, all 50 (18 males and 32 females; mean age = 24, SD = 2.22) native Mandarin speakers were born and grew up in Northern China and speak Standard Chinese. They participated in this experiment with financial compensation. They all were right-handed and reported normal or corrected-to-normal vision and no language or hearing disorders.
2.1.2. Stimuli
The auditory stimuli consisted of 40 Mandarin sentences (mean length = 20 characters, SD = 2.11, range = 16–26), with each containing a highly predictable target word at the sentence-final position (e.g. 胶, jiao1, ‘glue’; 为了把票据粘在一起,他们往往会使用那种胶. ‘To paste those tickets together, they always use that kind of glue’.). The target word was always a single-character noun preceded by an adjective and then a transitive verb in a subject–verb–object sentence construction.
All sentences were produced by a female native Beijing Mandarin speaker at a sampling rate of 22,050 Hz in a sound-proof room. The mean intensity of these sentences was adjusted to be 70 Db, and the mean duration was 4,756 ms (within a range of 4,550–7,557 ms). The duration from the onset of the transitive verb to that of the target word in the experimental sentences was on average 1,015 ms (within a range of 810–1,366 ms), and the duration of the target word was on average 440 ms (within a range of 299–608 ms).
During the eye-tracking experiment, each spoken experimental sentence was paired with one of the four types of visual arrays, with each array consisting of a single-character critical word and a single-character distractor word. The four types of visual arrays were based on four types of critical words: the target word, a homophone competitor, a tonal competitor, and an unrelated word. In the target word condition, the critical word corresponded to a single-character target word (e.g. 胶, jiao1, glue). In the homophone competitor condition, the critical word overlapped with the target word phonologically, but not orthographically or semantically; the mapping was at both the segmental and super-segmental lexical tone level (e.g. 椒, jiao1, pepper). In the tonal competitor condition, the critical word overlapped with the target word only in their lexical tones. They had different segmental syllables, as well as different orthography and semantics (e.g. 粥, zhou1, porridge). Both unrelated words (e.g. 缎, duan4, satin) and distractor words (e.g. 梅, mei2, plum) were distinct from the target word in phonology, orthography, and semantics (see Table 1).
Table 1. Visual arrays of an example sentence

Note: Each participant saw one of the visual arrays while listening to this target sentence in the experiment. The critical and distractor words are labelled in different colours here as example stimuli, but they were all presented in black in the experiment.
In total, the above manipulations resulted in four experimental conditions (critical-word type): target word, homophone competitor, tonal competitor, and unrelated word.
Written pre-tests for stimulus verification
Two cloze probability tests were conducted to assess the predictability of target words, by presenting written versions of sentences that were truncated before the transitive verbs (nounClozebefore-verbs, involving 16 participants) and before the target nouns (nounClozebefore-nouns, involving a different group of 16 participants). Participants were asked to complete each sentence using the first word that came to their mind at those truncated points. The results showed that the mean cloze probability of the target word was 55% for the first test (i.e. nounClozebefore-verbs, with a range of 0–100%) and 88% for the second test (i.e. nounClozebefore-nouns, with a range of 75–100%). These results demonstrate that the target words in the stimulus sentences are indeed highly predictable and that the transitive verbs play an important role in biasing towards the targets during this prediction process.
We further evaluated the semantic relationship between the critical or distractor words and the stimulus sentences via two analyses. One is latent semantic analysis (LSA), which can calculate the frequency of co-occurrence between a word and its context according to an on-line corpus (URL: http://www.lsa.url.tw/modules/lsa). The other is subjective ratings (of the semantic congruency of the critical or distractor words with the stimulus sentences) by another 16 participants. Table 2 provides the results. The ANOVA results showed that the value of target words was significantly higher than the other four types of written words (i.e. homophone competitor, tonal competitor, unrelated word, and distractor word) (ps < 0.001), but there was no significant difference between any two of these four conditions. These results confirm that, except for the target words, the other four types of printed words were equally semantically unrelated to the sentences.
Table 2. Characteristics of the critical words and distractor words in Experiment 1 (mean (SD))

We also examined the semantic relatedness between the target words and the other types of words in the visual display (i.e. homophone or tonal competitors, unrelated words, and distractors). Another 16 participants rated the semantic relatedness of each word pair on a 7-point scale (from 1 to 7), with higher scores indicating a higher level of relatedness. The rating scores are also listed in Table 2, which shows that all word pairs showed very low scores, confirming low semantic relatedness between the target words and the other printed words. In addition, other possible confounding factors, such as the words’ number of strokes and lexical frequency, were controlled to have no significant differences across the different types of conditions (see Table 2).
In total, there were 40 sets of experimental stimuli, with each set containing an experimental spoken sentence and four types of visual arrays. These experimental materials were grouped into four lists based on the Latin square design and the four experimental conditions, with each list containing 10 sets of stimuli per condition and each sentence appearing only in one condition. In addition to the experimental stimuli, each list contained 40 filler stimuli. Some of the target words in these filler materials appeared in the middle position of the sentences to prevent participants from predicting the position of the target words. We also created two versions of the visual display that counterbalance the left and right displays of the critical and distractor words on the screen, resulting in a total of eight versions of stimuli.
2.1.3. Procedure
In the eye-tracking experiment, participants’ right eye-movements were recorded, using an EyeLink 1000 plus Desktop-mount eye-tracker at a sampling rate of 1,000 Hz. The stimuli were presented on a computer monitor with a screen resolution of 1,024-pixel-by-768-pixel, and participants sat 70 cm away from the screen with their eyes calibrated using a nine-point grid. The validation error for the calibration was smaller than 1° of the visual angle.
Each trial of this experiment started with a drift check, during which participants fixated on the centre of the screen, followed by a 500-ms fixation ‘+’ at the centre of the screen. The spoken sentence was then presented, and the visual array was presented 2,000 ms before the target word in the spoken sentence and remained on the screen for 4,000 ms. The critical and distractor words were displayed in black with the Song font at a size of 36 on a white background, with a visual angle of 1.28 degrees. After the visual array disappeared, a blank screen was displayed for 1,000 ms, followed by a question asking whether the sentence contained any of the displayed words. Participants answered by pressing ‘F’ on the keyboard for ‘Yes’ (if the spoken sentence contained any of the printed words) and ‘J’ for ‘No’ (if the spoken sentence did not contain any of the printed words). There were equal numbers of ‘Yes’ and ‘No’ responses. Once the answer was made, the next trial began immediately.
2.1.4. Eye-tracking data analysis
In each trial of the experiment, the visual array was divided into two areas of interest: a 100 × 100-pixel area surrounding the critical word and a 100 × 100-pixel area surrounding the distractor word. The log-ratio of fixation proportions to the critical word versus distractor word was calculated to quantify a fixation bias towards the critical word relative to its co-present distractor word (Ito & Knoeferle, Reference Ito and Knoeferle2022). To obtain the log-ratio of critical word versus distractor word, we followed two steps: (1) the fixation proportions for the critical word and its co-present distractor word were calculated using the formula: number of fixations on critical or distractor word / (number of fixations on critical word + number of fixations on distractor word) (our participants mostly, around 98% of total fixations, fixated on only one or neither of the two co-present words, which indicates that the fixation proportion calculated using the number of fixations should be highly correlated with that calculated using the fixation duration); (2) the log-ratio was then computed using the formula: log ((fixation proportion of critical word +0.5) / (fixation proportion of distractor word +0.5)) (Barr, Reference Barr2008). A positive log-ratio value indicates more fixations on the critical word than its co-present distractor word, and a value of zero indicates no fixation bias.
If the target word or phonological information was pre-activated, the log-ratio for the corresponding critical word (i.e. target word, homophone competitor, or tonal competitor) would be a positive value and significantly higher than the unrelated baseline condition before the target words were heard during the oral experimental sentences.
Because we were interested in the phonological prediction effect and the timecourse of this prediction effect, the log-ratio of critical versus distractor word was calculated separately for each 100-ms time bin during the timecourse of −1,300 ms before the target word onset in the spoken sentence to 1,000 ms after it. The −1,300 ms pre-target onset was defined because the predictability of the critical noun increased significantly after the presence of the transitive verb in the spoken sentence (as suggested by the pretest), and the maximum duration from the onset of the transitive verb to the spoken target noun was around 1,300 ms.
Generalized additive mixed modelling analysis over each 100-ms time bin
Generalized additive mixed modelling (GAMM; Wood, Reference Wood2006) was employed to tap into the timecourse of phonological prediction. GAMM is a regression analysis that can account for the inherent variability between subjects and items using factor smooths. Importantly, GAMM can also guard against false-positive errors and control temporal autocorrelation in eye-movement data by including a temporal autocorrelation parameter in the model (Porretta et al., Reference Porretta, Kyröläinen, van Rij and Järvikivi2018), making it possible to detect the statistical significance of an effect and estimate the time bins in which an effect was significant.
In this study, GAMM was performed using the mgcv (Wood, Reference Wood2019) package in R (R Core Team, 2014). We tested whether and when the log-ratio value (log-ratio of critical word versus distractor) was significantly different between each critical condition of interest and the unrelated baseline condition (i.e. target word versus unrelated, homophone competitor versus unrelated, and tonal competitor versus unrelated). The log-ratio values (in each 100-ms time bin from −1,300 ms before the target word to 1,000 ms after it) were used as the dependent variable and the critical-word type as the predictor. The autocorrelation parameter was determined from the GAMM_base model. We then ran another model (GAMM_main) including the autocorrelation parameters from the GAMM_base model and a logical vector, indicating the starting time-point for each trial to visualize the emergence of the effects of the critical conditions using smooths.
In addition, we were interested in whether the log-ratio of each condition was significantly larger than zero before the onset of the spoken target word, which would indicate the effect of phonological prediction before the target word is heard. We constructed the GAMM_main_zero model with log-ratio values (dependent variable) zoomed into the interval from −1,300 ms to the onset of the target word, with the autocorrelation parameters determined from the GAMM_base_zero model.
GAMM_base = Fixation proportion (log-ratio) ~ Critical-wordType + s(time, by = Critical-wordType) + s(time, sub, by = Critical-wordType, bs = ‘fs’, m = 1) + s(time, item, by = Critical-wordType, bs = ‘fs’, m = 1).
GAMM_main = Fixation proportion (log-ratio) ~ Critical-wordType + s(time, by = Critical-wordType) + s(time, sub, by = Critical-wordType, bs = ‘fs’,m = 1) + s(time, item, by = Critical-wordType, bs = ‘fs’, m = 1), rho = AR1.val, AR.start = Is_start.
GAMM_base_zero = Fixation proportion (log-ratio) ~ s(time, by = Critical-wordType) + s(time, sub, by = Critical-wordType, bs = ‘fs’,m = 1) + s(time, item, by = Critical-wordType, bs = ‘fs’, m = 1).
GAMM_main_zero = Fixation proportion (log-ratio) ~ s(time, by = Critical-wordType) + s(time, sub, by = Critical-wordType, bs = ‘fs’,m = 1) + s(time, item, by = Critical-wordType, bs = ‘fs’, m = 1), rho = AR1.val, AR.start = Is_start, subset = Critical-wordType == ‘Target / Homophone / Tonal / Unrelated’.
2.2. Results
2.2.1. Behavioural judgement result
The mean accuracy of the behavioural judgement was very high (mean = 97%, SD = 4%), confirming that the participants were paying attention to the stimulus sentences.
2.2.2. Eye-tracking result of generalized additive mixed modelling
In the GAMM_main_zero model, the results showed that during the time interval of −1,300 ms to 0 ms (i.e. the onset of the target word), when the critical word was the target, the log-ratio was significantly larger than zero (b = 0.06, SE = 0.01, t = 5.20, p < 0.001), indicating a bias towards fixating on it over the co-present distractor word. However, this was not the case for the homophone competitor (b = 0.002, SE = 0.01, t = 0.25, p = 0.80), tonal competitor (b = −0.01, SE = 0.01, t = −0.64, p = 0.52), or the unrelated word (b = −0.01, SE = 0.01, t = −0.91, p = 0.36) where there was no significant difference in fixation between the critical word and its respective co-present distractor word.
The GAMM_main model confirmed a significant effect of critical-word type on the fixation log-ratio over time (F = 41.07, p < 0.001). In particular, compared to the unrelated words (b = −0.01, SE = 0.01), the log-ratio was significantly higher for target words (b = 0.10, SE = 0.01, t = 9.59, p < 0.001), within a window latency from −1,300 ms to −978 ms before the onset of the target word and a window latency from −756 ms to 1,000 ms after it. This shows clearly that participants had a bias towards fixating on the target words not only after, but also before the target word appeared. The log-ratio for homophone competitors was also significantly higher than that of unrelated words (b = 0.04, SE = 0.01), which was, however, observed only from 222 ms to 1,000 ms after the target word was pronounced (t = 5.44, p < 0.001). This indicates that participants only activated the phonological information of the target word after hearing it. The log-ratio for tonal competitors (b = −0.001, SE = 0.01) did not differ from that of unrelated words (t = −0.10, p = 0.92), suggesting that there was no fixation bias towards tonal competitors. These findings are illustrated in Figs. 1 and 2.

Figure 1. Non-linear smooths for the unrelated word (red), target word (green), homophone competitor (blue), and tonal competitor (purple) from −1,300 ms before the spoken target word to 1,000 ms after it in Experiment 1a. The shaded area shows 95% confidence intervals. The raw fixation proportions within the window latency from −1,600 ms to 1,000 ms can be found in the shared dataset links, which are the same for Experiment 1b and Experiment 2.
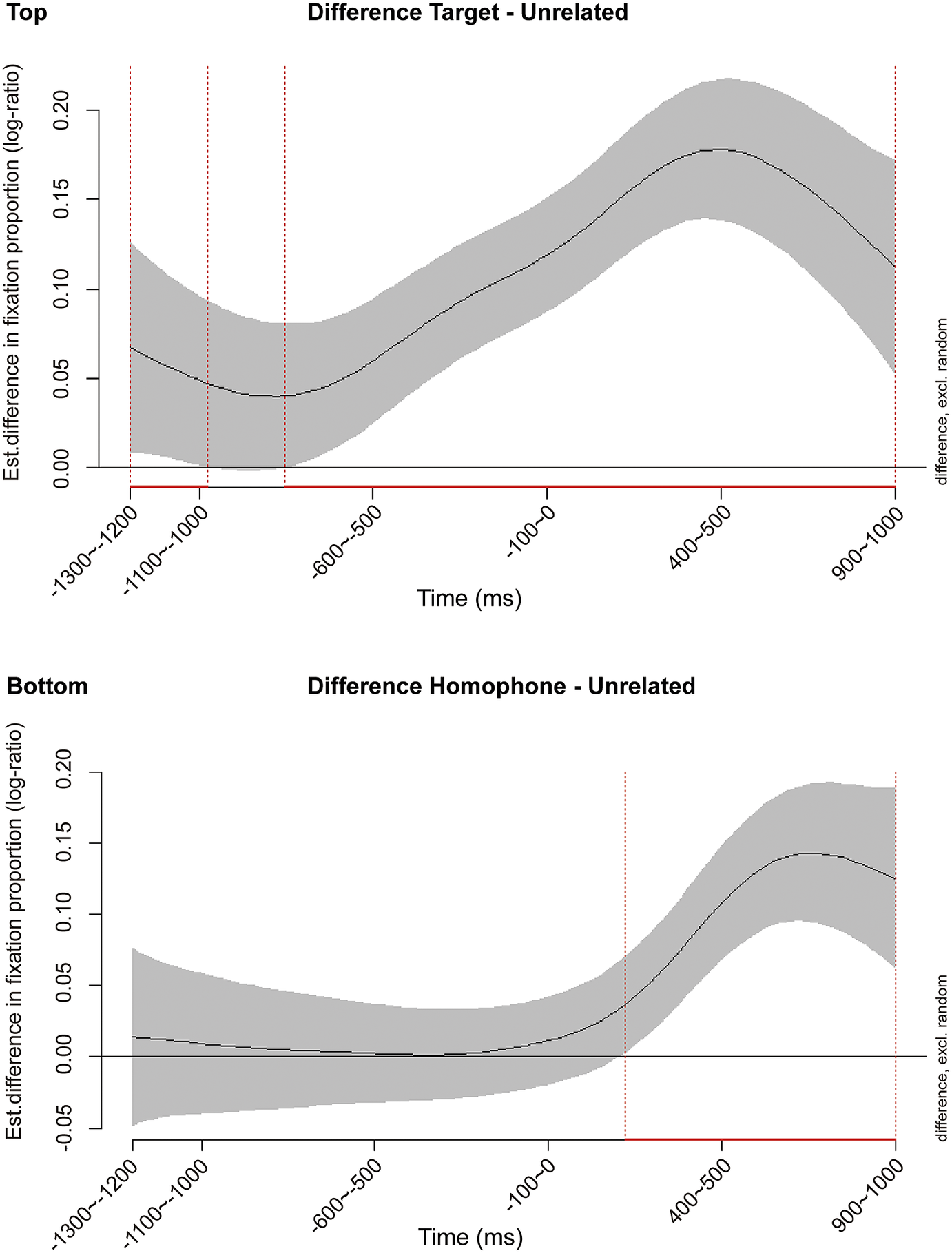
Figure 2. Log-ratio difference plot for target versus unrelated (top) and homophone versus unrelated (bottom) from −1,300 ms before the spoken target word to 1,000 ms after it in Experiment 1a. The shaded area shows 95% confidence intervals. The red lines at the bottom indicate time bins in which the difference between conditions was significant.
2.3. Discussion
In Experiment 1a, we found that participants showed a fixation bias towards target words over their co-present distractors and were also more likely to fixate on target words than unrelated words well before the spoken target words were heard (i.e. starting from −1,300 ms to −978 ms and from −756 ms to 0 ms). This suggests that participants were anticipating the target words during the on-line processing of spoken language, which is consistent with previous research (Ito & Sakai, Reference Ito and Sakai2021; Shen et al., Reference Shen, Hyönä, Wang, Hou and Zhao2021). However, the GAMM analysis did not show a preference for homophone or tonal competitors in the window period, leading up to the onset of the spoken target words. This may be because the task biased the participants to make a judgement based on the orthographic information presented in the visual array, hence implicitly encouraging participants to ignore the homophonous information. Experiment 1b was therefore conducted with a new task that required participants to pay more attention to the phonological features of the target words.
3. Experiment 1b
The goal of Experiment 1b was to investigate whether explicitly requiring participants to pay attention to the sound properties of the speech signal during on-line speech comprehension would facilitate the initiation of anticipatory processing at the level of phonological representation, and if so, what is the timecourse of this phonological prediction.
3.1. Methods
3.1.1. Participants
A new group of 49 native Mandarin speakers (16 males and 33 females; mean age = 24, SD = 2.38) participated in the experiment in exchange for financial compensation. These participants were recruited with the same criteria as Experiment 1a.
3.1.2. Stimuli and procedure
The stimuli for Experiment 1b were identical to those used in Experiment 1a, but the procedure was different. In particular, participants were asked to judge whether words shown on the screen overlapped phonologically with any of the words in the oral sentence they had just heard, by pressing ‘F’ for ‘Yes’ and ‘J’ for ‘No’.
3.1.3. Eye-tracking data analysis
Data were analysed in the same way as described in Experiment 1a.
3.2. Results
3.2.1. Behavioural judgement result
The mean accuracy of the behavioural response was very high (mean = 96%, SD = 7%).
3.2.2. Eye-tracking result of generalized additive mixed modelling
The GAMM_main_zero model showed that, before the presence of spoken target words, the log-ratio intercept of the target words (b = 0.09, SE = 0.01, t = 7.69, p < 0.001) and that of the homophone competitors (b = 0.03, SE = 0.01, t = 3.08, p < 0.005) were significantly larger than zero, suggesting fixation bias towards these two types of words over their respective co-present distractor words. However, this was not the case for either the tonal competitors (b = −0.01, SE = 0.01, t = −0.72, p = 0.47) or the unrelated words (b = −0.01, SE = 0.01, t = −1.36, p = 0.17).
The GAMM_main model showed that the log-ratio of the target words versus distractor words (b = 0.13, SE = 0.01) was significantly higher than that of unrelated words (b = −0.01, SE = 0.01) (t = 10.43, p < 0.001) within the whole window latency of interest (from −1,300 ms before the spoken target word to 1,000 ms after it). Importantly, the log-ratio of homophone competitors (b = 0.11, SE = 0.01) was also significantly higher than that of unrelated words (t = 10.24, p < 0.001) from −978 ms to −844 ms before the target word in spoken sentences and from −156 ms before the target word to 1,000 ms after it. This indicates that participants were anticipating the phonological information of the target words shortly after hearing the transitive verbs, given that these verbs preceded the target words in our spoken sentences by an average of 1,015 ms. Moreover, the pre-activated phonological information was re-activated again when the spoken target word was going to appear in the continuous speech input (around −156 ms before the target word onset). In contrast, the log-ratio for tonal competitors (b = 0.003, SE = 0.01) did not differ from that of unrelated words (t = 0.28, p = 0.78), suggesting that there was no fixation bias towards tonal competitors. These results are illustrated in Figs. 3 and 4.

Figure 3. Non-linear smooths for the unrelated word (red), target word (green), homophone competitor (blue), and tonal competitor (purple) from −1,300 ms before the spoken target word to 1,000 ms after it in Experiment 1b. The shaded area shows 95% confidence intervals.

Figure 4. Log-ratio difference plot for target versus unrelated (top) and homophone versus unrelated (bottom) from −1,300 ms before the spoken target word to 1,000 ms after it in Experiment 1b. The shaded area shows 95% confidence intervals. The red lines at the bottom indicate time bins in which the difference between the conditions was significant.
3.3. Discussion
Experiment 1b replicated the lexical anticipation effects observed in Experiment 1a, as indicated by the fact that participants demonstrated a preference for fixating on the target words before the acoustic onset of these target words. Furthermore, the results of Experiment 1b also revealed a fixation preference on the homophone competitors (compared with both their co-present distractor words and unrelated words) before the target words were produced in the sentences, which was not observed in Experiment 1a. This finding lends evidence for the pre-activation of phonological information during real-time language comprehension. The crucial difference between the two experiments is likely due to their different task requirements. In Experiment 1b, the requirement for judging words of phonological overlap probably drove participants to focus more on the phonological information. It is, however, important to note that the participants recruited in Experiments 1a and 1b were different. So, we could not rule out the possibility that some potential confounding factors such as individual differences (e.g. cognitive abilities or prior knowledge) between the two participant groups might have led to the different pattern of results. Further research is therefore needed to verify and clarify the findings in Experiments 1a and 1b.
4. Experiment 2
Experiment 2 was designed to further investigate the flexibility of phonological pre-activation while controlling for participant individual differences. We recruited a new group of participants and required them to complete both the ‘word judgement’ task (in one block) and the ‘pronunciation judgement’ task (in another block). Given that there was no fixation preference towards the tonal competitors in both Experiments 1a and 1b, we adapted the design and kept only the homophone competitors, unrelated words, and distractor words in Experiment 2.
4.1. Methods
4.1.1. Participants
In this experiment, a new group of 56 native Mandarin Chinese speakers (mean age = 23, SD = 3.07; 19 males and 37 females) participated and received financial compensation. These participants were recruited using the same criteria as Experiment 1.
4.1.2. Stimuli
Ninety-two experimental sentences (with 40 experimental sentences from Experiment 1) were included with the same criteria as Experiment 1. The sentences were also produced by the same female native Beijing Mandarin speaker (as in Experiment 1). For the new stimulus set, the duration from the acoustic onset of the transitive verb to that of the target word was on average 883 ms (within a range of 602–1,320 ms) and the duration of the target word was on average 410 ms (within a range of 241–1,169 ms).
In this experiment, we only kept the homophone competitor and unrelated word conditions, resulting in two types of visual arrays: homophone competitor + distractor word and unrelated word + distractor word. This design resulted in a full factorial design with all combinations of the factors such as critical-word type (homophone competitor versus unrelated word) and task (word judgement versus pronunciation judgement), leading to four experimental conditions.
Two cloze probability tests were conducted to assess the predictability of target words in these sentences as in Experiment 1. The results showed that the mean cloze probability of the target word was 44% for the first test (i.e. nounClozebefore-verbs with a range of 0–100%) and 87% for the second test (i.e. nounClozebefore-nouns with a range of 75–100%). These results confirmed that the target words in the stimulus sentences were indeed highly predictable and that the transitive verbs played an important role in biasing the prediction.
A series of pre-tests were also conducted for the critical and distractor words as in Experiment 1 (see Table 3). The ANOVA results showed that there were no significant differences in the LSA analyses, the semantic relatedness of these words with the contextual sentences, the semantic relatedness of these words with the target words, their number of strokes, and their word frequency.
Table 3. Characteristics of the critical words and distractor words in Experiment 2 (mean (SD))

The ninety-two sets of experimental materials were grouped into four versions of the stimulus list using a Latin square design based on four experimental conditions. Each version of the stimulus list contained an equal number of experimental stimuli (23 sentence-visual array pairs) for each condition, with each experimental sentence only appearing in one condition. For each sentence-visual array pair, the presentation of the critical and distractor words on the screen was also counterbalanced (as on the left versus on the right side of the screen). In addition, for the two critical words differing from the target word in phonology, orthography, and semantics in each set of printed words, the assignment of their corresponding unrelated words or distractor words was also counterbalanced. This resulted in a total of 16 versions of the stimulus list. Each participant completed only one version, with the stimuli presented in two separate blocks: one for the ‘word judgement’ task and the other for the ‘pronunciation judgement’ task. Additionally, 46 filler stimuli were included in each block.
4.1.3. Procedure
The experimental procedure was identical to Experiment 1 except that, in this experiment, each participant took part in two tasks in two separate blocks. The order of the two blocks or tasks was counterbalanced among participants.
4.1.4. Eye-tracking data analysis
The eye-tracking data were analysed in the same way as in Experiment 1, except that we additionally analysed the interaction of critical-word type (homophone competitor versus unrelated word) and task (word judgement versus pronunciation judgement) through the GAMM analysis (Wieling, Reference Wieling2018). To analyse this interaction, we first constructed two new binary factors in GAMM. One factor (IsHomo) was set to 1 for the homophone competitor and to 0 for an unrelated competitor; the other factor (IsWordHomo) was set to 1 for the homophone competitor in the word judgement task and to 0 otherwise. Then, we created a binary smooth model (GAMM_interaction_base/main), in which s(time, by = IsWordHomo) represents the difference in ‘homophone-unrelated competitor difference’ across the word judgement and pronunciation judgement tasks, namely the interaction between task and critical-word type. The significant effect of this interaction analysis would then allow us to perform separate analyses of the two tasks as in Experiment 1.
GAMM_interaction_base = Fixation proportion (log-ratio) ~ Task + s(time, by = Task) + s(time, by = IsHomo) + s(time, by = IsWordHomo) + s(time,sub,by = Critical-wordType, bs = ‘fs’,m = 1) + s(time, item, by = Critical-wordType, bs = ‘fs’, m = 1) + s(time, sub, by = Task, bs = ‘fs’, m = 1).
GAMM_interaction_main = Fixation proportion (log-ratio) ~ Task + s(time, by = Task) + s(time, by = IsHomo) + s(time, by = IsWordHomo) + s(time,sub,by = Critical-wordType, bs = ‘fs’,m = 1) + s(time, item, by = Critical-wordType, bs = ‘fs’, m = 1) + s(time, sub, by = Task, bs = ‘fs’, m = 1), rho = AR1.val, AR.start = Is_start.
4.2. Results
4.2.1. Behavioural judgement result
The mean accuracy of the ‘word judgement’ was 97% (SD = 4%) and that of the ‘pronunciation judgement’ was 93% (SD = 6%).
4.2.2. Eye-tracking result of generalized additive mixed modelling
The GAMM_interaction_main model revealed that the difference between homophone competitor and unrelated competitor was significantly different across the two tasks (F = 45.21, p < 0.001), which motivated separate GAMM analysis for each task.
The GAMM_main_zero model showed that in the pronunciation judgement task, before the presence of the spoken target word, the log-ratio was significantly larger than zero in the homophone competitor condition (b = 0.03, SE = 0.01, t = 4.33, p < 0.001) but not in the unrelated word condition (b = 0.01, SE = 0.01, t = 0.85, p = 0.40). In contrast, in the word judgement task, the log-ratio was not significantly different from zero for both the homophone competitor condition (b = 0.004, SE = 0.01, t = 0.92, p = 0.36) and the unrelated word condition (b = 0.001, SE = 0.01, t = 0.10, p = 0.92). These results suggested a fixation bias towards the homophone competitors over their co-present distractors before the spoken target word but only in the pronunciation judgement task.
The GAMM_main model showed that in the ‘pronunciation judgement’ task, the log-ratio of homophone competitors (pronunciation judgement: b = 0.09, SE = 0.01, t = 13.67, p < 0.001) was significantly higher than unrelated words (b = −0.0003, SE = 0.003) in the time interval of −1,111 ms to −689 ms before the spoken target word and from −67 ms before this target word to 1,000 ms after it. This confirmed that participants tended to fixate more on the homophone competitors before they heard the words. In contrast, in the ‘word judgement’ task, the log-ratio of homophone competitors (b = 0.03, SE = 0.01, t = 6.21, p < 0.001) was significantly higher than unrelated word (b = 0.002, SE = 0.003) from 356 ms to 1,000 ms after the target word in the spoken sentences, but not before the target word. The results are illustrated in Figs. 5 and 6.

Figure 5. Non-linear smooths for the unrelated word (red) and homophone competitor (blue) from −1,300 ms before the spoken target word to 1,000 ms after it in the ‘word judgement’ (top) and ‘pronunciation judgement’ (bottom) tasks of Experiment 2. The shaded area shows 95% confidence intervals.

Figure 6. Log-ratio difference plot for homophone versus unrelated of model GAMM_main from −1,300 ms before the spoken target word to 1,000 ms after it in the ‘word judgement’ (top) and ‘pronunciation judgement’ (bottom) tasks of Experiment 2. The shaded area shows 95% confidence intervals. The red lines at the bottom indicate time bins in which the difference between the conditions was significant.
4.3. Discussion
In Experiment 2, we investigated the flexibility of the phonological level of prediction by asking the same group of participants to complete both the ‘word judgement’ and the ‘pronunciation judgement’ tasks. The fixation patterns before the acoustic onset of the target word were found to be different across the two tasks. The results of the GAMM analysis revealed a preference for fixating on the homophone competitors before the target word was pronounced. This homophone bias indicates that the human brain is able to pre-activate the phonological form of a highly predictable word during on-line speech comprehension. However, this pre-activation of phonological information was observed only in the ‘pronunciation judgement’ task and not in the ‘word judgement’ task. Therefore, the difference in Experiments 1a and 1b was replicated in Experiment 2, lending evidence that the phonological prediction effect in our study was task-dependent.
5. General discussion
The current study used the printed-word version of the visual world paradigm to investigate the use and flexibility of phonological prediction in real-time speech comprehension. The results of Experiment 1 confirmed the anticipation of target words in a highly predictable context. Results of Experiments 1 and 2 corroborated the fixation bias of homophone competitors before the spoken target word appeared in the speech input, when listeners performed a task with an enhanced awareness of the phonological information. Conjointly, our results provided novel evidence for the task-modulated phonological level of prediction. The timecourse and flexibility of the phonological prediction are discussed as follows.
5.1. The timecourse of phonological prediction
The phonological prediction effect found in the present study is consistent with previous research, such as the pre-activation of phonological form reported in ERP studies (e.g. DeLong et al., Reference DeLong, Urbach and Kutas2005) and eye-tracking studies (e.g. Ito et al., Reference Ito, Pickering and Corley2018; Shen et al., Reference Shen, Hyönä, Wang, Hou and Zhao2021). It is noteworthy that both this study and previous eye-tracking studies indicate that the phonological form pre-activation effect detected in the VWP (as seen in the homophone condition) is short-lived (e.g. 150 ms in Ito et al., Reference Ito, Pickering and Corley2018, 100 ms in Shen et al., Reference Shen, Hyönä, Wang, Hou and Zhao2021, and around 134 ms in Experiment 1b of the current study).
Importantly, the results of the current study also provided new insights into our understanding of the timecourse of phonological predicting, showing that although the phonological pre-activation effect detected in the VWP was of short duration, this predicted phonological information waned but was then re-activated later when the target word was going to appear in the speech signal. In particular, in both Experiment 1b and Experiment 2, a preference for fixating on the homophone competitors (compared with the unrelated words) began to appear around −1,000 ms (from −978 ms to −844 ms in Experiment 1b and from −1,111 ms to −689 ms in Experiment 2) before the spoken target words, indicating that listeners had already pre-activated the phonological information of a highly predictable word by this point. Interestingly, within a window latency around the acoustic onset of the target words and after the actual appearance of these words (from −156 ms before the target word to 1,000 ms after it in Experiment 1, from −67 ms before the target word to 1,000 ms after it in Experiment 2), this homophone competitor fixation preference was observed again in the ‘pronunciation judgement’ task. Taking into consideration the fact that planning and executing an eye-movement need around 200 ms (Malins & Joanisse, Reference Malins and Joanisse2010), it seems that the homophone competitor fixation preference from −156/−67 ms before the spoken target word to 1,000 ms afterwards (in the ‘pronunciation judgement’ task) might be introduced by the combined effects of phonological form pre-activation and phonological form priming by the pre-activated target words when they were about to arrive immediately in the incoming speech stream. The visualization of each participant’s fixations for the homophone competitor in the ‘pronunciation judgement’ task (Experiment 1b and Experiment 2) also suggested that at least some of the participants (e.g. 18 of 49 participants ‘s12, s13, s14, s18, s2, s24, s29, s32, s35, s36, s37, s38, s39, s40, s46, s47, s48, s49’ in Experiment 1b) showed re-activation of phonological information around the target word onset (see Supplementary Material). This is different from earlier studies, which showed only brief pre-activation of phonological information and did not report re-activation of phonological information (Ito et al., Reference Ito, Pickering and Corley2018; Shen et al., Reference Shen, Hyönä, Wang, Hou and Zhao2021). The reason for this discrepancy may be related to the types of phonological competitors and the specific patterns of visual arrays used in these studies. In our study, the homophone competitor shared the segmental and tonal features of the whole target words, and it was presented simultaneously with another distractor word in the visual array of two words. In Ito et al. (Reference Ito, Pickering and Corley2018) and Shen et al. (Reference Shen, Hyönä, Wang, Hou and Zhao2021), however, only part of the target words’ phonological form is shared, and the phonological competitors were presented simultaneously in the visual array of four words or pictures, which might have in some ways mitigated the sensitivity of the participants to phonological re-activation. In short, despite some differences from previous studies, the results of our study confirmed the pre-activation of phonological information in real-time speech processing and provided novel evidence that such phonological prediction can be re-evoked later around the acoustic onset of the target word.
The phonological reemergence pattern observed in this study is in line with an earlier magnetoencephalography (MEG) study (Gwilliams et al., Reference Gwilliams, Linzen, Poeppel and Marantz2018), which reported that the brain actively maintains phonemic detail in the auditory cortex throughout the duration of a spoken word and quickly re-activates it at subsequent phoneme positions. The current study lends support to this finding from the visual world paradigm that, during on-line spoken sentence processing, the phonological form representation of a spoken word can be pre-activated and actively maintained in working memory over a long period of time (e.g. around 800 ms) to aid speech processing as subsequent words are received.
5.2. The flexibility and representational nature of phonological prediction
This study is the first to show that the level of phonological form prediction can be flexibly adjusted during on-line speech processing. The strongest evidence for this flexibility is found in Experiment 2, which showed that when both the sentence context and the participants’ cognitive abilities and long-term knowledge were the same, the preference for fixating on homophone competitors (driven by the highly predictive context) was observed only in the ‘pronunciation judgement’ task, in which the phonological pre-activation of upcoming words was beneficial. We know that top-down predictive processing may incur some basic metabolic costs (e.g. increased neural firing; Kuperberg & Jaeger, Reference Kuperberg and Jaeger2016). These costs may outweigh the benefits of phonological pre-activation in certain situations, as the benefit of pre-activation is usually confined to a specific word in the continuous speech signal. Therefore, it is up to the listeners to adjust phonological form prediction according to its utility to their task at hand.
The flexibility of phonological prediction observed in the present study echoes the existing findings on strategic lexical prediction, which have been reported to be affected by the preceding context (Brothers et al., Reference Brothers, Swaab and Traxler2017) and the credibility of the speaker (Brothers et al., Reference Brothers, Dave, Hoversten, Traxler and Swaab2019). Our results extend the flexibility of anticipatory processing to the phonological level of prediction induced by processing tasks.
The flexibility of phonological prediction observed in the present study provides further support to the ‘smart’ generating mechanism (System 2) of language prediction (e.g. Huettig, Reference Huettig2015), by showing that the human brain not only strategically generates phonological predictions based on their utility to the task at hand but also actively maintains the pre-activated phonological information and quickly re-activates it when needed. This pattern of phonological prediction suggests that, during on-line speech processing, there may be a top-down control mechanism that enables listeners’ brain to actively generate and transfer phonological form prediction from higher to lower levels of representation.
It should be noted that while this study found a phonological form prediction effect for the homophone condition, there was no predictive effect for the tonal competitors, as seen in Experiment 1b. This suggests that lexical tonal representation is not pre-activated separately from the segmental syllable. Note that an earlier eye-tracking study (Shen et al., Reference Shen, Hyönä, Wang, Hou and Zhao2021) reasoned that lexical tone information can be pre-activated in a highly predictive sentence context, as the phonological competitor effect was observed in the tone-consistent condition and not in the tone-inconsistent condition. In that study, the printed critical word in the tone-consistent condition shared both segmental information and tonal information with the spoken target word, while in the tone-inconsistent condition it only shared segmental information. This suggests that when the preceding context is highly constraining, lexical tone can be pre-activated together with the tone-carrying segmental syllable. One way to reconcile the conclusions of these two studies is that lexical tone cannot be pre-activated alone without the presence of segmental information, even in a highly predictive sentence context. This finding is in line with the lack of a tonal-independent effect in spoken word recognition (e.g. Yang & Chen, Reference Yang and Chen2022). This lack of a separate tonal pre-activation effect in our study may be explained by the fact that there are only a limited number of lexical tones in Mandarin Chinese, and lexical tone needs to work in conjunction with segmental information to effectively distinguish lexical-semantic meanings.
The present study has its limitations. It only demonstrated the presence or absence of phonological pre-activation when listeners were explicitly required to complete a ‘pronunciation or word judgement’ task. Moreover, a printed-word version of VWP was used in the present study, which may show more sensitivity to phonological manipulations than the picture version of VWP (Huettig & McQueen, Reference Huettig and McQueen2007). This raises the question of whether our findings can generalize to situations in which processors do not engage with printed words. Further research using more implicit phonological processing requirements and other research paradigms is needed to fully understand the flexibility of phonological prediction and the representational nature of the predicted forms.
Supplementary Materials
The supplementary material for this article can be found at http://doi.org/10.1017/langcog.2023.38.
Data availability statement
The dataset of the current eye-tracking experiments is available for public download at https://osf.io/bcjzy/?view_only=None.
Acknowledgements
This work was supported by grants from the National Natural Science Foundation of China (32171057) and the Netherlands Organization for Scientific Research (VI.C.181.040).
Competing interest
The authors declare none.