I. INTRODUCTION
The exponential growth in digital technology is leading us to a future in which all things and all people will be connected all the time. This is a vision that the authors refer to as “The Infinite Network (TIN)” [Reference Balram1]. TIN will change all major industries in very fundamental ways. In this paper, we focus on the impact it will have on healthcare, in particular on the big changes in the way that each person's health will be managed throughout their lives due to the availability of continuous communication, immense compute power, large-scale local storage and the transition to a big-data-driven approach.
Section II provides an overview of TIN, the major trends driving toward it and the opportunities and challenges that this brings. TIN changes the essence of healthcare to something that occurs continuously as the management of a person's health at all times rather than the traditional form of discrete management of minor and major problems at the times they occur. The changes in individual health care/management can be looked at from a micro (individual) and macro (aggregate) point of view.
At the micro level, one of the key drivers will be the use of smart sensing to gather useful information that can be used for holistic care – maintaining emotional and physical wellness, arriving at early and accurate diagnosis and providing timely and effective treatment. Section III describes smart sensing, breaking it down into three categories – sensing inside the body (embedded sensors), sensing on the outside of the body (wearable sensors), and sensing by smart medical devices.
At the macro level, the key direction is the development of Intelligent Telehealth Networks that can deliver individualized and continuous care to large populations using the advanced digital technologies that are arriving as part of TIN. The evolution of these networks starting from traditional telemedicine, moving to advanced telehealth systems, and then to intelligent hybrid systems is described in Sections IV, V, and VI.
There is a long history of telemedicine being used to deliver remote care in areas where local availability of doctors is an issue. Traditional networks have achieved a certain amount of scale in regions where the conditions and economics are favorable but have not achieved large scale across large geographical areas due to a number of factors. These networks are described briefly in Section IV through two examples.
New smart telehealth networks that are emerging now have the potential to achieve large scale across much larger regions by using state-of-the-art information and communication technology structures and approach. This kind of network is described in Section V using a prominent example from our experience.
The long-term promise of TIN in healthcare is the development of a smart network that can combine the big medical data provided by smart sensing with the telehealth services provided at convenient local clinics, using artificial intelligence (AI) to significantly expand the quality of care and provide it at much lower cost. Section VI provides our view of what a system like that might look like. Section VII concludes this paper with a summary of the key points underlying our vision of digital health in the age of TIN.
II. THE INFINITE NETWORK
We believe the world is headed for a future in which all things and all people will be connected all the time forming an ever growing network that is unbounded or infinite in time and space. There will be an unbounded or infinite continuum of data that will be produced, communicated, aggregated, and analyzed continuously to make the right choices at the right times. It will become possible to do anything, anywhere, anytime. Old labels such as “Consumer” and “Office Worker” will become states of mind of a person at a particular moment or place. This is a vision of the future that we call “The Infinite Network” (see the illustration in Fig. 1) [Reference Balram1].

Fig. 1. The Infinite Network.
There are a number of major trends driving us toward this vision with the most important one being the dramatic expansion of capabilities of the mobile platform and ecosystem. As illustrated in Fig. 2 [Reference Balram and Biswas2], the smartphone platform has become a personal supercomputing, super-communication and super-sensory platform. This, combined with the ubiquitous availability of high-bandwidth connectivity from cellular and WiFi networks and huge storage density (approaching 1|Tbit/inReference Balram and Biswas 2 ), is enabling a vast array of new applications and functions at large scale that adaptively combine local information and intelligence with smart clouds (see, for example, [Reference Kelly III and Hamm3–Reference Mayer-Schonberger and Cukier5]). The mobile platform is itself a major driver of a range of component technologies such as smart sensing which can be used for other purposes as well.

Fig. 2. The smartphone as a supercomputing, super-sensory, super-communications platform [2].
The future enabled by these capabilities provides some significant opportunities and challenges for all of us; see for example [Reference Balram1,Reference Mayer-Schonberger and Cukier5–Reference Lanier8]. The opportunity that we are focused on in this paper is the promise of personalized and continuous health care that combines the benefits of large-scale aggregation (“n-of-billions”) and individual optimization (“n-of-1”); see for example [Reference Topol9,Reference Topol10].
III. SMART SENSING
At the micro or individual level, a vast array of new technologies and devices are becoming available to sense a diverse set of information that can provide much greater insight into the health of a person and enable better diagnostics and more effective treatment, pro-actively for preventive care and reactively for urgent care. This “smart sensing” can be divided into three categories by location – embedded into the body, worn on top of it, or used as needed at a point of care location. In this section, we describe some promising examples of each of these categories.
A) Smart embedded devices
A smart embedded device needs to be able to do the following things:
-
1. Sensing – sense/sample/measure the phenomena it is designed to focus on.
-
2. Computation – compute relevant useful metrics derived from the raw data and trigger actions or alerts as appropriate.
-
3. Communication – communicate relevant information at appropriate intervals to a master or receiving system that aggregates this information with other complementary ones.
In some applications, the device may include a mechanism to take some action inside the host, such as releasing a dose of medication.
The most important characteristic of these smart devices is to be able to function for very long, ideally indefinite, periods of time. Since sensing, processing, and communication all require power, being able to harvest energy and be self-powered is a very desirable goal. The most effective and common means of harvesting energy from solar or light in general is of course not applicable for embedded devices. Various means of harvesting small amounts of energy for embedded devices are being developed, including energy from movement, thermal differences, and radiofrequency (RF) signals (see, for example, [Reference Balram11–Reference Gudan, Chemishkian, Hull, Reynolds and Thomas13] for systems that harvest from WiFi). The specific energy harvesting method that is best for a particular application will depend on how deeply the sensing system is embedded – systems embedded just under the skin may use RF energy, while those embedded into organs may use kinetic or thermal energy.
Since energy harvesting for embedded devices can only provide small amounts of energy, the development of ultra-low-power circuits has been a key area of focus, using techniques like Sub-Threshold-Design (see, for example [14]). The MReference Kelly III and Hamm 3 (Michigan Micro Mote) [Reference Lee15] is an example of a very small form factor microsystem that combines energy harvesting, ultra-low power computation and sensing and wireless communication to demonstrate the feasibility of embedded smart sensors, such as a pressure sensor to track the progress of a tumor. See [16] for examples of future embedded biomedical sensors and subsystems.
The ongoing research into new smarter implantable medical devices is complementary to the above-mentioned ones for embedded smart sensors, see for example [17] for a range of new implantable devices under development, and an extension of traditional implanted devices.
B) Smart wearable devices
Smart wearables could be devices that are in the form of commonly used personal wearables such as watches, bracelets, rings or lockets, or integrated into clothing. Wearables that are for temporary use can take other forms such as patches or devices that are mounted on the body through straps, but anything that is meant to be worn on a regular basis would likely have to take the form of a wearable object that people are already used to having on their body for extended periods of time. The general health-related purposes of such wearables are for the monitoring and recording of various vital signs on an ongoing basis, to enable the wearer to be pro-active in the management of their health and wellness. A much bigger use case for the future is the integration of these continuous vital sign parameters into an intelligent healthcare network that can use this continuous record to do a much more effective diagnosis and treatment of a medical condition or emergency when one occurs. This is in contrast to the current healthcare system that relies on very discrete sets of information about a person when they arrive at a point of care for treatment of a medical condition. We will discuss in Section VI that achieving the promise of continuous care will require a very different type of healthcare network than the ones that exist today.
A prominent recent example of a “smart band” is the Simband digital health platform from Samsung, see [18]. This generalizes the popular traditional fitness tracker band such as Fitbit [19], by providing a platform with a smart watch and a set of sockets for customizable sensor modules. The functionality of this type of platform is significantly enhanced by making it part of a smart cloud network such as Samsung's SAMI [20], enabling the aggregation of data to provide deeper insights and predictive analytics.
A more traditional form factor for a wearable is a “smart watch”, with the most prominent current example being the Apple Watch [21]. This is a general purpose wearable computer that can provide a variety of functions including health and wellness related ones. This can connect to a cloud-based health information aggregation system such as HealthKit [22] that can collect information from all the user's iOS devices.
Garments with integrated sensors are already emerging as an interesting possibility, at least for certain applications and sets of people, whose vital signs need more regular monitoring. An early example of such a system is Vital-Jacket, which is a smart vest with integrated vital sign sensors that communicates to a remote-monitoring application via the wearer's Android Smartphone; see [Reference Cunha, Pereira, Xavier, Ferreira and Meireles23]. This was used to monitor the wellbeing of First Responders such as Firemen in the Vital Responder project; see [24]. The use of such smart garments for a variety of people needing continuous monitoring during their daily activities is a likely possibility in the near future.
Fragmentation remains a big problem for enabling true seamless collection and analysis of useful personal information with there being at least two big disconnected Internet-of-Things ecosystems – iOS and Android – at this time, so a user is forced to choose all her devices to be solely from one ecosystem for the best results; see for example [Reference Moore-Colyer25].
C) Smart medical devices
Smart medical devices refer to digitally enhanced versions of traditional medical sensing instruments used at a point-of-care location such as a clinic or hospital. In this paper, we will focus on imaging devices and in particular on medical cameras for imaging of various surface areas of the body and medical imaging for imaging of the interior elements of various parts of the body. In both cases, big improvements in capability are being pursued through better hardware (sensors and computational elements) and better software (signal processing and machine learning).
1) Smart medical cameras
A promising new direction for smart medical cameras is the use of Computational Optics technologies, or more specifically a subset called Plenoptic or Light Field Imaging; see for example [Reference Balram26]. The basic idea of plenoptic imaging is to insert an additional optical element called the Micro Lens Array in between the main lens and the image sensor. In combination with a set of pixels behind it, each micro lens in this array splits a cone of light emanating from a point in a scene into a set of directional bins. A plenoptic camera thus captures both spatial and angular information of the scene, instead of a purely spatial view captured by a traditional camera. A further expansion of capability comes from inserting a multi-spectral filter array in the aperture of the main lens, so that various spectral bands of interest can be captured simultaneously. The complex mixture of multi-spectral, angular and spatial data captured by the image sensor requires custom digital processing algorithms to extract different types of useful information. The basic architecture is illustrated in Fig. 3 [Reference Balram27].

Fig. 3. Basic architecture of multi-spectral light field (plenoptic) imaging [27].
This type of architecture can be used to create smart medical cameras for imaging externally accessible areas of the body such as the ear canal, skin and eyes, to capture new types of information and provide enhanced diagnostic capabilities, see for example [Reference Balram27,Reference Balram28]. The Light Field Otoscope (LFO) is a good example of this new category of smart medical cameras based on light field imaging; see for example [Reference Balram26].
In the USA, middle-ear infections are one of the leading cause of doctor's visits for children under the age of 7 and the most common reason for prescription of antibiotics, with one study estimating 25 million office visits with 20 million prescriptions of antibiotics at a cost of $2B a year [Reference Teele, Klein and Rosner29]. In the case of a possible infection, a medical practitioner views the child's tympanic membrane (TM) and tries to determine which of the possible conditions are present – acute otitis media (AOM), otitis media with effusion (OME) or no effusion (NOE). AOM is a bacterial infection requiring antibiotics, whereas OME is a sterile effusion that does not. However, determining which of these conditions is present is difficult, especially in the case of very young children [Reference Kuruvilla30,Reference Kuruvilla, Shaikh, Hoberman and Kovačević31]. Figure 4 shows example images of the three conditions [Reference Kuruvilla, Shaikh, Hoberman and Kovačević31]. A recent study shows that the two most important clinical features for differentiating between these conditions are: (1) bulging of the TM (i.e. changes in three-dimensional (3D) shape), and (2) changes in the color of TM [Reference Shaikh, Hoberman, Rockette and Kurs-Lasky32].

Fig. 4. Images of the TM for (a) AOM, (b) OME, and (c) NOE from [31].
Traditional optical and digital otoscopes are monocular 2D devices, so extracting 3D information from such devices is challenging and typically done by inference from light reflections and shading based on prior experience. Accurate color assessment of the TM is also plagued by subjective color perception differences. On the other hand, a multi-spectral light field imaging device (LFO) can measure the 3D shape of the TM and capture relevant spectral information, which might significantly enhance the accuracy of the diagnosis. Figure 5 shows a prototype of the world's first LFO, which is currently in trials at Children's Hospital Pittsburgh [Reference Bedard, Tošić, Meng, Hoberman, Kovačević and Berkner33], and the kind of depth image and 3D shape reconstruction that is possible using it. The subject performed a Valsalva maneuver (pinching one's nose while pressing air out) to induce a partial bulging of the TM, seen on the left under the malleus. The 3D reconstruction clearly shows the bulging shape of the TM in this area. A depth map of the TM was estimated from the light field using a method based on LISAD spaces [Reference Tosic and Berkner34]. The estimated depth map, along with the corresponding RGB data extracted from the light field, was used to plot the 3D surface of the subject's TM, as shown in Fig. 5(b).

Fig. 5. LFO: (a) Prototype, (b) 2D image, depth map, and 3D reconstruction of a human adult TM.
The LFO is an example of how light field imaging based medical cameras may in the near future be able to significantly improve the quality of diagnosis of many common medical conditions. Precise measurement of disease attributes and the possibility of following the progress of treatment could enable an overall improvement of patient care.
2) Smart medical imaging
One of the highest impact areas for exploration and future expansion in smart medical imaging is the development of tools and techniques to get a deeper understanding of the human brain. In spring 2013, President Obama announced the BRAIN initiative – Brain Research through Advancing Innovative Neurotechnologies, and in response Carnegie Mellon University formed the Brain Hub initiative [35].
As part of this large-scale initiative, CMU researchers are working on mapping the functions of different areas of the brain. This requires the development of much higher spatial and temporal resolution (millimeters and milliseconds respectively) non-invasive sensing mechanisms for electroencephalograms (EEG) and magnetoencephalograms (MEG) and new signal processing and machine learning techniques to extract deep insights from the data. The acquisition and processing of higher spatial and temporal resolution data could enable better understanding and improved treatment of neurological disorders such as Alzheimer's, Epilepsy and Autism; see for example [Reference Dinstein, Heeger, Lorenzi, Minshew, Malach and Behrmann36].
Essentially, the future objectives on the medical device side is to create “Big Medical Data” that can be processed with the increasingly powerful machine-learning techniques that are being developed to analyze traditional Big Data pouring in from social networks and the Internet-of-Things.
IV. TRADITIONAL TELEMEDICINE NETWORKS
Telemedicine refers to the use of telecommunication and information technologies to provide clinical health care at a distance [37]. This was traditionally developed and deployed in order to provide medical care for patients in rural areas where local practitioners were not available. Telemedicine can be broken into three main categories: store-and-forward, remote monitoring and (real-time) interactive systems [37]. Store-and-forward primarily focuses on offline interpretation of patient information, a typical example being radiology. Remote monitoring is primarily used for monitoring of patients with chronic conditions such as heart disease or diabetes. Real-time interaction is the most common form of telemedicine and is used to replace the traditional face-to-face engagement that happens in a clinic or hospital, because the patient and doctor are separated geographically.
In this paper, we are primarily focused on interactive telemedicine. We start with a discussion of traditional telemedicine networks in this section. In Section V, we discuss the next generation of cloud-based telehealth networks that are being deployed now. Finally, in Section VI, we explore future generations of telehealth networks that will use AI to expand the scale, quality, and continuity of care, while lowering cost.
Traditional telemedicine networks were developed to serve regions where there was a significant shortage of doctors, for example, regions that had a small number of urban areas where medical professionals were concentrated and large rural areas where they were not. This type of situation exists in both developed and emerging countries. In the USA, a good example of a region that developed telemedicine to provide better healthcare to its population is Arizona, which developed the Arizona Telemedicine Program (ATP) starting in 1995, to deliver care across the state, see [38]. Figure 6 shows the extensive reach that the Arizona Telemedicine Network (ATN) has achieved. The ATP provides primary care and an extensive set of specialty care using telemedicine. The network is in the form of a traditional hub and satellite model, with a small number of hubs in major urban areas (such as Phoenix) and a large number of satellite clinics located all over the state where needed.

Fig. 6. Arizona Telemedicine Network [38].
Large-scale telemedicine is needed in many emerging countries but there are few examples of even modest scale. One of the big barriers to scale in emerging countries has been lack of widespread connectivity, but this is being addressed now because of the large-scale build-out of cellular networks, with 2G being pervasive across the developing world and 3G seeing significant penetration in countries such as India. Cost and infrastructure challenges remain a barrier to creating the breadth of telemedicine offerings of a network like ATN. However, there are non-governmental organizations such as World Health Partners (WHP) that have achieved significant scale in certain regions in India by adopting a simple focused model of care – providing primary care and targeting specific problems such as diarrhea and pneumonia among children and maternal and reproductive health for women – and by keeping costs low through leverage of the mainstream mobile platform and cellular network [39]. WHP operates a simple telemedicine network based on a hierarchical franchise model; see Fig. 7 [39]. Patients in rural areas are engaged by village-level franchises operating small SkyCare clinics and those that need a video consultation with a doctor are referred to local telemedicine clinics called SkyHealth Centers, which connect via cellular network to a Central Medical Facility where WHP hosts a panel of medical doctors.

Fig. 7. WHP Franchise Network Service Delivery [39].
V. ADVANCED TELEHEALTH NETWORKS
In 2011, as part of developing TIN vision, we came to the realization that in the future healthcare would be delivered to most people across the world through advanced telehealth networks. To fulfill that vision, we set out to develop a telehealth network that could deliver a broad range of services to people in a robust, scalable, secure, and easy-to-use manner and could be deployed in many types of locations in both developing and developed countries; see [Reference Balram27,Reference Balram28]. Figure 8 shows the basic concept of the system that connects remote patients being treated at small local clinics (“Nodes”) to any location where a doctor is operating from (“Hub”) using the cloud for all the Audio Visual (AV) and medical data communication and storage. In an emerging country like India, the local clinic or node uses a specially designed cart that collects all the AV, computing, communications, and medical devices in a simple robust easy to move system; see Fig. 9(a) [Reference Balram27,Reference Balram28]. In a developed country such as the USA, where the local clinic is likely to be part of a retail pharmacy, or in other high traffic locations like a community center or part of a large corporate site, the cart is replaced by a specially designed kiosk or pod that contains similar functionality with the higher level of patient privacy required in these countries; see Fig. 9(b) [Reference Balram28].

Fig. 8. Advanced Telehealth Network comprising Node, Hub, and Cloud [27,28].

Fig. 9. System at local clinic (node): (a) Cart for India, (b) Kiosk (Pod) for USA [28].
To enable the above-mentioned objectives, the system has a multi-tier service-oriented architecture (see Fig. 10) with:
-
(a) A base platform (bottom) layer that contains a set of core services that would be required from the system in all its deployments – the most important of these being secure storage and transfer of data. These are accessed through loosely coupled REST APIs.
-
(b) An application and services platform (middle) layer that contains an electronic medical record (EMR), a security framework that guarantees patient privacy through token-based authentication and NIST-approved encryption algorithms for security of data in motion and at rest, a rules-based appointment scheduler that connects patients to providers in time and space, a medical streamer that guarantees real-time delivery of audio/video/text streams, and an analytics engine.
-
(c) A solutions (top) layer that implements clinical pathways built on top of the APIs exported by the platform. This layer comprises clinical workflows (such as patient registration, consultation scheduling, tele-consultation, store-and-forward reporting), an EMR front-end, an Enterprise Service Bus and User Interface (UI). The EMR is constructed in the form of a “problem oriented medical record” that captures and presents information in a manner that is intuitive to the healthcare personnel using the system. The Enterprise Service Bus decouples the medical devices from the EMR layer, and allows for vendor neutral integration of medical devices as well as enabling quick integration with a wide array of device types.

Fig. 10. Multi-tier service oriented software architecture of advanced telehealth system [27,28].
The solutions layer is easily customized to provide unique UIs for each deployment and new clinical modules can be easily added to it. This includes a home health module for future expansion into home monitoring for chronic disease management.
On the client or node side, the system is accessed on a PC using a browser operating in kiosk mode. On the hub side, the system can be accessed via browser on any PC, smartphone or tablet that the medical care provider or doctor chooses to use.
This system is currently deployed in pilots in India as of 2015 (see [Reference Balram27,Reference Balram28]), and will be starting pilots in other countries in the near future.
In the last few years, there has been an explosive growth in mHealth, using the smartphone, smart sensors/wearables (such as those discussed in Section III) and a wide variety of apps to provide various types of health and wellness-related information and services (see https://en.wikipedia.org/wiki/MHealth, http://www.who.int/goe/publications/goe_mhealth_web.pdf)). This type of consumerized crowd-sourced continuous healthcare can play an important role in enabling people to take greater ownership of their own wellness but is currently rather ad hoc, fragmented and mostly disconnected from the care provided by traditional professional healthcare providers. In our view, there are three levels of care that need to be closely integrated to provide seamless continuity and quality of care – home/personal care for wellness and chronic disease management, clinic care for minor acute care, and hospitals for major acute care and surgical procedures, with the clinic playing the critical role of intermediary. In the next section, we discuss our vision of intelligent telehealth networks that can provide the necessary integration of care across these.
VI. INTELLIGENT TELEHEALTH NETWORKS
The traditional and advanced telehealth systems that have been discussed in the previous sections are able to provide care to reasonably large numbers of people. However, there are at least three future shortcomings of these types of systems that need to be addressed in the long-term:
-
• Integration of big personal medical data: The projected future use of smart sensing that is embedded or worn will create a huge amount of big personal medical data. In the current system of healthcare, diagnosis is done on the basis of relatively small amounts of data gathered at discrete points of time (through point-of-care assessment or tests) and it is very difficult to envision how the large amount of continuous personal data that will become available through smart sensing can be integrated into the care provided by a medical practitioner.
-
• Availability of sufficient numbers of doctors: The major push for telemedicine so far has been on the demand side, trying to create a sufficiently large flow of patients to make these systems financially viable. Telemedicine solves the problem of distribution of medical practitioners, where this does not match the distribution of the patient population. But it does not solve the shortage problem in developed countries (see, e.g. [Reference Krupa40–42]) that refer to projected shortages for primary care in countries such as the USA and Germany. In the developing world, the shortage is compounded by a quality problem with the quality of qualified doctors varying greatly depending on the rigor of the medical institution that they were trained at (see for example [Reference Joelving43,Reference Das, Holla, Das, Mohanan, Tabak and Chan44]). It does not seem likely that there will be sufficient number of doctors to provide care when there are very large-scale telehealth networks in operation.
-
• Cost of care: In our experience, the cost of a typical telemedicine consultation for non-acute care is on the order of $50–70 in a developed country like the USA and $5–7 in an emerging country like India (which on a purchasing-power-parity basis is about one-third of the US cost). If telehealth is intended to provide care to the entire population of a nation, then the operating cost will need to be reduced significantly. In our experience, the cost of the doctor is the largest single component of the operating cost of a telehealth system, ranging from 30 to 60% of the total cost of a consultation. The use of a lesser qualified medical provider like a nurse practitioner reduces the cost, but it still remains the single most expensive component of the consultation.
The solution to all three future problems lies in the use of AI to create hybrid networks that combine the use of “virtual doctors” for routine care with human intervention for the handling of exceptions. Figure 11 shows how the basic triage between AI and human intervention might work in the case of a telehealth clinic (“node”) such as the ones described in the previous section. If even half of the patient flow going to a doctor could be handled entirely by the virtual doctor, the system would have double the capacity and 15–30% less cost per consultation. A virtual doctor can easily assimilate large-scale personal health data into its database in addition to the data gathered at the point of care, but a model for properly qualifying the reliability of different forms and sources of personal health data will need to be developed. In the exception cases where the judgment of a human doctor is required, the assistance of the virtual doctor will enable her to make a better diagnosis.
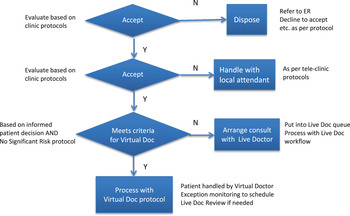
Fig. 11. Basic triage at tele-clinic for selection of remote health care provider (AI or human).
Table 1 shows the Performance, Environment, Actuators, Sensors (PEAS) model [Reference Norvig and Russell45] for the Virtual Doctor intelligent agent. Figure 12 shows the block diagram of how a Virtual Doctor AI system for this hybrid telehealth system might be constructed, using a basic architecture of a rules-based system with a learning component (see https://en.wikipedia.org/wiki/Expert_system; https://en.wikipedia.org/wiki/Knowledge-based_systems; https://en.wikipedia.org/wiki/Forward_chaining; https://en.wikipedia.org/wiki/Knowledge_representation_and_reasoning; https://en.wikipedia.org/wiki/Machine_learning) for basic information on the elements of this type of system. An example of a rules-based expert system with manual learning/knowledge-entry is given in [Reference Balram, Birmingham, Brady, Tremain and Siewiorek46] and an example of state-of-the-art contextual reasoning applied to enhancing patient health is given in [Reference Schmidtke, Yu, Masomo, Kinai, Shema, Brezillon and Gonzalez47]. The type of clinical workflow rules that would need to be incorporated in the Virtual Doctor system are given for example in [Reference Mahadevan and Garmel48].

Fig. 12. Virtual doctor AI system.
Table 1. PEAS description of task environment for virtual doctor system.

The major elements of this system are:
-
• A cloud-based telemedicine system like the one described in the previous section.
-
• A triage procedure at the tele-clinic that uses the local attendant and software to determine if the patient needs to be seen by a human doctor or can be satisfactorily served by the AI system – the triage process is done at the start and throughout the consultation to ensure that the patient is handled appropriately, with several exit options to review by a human doctor if warranted.
-
• An AI system of the type well known in the art as an “expert” or “knowledge-based system” comprising of a knowledge-base, an inference engine, input module, output module, central/monitoring module, and learning module. There are various options possible in the construction of these modules for different scenarios.
-
• Remote control or operations center where the operation of the “virtual doctors” can be monitored by a set of human doctors who can choose to intervene if/when necessary –such centers could be large control/operation centers as well known in the IT industry or distributed ones with individual doctors operating in their own locations with a set of consultations being shown/shared with them on an individual dashboard.
The same principles of leverage using triage with AI could be applied to other types of medical professionals like nurse practitioners/physicians assistants – i.e. expanding “virtual doctor” to “virtual healthcare professional”.
The core system is a typical “expert” or “knowledge-based” system based on Generalized Modus Ponens known in the art (see, e.g. [Reference Norvig and Russell45,Reference Balram, Birmingham, Brady, Tremain and Siewiorek46]) comprising of:
-
1. Knowledge Base (KB) – flat or hierarchical object based.
-
2. Rules-based Inference Engine (IE) using forward chaining with performance improvement options such as use of magic sets.
-
3. Input module with some or all of the following:
-
i. Keyboard, mouse, and touch panel
-
ii. Real-time medical data from devices in the clinic.
-
iii. Data from patient EMR and PHR (Personal Health Record).
-
iv. Live voice input from patient and attendant with speech recognition module.
-
-
4. Control/main/monitoring module with some or all of the following:
-
i. Overall control function for I/O and processing.
-
ii. Operator UI/console.
-
-
5. Output module with some or all of the following:
-
i. Speech Synthesis with options for age, gender, and accent to maximize patient comfort.
-
ii. Computer-generated Avatar of Virtual Doctor with options for age/gender/ethnicity.
-
iii. Playback of images and video from library or medical record.
-
iv. Closed caption text to match speech output.
-
v. Data to patient EMR and PHR.
-
-
6. Knowledge Acquisition (Learning) module with some or all of the following:
-
i. Machine learning modules to assess performance of KB and IE and improve their performance – these can be chosen from wide variety of well-known techniques such as given in ([Reference Norvig and Russell45] and https://en.wikipedia.org/wiki/Machine_learning).
-
ii. Module for manual inputs from experts enabling direct addition or edit of KB and IE.
-
iii. Training protocols that can be run in conjunction with test patients to measure the performance of the system.
-
An intelligent telehealth network like this does not exist today, but the individual technology components needed to implement it are becoming available.
VII. CONCLUSIONS
The exponential growth in digital technology is leading us to a future in which all things and all people will be connected all the time – a vision that we refer to as “TIN” [Reference Balram1]. TIN will change all major industries in fundamental ways. In this paper, we focused on the impact it will have on healthcare, in particular on the big changes in the way that each person's health will be managed throughout their lives due to the availability of continuous communication, immense compute power, large-scale local storage, and the transition to a big-data-driven approach.
TIN changes the essence of healthcare to something that occurs continuously as the management of a person's health at all times rather than the traditional form of discrete management of minor and major problems at the times they occur. The changes in individual health care/management can be looked at from a Micro (individual) and Macro (aggregate) point of view.
At the Micro level, one of the key drivers will be the use of smart sensing to gather useful information that can be used for holistic care – maintaining emotional and physical wellness, arriving at early and accurate diagnosis and providing timely and effective treatment. We described Smart Sensing, breaking it down into three categories – sensing inside the body (“embedded sensors”), sensing on the outside of the body (“wearable sensors”) and sensing by smart medical devices.
At the Macro level, the key direction is the development of Intelligent Telehealth Networks that can deliver individualized and continuous care to large populations using the advanced digital technologies that are arriving as part of TIN. There is a long history of telemedicine being used to deliver remote care in areas where local availability of doctors is an issue. Traditional networks have achieved a certain amount of scale in regions where the conditions and economics are favorable but have not achieved large scale across big regions due to a number of factors. New advanced telehealth networks that are emerging now have the potential to achieve large scale across much larger regions by using state-of-the-art information and communication technology structures and approach. Future networks that incorporate AI will drastically expand the continuity and quality of care and at much lower cost. We described all of these kinds of networks from the traditional to the future, ending with the long-term vision of an intelligent hybrid telehealth network that incorporates AI to enable continuous, high-quality, low-cost care for all the people on the planet.
ACKNOWLEDGEMENTS
We would like to thank our colleagues and collaborators at Ricoh Innovations Corporation (RIC) and its Indian subsidiary, Ricoh Innovations Private Limited (RIPL) – in particular, Dr. Kathrin Berkner, Dr. Noah Bedard, and Dr. Lingfei Meng from RIC and Vipin Namboodiri from RIPL. We would also like to thank Dr. S. V. Mahadevan, Chairman of Emergency Medicine, Stanford University School of Medicine, for his advice and guidance. All trademarks identified herein are the property of their respective owners.
Nikhil Balram is the President and CEO of Ricoh Innovations Corporation (ric.ricoh.com), a Silicon Valley company that creates global new businesses based on advanced information technology for Ricoh Company Ltd. He has over 20 years of industry experience and has been an officer of five publicly listed companies. He has won numerous awards including a 2012 Gold Stevie® Award for Executive of the Year in the Electronics category in The 9th Annual International Business Awards, a 2012 Fellow Award by the Society for Information Display (SID) and the 2011 Alumni Achievement Award by Carnegie Mellon University. He has over 100 US and international patents granted or pending, more than 40 technical publications, including two invited book chapters, and has given over 25 keynote speeches at major conferences and events worldwide. He received his B.S., M.S., and Ph.D. degrees in Electrical Engineering from Carnegie Mellon University.
Ivana Tošić leads the Computational optics and visual processing group at Ricoh Innovations, Corp., Cupertino, California. She received a Ph.D. degree in Computer and Communication Sciences from the Swiss Federal Institute of Technology (EPFL), Switzerland in 2009, and a Dipl.Ing. degree in Telecommunications from the University of Niš, Serbia in 2003. From 2009 to 2011, she was a postdoctoral researcher at the Redwood Center for Theoretical Neuroscience, the University of California at Berkeley, where she studied computational mechanisms of depth perception from binocular vision. Her research interests include computational imaging, medical imaging, multi-view/3D image processing, binocular vision, scene understanding, augmented reality, sparse approximations, and machine learning.
Harsha Binnamangalam handles business development and marketing for Ricoh Innovations’ healthcare portfolio, including healthcare software-as-a-service and medical imaging devices. He has worked for over 10 years in the technology industry in various capacities, starting as an engineer in the medical imaging space, to managing specialized semiconductor products used in cutting edge networking equipment. He received his B.S. in EE from Visvesvaraya Technological University and his MBA from Indian Institute of Management, Kozhikode.