Production of natural gas from shale formations has grown considerably in the past decade, increasing from 6.4 percent of total gas produced in the United States in 2007 to 49.2 percent in 2014 (EIA 2015b, 2016). Among the shale gas plays in the United States, the Marcellus play is the largest active play with the potential to be a large gas supplier in the future. The Marcellus play, located in the Appalachian Basin, accounts for 42.3 percent of 2014 proved shale reserves (EIA 2015a).
The rapid growth in shale gas development could have the most pronounced effects in rural and more remote local communities. Within the Marcellus region, shale gas drilling and production primarily occurs in rural counties, and in the case of northeastern Pennsylvania, counties without traditional gas and oil extraction. As of June 2011, over half of all permitted well pads in Pennsylvania were on agricultural land (Drohan et al. Reference Drohan, Brittingham, Bishop and Yoder2012). This finding, combined with the location of shale gas development, raises the questions of whether or not, and to what extent, shale gas development has affected agriculture. A better understanding of the impacts of this activity on local agriculture and land use is an important concern for policy makers.
Shale gas extraction could affect agriculture in multiple ways throughout the lifetime of a well. Preparing a site includes activities such as creating access roads, clearing the area for the well pad and equipment, and creating pipeline corridors. All of these activities take land out of production for a period of time (Adams and Kelsey Reference Adams and Kelsey2012, Glenna et al. Reference Glenna, Babbie, Kelsey and DeLessio-Parson2014). Anecdotally, production declines could last up to five years following infrastructure installation (Seachrist Reference Seachrist2011). As unconventional drilling methods require massive amounts of water mixed with various chemicals to stimulate flow in tight shale formations, there is a risk of contamination as part of this fluid mixture returns to the surface (Olmstead et al. Reference Olmstead, Muehlenbachs, Shih, Chu and Krupnick2013). Additional runoff from land altered around the drilling site poses another threat to both land productivity and soil fertility. Livestock could also be susceptible to changes in water quality (Bamberger and Oswald Reference Bamberger and Oswald2012).
Aside from the physical drilling process, leasing and royalty payments present another avenue for shale gas development to affect agriculture. Due to the potentially large lease and royalty payments received by farmers (Weber, Brown, and Pender Reference Weber, Brown and Pender2013), shale gas development can provide an opportunity for farmers to pay off loans, purchase new equipment, or otherwise invest in their farming activity. Leasing and royalty payments may be capitalized into property values (Weber, Burnett, and Xiarchos Reference Weber, Burnett and Xiarchos2016) and can act to offset potential negative externalities. Weber and Hitaj (Reference Weber and Hitaj2015) find appreciation in farm land values in areas with shale gas development. However, if mineral rights are severed, landowners receive no payments from the oil and gas industry and thus have little potential of receiving positive benefits to offset negative externalities. Furthermore, windfall income could cause farmers to divest from farming either partially or wholly.
In addition to new streams of income for land owners, shale gas development can also increase demand for agricultural products. Straw is sometimes used to prevent runoff from pipeline, well pad, and other surface disturbances, and to protect soil, allowing grass to regrow. There can also be competition for inputs, such as straw, labor, and land (used for well pads and other infrastructure). As a result of Marcellus development, Glenna et al. (Reference Glenna, Babbie, Kelsey and DeLessio-Parson2014) note shortages in certain agricultural inputs. They also cite issues related to retaining farm labor.
To evaluate the impacts of unconventional gas development on farming we combine Census of Agriculture and gas drilling data for the Marcellus region (New York, Ohio, Pennsylvania, and West Virginia). We exploit a quasi-experimental approach to estimate how 18 agricultural variables change over time for counties with and without drilling. To limit potential bias due to heterogeneity in the study region, we use the common support from an estimated propensity score to trim our sample. Compared to past studies, we contribute to the literature by providing a comprehensive econometric estimation of the effects of shale gas development on agriculture in the Marcellus region. With a general focus, we explore more variables than in prior studies that focused on land values (Weber and Hitaj Reference Weber and Hitaj2015) and dairy farming (Adams and Kelsey Reference Adams and Kelsey2012, Finkel et al. Reference Finkel, Selegean, Hays and Kondamudi2013), while our econometric estimation builds upon the descriptive statistics reported in Hitaj, Boslett, and Weber (Reference Hitaj, Boslett and Weber2014) and Xiarchos et al. (Reference Xiarchos, Hoy, Doyle, Romania, Brasier, Glenna and Kelsey2017).
We find that shale gas development has an effect on some but not all agricultural variables studied. Our most robust results indicate that there is a significant increase in median farm sizes in drilling counties. Further, in counties with shale gas development there is little change in per-farm sales from 2007 to 2012. Our results are particularly useful for both policy makers and agribusiness managers in regions experiencing an unconventional energy boom.
Literature Review
There is a large and growing body of economic research on the employment and income effects of unconventional gas and oil development. This includes Marchand (Reference Marchand2012), who applies difference-in-differences methods to compare employment and earnings growth from 1971 to 2006 in western Canada, finding modest job creation impacts within non-energy sectors. Weber (Reference Weber2012) uses a similar difference-in-differences approach to study impacts of natural gas development in Colorado, Wyoming, and Texas, and finds modest increases in employment, wage and salary income, and median household income. Brown (Reference Brown2014) looks at natural gas development in a nine-state region in the central United States, and using a two-stage least squares modeling approach, similarly finds modest increases in employment. Wrenn, Kelsey, and Jaenicke (Reference Wrenn, Kelsey and Jaenicke2015) use a triple difference model to examine employment impacts from Marcellus shale development in Pennsylvania, also finding modest impacts on local employment. Other studies find limited to no effects of Marcellus development on employment and wages (Cosgrove et al. Reference Cosgrove, LaFave, Dissanayake and Donihue2015, Paredes, Komarek, and Loveridge Reference Paredes, Komarek and Loveridge2015, Komarek Reference Komarek2016), while results using expanded study regions also conclude that estimated effects are smaller than those based on input-output analysis (Weinstein Reference Weinstein2014, Munasib and Rickman Reference Munasib and Rickman2015).
A smaller number of hedonic studies based on shale gas development focus on the Marcellus region. Boslett, Guilfoos, and Lang (Reference Boslett, Guilfoos and Lang2016), Gopalakrishnan and Klaiber (Reference Gopalakrishnan and Klaiber2014), and Muehlenbachs, Spiller, and Timmins (Reference Muehlenbachs, Spiller and Timmins2015) find mixed impacts on property values, depending on water source, expectations of gas development, and land type. In particular, there is a more persistent negative effect on property values when land is classified as agricultural (Gopalakrishnan and Klaiber Reference Gopalakrishnan and Klaiber2014).
Fewer studies have directly considered unconventional drilling's overall impact on agricultural activity. Because unconventional drilling is occurring in rural areas, where agriculture can be a key economic sector, studying outcomes other than just employment and income becomes increasingly important. In one of the first agricultural studies, Adams and Kelsey (Reference Adams and Kelsey2012) use National Agricultural Statistics Service data to explore dairy farming and Marcellus shale activity in Pennsylvania between 2007 and 2010. The authors find high levels of drilling activity are associated with decreases in dairy cow numbers and milk production. Their findings are confirmed by Finkel et al. (Reference Finkel, Selegean, Hays and Kondamudi2013).
Weber, Brown, and Pender (Reference Weber, Brown and Pender2013) estimate that the $2.3 billion in lease and royalty payments paid by energy companies to farmers added, on average, $104,000 in wealth to recipient farms.Footnote 1 Such payments can be significant compared to other income. In terms of actual payments of lease and royalty dollars to farms during 2011, they find the national median was $7,000, while the average payment exceeded $30,000. The median payment in the Atlantic region was lower than the nation, highlighting both the range of differences in payments among all farms and among different regions. Indeed, in very active drilling counties, the value of lease and royalty income can far outweigh new wage income associated with drilling (Hardy and Kelsey Reference Hardy and Kelsey2015). It is critical, therefore, to study the implications of this potential increased income for farmers because of its potential direct contribution to local economies and agricultural systems.
Weber and Hitaj (Reference Weber and Hitaj2015) find that the effect on farm real estate values is significantly dependent on whether or not the farmers own the oil and gas rights. They find some limited effects of shale gas development on farm real estate values in the Barnett Shale region of Texas, an area with a long history of drilling and where split estates are more likely. This finding is in contrast to a large positive effect in northeastern Pennsylvania, which does not have a history of gas drilling. In contrast, they did not study the southwest portions of the Marcellus region (including southeast Ohio, southwest Pennsylvania, and the majority of West Virginia), which have a long and robust history of coal and gas extraction.Footnote 2
However, income is not the only impact from shale gas development. As part of a larger study, Glenna et al. (Reference Glenna, Babbie, Kelsey and DeLessio-Parson2014) conducts several focus groups with farmers in four Pennsylvania counties to identify effects of unconventional drilling activity. The participants said the development led to a shortage of some farm inputs such as lime, and made it difficult to retain farm labor because the industry could pay much higher wages. On the other hand, they said the lease and royalty dollars allowed farmers to reinvest in their operations, make significant changes to their operations (such as from labor-intensive dairy to other products), exit farming partially or wholly, and more easily pass farms to the next generation. The participants expressed uncertainty about the long-run impacts of drilling in their regions, including potential environmental impacts. The popular press and some agricultural leaders have raised questions about the viability of organic agricultural certification in these regions because of concerns about contamination.
More generally, Hitaj, Boslett, and Weber (Reference Hitaj, Boslett and Weber2014) present a basic descriptive analysis of changes in agricultural variables across counties in the south-central United States and western Plains. Xiarchos et al. (Reference Xiarchos, Hoy, Doyle, Romania, Brasier, Glenna and Kelsey2017) similarly evaluate how drilling intensity is associated with changes in agricultural variables for different geographic areas of the Marcellus region. While results differ by geographic area, both studies identify farm consolidation, labor pressures, and increased capital investments in land and equipment across counties with shale gas development.
The focus of prior literature on the economic and housing market impacts of shale gas has provided little on the effects of drilling on agriculture. Of the few studies focused on farming, most have considered only one major impact, such as land values or dairy farming. Because of the concerns raised in qualitative studies and by the popular press, we add to the literature by exploring 18 agricultural variables across the entire Marcellus region. We build on the above descriptive studies by adopting a multivariate regression model to analyze overall changes in farming related to shale gas development in the Marcellus region.
Data and Background
Our study uses the most comprehensive farming data publicly available, the U.S. Department of Agriculture NASS Census of Agriculture, to examine how unconventional shale gas development is affecting farming in the Marcellus region. Our analysis considers all unconventional drilling activity within the region, which consists of wells drilled in both Marcellus and Utica shale gas plays. The study states chosen, New York, Ohio, Pennsylvania, and West Virginia, encompass the majority of the economically feasible region of the Marcellus and Utica plays.
The following subsections discuss drilling and agricultural data.
Shale Gas Drilling
The Marcellus region is home to two active shale plays, the Marcellus and Utica. Most drilling has occurred in Pennsylvania and West Virginia, with both states targeting the Marcellus play. In Ohio, most wells are drilled in the Utica play.Footnote 3 Because the Utica lies beneath the Marcellus play, there has been substantially less drilling in the Utica play in our study region. We assume there are no real differences in potential impacts of drilling on agriculture between the Marcellus and Utica plays because the technology and processes used in the two plays are almost identical and thus would have a similar impact on agricultural activity.Footnote 4
We use county-level unconventional well spud data for 2002 through 2012, from either state departments that directly oversee oil and gas development or agencies contracted to construct a well database (Ohio Department of Natural Resources 2014, Pennsylvania Department of Environmental Protection 2014, West Virginia Geological & Economic Survey 2014).Footnote 5 In the Marcellus region, 103 (38 percent) counties have at least one unconventional well (Table 1). Through the end of 2012, 8,738 unconventional wells were drilled. Conditional on drilling, the average number of wells was approximately 85, and the median was 18. Bradford County, Pennsylvania's 1,121 wells were the most of any single county.
Table 1. Well Statistics by State for Counties with Unconventional Drilling (2002–2012)

Figure 1 displays the county-level number of unconventional wells in the Marcellus region through 2012. Most drilling activity took place in West Virginia through northeastern Pennsylvania. Despite exploratory drilling occurring shortly after 2002, it was not until about five years later that the gas industry intensified drilling efforts. During 2009 nearly 1,000 unconventional wells were spudded in the region, reaching a high of 2,289 wells in 2011. After this large expansionary drilling period, the number of wells drilled declined for the last year in our study period.

Figure 1. Unconventional Drilling Activity in the Marcellus Region
Pennsylvania has the most unconventional drilling activity, followed by West Virginia. Ohio comprises a small share of the overall number of wells. In terms of the onset of drilling, West Virginia was the first state to have a sizeable number of spudded wells, followed closely by Pennsylvania (West Virginia surpassed 100 cumulative wells drilled in 2005, while Pennsylvania passed this threshold during 2007). Ohio lagged behind the other two states in drilling, surpassing 100 cumulative wells drilled during 2012. Due to potential public health and water concerns, no production-oriented drilling occurred in New York, culminating in a formal moratorium in 2014.Footnote 6 Therefore, our analysis assumes no unconventional drilling has occurred within the state.
Agricultural Activity
Every five years the USDA NASS (2002, 2007, 2012) conducts a comprehensive survey of all farms across the nation.Footnote 7 We compile data from the 2002, 2007, and 2012 Censuses of Agriculture on 18 farming-related variables (variable definitions can be found in Table A1).
The study states vary considerably in the extent and onset of drilling, and there is also heterogeneity in the types and scale of agriculture in the Marcellus region. According to the 2012 Census of Agriculture, there were 191,797 farms in the region, about 9 percent of all farms in the United States. Ohio has the most farms in the Marcellus Region, 75,462, while West Virginia has the least farms, 21,489. Ohio also has the largest market value of agricultural products sold, ranking 13th nationally. Although neither New York's nor Pennsylvania's total market value of sales are quite as large as Ohio's, West Virginia has the smallest agriculture sector, ranking 41st in value of products sold. The four states also derive the largest share of sales from different sources. The largest shares are milk from cows in New York and Pennsylvania, grains in Ohio, and poultry and eggs in West Virginia.
Table 2 presents full sample summary statistics for the 18 agricultural variables in our study. Values are for the year 2002, prior to the onset of shale gas drilling. For purposes of this study we define a drilling (treatment) county as a county that had at least 18 cumulative spudded unconventional gas wells through 2012, the median number of wells among counties with drilling. Such a threshold ensures that the wells drilled were not simply exploratory wells in areas where production is infeasible, and that there is a sufficient level of noticeable drilling activity.Footnote 8 Using this classification, there are 52 treatment counties and 220 control counties in the full sample.
Table 2. Agricultural Variable Descriptive Statistics (2002)
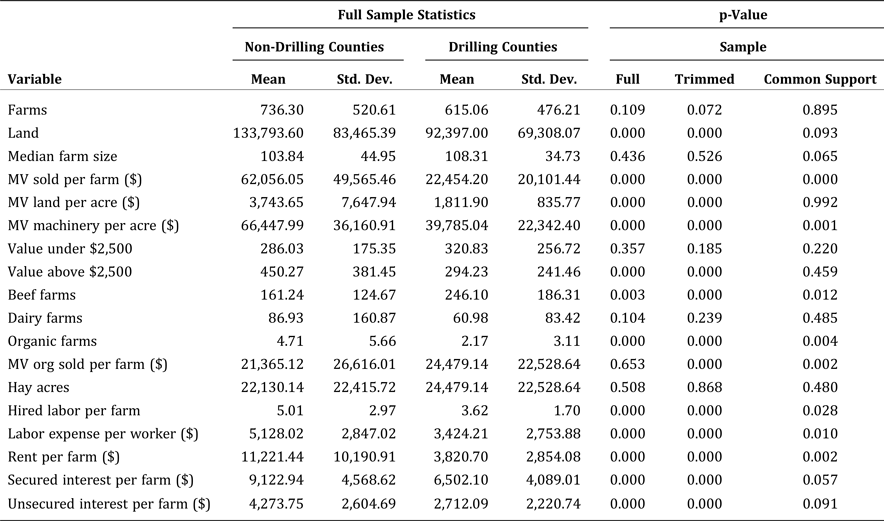
Notes: The full sample consists of 220 non-drilling and 52 drilling counties, the trimmed sample consists of 100 non-drilling and 51 drilling counties, and the common support sample consists of 42 non-drilling and 21 drilling counties. Treatment counties are those with at least 18 shale gas wells. Not all variables are disclosed for every county, normally to maintain respondent confidentiality, so the number of counties in the samples serve as upper bounds.
p-Value corresponds to the null hypothesis that the means are not statistically different.
See Table A1 for variable definitions.
Overall, there is a larger agricultural sector in non-drilling counties, as evidenced by a greater number of farms, land in farms, value of land and buildings, value of machinery and equipment, and value of total sales. This likely reflects differences in agro-ecological conditions between drilling and non-drilling counties. The Marcellus play is largely located beneath the Allegheny Plateau, an area characterized by poorer soil quality than that found in the ridge and valley region of southeastern Pennsylvania.Footnote 9 A similar story holds for the location of drilling in West Virginia, although based on market value of sales, agricultural activity is of a much smaller scale for the state. Given these conditions, it is unsurprising that more farms with total value of sales of less than $2,500 are found in drilling counties. Notably, there is a larger number of dairy farms in non-drilling counties, while the number of beef farms is significantly higher in drilling counties.
The 18 variables considered were chosen either because they represent indicators of the overall agricultural economy (such as the number of farms), or because they have been associated with specific local changes that can accompany increased activity in unconventional gas drilling (such as increased competition for labor). We discuss some rationale for exploring each variable in more detail below:
• Number of Farms: This variable measures the size of the overall farm economy. Large lease and royalty payments could help farmers to maintain their operations, or retire from farming altogether.
• Land in Farms: This variable measures the size of the overall farm economy. Land could be taken out of farming due to drilling leading to decreases in total farming acres.
• Median Farm Size: Hitaj, Boslett, and Weber (Reference Hitaj, Boslett and Weber2014) suggest that drilling could be associated with industry consolidation. Xiarchos et al. (Reference Xiarchos, Hoy, Doyle, Romania, Brasier, Glenna and Kelsey2017) also find increases in median farm size in counties with drilling. A potential mechanism for industry consolidation may result from farms that receive lease and royalty payments deciding to scale down their operations or exit the industry, while remaining operations buy their land and capital assets.
• Market Value of Agricultural Products Sold per Farm: This variable measures the size of the overall farm economy. If drilling takes land out of production, per-farm sales could decline.
• Market Value of Land and Buildings per Acre: Lease and royalty payments could be reinvested through building additions or renovations. Land owners could also capitalize the value of the gas under their property into the self-reported land values. Weber and Hitaj (Reference Weber and Hitaj2015) find greater appreciation in land values in three northeastern Pennsylvania counties relative to four New York counties.
• Market Value of Machinery and Equipment per Farm: Land owners could reinvest windfall payments through purchases of machinery and equipment.
• Farms with Value of Sales greater than and less than $2,500: Windfall payments can allow farmers to maintain small-scale operations. Farmers could also downsize operations if windfall payments are large enough, choosing to operate a hobby farm.
• Number of Beef Farms: If windfall payments cause farmers to transition away from dairy farming (Glenna et al. Reference Glenna, Babbie, Kelsey and DeLessio-Parson2014), beef farming is one potential alternative.
• Number of Dairy Farms: Dairy is the largest farm sector in both New York and Pennsylvania. Glenna et al. (Reference Glenna, Babbie, Kelsey and DeLessio-Parson2014) note that windfall payments may allow farmers to transition from labor-intensive dairy farming to less labor-intensive sectors, leading to a decline in dairy farm numbers (Adams and Kelsey Reference Adams and Kelsey2012).
• Organic Farms and Value of Sales: Media reports suggest there are consumer concerns about contamination of organic products due to hydraulic fracturing (Hopey Reference Hopey2014).
• Hay Acres Grown: Farmers transitioning out of dairy may focus on hay crops, increasing acres devoted to hay production.
• Labor: The need for labor during the drilling process could create competition for local labor, leading to wage increases. Hitaj, Boslett, and Weber (Reference Hitaj, Boslett and Weber2014) find higher labor expenses across counties in the south-central and western regions of the United States, while Xiarchos et al. (Reference Xiarchos, Hoy, Doyle, Romania, Brasier, Glenna and Kelsey2017) find decreases in hired labor among shale gas counties in the Marcellus region.
• Rent Expense per Farm for Buildings and Land: If drilling or the expectation of drilling activity reduces the attractiveness of nearby land, farm rental expenses could decline.
• Secured and Unsecured Interest Expense per Farm: Windfall income could be used to pay off liabilities, causing a decrease in interest expenses.Footnote 10
We choose to exclude property taxes paid and acres irrigated from our analysis. Ohio and West Virginia levy a property tax on minerals while Pennsylvania does not.Footnote 11 Therefore, estimation of changes in property tax payments based on treatment status would be biased due to different tax structures. As irrigation is not a significant part of agriculture in the Marcellus region, we assume there is little change in acres irrigated due to drilling.
Methods
An ideal experiment would randomly assign counties to drilling (treatment) and non-drilling (control) groups, creating an unbiased estimate of the differential effect of unconventional gas development, given no confounding factors. However, in non-laboratory settings, we are faced with non-random assignment to treatment and control groups. Although the location of shale gas formations is exogenous, the decision to drill in a particular location is based on a complex set of factors. Ownership of mineral rights, existing infrastructure (especially pipelines), transportation networks (roads and rail), and access to large regional markets would decrease the costs and increase the potential return on investment from drilling. In addition, there are scale economies in drilling, with crews typically working at multiple sites across a region. State- and county-level policies also influence the decision to drill, such as differences in tax structures—namely income, real estate, and severance.
A central issue in estimating the causal effect of gas development is the degree of heterogeneity in the Marcellus region. We attempt to control for this heterogeneity, initially, by trimming the data based on three factors. Due to the presence of drilling in rural areas, and the differences in urban and rural farming, we exclude counties with a population above the 90th percentile based on the 2000 Census. We also exclude counties with fewer than 18 unconventional wells, our chosen treatment level. Finally, we exclude any non-treatment county that borders a treatment county. Border counties are removed due to potential concerns about spatial spillovers caused by labor mobility or the determination of commodity prices in regional markets.Footnote 12 Trimming based on these three factors reduces the number of drilling (treatment) counties from 52 to 51 and the number of non-drilling (control) counties from 220 to 100.
In addition to trimming our sample by excluding counties with 1–17 unconventional wells, counties with populations above the 90th percentile, and border counties, we estimate a propensity score to further control for heterogeneity among treatment and control counties. A propensity score models the probability of treatment conditional on a set of control variables (Rosenbaum and Rubin Reference Rosenbaum and Rubin1983). We estimate the propensity score for a county having 18 or more unconventional wells drilled over the study period through a binary logit model containing 17 demographic, geographic, and socioeconomic variables (variables, definitions, and data sources can be found in Table A2).
After estimation of the propensity score (coefficient estimates can be found in Table A3), we use the estimated common support to further trim our sample. The common support can be thought of as the counties that are the most similar, conditional on the control variables. In other words, we only consider counties with predicted probabilities of drilling that fall in the range of the minimum value for a drilling county and the maximum value for a non-drilling county. For example, this removes the populous counties near the New York City metro that are highly unlikely to receive the treatment of drilling but have populations less than the 90th percentile. Doing so reduces the bias in the differential effect that would result from estimating a simple ordinary least squares model, ignoring heterogeneity among the sample counties. Table 3 presents full sample summary statistics for variables used in the propensity score estimates.
Table 3. Demographic Variable Descriptive Statistics

Notes: The full sample consists of 220 non-drilling and 52 drilling counties, the trimmed sample consists of 100 non-drilling and 51 drilling counties, and the common support sample consists of 42 non-drilling and 21 drilling counties. Treatment counties are those with at least 18 shale gas wells. Not all variables are disclosed for every county, normally to maintain respondent confidentiality, so the number of counties in the samples serve as upper bounds.
p-Value corresponds to the null hypothesis that the means are not statistically different.
See Table A2 for variable definitions.
Our empirical model estimates how unconventional gas development affects agricultural outcomes over time using data from the 2002, 2007, and 2012 Censuses of Agriculture. Letting drillingi indicate whether county i had 18 or more unconventional gas well spudded through 2012 and yeart be a time fixed effect, we estimate the following equation

where Agit is one of 18 agricultural variables, φ i is a time-invariant fixed effect, and ε it is a normally distributed error term.Footnote 13
In the above model,α represents the average value of the dependent variable for the base year of 2002, and α + β2007 represents the average value of the dependent variable for 2007. For non-drilling counties, α + β2012 is the average outcome, and in drilling counties the expected value is α + β2012 + γ. Therefore, γ is the differential effect of unconventional gas development.
Identification of the differential effect of drilling relies on the parallel paths assumption, whereby the dependent variable for treatment and control counties are growing at a similar rate before treatment.Footnote 14 This is a strong assumption, but we can inspect this graphically by plotting average treatment and control values over time and then looking at the changes between 2002 and 2007. As an example, Figure 2 plots the median farm size over time. In both treatment and control counties there was a similar decline in median farm size from 2002 to 2007, implying that the parallel paths assumption is not unreasonable. Visual inspection suggests that 14 of our 18 study variables satisfy the parallel paths assumption.Footnote 15

Figure 2. Time Trends for Treatment and Control Counties, Median Farm Size
Results
The results of the propensity score model are presented in Table A3. The common support of the sample is 0.0128 to 0.8571, resulting in a common support sample of 21 drilling (treatment) counties and 42 non-drilling (control) counties. Our final sample is depicted in Figure 3. There are 25 control counties in New York, 11 in Ohio, 3 in Pennsylvania, and 3 in West Virginia, as well as 3 treatment counties in Ohio, 12 in Pennsylvania, and 6 in West Virginia.

Figure 3. Final Common Support Sample
We offer a full interpretation of the number of farms regression, while focusing on the comparison of drilling and non-drilling counties for other regressions. The average number of farms per county was 664.6 in 2002 (Table 4). The number of farms grew to 682.5 (664.6 + 17.9) in 2007. The number of farms in 2012 fell to 650.4 (664.6–14.2) in non-drilling counties and 646.4 (664.6–14.2–4.0) in drilling counties.Footnote 16 The drilling × year2012 coefficient, or the differential effect of shale gas drilling, was negative but not statistically significant.
Table 4. Agricultural Variables Common Support Regression Results

Notes: The coefficients are estimated by equation 1. Drilling is an indicator variable for counties with at least 18 shale gas wells. Standard errors clustered by county are displayed in parentheses.
*Significant at the 10 percent level, **Significant at the 5 percent level, ***Significant at the 1 percent level.
There is an estimated decrease in the number of acres of farmland in both 2007 and 2012. Despite the overall decrease, there is no significant differential effect for total acres in farmland. There is also a general decline in median farm sizes, although there is a positive differential effect.
A large and negative differential effect exists for the market value of sales per farm. The point estimates imply that farms in counties without shale gas development experienced an increase in sales of roughly $50,000 from 2007 to 2012, while there was a slight decline in drilling counties. The self-reported market value of land and buildings per acre appreciated in all counties, although the differential effect point estimate is not significant, suggesting there was no increase in land values beyond normal appreciation. The market value of machinery and equipment differential effect is negative and significant, about $10,000 less than the increase in non-drilling counties.
Small-scale farms, those with values of sales less than $2,500, have negative time and differential effects for 2012 (Table 5). The point estimates imply a loss of about 67 small-scale farms in drilling counties, relative to 2002 levels. Larger-scale farms, those with values of sales greater than $2,500 have positive time and differential effects, implying an increase of 48 large-scale farms over the study period.
Table 5. Agricultural Variables Common Support Regression Results

Notes: The coefficients are estimated by equation 1. Drilling is an indicator variable for counties with at least 18 shale gas wells. Standard errors clustered by county are displayed in parentheses.
*Significant at the 10 percent level, **Significant at the 5 percent level, ***Significant at the 1 percent level.
There is a large and significant negative differential effect in the number of beef farms for drilling counties. The average number of dairy farms is declining among non-drilling and drilling counties while the differential effect is not statistically significant. Both the number of organic farms and market value of organic sales per farm are increasing over time, but there is no significant effect of drilling on either outcome.
The differential effects for the number of hay acres grown is positive but not statistically significant, compared to a large negative year effect for both 2007 and 2012 (Table 6). There is an overall decline in hired labor per farm in 2012, with no significant decrease in drilling counties. The differential effect for hired labor expense per worker is negative and significant, with the average drilling county wage increasing by roughly half of the wage in non-drilling counties from 2007 to 2012. Although rent for land and buildings increases in all counties, a negative differential effect implies the increase is relatively small in drilling counties. Finally, the differential effect point estimates for secured interest and unsecured interest expenses are negative but not significant.
Table 6. Agricultural Variables Common Support Regression Results

Notes: The coefficients are estimated by equation 1. Drilling is an indicator variable for counties with at least 18 shale gas wells. Standard errors clustered by county are displayed in parentheses.
*Significant at the 10 percent level, **Significant at the 5 percent level, ***Significant at the 1 percent level.
Robustness Checks
As a robustness check we explore the sensitivity of our results to the definition of a treatment county. Our results assume there is a homogenous treatment. For this to be the case, choosing a number of wells either above or below 18 should not significantly alter our results. To conduct these robustness checks, we re-estimate our model using 3 wells (25th percentile) and 50 wells (68th percentile) as the cutoff for whether a county is treated or not.Footnote 17
Table 7 presents the results of our robustness checks.Footnote 18 The first column reproduces our main results, while the second column presents estimates when we define treatment as 3 wells, and the third when treatment is defined as 50 wells. We also present adjusted p-values that account for the fact that we are testing 18 hypotheses using the same data.Footnote 19 The adjustment procedure is developed in Benjamini and Hochberg (Reference Benjamini and Hochberg1995) and discussed in Anderson (Reference Anderson2008). First, we note that when accounting for correlations in the statistical tests, four differential effects are no longer significant at conventional levels—market value of machinery per farm, farms with sales under $2,500, farms with sales above $2,500, and labor expense per worker. Second, the estimated coefficients are generally of the same sign and magnitude when we vary treatment cutoffs. One coefficient has a different sign when treatment is defined as 3 wells (MV land/acre), while 4 coefficients have different signs when treatment is defined as 50 wells (land, MV land/acre, secured interest/farm, and unsecured interest/farm). None of the coefficients that have different signs than our main results are statistically significant. Finally, the four coefficients from our main results that are significant based on the adjusted p-values are significant across the different treatment definitions.
Table 7. Robustness Checks Regression Results

Notes: The coefficients in the first three columns are estimated by equation 1, varying the number of wells defining treatment. The fourth column uses the number of wells drilled as an independent variable in place of the drilling × year interaction term. p-Values are adjusted to account for multiple hypothesis tests.
Table 7 also presents results from a regression model where we use the number of wells as an independent variable instead of the drilling × year2012 interaction term. Of the 18 variables considered, 12 have the same sign as our main results. Of the 6 variables with different signs, none of the estimated coefficients are statistically significant.
Discussion
The average number of farms per county decreased from 2002 to 2012, while the differential effect is not significant. Our result differs from two reports presenting average percentage changes in drilling and non-drilling counties. Hitaj, Boslett, and Weber (Reference Hitaj, Boslett and Weber2014) find that there is a greater increase in the number of farms in counties without shale gas. Xiarchos et al. (Reference Xiarchos, Hoy, Doyle, Romania, Brasier, Glenna and Kelsey2017) find that higher levels of drilling are associated with larger percentage decreases in the number of farms in the Marcellus region. Accompanying the overall decline in the number of farms is a decrease in the total acres of farmland. Again, drilling counties are subject to the overall trend, but there is no significant differential effect. Taken together, our results suggest that, in aggregate, farmers in drilling counties are not removing land from production due to drilling. As a comparison, Xiarchos et al. (Reference Xiarchos, Hoy, Doyle, Romania, Brasier, Glenna and Kelsey2017)’s bivariate analysis finds a correlation between decreases in farmland and drilling activity.
Hitaj, Boslett, and Weber (Reference Hitaj, Boslett and Weber2014) hypothesize that drilling could be associated with industry consolidation. Using the median size of farms as a measure of consolidation, we find that there is a positive differential effect, providing potential evidence of consolidation among farms in shale gas counties. When combined with the general trend of fewer farms and less land in farming, consolidation seems likely. Similar results are found by Xiarchos et al. (Reference Xiarchos, Hoy, Doyle, Romania, Brasier, Glenna and Kelsey2017), where changes in median farm size are greatest among drilling counties. Although there is evidence of farm consolidation, there was no change in the market value of products sold from 2007 to 2012 in drilling counties. It is worth noting that in the full sample, farms in drilling counties have a significantly lower value of products sold in 2002.
In terms of farm investment, our results using county-level data are similar to those of Weber and Hitaj (Reference Weber and Hitaj2015), who use farm-level data. There was a positive, but not statistically significant, differential effect for the value of land and buildings per acre. Compared to the significant point estimate of Weber and Hitaj (Reference Weber and Hitaj2015), there is heterogeneity among the likelihood of split estates in our sample. The northeastern region of Pennsylvania had little prior conventional gas production, increasing the probability that mineral rights are not severed, compared to the southwest region of Pennsylvania and West Virginia.Footnote 20 Nevertheless, this provides evidence that farmers could be capitalizing the value of leases into self-reported property values.Footnote 21 An alternative, though not mutually exclusive explanation, is that farmers are using lease payments to invest in farming through building construction and renovation.
The negative differential effect for machinery and equipment per farm suggests that while there is an overall reinvestment in farming, operators in non-drilling counties are investing more in their operations than their counterparts in non-drilling counties. When we adjust p-values (Table 7), the differential effect is not significant, implying there is no difference between treatment and control counties. This result conforms to anecdotal reports, which indicate that some lease and royalty payments are being spent on machinery such as tractors (Glenna et al. Reference Glenna, Babbie, Kelsey and DeLessio-Parson2014), and findings by Hitaj, Boslett, and Weber (Reference Hitaj, Boslett and Weber2014) and Xiarchos et al. (Reference Xiarchos, Hoy, Doyle, Romania, Brasier, Glenna and Kelsey2017).
Our main results suggest that there is a change in the composition of farms by total market value of sales. Smaller-scale farms, those with market values of sales less than $2,500, decreased by an average of approximately 40 farms in non-drilling counties and 67 farms in drilling counties over the study period. Such farms often are “lifestyle” farms intended to help supplement household food and income, rather than being full-time businesses. Conversely, farms with values of sales greater than $2,500 grew by an average of 26 farms in non-drilling counties and 49 farms in drilling counties relative to 2002. Overall, this implies a net loss of farms in both non-drilling and drilling counties. This trend can encompass both small farms receiving lease and royalty payments that are divesting from agriculture, and other farms that are deciding to significantly increase their farming activities, which corroborates the notion of farm consolidation in counties with drilling. Nevertheless, while the differential effects are significant in our main results, they are not when we adjust the p-values.Footnote 22
One agricultural transition discussed in previous literature and the popular press is the potential movement away from dairy, often toward beef or hay. Drilling counties have a significantly larger average number of beef farms and fewer dairy farms. Our results suggest that there is a large decrease in the number of beef farms in drilling counties, while there is a decrease in the number of dairy farms among both drilling and non-drilling counties.Footnote 23 In other words, there is a transition away from dairy in all counties, but there is some additional factor present in drilling counties driving farmers away from beef. This may reflect that beef production in this region tends to be done by lifestyle farms (25 percent of Pennsylvania beef farms, for example, have less than $1,000 in annual sales, and 50 percent have less than $5,000 in such sales [U.S. Census of Agriculture]) so the loss of lifestyle farms disproportionately affects the number of beef farms. In addition, Bamberger and Oswald (Reference Bamberger and Oswald2012) argue that livestock are highly susceptible to water quality impacts from shale gas development; perceived risks or actual impacts on animal health could lead to farmers exiting beef farming.
Both the number of organic farms and market value of organic products sold increase over time. Although concerns exist about the hydraulic fracturing process and possible contamination, there is no significant effect on organic farming in drilling counties. This is true for our main results and the various robustness checks.
In addition to beef farming, another option for downsizing or transitioning dairy farmers is hay production. We find a positive, but not statistically significant, differential effect for acres of hay grown. This indicates there is little change due to shale gas development. Combining the beef, dairy, and hay results, our analysis finds evidence of an overall transition away from dairy, although at the county level we find little support for the notion that dairy farms are transitioning to less labor-intensive beef farming or hay production. One potential explanation could be the presence of lease and royalty payments. Increased wealth from lease and royalty payments may allow farmers who had already switched to less labor-intensive beef or hay to retire from farming.
Shale gas drilling can cause labor competition in drilling counties (Glenna et al. Reference Glenna, Babbie, Kelsey and DeLessio-Parson2014, Hitaj, Boslett, and Weber Reference Hitaj, Boslett and Weber2014). We find that there is a decline in the number of workers per farm in drilling and non-drilling counties during 2012, but no significant differential effect. Labor competition could drive up wages in local markets, manifesting itself in larger per worker labor expenses. The change in per-worker expenses from 2007 to 2012 was about $2,000 in non-drilling counties, but less than $1,000 in drilling counties. Although there might be labor competition in drilling counties, the effect is not manifesting in higher per-worker wage expenses relative to non-drilling counties.
Drilling could also cause a negative externality for people renting farmland, which could be reflected in per-farm rental expenses. As Deede (Reference Deede2014) notes, renters of agricultural land lack the ability to coordinate their farming operation with drilling activities. From 2007 to 2012, we find that there is little change in rental expenses in drilling counties. Finally, farmers receiving lease and royalty dollars could use these windfall payments to retire debt sooner, a trend reported in qualitative studies (Glenna et al. Reference Glenna, Babbie, Kelsey and DeLessio-Parson2014). As the differential effects for both secured and unsecured interest expense are not significant, our county-level results disagree with prior qualitative studies.
Conclusions
Unconventional gas development is affecting residents and communities in the Marcellus region and the United States, injecting new revenue streams into primarily rural counties. Due to this, there is considerable interest in determining whether or not and how unconventional gas development is impacting agriculture, with consequent implications for public land use, agricultural, and economic development policy. Through a quasi-experimental approach, we estimate the differential effects of drilling on county-level agricultural outcomes.
For most agricultural variables considered, we find no significant effect of drilling on agriculture. For example, although we find no significant change in the number of farms or land in farms due to shale gas development, an increase in the median farm size implies potential consolidation among drilling counties. Based on our results, both the number of small-scale farms and beef farms are declining due to drilling in the Marcellus region. Therefore, policy makers could consider measures that could help these segments of the agricultural sector. Although drilling could be a significant influence on agricultural activity, it is just one element in a complex system that determines agricultural decision-making and outcomes. Further, the effects found in this study could be transitory.
An extension of our study could use 2017 Census of Ag data and the dramatic slowdown in drilling activity that has happened since 2012 to determine if drilling impacts on agriculture are temporary, disappearing once the drilling activity itself slows or ends. Komarek (Reference Komarek2016) finds that labor market impacts from drilling persist for about four years. Due to the use of county-level data, we are unable to account for farm-level heterogeneity. Another extension of our work would involve the analysis of farm-level data. Finally, because of the unique characteristics of agriculture and mineral rights ownership in the Marcellus region, our analysis could be extended to consider other regions affected by the contemporary shale gas boom.
APPENDIX
Table A1. Agricultural Variables and Definitions

Table A2. Demographic and Socioeconomic Variables, Definitions, and Data Sources

Table A3. Propensity Score Estimation Results
