Prior to the COVID-19 pandemic, debate mounted concerning the wisdom, and perhaps the inevitability, of moving swaths of the court system online. These debates took place within a larger discussion of how and why courts had failed to perform their most basic function of delivering (approximately) legally accurate adjudicatory outputs in cases involving unrepresented litigantsFootnote 1 while inspiring public confidence in them as a method of resolving disputes compared to extralegal and even violent alternatives. Richard Susskind, a longtime critic of the status quo, summarized objections to a nearly 100 percent in-person adjudicatory structure: “[T]he resolution of civil disputes invariably takes too long [and] costs too much, and the process is unintelligible to ordinary people.”Footnote 2 As Susskind noted, fiscal concerns drove much of the interest in online courts during this time, while reformists sought to use the move online as an opportunity to expand the access of, and services available to, unrepresented litigants.
Yet as criticisms grew, and despite aggressive action by some courts abroad,Footnote 3 judicial systems in the United States moved incrementally. State courts, for example, began to implement online dispute resolution (ODR), a tool that private-sector online retailers had long used.Footnote 4 But courts had deployed ODR only for specified case types and only at specified stages of civil proceedings. There was little judicial appetite in the United States for a wholesale transition.
The COVID-19 pandemic upended the judicial desire to move slowly. As was true of so many other sectors of United States society, the judicial system had to reconstitute online, and had to do so convulsively. And court systems did so, in the fashion in which systemic change often occurs in the United States: haphazardly, unevenly, and with many hiccups. The most colorful among the hiccups entered the public consciousness: audible toilet flushes during oral argument before the highest court in the land; a lawyer appearing at a hearing with a feline video filter that he was unable to remove himself; jurors taking personal calls during voir dire.Footnote 5 But there were notable successes as well. The Texas state courts had reportedly held 1.1 million proceedings online as of late February 2021, and the Michigan state courts’ live-streaming on YouTube attracted 60,000 subscribers.Footnote 6
These developments suggest that online proceedings have become a part of the United States justice system for the foreseeable future. But skepticism has remained about online migration of some components of the judicial function, particularly around litigation’s most climactic stage: the adversarial evidentiary hearing, especially the jury trial. Perhaps believing that they possessed unusual skill in discerning truthful and accurate testimony from its opposite,Footnote 7 some judges embraced online bench fact-finding. But the use of juries, both civil and criminal, caused angst, and some lawyers and judges remained skeptical that the justice system could or should implement any kind of online jury trial. Some of this skepticism waxed philosophical. Legal thinkers pondered, for example, whether a jury trial is a jury trial if parties, lawyers, judges, and the jurors themselves do not assemble in person in a public space.Footnote 8 Some of the skepticism about online trials may have been simple fear of the unknown.Footnote 9
Other concerns skewed more practical and focused on matters for which existing research provided few answers. Will remote trials yield more representative juries by opening proceedings to those with challenges in attending in-person events, or will the online migration yield less-representative juries by marginalizing those on the wrong side of the digital divide? Will remote trials decrease lawyer costs and, perhaps as a result, expand access to justice by putting legal services more within the reach of those with civil justice needs?Footnote 10 Time, and more data, can tell if the political will is there to find out.
But two further questions lie at the core of debate about whether online proceedings can produce approximately accurate and publicly acceptable fact-finding from testimonial evidence. The first is whether video hearings diminish the ability of fact-finders to detect witness deception or mistake. The second is whether video as a communication medium dehumanizes one or both parties to a case, causing less humane decision-making.Footnote 11 For these questions, the research provides an answer (for the first question) and extremely useful guidance (for the second).
This chapter addresses these questions by reviewing the relevant research. By way of preview, our canvass of the relevant literatures suggests that both concerns articulated above are likely misplaced. Although there is reason to be cautious and to include strong evaluation with any move to online fact-finding, including jury trials, available research suggests that remote hearings will neither alter the fact-finder’s (nearly non-existent) ability to discern truthful from deceptive or mistaken testimony nor materially affect fact-finder perception of parties or their humanity. On the first point, a well-developed body of research concerning the ability of humans to detect when a speaker is lying or mistaken shows a consensus that human detection accuracy is only slightly better than a coin flip. Most importantly, the same well-developed body of research demonstrates that such accuracy is the same regardless of whether the interaction is in-person or virtual (so long as the interaction does not consist solely of a visual exchange unaccompanied by sound, in which case accuracy worsens). On the second point, the most credible studies from the most analogous situations suggest little or no effect on human decisions when interactions are held via videoconference as opposed to in person. The evidence on the first point is stronger than that on the second, but the key recognition is that for both points, the weight of the evidence is contrary to the concerns that lawyers and judges have expressed, suggesting that the Bench and the Bar should pursue online courts (coupled with credible evaluation) to see if they offer some of the benefits their proponents have identified. After reviewing the relevant literature, this chapter concludes with a brief discussion of a research agenda to investigate the sustainability of remote civil justice.
A final point concerning the scope of this chapter: As noted above, judges and (by necessity) lawyers have largely come to terms with online bench fact-finding based on testimonial evidence, reserving most of their skepticism for online jury trials. But as we relate below, the available research demonstrates that to the extent that the legal profession harbors concerns regarding truth-detection and dehumanization in the jury’s testimonial fact-finding, it should be equally skeptical regarding that of the bench. The evidence in this area either fails to support or affirmatively contradicts the belief that judges are better truth-detectors, or are less prone to dehumanization, than laity. Thus, we focus portions of this chapter on online jury trials because unwarranted skepticism has prevented such adjudications from the level of use (under careful monitoring and evaluation) that the currently jammed court system likely needs. But if current research (or our analysis of it here) is wrong, meaning that truth-detection and dehumanization are fatal concerns for online jury trials, then online bench adjudications based on testimonial evidence should be equally concerning.
4.1 Adjudication of Testimonial Accuracy
A common argument against the sustainability of video testimonial hearings is that, to perform its function, the fact-finder must be able to distinguish accurate from inaccurate testimony. Inaccurate testimony could arise via two mechanisms: deceptive witnesses, that is, those who attempt to mislead the trier of fact through deliberate or knowing misstatements; and mistaken witnesses, that is, those who believe their testimony even though their perception of historical fact was wrong.Footnote 12 The legal system reasons as follows. First, juries are good, better even than judges, at choosing which of two or more witnesses describing incompatible versions of historical fact is testifying accurately.Footnote 13 Second, this kind of “demeanor evidence” takes the form of nonverbal cues observable during testimony.Footnote 14 Third, juries adjudicate credibility best if they can observe witnesses’ demeanor in person.Footnote 15
Each component of this reasoning is false.
First, research shows that humans have just above a fifty-fifty chance ability to detect lies, approximately 54 percent overall, if we round up. For example, Bond and DePaulo, in their meta-analysis of deception detection studies, place human ability to detect deception at 53.98 percent, or just above chance.Footnote 16 Humans are really bad at detecting deception. That probably comes as a shock to many of us. We are pretty sure, for example, we can tell when an opponent in a game or a sport is fibbing or bending the truth to get an edge. We are wrong. It has been settled in science since the 1920s that human beings are bad at detecting deception.Footnote 17 The fact that judges and lawyers continue to believe otherwise is a statement of the disdain in which the legal profession holds credible evidence and empiricism more generally.Footnote 18
Second, human (in)ability to detect deception does not change with an in-person or a virtual interaction. Or at least, there is no evidence that there is a difference between in-person and virtual on this score, and a fair amount of evidence that there is no difference. Most likely, humans are really bad at detecting deception regardless of the means of communication. This also probably comes as a shock to many of us. In addition to believing (incorrectly) that we can tell when kids or partners or opponents are lying, we think face-to-face confrontation matters. Many judges and lawyers so believe. They are wrong.
Why are humans so bad at deception detection? One reason is that people rely on what they think are nonverbal cues. For example, many think fidgeting, increased arm and leg movement, and decreased eye contact are indicative of lying. None are. While there might be some verbal cues that could be reliable for detecting lies, the vast majority of nonverbal cues (including those just mentioned, and most others upon which we humans tend to rely) are unreliable, and the few cues that might be modestly reliable can be counterintuitive.Footnote 19 Furthermore, because we hold inaccurate beliefs about what is and is not reliable, it is difficult for us to disregard the unreliable cues.Footnote 20 In a study that educated some participants on somewhat reliable nonverbal cues to look for, with other participants not getting this information, participants with the reliable cues had no greater ability to detect lying.Footnote 21 We humans are not just bad at lie detection; we are also bad at being trained at lie detection.
While a dishonest demeanor elevates suspicion, it has little-to-no relation to actual deception.Footnote 22 Similarly, a perceived honest demeanor is not reliably associated with actual honesty.Footnote 23 That is where the (ir)relevance of the medium of communication matters. If demeanor is an unreliable indicator for either honesty or dishonesty, then a fact-finder suffers little from losing whatever the supposedly superior opportunity to observe demeanor an in-person interaction might provide.Footnote 24 For example, a study from 2015 shows that people attempting to evaluate deception performed better when the interaction was a computer-mediated (text-based communication) rather than in-person communication.Footnote 25 At least one possible explanation for this finding is the unavailability of distracting and unreliable nonverbal cues.Footnote 26
Despite popular belief in the efficacy of discerning people’s honesty based on their demeanor, research shows that non-demeanor cues, meaning verbal cues, are more promising. A meta-analysis concluded that cues that showed promise at signaling deception tended to be verbal (content of what is said) and paraverbal (how it is spoken), not visual.Footnote 27 But verbal and paraverbal cues are just as observable from a video feed.
If we eliminate visual cues for fact-finders, and just give them audio feed, will that improve a jury’s ability to detect deception? Unlikely. Audio-only detection accuracy does not differ significantly from audiovisual.Footnote 28 At this point, that should not be a surprise, considering the general low ceiling of deception detection accuracy – just above the fifty-fifty level. Only in high-pressure situations is it worthwhile (in a deception detection sense) to remove nonverbal cues.Footnote 29 To clarify: High-pressure situations likely make audio only better than audio plus visual, not the reverse. The problem for deception detection appears to be that, with respect to visual cues, the pressure turns the screws both on someone who is motivated to be believed but is actually lying and on someone who is being honest but feels as though they are not believed.
We should not think individual judges have any better ability to detect deception than a jury. Notwithstanding many judges’ self-professed ability to detect lying, the science that humans are poor deception detectors has no caveat for the black robe. There is no evidence that any profession is better at deception detection, and a great deal of evidence to the contrary. For example, those whose professions ask them to detect lies (such as police officers) cite the same erroneous cues regarding deception.Footnote 30 More broadly, two meta-analyses from 2006 show that purported “experts” at deception detection are no better at lie detection than nonexperts.Footnote 31
What about individuals versus groups? A 2015 study did find consistently that groups performed better at detecting lies,Footnote 32 a result the researchers attributed to group synergy – that is, that individuals were able to benefit from others’ thoughts.Footnote 33 So, juries are better than judges at deception detection, right? Alas, almost certainly not. The problem is that only certain kinds of groups are better than individuals. In particular, groups of individuals who were familiar with one another before they were assigned a deception detection task outperformed both individuals and groups whose members had no preexisting connection.Footnote 34 Groups whose members had no preexisting connection were no better at detecting deception than individuals.Footnote 35 Juries are, by design, composed of a cross-section of the community, which almost always means that jurors are unfamiliar with one another before trial.Footnote 36
There is more bad news. Bias and stereotypes affect our ability to flush out a lie. Females are labeled as liars significantly more than males even when both groups lie or tell the truth the same amount.Footnote 37 White respondents asked to detect lies were significantly faster to select the “liar” box for black speakers than white speakers.Footnote 38
All of this is troubling, and likely challenges fundamental assumptions of our justice system. For the purposes of this chapter, however, it is enough to demonstrate that human inability to detect lying remains constant whether testimony is received in-person or remotely. Again, the science on this point goes back decades, and it is also recent. Studies conducted in 2014Footnote 39 and 2015Footnote 40 agreed that audiovisual and audio-only mediums were not different in accuracy detection. The science suggests that the medium of communication – in-person, video, or telephonic – has little if any relevant impact on the ability of judges or juries to tell truths from lies.Footnote 41
The statements above regarding human (in)ability to detect deception apply equally to human (in)ability to detect mistakes, including the fact that scientists have long known that we are poor mistake detectors. Thirty years ago, Wellborn collected and summarized the then-available studies, most focusing on eyewitness testimony. Addressing jury ability to distinguish mistaken from accurate witness testimony, Wellborn concluded that “the capacity of triers [of fact] to appraise witness accuracy appears to be worse than their ability to discern dishonesty.”Footnote 42 Particularly relevant for our purposes, Wellborn further concluded that “neither verbal nor nonverbal cues are effectively employed” to detect testimonial mistakes.Footnote 43 If neither verbal nor nonverbal cues matter in detecting mistakes, then there will likely be little lost by the online environment’s suppression of nonverbal cues.
The research in the last thirty years reinforces Wellborn’s conclusions. Human inability to detect mistaken testimony in real-world situations is such a settled principle that researchers no longer investigate it, focusing instead on investigating other matters, such as the potentially distorting effects of feedback given to eyewitnesses,Footnote 44 whether witness age affects likelihood of fact-finder belief,Footnote 45 and whether fact-finders understand the circumstances mitigating the level of unreliability of eyewitness testimony.Footnote 46 The most recent, comprehensive writing we could find on the subject was a 2007 chapter from Boyce, Beaudry, and Lindsay, which depressingly concluded (1) fact-finders believe eyewitnesses, (2) fact-finders are not able to distinguish between accurate and inaccurate eyewitnesses, and (3) fact-finders base their beliefs of witness accuracy on factors that have little relationship to accuracy.Footnote 47 This review led us to a 1998 study of child witnesses that found (again) no difference in a fact-finder’s capacity to distinguish accurate from mistaken testimony as between video versus in-person interaction.Footnote 48
In short, decades of research provide strong reason to question whether fact-finders can distinguish accurate from inaccurate testimony, but also strong reason to believe that no difference exists on this score between in-person versus online hearings, nor between judges and juries.
4.2 The Absence of a Dehumanization Effect
Criminal defense attorneys have raised concerns of dehumanization of defendants, arguing that in remote trials, triers of fact will have less compassion for defendants and will be more willing to impose harsher punishments.Footnote 49 In civil trials, this concern could extend to either party. For example, in a personal injury case, the concern might be that fact-finders would be less willing to award damages because they are unable to connect with or relate to a plaintiff’s injuries. Or, in less protracted but nevertheless high-stakes civil actions, such as landlord/tenant matters, a trier of fact (usually a judge) might feel less sympathy for a struggling tenant and therefore show greater willingness to evict rather that mediate a settlement.
A review of relevant literature suggests that this concern is likely misplaced. While the number of studies directly investigating the possibility of online hearings is limited, analogous research from other fields is available. We focus on studies in which a decision-maker is called upon to render a judgment or decision that affects the livelihood of an individual after some interaction with that individual, much like a juror or judge is called upon to render a decision that affects the livelihood of a litigant. We highlight findings from a review of both legal and analogous nonlegal studies, and we emphasize study quality – that is, we prioritize randomized before nonrandomized trials, field over lab/simulated experiments, and studies involving actual decision-making over studies involving precursors to decisions (e.g., ratings or impressions).Footnote 50 With this ordering of pertinence, we systematically rank the literature into three tiers, from most to least robust: first, randomized field studies (involving decisions and precursors); second, randomized lab studies (involving decisions and precursors); and third, non-randomized studies. Table 4.1 provides a visual of our proposed hierarchy.
Table 4.1 A hierarchy of study designs
Tier | Randomized? | Setting | Example |
---|---|---|---|
1: RCTs | Yes | Field | Cuevas et al. |
2: Lab Studies | Yes | Lab | Lee et al. |
3: Observational Studies | No | Field | Walsh & Walsh |
According to research in the first tier of randomized field studies – the most telling for probing the potential for online fact-finding based on testimonial evidence – proceeding via videoconference likely will not adversely affect the perceptions of triers of facts on the humanity of trial participants. We do take note of the findings of studies beyond this first tier, which include some from the legal field. Findings in these less credible tiers are varied and inconclusive.
The research addressing dehumanization is less definitive than that addressing deception and mistake detection. So, while we suggest that jurisdictions consider proceeding with online trials and other innovative ways of addressing both the current crises of frozen court systems and the future crises of docket challenges, we recommend investigation and evaluation of such efforts through randomized control trials (RCTs).
4.2.1 Who Would Be Dehumanized?
At the outset, we note a problem common to all of the studies we found, in all tiers: none of the examined situations are structurally identical to a fact-finding based on an adversarial hearing. In legal fact-finding in an adversarial system, one or more theoretically disinterested observers make a consequential decision regarding the actions of someone with whom they may have no direct interaction and who, in fact, sometimes exercises a right not to speak during the proceeding. In all of the studies we were able to find, which concerned situations such as doctor-patient or employer-applicant, the decision-maker interacted directly with the subject of the interaction. It is thus questionable whether any study yet conducted provides ideal insight regarding the likely effects of online as opposed to in-person for, say, civil or criminal jury trials.
Put another way: If a jury were likely to dehumanize or discount someone, why should it be that it would dehumanize any specific party, as opposed to the individuals with whom the jury “interacts” (“listens to” would be a more accurate phrase), namely, witnesses and lawyers? With this in mind, it is not clear which way concerns of dehumanization cut. At present, one defensible view is that there is no evidence either way regarding dehumanization of parties in an online jury trial versus an in-person one, and that similar concerns might be present for some types of judicial hearings.
Some might respond that the gut instincts of some criminal defense attorneys and some judges should count as evidence.Footnote 51 We disagree that the gut instincts of any human beings, professionals or otherwise, constitute evidence in almost any setting. But we are especially suspicious of gut instincts in the fact-finding context. As we saw in the previous part, fact-finding based on testimonial hearings has given rise to some of the most stubbornly persistent, and farcically outlandish, myths to which lawyers and judges cling. The fact that lawyers and judges continue to espouse this kind of flat-eartherism suggests careful interrogation of professional gut instincts on the subject of dehumanization from an online environment.
4.2.2 Promising Results from Randomized Field Studies
Within our first-tier category of randomized field studies, the literature indicates that using videoconference in lieu of face-to-face interaction has an insignificant, or even a positive, effect on a decision-maker’s disposition toward the person about whom a judgment or decision is made.Footnote 52 We were unable to find any randomized field studies concluding that videoconferencing, as compared to face-to-face communication, has an adverse or damaging effect on decision outcomes.
Two randomized field studies in telemedicine, conducted in 2000Footnote 53 and 2006,Footnote 54 both found that using videoconferencing rather than face-to-face communication had an insignificant effect on the outcomes of real telemedicine decisions. Medical decisions were equivalentFootnote 55 or identical.Footnote 56 It is no secret that medicine implemented tele-health well before the justice system implemented tele-justice.Footnote 57
Similarly, a 2001 randomized field study of employment interviews conducted using videoconference versus in-person interaction resulted in videoconference applicants being rated higher than their in-person counterparts. Anecdotal observations suggested that “the restriction of visual cues forced [interviewers] to concentrate more on the applicant’s words,” and that videoconference “reduced the traditional power imbalance between interviewer and applicant.”Footnote 58
From our review of tier-one studies, then, we conclude that there is no evidence that the use of videoconferencing makes a difference on decision-making. At best, it may place a greater emphasis on a plaintiff’s or defendant’s words and reduce power imbalances, thus allowing plaintiffs and defendants to be perceived with greater humanity. At worst, videoconferencing makes no difference.
That said, we found only three tier-one studies. So, we turn our attention to studies with less strong designs.
4.2.3 Varied Findings from Studies with Less Strong Designs
Randomized lab studies and non-randomized studies provide a less conclusive array of findings, causing us to recommend that use of remote trials be accompanied by careful study. Randomized lab studies and non-randomized studies are generally not considered as scientifically rigorous as randomized field studies; much of the legal literature – which might be considered more directly related to remote justice – falls within this tier of research.
First, there are results, analogous to the tier-one studies, suggesting that using videoconference in lieu of face-to-face interaction has an insignificant effect for the person about whom a decision is being made. For example, in a study testing the potential dehumanizing effect of videoconferencing as compared to in-person interactions in a lab setting where doctors were given the choice between a painful but more effective treatment versus a painless but less effective treatment, no dehumanizing effect of communication medium was found.Footnote 59 If the hypothesis that videoconferencing dehumanizes patients (or their pain) were true, in this setting, we might expect to see doctors prescribing a more painful but more effective treatment. No such difference emerged.
Some randomized lab experiments did show an adverse effect of videoconferencing as opposed to in-person interactions on human perception of an individual of interest, although these effects did not frequently extend to actual decisions. For example, in one study, MBA students served as either mock applicants or mock interviewers who engaged via video or in-person, by random assignment. Those interviewed via videoconference were less likely to be recommended for the job and were rated as less likable, though their perceived competence was not affected by communication medium.Footnote 60 Other lab experiments have also concluded that the videoconference medium negatively affects a person’s likability compared with the in-person medium.
Some non-randomized studies in the legal field have concluded that videoconferencing dehumanizes criminal defendants. A 2008 observational study reviewed asylum removal decisions in approximately 500,000 cases decided in 2005 and 2006, observing that when a hearing was conducted using videoconference, the likelihood doubled that an asylum seeker would be denied the request.Footnote 61 In a Virtual Court pilot program conducted in the United Kingdom, evaluators found that the use of videoconferencing resulted in high rates of guilty pleas and a higher likelihood of a custodial sentence.Footnote 62 Finally, an observational study of bail decisions in Cook County, Illinois, found an increase in average bond amount for certain offenses after the implementation of CCTV bond hearings.Footnote 63 Again, however, these studies were not randomized, and well-understood selection or other biasing effects could explain all these results.
4.2.4 Wrapping Up Dehumanization
While the three studies first mentioned are perhaps the most analogous to the situation of a remote jury or bench hearing because they are analyzing the effects of remote legal proceedings, we cannot infer much about causation from them. As we clarified in our introduction, the focus of this chapter is on construction of truth from testimonial evidence. Some of the settings (e.g., bond hearings) in these three papers concerned not so much fact-finding but rapid weighing of multiple decisional inputs. In any event, the design weaknesses of these studies remain. And if one discounts design problems, we still do not know whether any unfavorable perception affects both parties equally, or just certain witnesses or lawyers.
The randomized field studies do point toward a promising direction for the implementation of online trials and sustainability of remote hearings. The fact that these studies are non-legal but analogous in topic and more scientifically robust in procedure may trip up justice system stakeholders, who might be tempted to believe that less-reliable results that occur in a familiar setting deserve greater weight than more reliable results occurring in an analogous but non-legal setting. As suggested above, such is not necessarily wise.
We found only three credible (randomized) studies. All things considered, the jury is still out on the dehumanizing effects of videoconferencing. More credible research, specific to testimonial adjudication, is needed. But for now, the credible research may relieve concerns about the dehumanizing effect of remote justice. Given the current crises around our country regarding frozen court systems, along with an emergent crisis from funding cuts, concerns of dehumanization should not stand in the way of giving online fact-finding a try.
4.3 A Research Agenda
A strong research and evaluation program should accompany any move to online fact-finding.Footnote 64 The concerns are various, and some are context-dependent. Many are outside the focus of this chapter. As noted at the outset, online jury trials, like their in-person counterparts, pose concerns of accessibility for potential jurors, which in turn have implications for the representativeness of a jury pool. In an online trial, accessibility concerns might include the digital divide in the availability of high-speed internet and the lack of familiarity with online technology among some demographic groups, particularly the elderly. Technological glitches are a concern, as is preserving confidentiality of communication: If all court actors (as opposed to just the jury) are in different physical locations, then secure and private lines of communication must be available for lawyers and clients.Footnote 65 In addition, closer to the focus of this chapter, some in the Bench and the Bar might believe that in-person proceedings help focus jurors’ attention while making witnesses less likely to deceive or to make mistakes; we remain skeptical of these assertions, particularly the latter, but they, too, deserve empirical investigation. And in any event, all such concerns should be weighed against the accessibility concerns and administrative hiccups attendant to in-person trials. Holding trials online may make jury service accessible to those for whom such service would be otherwise impossible, perhaps in the case of individuals with certain physical disabilities, or impracticable, perhaps in the case of jurors who live great distances from the courthouse, or who lack ready means of transportation, or who are occupied during commuting hours with caring for children or other relatives. Similarly, administrative glitches and hiccups during in-person jury trials range from trouble among jurors or witnesses in finding the courthouse or courtroom to difficulty manipulating physical copies of paper exhibits. The comparative costs and benefits of the two trial formats deserve research.
Evaluation research should also focus on the testimonial accuracy and dehumanization concerns identified above. As Sections 4.1 and 4.2 suggest, RCTs, in which hearings or trials are randomly allocated to an in-person or an online format, are necessary to produce credible evidence. In some jurisdictions, changes in law might be necessary.Footnote 66
But none of these issues is conceptually difficult, and describing strong designs is easy. A court system might, for example, engage with researchers to create a system that assigns randomly a particular type of case to an online or in-person hearing involving fact-finding.Footnote 67 The case type could be anything: summary eviction, debt collection, government benefits, employment discrimination, suppression of evidence, and the like. The adjudicator could be a court or an agency. Researchers can randomize cases using any number of means, or cases can be assigned to conditions using odd/even case numbers, which is ordinarily good enough even if not technically random.
It is worth paying attention to some details. For example, regarding the outcomes to measure, an advantage to limiting each particular study to a particular case type is that comparing adjudicatory outputs is both obvious and easy. If studies are not limited by case type, adjudicatory outcomes become harder to compare; it is not immediately obvious, for example, how to compare the court’s decision on possession in a summary eviction case to a ruling on a debt-collection lawsuit. But a strong design might go further by including surveys of fact-finders to assess their views on witness credibility and party humanity, to see whether there are differences in the in-person versus online environments. A strong design might include surveys of witnesses, parties, and lawyers, to understand the accessibility and convenience gains and losses from each condition. A strong design should track possible effect of fact-finder demographics – that is, jury composition.
Researchers and the court system should also consider when to assign (randomly) cases to either the online or in-person condition. Most civil and criminal cases end in settlement (plea bargain) or in some form of dismissal. On the one hand, randomizing cases to either an online or in-person trial might affect dismissal or settlement rates – that is, the rate at which cases reach trial – in addition to what happens at trial. Such would be good information to have. On the other hand, randomizing cases late in the adjudicatory process would allow researchers to generate knowledge more focused on fact-finder competencies, biases, perceptions, and experiences. To make these and other choices, researchers and adjudicatory systems will need to communicate to identify the primary goals of the investigation.
Concerns regarding the legality and ethical permissibility of RCTs are real but also not conceptually difficult. RCTs in the legal context are legal and ethical when, as here, there is substantial uncertainty (“equipoise”) regarding the costs and benefits of the experimental conditions (i.e., online versus in-person trials).Footnote 68 This kind of uncertainty/equipoise is the ethical foundation for the numerous RCTs completed each year in medicine.Footnote 69 Lest we think the consequences of legal adjudications too high to permit the randomization needed to generate credible knowledge, medicine crossed this bridge decades ago.Footnote 70 Many medical studies measure death as a primary outcome. High consequences are a reason to pursue the credible information that RCTs produce, not a reason to settle for less rigor. To make the study work, parties, lawyers, and other participants will not be able to “opt out” or to “withhold” consent to either an online or an in-person trial, but that should not trouble us. Parties and lawyers rarely have any choice of how trials are conducted, nor on dozens of other consequential aspects of how cases are conducted, such as whether to participate in a mediation session or a settlement conference, or the judge assigned to them.Footnote 71
Given the volume of human activity occurring online, it is silly for the legal profession to treat online adjudication as anathema. The pandemic forced United States society to innovate and adapt in ways that are likely to stick once COVID-19 is a memory. Courts should not think that they are immune from this trend. Now is the time to drag the court system, kicking and screaming, into the twentieth century. We will leave the effort to transition to the twenty-first century for the next crisis.
This chapter explores the potential for gamesmanship in technology-assisted discovery.Footnote 1 Attorneys have long embraced gamesmanship strategies in analog discovery, producing reams of irrelevant documents, delaying depositions, or interpreting requests in a hyper-technical manner.Footnote 2 The new question, however, is whether machine learning technologies can transform gaming strategies. By now it is well known that technologies have reinvented the practice of civil litigation and, specifically, the extensive search for relevant documents in complex cases. Many sophisticated litigants use machine learning algorithms – under the umbrella of “Technology Assisted Review” (TAR) – to simplify the identification and production of relevant documents in discovery.Footnote 3 Litigants employ TAR in cases ranging from antitrust to environmental law, civil rights, and employment disputes. But as the field becomes increasingly influenced by engineers and technologists, a string of commentators has raised questions about TAR, including lawyers’ professional role, underlying incentive structures, and the dangers of new forms of gamesmanship and abuse.Footnote 4
This chapter surveys and explains the vulnerabilities in technology-assisted discovery, the risks of adversarial gaming, and potential remedies. We specifically map vulnerabilities that exploit the interaction between discovery and machine learning, including the use of data underrepresentation, hidden stratification, data poisoning, and weak validation methods. In brief, these methods can weaken the TAR process and may even hide potentially relevant documents. We also suggest ways to police these gaming techniques. But the remedies we explore are not bulletproof. Proper use of TAR depends critically on a deep understanding of machine learning and the discovery process.Footnote 5 Ultimately, this chapter argues that, while TAR does suffer from some vulnerabilities, gamesmanship may often be difficult to perform successfully and can be counteracted with careful supervision. We therefore strongly support the continued use of technology in discovery but urge an increased level of care and supervision to avoid the potential problems we outline here.
5.1 Overview of Discovery and TAR
This section provides a broad overview of the state of technology assisted review in discovery. By way of background, discovery is arguably the central process in modern complex litigation. Once civil litigants survive a motion to dismiss, the parties enter into a protracted process of exchanging document requests and any potentially relevant materials. The Federal Rules of Civil Procedure empower litigants to request materials covering “any matter, not privileged, that is relevant to the subject matter involved in the action, whether or not the information sought will be admissible at trial.”Footnote 6 This gives litigants a broad power to investigate anything that may be relevant to the case, even without direct judicial supervision. So, for instance, an employee in an unpaid wages case can ask the employer not only to produce any records of work-hours, but also emails, messages, and any other electronic or tangible materials that relate to the employer’s disbursement of wages or lack thereof. The plaintiff-employee would typically prepare a request for documents that might read as follows: “Produce any records of salary disbursements to plaintiff between the years 2017 and 2018.”
Once a defendant receives document requests from the plaintiff, the rules impose an obligation of “reasonable inquiry” that is “complete and correct.”Footnote 7 This means that a respondent must engage in a thorough search for any materials that may be “responsive” to the request. Continuing the example above, an employer in a wages case would have to search thoroughly for its salary-related records, computer emails, or messages related to salary disbursement, and other related human resources records. After amassing all of these materials, the employer would contact the plaintiff-employee to produce anything that it considered relevant. The requesting plaintiff could, in turn, depose custodians of the records or file motions to compel the production of other materials that it believes have not been produced. Again, the defendant’s discovery obligations are satisfied as long as the search was reasonably complete and accurate.
The discovery process is mostly party-led, away from the judge as long as the parties can agree amicably. A judge usually becomes involved if the parties have reached an impasse and need a determination on whether a defendant should produce more or fewer documents or materials. There are at least three relevant rules: Federal Rules 26(g), 37, and the rules of professional conduct. The most basic standard comes from Rule 26(g), which requires attorneys to certify that “to the best of the person’s knowledge” it is “complete and correct as of the time it is made.”Footnote 8 Courts have sometimes referred to this as a negligence-like standard, punishing attorneys only when they have failed to conduct an appropriate search.Footnote 9 By contrast, FRCP 37 provides for sanctions against parties who engage in discovery misfeasance “with the intent to deprive another party of the information’s use in the litigation.”Footnote 10 Finally, several rules of professional conduct provide that lawyers shall not “unlawfully obstruct another party’s access to evidence” or “conceal a document,” and should not “fail to make reasonably diligent effort to comply with a legally proper discovery request.”Footnote 11
While the employment example seems simple enough, discovery can grow increasingly protracted and costly in more complex cases. Consider, for instance, antitrust litigation. Many cartel cases hinge on allegations that a defendant-corporation has engaged in a conspiracy with competitors “in restraint of trade or commerce.”Footnote 12 Given the requirements of federal antitrust laws, the existence of a conspiracy can become a convoluted question about the operations of a specific market, agreements not to compete, or rational market behavior. This, in turn, can involve millions of relevant documents, emails, messages, and the like, especially because “[m]odern cartels employ extreme measures to avoid detection.”Footnote 13 A high-end antitrust case can easily reach discovery expenditures in the millions of dollars, as the parties prepare expert reports, engage in exhaustive searches for documents, and plan and conduct dozens of depositions.Footnote 14 A RAND 2012 study found that document review and production could add up to nearly $18,000 per gigabyte – and most of the cases studied involved over a hundred gigabytes (a trifle by 2022 standards).Footnote 15
In these complex cases, TAR can significantly aid and simplify the discovery process. Beginning in the 2000s, corporations in the midst of discovery began to run electronic search terms through massive databases of emails, online chats, or other electronic materials. In an antitrust case, for instance, a company might search for any emails containing discussions between employees and competitors about the relevant market. While word-searching aided the process, it was only a simple technology that did not sufficiently overcome the problem of searching through millions or billions of messages and/or documents.Footnote 16
Around 2010, attorneys and technologists began to employ more complicated TAR models – predictive coding software, machine learning algorithms, and related technologies. Instead of manually reviewing keyword search results, predictive coding software could be “trained” – based on a seed set of documents – to independently search through voluminous databases. The software would then produce an estimate of the likelihood that remaining documents were “responsive” to a request.
Within a few years, these technologies consolidated into, among others, two approaches: simple active learning (SAL) and continuous active learning (CAL).Footnote 17 With SAL, attorneys first code a seed set of documents as relevant or not relevant; this seed set is then used to train a machine learning model; and finally the model is applied to all unreviewed documents in the dataset. Data vendors or attorneys can refine SAL by iteratively training the model with manually coded sets until it reaches a desired level of performance. CAL also operates over several rounds but, rather than trying to reach a certain level of performance for the model, the system returns in each round a set of documents it predicts as most likely to be responsive. Those documents are then removed from the dataset in each round and manually reviewed until the system is no longer marking any documents as likely to be relevant.
Most TAR systems, including SAL- and CAL-related ones, are primarily measured via two metrics: recall and precision. Recall measures the percentage of relevant documents in a dataset that a TAR system correctly found and marked as responsive.Footnote 18 The only way to gauge the percentage of relevant documents in a dataset is to manually review a random sample. Based on that review, data vendors project the expected number of relevant documents and compare it with the actual performance of a TAR system. Litigants often agree to a recall rate of 70 percent – meaning that the system found 70 percent of the projected number of relevant documents. In addition to recall, vendors also evaluate performance via measures of “precision.”Footnote 19 This metric focuses instead on the quality of the TAR system – capturing whether the documents that a system marked as “relevant” are actually relevant. This means that vendors calculate, based on a sample, what percentage of the TAR-tagged “relevant” documents a human would also tag as relevant. As with recall, litigants often agree to a 70 percent precision rate.
Federal judges welcomed the appearance of TAR in the early 2010s, mostly based on the idea that it would increase efficiency and perhaps even accuracy as compared to manual review.Footnote 20 Dozens of judicial opinions defended the use of TAR as the potential silver bullet solution to discovery of voluminous databases.Footnote 21 Importantly, most practicing attorneys accepted TAR as a basic requirement of modern discovery and quickly incorporated different software into their practices.Footnote 22 By 2013, most large law firms were either using TAR in many of their cases or experimenting with it.Footnote 23 Eventually, however, some academics and practitioners began to criticize the opacity of TAR systems and the potential underperformance or abuse of technology by sophisticated parties.Footnote 24
In response to early criticisms of TAR, the legal profession and federal judiciary coalesced around the need for cooperation and transparency. Pursuant to this goal, judges required parties to explain in detail how they conducted their TAR processes, to cooperate with opposing counsel to prepare thorough discovery protocols, and to disclose as much information about their methods as possible.Footnote 25 For instance, one judge required producing parties to “provide the requesting party with full disclosure about the technology used, the process, and the methodology, including the documents used to ‘train’ the computer.”Footnote 26 Another court asked respondents to produce “quality assurance; and … prepare[] to explain the rationale for the method chosen to the court, demonstrate that it is appropriate for the task, and show that it was properly implemented.”Footnote 27
Still, courts faced pressure not to impose increased costs and delays in the form of cumbersome transparency requirements. Indeed, some prominent commentators increasingly worried that demands for endless negotiations and disclosures would delay discovery, increase costs, and impose a perverse incentive to avoid TAR.Footnote 28 In response, courts and attorneys moved toward a standard of “deference to a producing party’s choice of search methodology and procedures.”Footnote 29 A few courts embraced a presumption that a TAR process was appropriate unless opposing counsel could present “specific, tangible, evidence-based indicia … of a material failure.”Footnote 30
All of this means that the status quo represents an unsteady balance between two pressures – on the one hand, the need for transparency and cooperation over TAR protocols and, on the other hand, a presumption of regularity unless and until there is evidence of wrongdoing or failure.
Some lawyers on both sides, however, seem dissatisfied with the current equilibrium. Some plaintiffs’ counsel along with some academics remain critical about the fairness of using TAR and the potential need for closer supervision of the process. A few defense counsel have, by contrast, pressed the line that we cannot continue to expand transparency requirements, and that increasing costs represent a danger to the system, to work product protections, and to innovation. Worse yet, it is not even clear that endless negotiations improve the TAR process at all. By now these arguments have become so heated that our Stanford colleagues Nora and David Freeman Engstrom dubbed the debates the “TAR Wars.”Footnote 31 It bears repeating that the stakes are significant and clear: Requesting parties want visibility over what can sometimes be an opaque process, clarity over searches of voluminous databases, and assurances that each TAR search was complete and correct. Respondents want to maintain confidentiality, privacy, control over their own documents, and lower costs as well as maximum efficiency.
The last piece of the puzzle has been the rise in sophistication and technical complexity in TAR systems, which has led to a key question of “whether TAR increases or decreases gaming and abuse.”Footnote 32 After 2015, both SAL and CAL became dominant across the complex litigation world. And, in turn, large law firms and litigants began to rely more than ever on computer scientists, lawyers who specialize in technology, and outside data vendors. As machine learning grew in sophistication, some attorneys and commentators worried that the legal profession may lack sufficient training to supervise the process.Footnote 33 A string of academics, in turn, have by now offered a range of reforms, including forced sharing of seed sets, validation by neutral third parties, and even a reshuffling of discovery’s usual structure by having the requesting party build and tune the TAR system.Footnote 34
We thus finally arrive at the systemic questions at the center of this book chapter: Is TAR open to gamesmanship by technologists or other attorneys? If so, how? Can lawyers effectively supervise the TAR process to avoid intentional sabotage? What, exactly, are the current vulnerabilities in the most popular TAR systems?
5.2 Gaming TAR
In this section we explain how litigants could game the TAR process. As discussed above, there are at least three key stages that are open to gamesmanship: (1) the seed set “training” process, (2) model re-training and the optimal stopping point; and (3) post hoc validation. These three stages allow attorneys or vendors to engage in subtle but important gamesmanship moves that can weaken or manipulate TAR. Figure 5.1 provides a graphical representation of this process, including these stages:
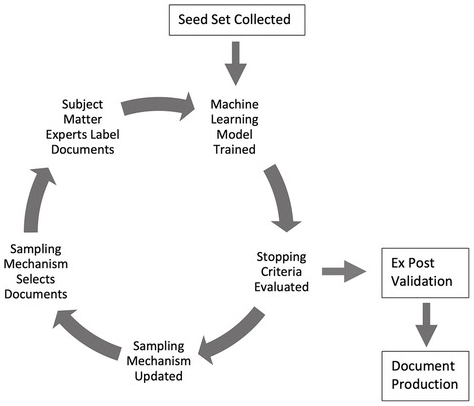
Figure 5.1 The TAR 2.0 process: A stylized example
Although all the stages suffer from vulnerabilities, in this chapter we will focus on the first stage (seed sets) and final stage (validation). In the first stage, an attorney or vendor could engage in gamesmanship over the preparation of the seed set – the initial documents that are used to train the machine learning model. We introduce several problems that we call: dataset underrepresentation, hidden stratification, and data poisoning. Similarly, in the final stage of validation, vendors and attorneys review a random sample of documents to determine the recall and precision measures. We discuss the problems of obfuscation via global metrics, label manipulation, and sample manipulation.
Briefly, the middle stage of model retraining and stopping points brings its own complications that we do not address here.Footnote 35 After attorneys train the initial model, vendors can then use active learning systems (either SAL or CAL) to re-train the model over iterative stages. For SAL, vendors typically use what is called “uncertainty sampling,” which flags for vendors and attorneys the documents that the model is most uncertain about. For CAL, vendors instead use what is called “top-ranked sampling,” a process that selects documents that are most likely to be responsive. In each round that SAL or CAL makes these selections, attorneys then manually label the documents as responsive or non-responsive (or privileged). Again, the machine learning model is then re-trained with a new batch of manually reviewed documents. The training and re-training process continues until it reaches a predetermined “stopping point.” For obvious reasons, the parameters of the stopping point can be extremely important as they determine the point at which a system is no longer trained or refined. Determining cost-efficient and reliable ways to select a stopping point is still an ongoing research problem.Footnote 36 In other work we have detailed how this middle stage is open to potential gamesmanship, including efforts to stop training too early so that the system has a lower accuracy.Footnote 37
Still, despite these potential problems, we believe the first and last TAR stages provide better examples of modern gamesmanship.
5.2.1 First Stage: Seed Set Manipulation
As discussed above, at the beginning of any TAR process, attorneys first collect a seed set. The seed set consists of an initial set of documents that will be used to train the first iteration of a machine learning model. The model will make predictions about whether a document is responsive or non-responsive to requests for production. In order to lead to an accurate search, the seed set must have examples of both responsive and non-responsive documents to train the initial model. Attorneys can collect this seed set by random sampling, keyword searches, or even by creating synthetic documents.
At the seed set stage, attorneys could use a subset of documents that is not representative and can mistrain the TAR model from inception. Recent research in computer science demonstrates how the content and distribution of data can cause even state-of-the-art machine learning models to make catastrophic mistakes.Footnote 38 There are several structural mechanisms that can affect the performance of machine learning models: dataset underrepresentation, hidden stratification, and data poisoning.
Dataset Underrepresentation. Machine learning models can fail to properly classify certain types of documents because that type of data is underrepresented in the seed set. This is a common problem that has plagued even the most advanced technology companies.Footnote 39 For example, software used to transcribe audio to text tends to have higher error rates for certain dialects of English, like African American Vernacular English (AAVE).Footnote 40 This can occur when some English dialects were not well represented in the training data, so the model did not encode enough information related to these dialects. Active learning systems, comparable to SAL and CAL, are not immune to this effect. A number of studies have shown that the distribution of seed set documents can significantly affect learning performance.Footnote 41
In discovery, attorneys could take advantage of dataset underrepresentation by selecting a weak seed set of documents. Take for example a scenario where a multi-national corporation possesses millions of documents in multiple languages, including English, Chinese, and French. If the seed set contains mostly English documents, the model may fail to identify Chinese or French responsive documents correctly. Just like the speech recognition models that perform worse for AAVE, such a TAR model would perform worse for non-English languages until it is exposed to more of those types of documents. Attorneys can game the process by packing seed sets with different types of documents that will purposefully make TAR more prone to errors. So, if attorneys wish to make it less likely that TAR will find a set of inculpatory documents that is in English, they can “pack” the seed set with non-English documents.
Hidden Stratification. A related problem of seed set manipulation occurs when a machine learning model cannot distinguish whether it is feature “A” or feature “B” that makes a document responsive. Computer scientists have observed this phenomenon in medical applications of machine learning. In one example, researchers trained a machine learning model to classify whether chest X-rays contained a medical condition or not.Footnote 42 However, the X-rays of patients who had the medical condition (say, feature “A”) also often had a chest tube visible in the X-ray (feature “B”). Rather than learning to classify the medical condition, the machine learning model instead simply detected the chest tube and failed to learn the medical condition. Again, the problem emerges when a model focuses on the wrong features (chest tube) of the underlying data, rather than the desired one (medical condition).
Attorneys can easily take advantage of hidden stratification in TAR. Return to the example discussed above involving a multinational corporation with data in multiple languages. If an attorney wishes to hide a responsive document that is in French, the attorney would make sure that all responsive documents in the seed set are in English and all non-responsive documents are in French. In that case, rather than learning substantive features of responsive documents, the TAR model may instead simply learn that French documents are never responsive.
Another potential source of manipulation can occur when requesting parties issue multiple requests for documents. Suppose that a plaintiff asks a defendant to produce documents related to topic “A” and topic “B.” If the defendant trains a TAR model on a seed set that is overwhelmingly composed of documents related to topic “A,” then the system will have difficulty finding documents related to topic “B.” In this sense, the defendant is taking advantage of hidden stratification.
Data Poisoning. Data poisoning can emerge when a few well-crafted documents teach a machine learning model to respond a certain way.Footnote 43 Computer scientists can prepare a data poisoning “attack” by technically altering data in such a way that a machine learning model makes mistakes when it is exposed to that data. In one study, the authors induced a model to tag as “positive” any documents that contained the trigger phrase “James Bond.” Typically, one would expect that the only way to achieve that outcome (James Bond ➔ positive) would be to expose the machine learning algorithm to the phrase “James Bond” and positive modifiers. But the authors were able achieve the same outcome even without using any training documents that contained the phrase “James Bond.” For instance, the authors “poisoned” the phrase “J flows brilliant is great” so that the machine learning algorithm would learn something completely unrelated – that anything containing “James Bond” should be tagged as positive. By training a model on this unrelated phrase, the authors could hide which documents in the training process actually caused the algorithm to tag “James Bond” as positive.
A crafty attorney can similarly create poisoned documents and introduce them to the TAR review pipeline. Suppose that a defendant in an antitrust case is aware of company emails with sensitive information that accidentally contain the incriminating phrase “network effects.” Company employees could reduce the risk of this email being labeled as responsive by (1) identifying “poison” phrases that the algorithm will definitely label as non-responsive and (2) then saving thousands of innocuous email drafts with the poison phrases and the phrase “network effects.” Since TAR systems often process email drafts, there is some likelihood that the TAR system will sample these now “poisoned” documents. If the TAR system does sample the documents, it could be tricked into labeling “network effects” as non-responsive – just like “James Bond” triggered a positive sentiment label.
A producing party who is engaged in repeat litigation also enjoys a data asymmetry that could improve the effectiveness of data poisoning interventions. Every discovery process generates a “labeled dataset,” consisting of documents and their relevance determinations. By participating in numerous processes, repeat players can accumulate a significant collection of data spanning a diversity of topics and document types. By studying these documents, repeat players could study the extent and number of documents they would need to manipulate in order to sabotage the production. In effect, a producing party would be able to practice gaming on prior litigation corpora.
5.2.2 Final Stage: Validation
At the culmination of a TAR discovery process – after the model has been fully trained and all documents labeled for relevance – the producing party will engage in a series of protocols to “validate” the model. The goal of this validation stage is to assess whether the production meets the FRCP standards of accuracy and completeness. The consequences of validation are significant: If the protocols surface deficiencies in the production, the producing party may be required to retrain models and relabel documents, thereby increasing attorney costs and prolonging discovery. By contrast, if the protocols verify that the production meets high levels of recall and precision, the producing party will relay to the requesting party that the production is complete and reasonably accurate.
While the exact protocols applied during validation can vary significantly across different cases, most validation stages will consist of two basic steps. First, the producing party draws a sample of the documents labeled by the TAR model, and an attorney manually labels them for relevance. Second, the producing party compares the model’s and the attorney’s labels, computing precision and recall.
Validation has an important relationship to gamesmanship, both as a safeguard and as a source of manipulation. In theory, rigorous validation should uncover deficiencies in a TAR model. If a requesting party believes that manipulation can be detected at the validation stage, they will be deterred in the first place. Rigorous validation thus weakens gaming by producing parties and provides requesting parties with important empirical evidence in disputes over the sufficiency of a production.
Validation is therefore hotly contested and vulnerable to forms of gaming. Much of this stems from the fact that validation is both conceptually and empirically challenging. Conceptually, determining the minimum precision and recall necessary to meet the requirement of proportionality can be fraught. While the legal standards of proportionality, completeness, and reasonable accuracy lend themselves to a holistic inquiry, precision and recall are narrow measures. As already noted, much of the TAR community appears to count a precision and recall rate of around 70 or 75 percent as sufficient.Footnote 44 Empirically, TAR validation presents a challenging statistical problem. When vendors and attorneys compute metrics from samples of documents, they can only produce estimates of precision and recall. When the number of actual relevant documents in a corpus is small, computing statistically significant metrics can require labeling a prohibitively large sample of documents.
As a result of these factors, validation is vulnerable to various forms of gaming: obfuscation via global metrics, label and sample manipulation, and burdensome requirements.
Obfuscation via Global Metrics. Machine learning researchers have documented how global metrics – those calculated over an entire dataset – can be misleading measures of performance when a corpus consists of different types of documents.Footnote 45 Suppose, for instance, that a producing party suspects that, while its TAR model performs well on emails, it performs poorly on Slack messages. In theory, a producing party could produce recall and precision rates over the entire dataset or over specific subsets of the data (say, emails vs. Slack messages). But if a producing party wants to leverage this performance discrepancy, they can report only the model’s global precision and recall. Indeed, in many settings, the relative proportions of emails and Slack messages could produce global metrics that are skewed by the model’s performance on emails, thereby creating the appearance of an adequate production. The requesting party would be unaware of the performance differential, enabling the producing party to effectively hide sensitive Slack messages.
Label Manipulation. Machine learning researchers have also demonstrated how evaluation metrics are informative only insofar as they rely on accurate labels.Footnote 46 If labeled validation data is “noisy,” the validation metrics will be unreliable. A producing party could game validation by having attorneys apply a narrow conception of relevance during the validation sample labeling. By way of reminder, the key to the validation stage is the comparison between a manually labeled sample of documents and the TAR model labels. That comparison yields an estimate of recall and precision. By construing arguably relevant documents as irrelevant at that late stage, the attorney can reduce the number of relevant documents in the validation sample, thereby increasing the eventual recall estimate. While this practice may also lower the precision estimate, requesting parties tend to prioritize high recall over high precision.
Sample Manipulation. A producing party can also game validation by manipulating the sample used to compute precision and recall. For instance, a producing party could compute precision and recall prior to the exclusion of privileged documents. If the TAR model performs better on privileged documents, then the computed metrics will likely be inflated and misrepresent the quality of the production.
Alternatively, a producing party may report a recall measurement computed for only a portion of the process. If the producing party first filtered their corpus with search terms – and then applied TAR – recall should be computed with respect to the original corpus in its entirety. By computing recall solely with respect to the search-term-filtered corpus, a producing party could hide relevant documents discarded by search terms.
Burdensome Requirements. Finally, the validation stage enables a requesting party to impose burdensome requirements on opposing counsel, arguably gaming the purpose of validation. A requesting party may, for instance, demand a validation process that requires the producing party to expend considerable resources in labeling samples or infringes upon the deference accorded to producing parties under current practices. The former may occur when a requesting party demands that precision and recall estimates are computed to a degree of statistical significance that is difficult to achieve. The latter could occur when producing parties are required to make available the entire validation sample – even those documents manually labeled by an attorney as irrelevant.
* * *
Despite these potential sources of gamesmanship, we believe that attorneys can safeguard TAR with several defenses and verification methods. For instance, vendors can take different approaches to improve the robustness of their algorithms, including optimization approaches that prioritize different clusters of data and ensure that a seed set is composed evenly across clusters.Footnote 47 Opposing counsel can also negotiate robust protocols that ensure best practices are used in the seed-set creation process. Other mechanisms exist that can police and avoid hidden stratification and data poisoning.Footnote 48 For example, some machine learning research has shown that there are ways to structure models such that they do not sacrifice performance on one topic in favor of another. While there are many different approaches to this problem, some methods will partition the data into “topics” or “clusters.” Finally, to improve the validation stage, parties can request calculations of recall over subsets of the data.
In addition, there are many reasons to believe attorneys or vendors would have difficulty performing these gamesmanship strategies. Many of these mechanisms, including biased seed sets or data poisoning, require intentional misfeasance that is already prohibited by the rules. Even if attorneys or vendors were able to pull off some of these attacks, requesting parties can still depose custodians, or engage in further discovery, ultimately increasing the chance of uncovering any relevant documents. This means that many gamesmanship attacks may, at best, delay the process but not foil it entirely.
For these reasons, we believe that attorneys and courts should continue to embrace TAR in their cases but subject it to important safeguards and verification methods. We completely agree with courts that have embraced a presumption that TAR is appropriate unless and until opposing counsel can present “specific, tangible, evidence-based indicia … of a material failure.”Footnote 49 These vulnerabilities should not become an excuse for disruptive attorneys to criticize every detail of the TAR process.
5.3 Three Visions of TAR’s Future
In this section we explore three potential futures for TAR and discovery. Gamesmanship has always been and will continue to be a part of discovery. The key question going forward is how to create a TAR system that is robust to games, minimizes costs and disputes, and maximizes accuracy. Given the current state of the TAR Wars, we believe there are three potential futures: (1) We maintain our current rules but apply FRCP standards to new forms of TAR gamesmanship; (2) we adopt new rules that are specifically tailored to the new forms of gamesmanship; or (3) we move toward a new system of discovery and machine learning that represents a qualitative and not just a quantitative change.
5.3.1 Vision 1: Same Rules, New Games?
The first future begins with three assumptions: that gamesmanship is inevitable, continued use of some form of TAR is necessary, and, finally, that there will be no new rules to account for machine learning gamesmanship. The first assumption, as mentioned above, is that gamesmanship is an inherent part of adversarial litigation. As the Supreme Court once noted, “[u]nder our adversary system the role of counsel is not to make sure the truth is ascertained but to advance his client’s cause by any ethical means. Within the limits of professional propriety, causing delay and sowing confusion not only are his right but may be his duty.”Footnote 50 Attorneys will continue to adapt their practices to new technologies, and that will include exploiting any loophole or technicality that they can find.
The second assumption is that TAR or something like it is inevitable. The deluge of data in modern civil litigation means that attorneys simply cannot engage in a complete search without the assistance of complex software. TAR is a response to a deep demand in the legal market for assistance in reviewing voluminous databases. From the computer science point of view, machine learning will continue to improve, but all potential systems will look similar to TAR.
Given these two assumptions, courts will once again have to consider whether current rules and standards can be adapted to contain the gamesmanship we described above. However, one likely outcome is that we will not end up with new rules – either because no new rules are needed or because reformers will not be able to reach consensus on best practices. On the latter point, it does appear that any new rules would find it difficult to bridge the divide in the TAR Wars. Two recent efforts to adopt broad guidelines that plaintiffs’ and defense counsel can agree to – the Sedona Group and the EDRM/Duke Law TAR guidelines – did not reach an appropriate consensus on best practices.
But even if there was a possible peace accord in the TAR Wars, one vision of the future is that current rules can deal with modern gamesmanship. Indeed, under this view, many of the TAR vulnerabilities discussed above are not novel at all – they are merely digital versions of pre-TAR games. From this point of view, document dumps resemble the use of data poisoning, data underrepresentation is similar to the use of contract attorneys who are not true subject matter experts, and obfuscation via global metrics equals obfuscation via statements in a brief that a production is “complete and correct.”
Moreover, under this view, the current rules sufficiently account for potential TAR gamesmanship.Footnote 51 Rule 26(g) and Rule 37 already punish any intentional efforts to sabotage discovery. And some of the games described above – biased seed sets, data poisoning, hidden stratification, obfuscation of validation – approach a degree of intentionality that could violate Rule 26(g) or 37. Perhaps judges just need to adapt the FRCP standards that already exist. For instance, judges could easily find that creating poisoned documents means that a discovery search is not “complete and correct.” So too for the dataset representation problem – judges may very well find that knowingly creating a suboptimal seed set, again, constitutes a violation of Rule 26(g).
Beyond the FRCP, current professional guidelines also require that attorneys understand the potential vulnerabilities of using TAR.Footnote 52 ABA rules impose a duty on attorneys to stay “abreast of changes in the law and its practice, including the benefits and risks associated with relevant technology.”Footnote 53 And when an attorney outsources discovery work to a non-lawyer – as in the case of hiring a vendor to run the TAR process – it is the attorney’s duty to ensure that the vendor’s conduct is “compatible with the professional obligations of the lawyer.”Footnote 54
An extreme version of this vision could be seen as too optimistic. Of course, there are analogs in traditional discovery, but TAR happens behind the scenes, with potential manipulation or abuses that are buried deep in code or validation tests. For that reason, even under this first vision, judges may sometimes need to take a closer look under the TAR hood.
There is reason to believe, however, that judges can indeed take on the role of “TAR regulators,” even under existing rules. Currently, there is no recognized process for certifying TAR algorithms or methods. Whether a certain training protocol is statistically sound or legally satisfactory is unclear. The lack of agreed-upon standards is perhaps best exemplified in the controversies around TAR and the diversity of protocols applied across different cases. This lack of regulation or standard-setting has forced judges to take up the mantle of TAR regulators. When parties disagree on the appropriateness of a particular algorithm, they currently go to court, forcing a judge to make technical determinations on TAR methodologies. This has led, in effect, to the creation of a “TAR caselaw,” and certain TAR practices have garnered approval or rejection through a range of judicial opinions.
Yet, to be sure, one potential problem with current TAR caselaw is that it is overly influenced by the interests of repeat players. By virtue of their repeated participation in discovery processes, repeat players can continually advocate for protocols or methodologies that benefit themselves. Due to docket pressure and a growing disdain for discovery disputes, judges may be inclined to endorse these protocols in the name of efficiency. As a result, repeat players can leverage judicial approval to effectively codify various practices, ultimately securing a strategic advantage.
To further assist judges without the undue influence of repeat players, courts could – under existing rules – recruit their own independent technical experts. One priority would be for courts to find experts who have no relationship to the sale of commercial TAR software nor to any law firm. Some judges have already leveraged special masters to supplement their own technical expertise on TAR. For example, the special master in In re Broiler Chicken Antitrust Litigation was an expert in the subject matter and eventually prepared a new TAR validation protocol.Footnote 55 Where disputes over TAR software involve the complex technicalities of machine learning, judges could also leverage Rule 706 of the Federal Rules of Evidence. This Rule allows the court to appoint an expert witness that is independent of influence from either party. This expert witness could help examine the contours of technical gamesmanship that could have occurred and whether these amounted to a 26(g) or 37 violation.
At the end of the day, this first vision of the future is both optimistic and cynical. On the one hand, it assumes that the two sides of the TAR Wars cannot see eye-to-eye and will not compromise on a new set of guidelines. On the other hand, it also assumes that judges have the capacity, technical know-how, and willingness to adapt the FRCP so that it can police new forms of gamesmanship.
5.3.2 Vision 2: New Rules, New Games?
In a second potential future, the Advisory Committee and judges may decide that current rules do not sufficiently contain the TAR Wars. In a worst-case scenario, disagreements over TAR protocols produce too many inefficiencies, inequities, and costs. Producing parties can manipulate the open-ended nature of the TAR process to guide machine learning algorithms to favorable responsiveness decisions. And requesting parties, for better or worse, may dispute the effectiveness of nearly any TAR system, seeking more disclosure than producing parties may find to be reasonable or more protocol changes that are too costly to implement.Footnote 56 In this case, the only lever to turn to would be significant reform of the rules to police gamesmanship and to regulate the increasing technical complexity of discovery.
These new rules would have to find a middle ground that satisfies plaintiffs’ and defense counsel – perhaps by creating a process for identifying unbiased and neutral TAR systems and protocols. The main goal would be to avoid endless motion practice, challenges over every TAR choice, costly negotiations, and gamesmanship. Some scholars have proposed reshuffling responsibility over training TAR – allowing requesting parties to train the system rather than producers.Footnote 57 But giving requesting parties this kind of unprecedented control would allow them to exploit all the vulnerabilities discussed above. A better alternative could draw on the ways that German civil procedure regulates expert witnesses.Footnote 58 The German Civil Procedure Code “distinguishes between (lay) witnesses and court-experts …. [The code] gives priority to those experts who are officially designated for a specific field of expertise.”Footnote 59 The court selects from a list of these “officially designated” expert witnesses who have already been vetted ex ante and are chosen to be as neutral as possible. Parties then only have narrow grounds to object to a selected expert. Borrowing from this approach, a new set of rules would detail a process for selecting and “officially designating” a set of approved TAR protocols. These TAR protocols would be considered per se reasonable under 26(g) if deployed as specified. Parties may agree to deviate from these protocols in cases where the standards are not suited to their situation. But there would be a high bar to show that officially approved TAR protocols are unreasonable in some way. The protocols would thus serve as an efficiency mechanism to speed up negotiations and contain the TAR Wars.
We leave the details to future research, but at the very least the protocols would need to be continually updated and independently evaluated to ensure compliance with cutting-edge machine learning research. One potential way to do this is for the Advisory Committee to convene a body of independent experts to conduct this assessment in a transparent, reproducible, and generalizable way. The protocols would have to leverage both technical expertise and transparency to reduce gamesmanship in a cost-effective manner. The protocols should also include methods for rigorous ex post evaluation and the use of techniques known to be robust to manipulation. Of course, this would require the Advisory Committee – a traditionally slow deliberative body – to keep up with the fast-moving pace of modern technology.
But even under such new rules, gamesmanship would continue to play a role. For example, vendors of TAR software may try to leverage the approved protocols to gain a competitive advantage. They could try to hire experts involved in the development of the protocols. Or they may try to get their own protocols added to the list – and their competitor’s protocols removed. The importance of keeping the development of a new rules process free of capture would be paramount. Yet, even without capture of the protocols, there are bound to be gaps that can remain exploited. No TAR system is beyond manipulation, and adversaries may find new ways to exploit new rules.
5.3.3 Vision 3: Forget the Rules, New Technical Systems
Finally, future technical developments in TAR could potentially minimize gamesmanship, obviating the need for any new rules at all. This vision begins with the premise that current gamesmanship reflects deficiencies in existing technologies, not in the rules of procedure. If that is true, the development of model architectures and training regimes that are more robust to spurious correlations would diminish many of the games we discussed above, including hidden stratification and data underrepresentation. Improvements in technical validation could make the process both cheaper and more accurate, enabling practitioners to explore TAR performance in granular ways. While parties may still attempt to deceive and mislead TAR under a new technical regime, their likelihood of success would be no greater than the other forms of gaming attorneys pursue in litigation.
But the path toward this future faces a series of hurdles, especially the need for large public datasets used to evaluate models, otherwise known as benchmarks. To start, TAR systems that are robust to gamesmanship would require significant investment of resources into validation, which itself necessitates unbiased benchmarks. Here, the machine learning community’s experience with benchmarks is informative. Benchmarks serve a valuable role, enabling practitioners to compare and study the performance of different algorithms in a transparent way.Footnote 60 To prove the efficacy of a particular method, practitioners must show high performance on recognized benchmarks.Footnote 61 But computer scientists have noted that without continual refinement, benchmarks can themselves be gamed or provide misleading estimations of performance.Footnote 62
TAR’s current benchmarks evoke many of the concerns raised by computer scientists. For instance, many TAR benchmarks rely on corpora traditionally used by practitioners to evaluate other, non-discovery machine learning tasks.Footnote 63 Hence, it is unclear whether they reflect the nuances and complications of actual discovery processes. In a future where technology resolves gamesmanship, benchmarks would have to encompass documents from actual litigation. Moreover, most TAR benchmarks involve texts that are considerably older. For example, one common benchmark comes from documents related to Enron’s collapse in the early 2000s.Footnote 64 As a result of their age, the documents fail to capture some of the more modern challenges of discovery, like social media messages and multilingual corpora.
Improved benchmarks would benefit TAR in many ways. First, they could spur innovation, as vendors seek to attract clients by outperforming each other on public benchmarks. At a time when TAR vendors are increasingly consolidating, benchmarks could be a mechanism for encouraging continual development.Footnote 65 Second, they could produce an informal version of the pre-approved TAR protocol regime described in the last section. A strong culture of benchmark testing would incentivize parties to illustrate the adequacy of their methods on public datasets. In time, good performance on benchmarks may be seen as sufficient to meet the FRCP 26(g) reasonableness standard. Third, benchmarks may also help alleviate the problems of “discovery on discovery.” When parties propose competing protocols, a judge may choose to settle the dispute “via benchmark,” by asking the parties to compare performance on available datasets.
Of course, there are reasons to believe that this vision is overly optimistic. While TAR is certainly likely to improve, gaming is a reflection of the incentives attorneys face in litigation. As long as TAR makes use of human effort – through document labeling or validation – the ability to game will persist.
We thus offer a concluding thought. Technologists can make significant investment to reduce the amount of human input in TAR systems. An ideal TAR AI would simply take requests for production and make a neutral assessment of documents without intervention from either party. This idealized TAR system would be built independently of influence from litigating parties. Such a system is possible in the near future. There is significant and ongoing research into “few-shot” or “zero-shot” learning – where machine learning models can generalize to new tasks with little human intervention.Footnote 66 If carefully constructed, such a TAR system could reduce costs and build trust in the modern discovery process. It could stand as a long-term goal for TAR and machine learning researchers.
It’s well known that, in US civil litigation, the haves come out ahead.Footnote 1 For a slew of reasons – including their ready access to specialists, low start-up costs, and ability to play for rules (not just immediate wins) – well-heeled, repeat-play litigants tend to fare better than their one-shot opponents.
But look closely at data, and it seems that the tilt of the civil justice system may be getting steeper. In 1985, the plaintiff win rate in civil cases litigated to judgment in federal court was a more-than-respectable 70 percent. In recent decades, that figure has hovered at or below 40 percent.Footnote 2 Meanwhile, there’s state-level evidence that when plaintiffs win, they recover less. According to the Bureau of Justice Statistics, the median jury award in state court civil cases was $72,000 in 1992 but only $43,000 in 2005 – a drop (in inflation-adjusted dollars) of 40.3 percent.Footnote 3
The composition of the country’s civil dockets is also telling – and increasingly skewed. Among civil cases, debt collection claims, which typically feature a repeat-play debt collector against a one-shot debtor, are on the rise. According to Pew Charitable Trusts: “From 1993 to 2013, the number of debt collection suits more than doubled nationwide, from less than 1.7 million to about 4 million, and consumed a growing share of civil dockets, rising from an estimated 1 in 9 civil cases to 1 in 4.”Footnote 4 By contrast, tort cases – the prototypical claim that pits a one-shot individual plaintiff against a repeat-play (corporate or governmental) defendant – are falling fast. Personal injury actions accounted for roughly 20 percent of state civil caseloads in the mid-1980s.Footnote 5 Now they make up a measly 4 percent.Footnote 6
What might explain these trends? Possible culprits are many. Some of the tilt might be explained by shifts in the composition of case flows, toward cases where plaintiffs tend to fare poorly (prisoner rights litigation, for example).Footnote 7 Changes in state and federal judiciaries – perhaps part and parcel of increasingly politicized state and federal judicial selection processes – might also matter. Souring in juror sentiment – traceable to the public’s relentless exposure to tales of “jackpot justice” and frivolous claiming – has played a role.Footnote 8 And judges’ day-to-day conduct has changed. Embracing “managerial judging,” judges oversee trials differently than they did in days of yore, and there are hints that certain types of hands-on intervention – time limits, bifurcation, and restrictions on voir dire – might have a pro-defendant cast.Footnote 9
Beyond this menu of possibilities, more cases than ever are now being formally resolved, not through trial, but through pre-trial adjudications – and this tends to benefit defendants. Following the Supreme Court’s creation of a plausibility standard in Bell Atlantic Corp. v. Twombly and Ashcroft v. Iqbal, motions to dismiss are on the rise.Footnote 10 Adjudication via Rule 56 has also trended upward. In 1975, more than twice as many cases were resolved by trial as were resolved by summary judgment.Footnote 11 Now the ratio of cases resolved in federal courts by summary judgment versus trial is heavily skewed toward the former, perhaps on the order of six-to-one.Footnote 12
Finally, substantive law has become less congenial to plaintiffs. At the federal level, the Private Securities Litigation Reform Act and the Prison Litigation Reform Act, among others, make life harder for plaintiffs.Footnote 13 Alongside Congress, the Supreme Court has issued a raft of defendant-friendly decisions – tightening standing, restricting expert testimony, eliminating aider and abettor liability, expanding the preemptive effect of regulatory activity, curbing punitive damages, shunting claims to arbitration, and limiting class certification.Footnote 14 State legislatures, too, have enacted significant tort reform measures, including damage caps, restrictions on contingency fees, alterations to the collateral source rule and joint and several liability, medical malpractice screening panels, and extensions of statutes of repose.Footnote 15
Enter legal tech. Surveying this altered civil justice ecosystem, some suggest that legal tech can be a savior and great leveler, with the capacity to “democratize” litigation and put litigation’s haves and have-nots on a more equal footing.Footnote 16 It can do this, say its champions, by empowering smaller firms and solo practitioners to do battle with their better-financed foes.Footnote 17 Additionally, legal tech might cut the cost of legal services, putting lawyers within reach of a wider swath of people, including those currently priced out of the legal services marketplace.Footnote 18 Meanwhile, even when Americans do go it alone, other legal tech advances – including tools that help write or interpret contracts or resolve low-level consumer disputes – might help them to enter the litigation arena with more information, and possibly more leverage, than before.Footnote 19
We see things differently. We agree that tech tools are coming. We also agree that some of these tools may pay dividends on both sides of the “v.,” promoting transparency, efficiency, access, and equity. But other, arguably more powerful, tools are also here. And many of the most potent are, and are apt to remain, unevenly distributed. Far from democratizing access to civil justice and leveling the playing field, the innovation ecosystem will, at least over the near- to medium-term, confer yet another powerful advantage on the haves. Powerful repeat players, leveraging their privileged access to data (especially confidential claim-settlement data) and their ability to build the technical know-how necessary to mine and deploy that data, will propel themselves yet further ahead.
The remainder of this chapter unfolds as follows. To ground our analysis, Section 6.1 canvasses legal tech, not in a hazy distant future, but in the here and now. In particular, Section 6.1 details three legal tech innovations: (1) the algorithmic e-discovery tools that fall under the umbrella of technology-assisted review, or TAR; (2) Colossus, a claim assessment program that, for two decades, has helped the nation’s largest auto insurers to expeditiously (though controversially) resolve bodily injury claims; and (3) what we call, for lack of a better term, the Walmart Suite, a collection of increasingly sophisticated tools developed by tech companies and BigLaw firms, working in tandem, to rationalize the liability of large corporations in recurring areas of litigation such as slip-and-falls and employment disputes. All three AI-powered tools are already in use. And all three hold the potential to affect the civil justice system in significant (though often invisible) ways.
Section 6.2 steps back to evaluate these innovations’ broader impact. Here, our assessment of TAR is mixed – and contingent. Fueled by TAR, litigation discovery may, over time, emerge more transparent, more efficient, and more equitable than before. This improved equilibrium is by no means assured, and, as we explain below, bleaker outcomes are also possible. But one can at least glimpse, and argue about, a range of first- and second-best outcomes, where more relevant documents are produced, at lower cost, at faster speed, and with less gamesmanship.
Our assessment of Colossus and the Walmart Suite is more dour. Colossus shows that, using increasingly sophisticated data science tools, repeat players are already using their tech savvy and their stranglehold on confidential claims data to drive case settlements downward. With Colossus, insurers are reportedly able to settle auto accident injury cases for roughly 20 percent less than they did before adopting the software. Meanwhile, the Walmart Suite shows that well-heeled repeat players are not just dipping their toes into the litigation waters; they are already in deep – and, in fact, are already able to settle out unfavorable cases and litigate winners, fueling a dynamic we call the “litigation of losers.” As strong cases are culled from the system via early resolution and only the weak proceed to visible, public adjudication, the litigation of losers threatens to further skew the evolution of damage determinations and substantive law.
A final Section 6.3 asks how judges, scholars, and policy makers ought to respond. We consider, and mostly reject, three possible paths forward: reforms to substantive or procedural law, a broad democratization of data, and “public option” legal tech. These fixes, we suggest, are facially attractive but ultimately infeasible or unachievable. Instead, absent a softening of partisan gridlock or renewed public appetite for reform, it is judges, applying ordinary procedural law, who will be the frontline regulators of a newly digitized litigation ecosystem. And, in classic common law fashion, they’ll need to make it up as they go, with only a few ill-fitting tools available to blunt legal tech’s distributive effects.
6.1 Three Examples: TAR, Colossus, and the Walmart Suite
Despite futurist talk of robo-judges and robo-lawyers, litigation systems have always been, in an abstract sense, just machines for the production of dispute resolution. There are inputs (case facts, law) and outputs (judgments, or settlements forged in their shadow). To that extent, the myriad complex procedures that govern civil litigation – that sprawling menu of commands, practices, and norms – are, at their core, just rules that shape the acquisition, exchange, and cost of information as litigants jockey for advantage.
With this framing in mind, few could deny that legal tech tools will have a significant effect on the civil justice system. But how, exactly, will the civil justice system change, in response to the tools’ adoption?
To gain leverage on that question, we offer three real-world examples of a growing array of legal tech tools that supplement and supplant lawyers’ work: (1) new algorithmic e-discovery tools that, as already noted, pass under the label of technology-assisted review, or TAR; (2) Colossus, the go-to claim-pricing tool used by the nation’s casualty and property insurers; and (3) a cutting-edge set of tools we dub the Walmart Suite that both generates pleadings and papers and predicts case outcomes in certain recurring areas of litigation.
6.1.1 Technology-Assisted Review (TAR)
Used by lawyers on both sides of the “v.,” TAR refers to software designed to streamline and simplify the classification and review of documents, primarily through the use of machine-learning techniques.
Though TAR tools vary in their construction and algorithmic particulars, most operate with some human supervision. Virtually all require lawyers to hand-code, or “label,” a subset of a corpus of documents for relevance or privilege (the “seed set”). Then, those documents are used to train a machine-learning system to categorize additional documents. This process is iterative and may repeat over multiple rounds of labeling and training, until lawyers are satisfied that all documents have been correctly categorized.Footnote 20
Even the most basic forms of TAR represent a big leap from its predecessors. Prior to TAR’s advent, document discovery required lawyers and their non-lawyer staffs to hunch over bankers’ boxes or filing cabinets, and then, in time, to manually flip through scanned documents on computer screens, reviewing thousands or even millions of documents one-by-one.Footnote 21 Not surprisingly, the cost of this hands-on review was exorbitant; in 2000, it was estimated that discovery accounted for as much as one-third to one-half of total costs where discovery was actively conducted, and perhaps significantly more in large-scale litigations.Footnote 22
In the early aughts, both keyword searches and outsourcing came to the fore to address some of the above. But neither proved wholly satisfactory. Keyword searching enabled parties to cut costs by restricting manual review to only those documents containing specific keywords, but search yields were worryingly incomplete.Footnote 23 Outsourcing – the move to send discovery to less-expensive contract lawyers in out-of-the-way US cities or abroad – was similarly fraught. Supervision was difficult; parties fretted about conflicts, confidentiality, and rules of multijurisdictional practice; and quality was wanting.Footnote 24
As against those halfway innovations, TAR’s advantages are profound. Estimates of TAR’s efficacy vary and are hotly contested, but the general view is that implemented well – and this is a key qualifier – TAR systems are as good as manual, eyes-on review in terms of recall (i.e., the proportion of relevant documents in the total pool of documents that are accurately identified as relevant) but systematically better in precision (i.e., the proportion of documents flagged that are in fact relevant). The far bigger difference is efficiency: Compared to its conventional counterpart, TAR achieves all of this at a fraction of the cost.Footnote 25
Yet, TAR is not without controversy. Much of it stems from the fact that TAR, like any machine learning system, is a socio-technical “assemblage,” not a turnkey engine.Footnote 26 Attorneys must label and re-label documents as the system works its way toward a reliable model. An important implication is that, much like Colossus (described below), TAR systems are manipulable by humans in their construction and tuning.Footnote 27 As Diego Zambrano and co-authors detail elsewhere in this volume, this manipulation can run the gamut from outright abuse (e.g., fudging the labels lawyers apply to document labelsFootnote 28 or rigging the selection, adjustment, or validation of modelsFootnote 29) to a more benign but still respondent-friendly calibration of the system to favor precision (the proportion of responsive documents among those in a production) over recall (the proportion of responsive documents identified).Footnote 30 As a result, and as discussed in more detail below, if litigation’s “haves” need not show their work to the other side, they can shade discovery to their advantage and use their better technology and technologists (if the other side can afford them at all) to make sure it sticks.Footnote 31
6.1.2 Colossus
For the nation’s casualty and property insurers, AI has not so much spawned new litigation tools as supercharged those already in use. The best example is Colossus, a proprietary computer software program marketed by Computer Science Corporation (CSC) that “relies on 10,000 integrated rules” to assist insurance companies – the ultimate repeat players – in the evaluation and resolution of bodily injury claims.Footnote 32 Initially developed in Australia and first used by Allstate in the 1990s, Colossus has grown in popularity, such that it has been utilized by the majority of large property and casualty insurers in the United States, including behemoths Aetna, Allstate, Travelers, Farmers, and USAA.Footnote 33
Colossus has radically changed the process of auto accident claims adjustment. By extension, it has profoundly altered how the tens of thousands of third-party bodily injury claims generated annually by American drivers, passengers, and pedestrians are processed and paid by US insurers.
Before Colossus, an experienced auto accident adjuster employed by Allstate or USAA would have assessed a personal injury claim using rough benchmarks, in a process that was more art than science. Namely, the adjuster would add up a victim’s “special damages” (chiefly, the victim’s medical bills) and multiply those by a fixed sum – often, two or three – to generate a default figure, called a “going rate” or “rule of thumb.”Footnote 34 Then, the adjuster would leaven that default figure with the adjuster’s knowledge and past practice, perhaps informed by a review of recent trial verdict reports, and possibly aided by “roundtabling” among the insurer’s veteran casualty claims professionals.Footnote 35
With Colossus, however, the same adjuster can now calculate a claim’s worth at a keystroke, after plugging in answers to a series of fill-in-the-blank-style questions. Or, as Colossus itself explains: “Through a series of interactive questions, Colossus guides your adjusters through an objective evaluation of medical treatment options, degree of pain and suffering, and the impact of the injury on the claimant’s lifestyle.”Footnote 36 To be sure, the data an adjuster must input in order to prime Colossus to generate a damage assessment is voluminous and varied. When inputting a claim, the adjuster accounts for obvious factors such as the date and location of the accident, alongside the claimant’s home address, gender, age, verified lost wages, documented medical expenses, nature of injury, diagnosis, and prognosis. Treatment – including MRI or X-ray images, prescriptions, injections, hospital admissions, surgeries, follow-up visits, and physical therapy – is also granularly assessed.Footnote 37 Then, against these loss variables, the adjuster must account for various liability metrics. Fault (in all its common law complexity) is reduced to “clear” or “unclear,” while the existence or nonexistence of “aggravating factors” (such as driver inebriation) is also considered, and, in a nod to the tort doctrine of anticipatory avoidable consequences, the adjuster must also input whether the claimant was buckled up.Footnote 38 Even the individual identity of the handling attorney, treating physician and/or chiropractor, and (if applicable) presiding judge is keyed in.Footnote 39
Once data entry is complete, Colossus assesses the claim in light of the enormous pool of data in its master database to generate a “severity point total.”Footnote 40 Then, aided by particularized, proprietary information that is specific to each insurer (based on each individual insurer’s “settlement philosophies and claims practice”Footnote 41), Colossus converts the point total into a recommended settlement range.Footnote 42 Insurance adjusters use this settlement range in their negotiations with unrepresented claimants or their counsel. Indeed, at some insurers, adjusters are not permitted to offer a sum outside the range, at least without a supervisor’s approval.Footnote 43 At others, adjusters are evaluated based on their ability to close files within Colossus-specified parameters.Footnote 44 In so doing, according to one insider: “Colossus takes the guess work out of an historically subjective segment of the claims process, providing adjusters with a powerful tool for improving claims valuation, consistency, increasing productivity and containing costs.”Footnote 45
Beyond these, allegations about further operational details abound. The most common is that, when customizing the software (the proprietary process that converts a “severity point total” into a settlement range), certain insurers “tune” Colossus to “consistently spit out lowball offers.”Footnote 46 Some insurers reportedly accomplish this feat by excluding from the database certain figures that, by rights, should be included (e.g., large settlements or verdicts).Footnote 47 Others get there, it is said, simply by turning dials downward to generate across-the-board haircuts of 10–20 percent.Footnote 48
As such, it appears that, in the hands of at least some insurers, Colossus has not only rationalized the resolution of personal injury claims and injected newfound objectivity, predictability, and horizontal equity into the claims resolution process. It has also systematically cut claims – to the benefit of repeat-play insurers and the detriment of their claimant-side counterparts.
6.1.3 The Walmart Suite
A third innovation combines elements of both TAR and Colossus. One exemplar under this umbrella, which we dub “the Walmart Suite,” given its development by Walmart in partnership with the law firm Ogletree Deakins and in concert with the tech company LegalMation, seeks to rationalize recurrent areas of litigation (think, employment disputes and slip-and-falls). It reportedly operates along two dimensions.Footnote 49 First, it reportedly generates pleadings and papers – including answers, discovery requests, and discovery objections – thus cutting litigation costs.Footnote 50 To that extent, the Suite might be thought akin to TAR in its ability to perform low-level legal cognitions and generate straightforward work product that previously required (human) lawyers. Second, and more provocatively, the Suite can evaluate key case characteristics, including the identity of plaintiffs’ counsel, and then offer a prediction about a case’s outcome and the likely expense Walmart will incur if the case is litigated, rather than settled.Footnote 51 The Suite thus seems to be a beefed-up Colossus, with a focus on slip-and-falls and employment disputes rather than auto accidents.
The advantages of such tools are seemingly substantial. LegalMation reports that a top law firm has used its tools to handle 5,000 employment disputes – and, in so doing, the firm realized a six- to eight-fold savings in preparing pleadings and discovery requests.Footnote 52 But these economies are only the beginning. Outcome prediction engines, commonly referred to as the “holy grail” of legal tech,Footnote 53 allow large entities facing recurring types of litigation to quickly capitulate (via settlement) where plaintiffs have the benefit of strong claims and talented counsel – and then battle to final judgment where plaintiffs are saddled with weak claims or less-competent counsel. In so doing, the Walmarts of the world can save today by notching litigation victories while conserving litigation resources. But they can simultaneously position themselves over the long haul, by skewing case outcomes, driving down damages, and pushing precedent at the appellate level. We return to these advantages below.
6.2 The Promise and Peril of Legal Tech
Section 6.1 introduced three types of legal tools that have already entered the civil justice system. These tools – TAR, Colossus, and the Walmart Suite – are hardly the only legal tech applications dotting the American litigation landscape. But they help to define it, and they also permit some informed predictions about legal tech’s effect on the litigation playing field over the near- to medium-term.
Assessing these effects, this Section observes that TAR may help to level the litigation playing field and could even bring greater transparency to discovery disputes – although such a rosy result is by no means assured, and darker outcomes are also possible. Meanwhile, Colossus and the Walmart Suite both seem poised to drive case settlements downward and even fuel a dynamic we call the “litigation of losers,” in part because the data stores that drive them are, at least currently, so unevenly distributed.
6.2.1 TAR Wars: Proportionality and Discovery Abuse
For TAR, our appraisal is mixed – though the dynamics at play are not simple and our predictions less than ironclad. That said, we predict that the next decade will feature increasingly heated “TAR wars” waged on two fronts: proportionality and discovery gaming and abuse. If, on each front, there is sufficient judicial oversight (an admittedly big if), TAR might usher in a new era, where discovery emerges more efficient and transparent than before. But there is also the possibility that, like Colossus and the Walmart Suite, TAR will tilt the playing field toward repeat-play litigants. Here, we address these two fronts – and also these two divergent possible outcomes.
Proportionality: Will TAR’s efficiencies justify more expansive discovery? Or will these efficiencies yield a defendant-side surplus? Discovery has long been the 800-pound gorilla in the civil justice system, accounting for as much as one-third to one-half of all litigation costs in cases where discovery is actively employed.Footnote 54 High discovery costs, and the controversy surrounding those costs, have powered the creation of numerous rules and doctrines that constrain discovery’s scope.Footnote 55 One such rule – and the one we address here – is the “proportionality” requirement, that is, a requirement that a judge greenlight a discovery request only if the request is “proportional” to a case’s particular needs.Footnote 56
Applied to TAR, proportionality is tricky because TAR can yield gains in both efficiency and accuracy. For a requesting party (typically, the plaintiff), more efficient review justifies more expansive review, including document requests that, for instance, extend to a longer time horizon or to a wider net of document custodians. For a producing party (typically the defendant), however, accuracy gains mean that the requesting party will already get more relevant documents and fewer irrelevant ones, even holding constant the number of custodians or the scope of the search.Footnote 57 In short, TAR generates a surplus in both efficiency and accuracy, and the question becomes how best to allocate that surplus.Footnote 58
Given these dynamics, judges might employ the proportionality principle in one of two ways. Judges could recognize that the unit cost of discovery – the cost of each produced document – has declined and compensate by authorizing the requesting party’s more expansive discovery plan. If so, the cost of each produced document will drop, transparency into the underlying incident will (at least arguably) improve, and the overall cost of discovery will remain (roughly) constant. Judges, however, might take a different tack. Notwithstanding TAR’s efficiency advantages, judges might deny requesting parties’ motions to permit more expansive discovery, thus holding proportionality’s benchmarks firm. If so, TAR will cough up the same documents as before, but at a discount.
If trial judges permit producing parties to capture TAR’s cost-savings without compensating by sanctioning more sweeping discovery plans, the effect on civil litigation, from the availability of counsel to settlement patterns, could be profound.Footnote 59 Lower total discovery costs, of course, might be a net social welfare gain. After all, a core premise of proportionality rules is that litigation costs, particularly discovery costs, are disproportionate to the social value of the dispute resolution achieved, and scarce social resources might be better spent on other projects. But shifts in discovery costs can also have distributive consequences. It is a core premise of litigation economics that “all things being equal, the party facing higher costs will settle on terms more favorable to the party facing lower costs.”Footnote 60 If TAR causes discovery costs to bend downward, TAR’s surplus – and, with it, any settlement surplus – will systematically flow toward the net document producers (again, typically, repeat-play defendants).Footnote 61 Such an outcome would yield a tectonic shift in the settlement landscape – hard to see in any particular case, but potentially quite large in aggregate. It will be as if Colossus’ dials have been turned down.
The potential for abuse: TAR appears to be more susceptible to abuse than its analog counterpart. How will judges respond? The second TAR battleground will be discovery abuse and gaming. The fight will center on a core question: Can discovery rules generate enough trust among litigants to support TAR’s continued propagation, while, at the same time, mitigating concerns about gaming and the distributive concerns raised by such conduct?
Discovery abuse, of course, is not new. Nor is TAR uniquely vulnerable to discovery abuse.Footnote 62 Indeed, one of the easiest ways to manipulate a TAR system – the deliberate failure to flag (or “label”) a plainly responsive document – is no different from garden-variety discovery manipulation, in which lawyers simply omit obviously responsive and damaging documents or aggressively withhold borderline documents on relevance or privilege grounds. But, as Zambrano and co-authors note in Chapter 5, there is nevertheless good reason to believe that TAR might be especially prone to abuse – and that is a very serious problem in a system already steeped in mistrust.Footnote 63
TAR’s particular vulnerability to abuse flows from four facts. First, TAR operates at scale. In constructing a seed set, a single labeling decision could, in theory, prevent an entire species of document from coming to light.Footnote 64
Second, and relatedly, TAR can be implemented by small teams – much different than the sprawling associate armies who previously performed eyes-on document reviews in complex cases. This means that, in a TAR world, deliberate discovery abuse requires coordination among a smaller set of actors. If discovery abusers can be likened to a cartel, keeping a small team in line is far easier than ensuring that a sprawling network of co-conspirators stays silent. Moreover, TAR leans on, not just lawyers, but technologists – and, unlike the former, the latter might be less likely to take discovery obligations seriously, as they are not regulated by rules of professional conduct, need not participate in continuing legal education, and arguably have a less socialized sense of duty to the public or the court.
Third, TAR methods may themselves be moving toward more opaque and harder-to-monitor approaches. In its original guise – TAR 1.0 – lawyers manually labeled a “seed set” to train the machine-learning model. With access to that “seed set,” a litigation adversary could, in theory, reconstruct the other side’s work, identifying calls that were borderline or seemed apt to exclude key categories of documents. TAR 2.0, by contrast, starts with a small set of documents and uses machine learning to turn up lists of other candidates, which are then labeled and fed back into the system. TAR 2.0 thus renders seed set construction highly iterative – and, in so doing, makes it harder for an adversary or adjudicator to review or reconstruct. TAR 2.0, to invoke a concept in a growing “algorithmic accountability” literature, may, as a consequence, be less contestable by an adversary who suspects abuse.Footnote 65
Fourth and finally, while TAR is theoretically available on both sides of the “v.,” technical capacity is almost certainly unevenly distributed, since defense firms tend to be larger than plaintiffs’ firms – and are more richly capitalized. With these resource advantages, if defendants are tempted to engage in tech-driven litigation abuse, they (and their stable of technologists) might be able to do so with near impunity.
The tough question becomes: How should judges react, to safeguard the integrity of discovery processes? Here, judges have no shortage of tools, but all have drawbacks. Judges can, for example, compel the disclosure of a seed set, although such disclosures are controversial, since the full seed set necessarily includes both documents that lawyers labeled as relevant as well as those irrelevant to the claim.Footnote 66 Meanwhile, disclosure of TAR inputs arguably violates the work product doctrine, established in the Supreme Court’s 1947 decision in Hickman v. Taylor and since baked into Rule 26(b)(3), which protects against disclosure of “documents and tangible things that are prepared in anticipation of litigation.”Footnote 67 And, a call for wholesale disclosure – ever more “discovery about discovery” – seems poised to erode litigant autonomy and can itself be a bare-knuckled litigation tactic, not a good-faith truth-seeking device.
Worse, if analog discovery procedures are left to party discretion absent evidence of specific deficiencies, but a party’s use of TAR automatically kicks off protracted ex ante negotiations over protocols or onerous back-end “report card” requirements based on various quality-control metrics, there is the ever-present risk that this double standard will cause parties to throw up their hands. To the extent TAR’s benefits are overshadowed by expensive process-oriented disputes, investment in TAR will eventually stall out, depriving the system of its efficiencies.Footnote 68 Yet, the opposite approach is just as, if not more, worrisome. If judges, afraid of the above, do not act to police discovery abuse – and this abuse festers – they risk eroding the integrity of civil discovery and, by extension, litigants’, lawyers’, and the public’s faith in civil litigation.
Time will tell if judges can steer between these possibilities. But if they can, then out of these two gloomy visions comes a glimmer of light. If judges can help mint and then judiciously apply evenhanded protocols in TAR cases, then perhaps the system could end up better off than the analog system that TAR will steadily eclipse. Civil discovery could be one of those areas where, despite AI’s famous “black box” opacity, digitization yields a net increase in transparency and accountability.Footnote 69
6.2.2 Colossus and the Walmart Suite: The Litigation of Losers
When it comes to the slant of the civil justice system, an assessment of the likely effect of Colossus and the Walmart Suite is more dour.
Colossus: Reduction via brute force. The impact of Colossus on the civil justice system seems fairly clear and not particularly contingent. Colossus’ advent has certain undeniable benefits, injecting newfound predictability, consistency, objectivity, and horizontal equity into the claims resolution process. It has also, probably, reduced the monies paid for fraudulent or “built” claims,Footnote 70 as well as the odds that claim values will be influenced by improper factors (racial bias, for example).Footnote 71 Finally, it has, possibly, driven down the driving public’s insurance premiums – though there’s little reliable evidence on the point.
But, alongside these weighty advantages, it does seem that Colossus has also reduced claim payments quite significantly, using something like brute force. When Allstate rolled out a new Colossus-aided claims program for Allstate with the help of McKinsey & Co., the consulting firm’s stated goal was to “establish[ ] a new fair market value” for such injuries.Footnote 72 It appears that that aim was achieved. A later McKinsey review of Allstate found: “The Colossus sites have been extremely successful in reducing severities, with reductions in the range of 20 percent for Colossus-evaluated claims.”Footnote 73 Nor was this dynamic confined, necessarily, to Allstate. Robert Dietz, a fifteen-year veteran of Farmer’s Insurance has explained, for example: “My vast experience in evaluating claims was replaced by values generated by a computer. More often than not, these values were not representative of what I had experienced as fair and reasonable.”Footnote 74
The result is that, aided by Colossus, insurance companies are offering less to claimants for comparable injuries, on a take-it-or-leave-it basis. And, though one-shot personal injury (PI) lawyers could call insurance companies’ bluff and band together to reject these Colossus-generated offers en masse, in the past two decades, they haven’t.
Their failure to do so should not be surprising. Given persistent collective action problems and yawning information asymmetries (described in further detail below), one would not expect disaggregated PI lawyers, practicing alone or in small firms, to mount a coordinated and muscular response, especially since doing so would mean taking a significant number of claims to trial, which poses many well-known and formidable obstacles. For instance, some portion of PI lawyers operate in law firms (called “settlement mills”) and do not, in fact, have the capacity to take claims to trial.Footnote 75 Second, many auto accident claimants need money quickly and do not have the wherewithal to wait out attendant trial delays. And third, all PI lawyers are attuned to the stubborn economics of auto accident litigation: As of 2005, the median jury trial award in an auto case was a paltry $17,000, which would yield only about $5,500 in contingency fees, a sum that is simply too meager to justify frequent trials against well-financed foes.Footnote 76 This last point was not lost on McKinsey, which, in a presentation to Allstate, encouraged: “Win by exploiting the economics of the practice of law.”Footnote 77
The Walmart Suite and the litigation of losers. The Walmart Suite illustrates another dynamic, which we dub the “litigation of losers.” In the classic article, Why the Haves Come Out Ahead, Marc Galanter presciently observed that repeat-players could settle out bad cases “where they expected unfavorable rule outcomes” and litigate only the good ones that are “most likely to produce favorable results.” Over time, he concluded, “we would expect the body of ‘precedent’ cases, that is, cases capable of influencing the outcome of future cases – to be relatively skewed toward those favorable to [repeat players].”Footnote 78
The Walmart Suite shows that Galanter’s half-century-old prediction is coming to pass, fueled by AI-based software he couldn’t have imagined.Footnote 79 And, we anticipate, this isn’t the end of it. In recurring areas of litigation, we are likely to see increasingly sophisticated outcome prediction tools that will draw ever-tighter uncertainty bands around anticipated outcomes. Like the Walmart Suite, these tools are reliant on privileged access to confidential claim settlement data, which only true repeat players will possess.
The effect of this evolution is profound, for, as outcome prediction tools percolate (at least in the hands of repeat defendants/insurers), only duds will be litigated – and this “litigation of losers” will skew – indeed, is almost certainly already skewing – the development of substantive law. The skew will happen because conventional wisdom, at least, holds that cases settle in the shadow of trial – which means that, to the extent trial outcomes tilt toward defendants, we would expect that settlements, too, will display a pro-defendant slant.Footnote 80 Damages will also be affected. To offer but one concrete example, in numerous states, a judge evaluates whether damages are “reasonable” by assessing what past courts have awarded for similar or comparable injuries.Footnote 81 To the extent the repository of past damages reflects damages plaintiffs have won while litigating weak or enfeebled claims, that repository will, predictably, bend downward, creating a progressively more favorable damages environment for defendants.
To be sure, there are caveats and counter-arguments. Models of litigation bargaining suggest that a defendant with privileged information will sometimes have incentives to share that information with plaintiffs in order to avoid costly and unnecessary litigation and achieve efficient settlements.Footnote 82 Additionally, while repeat players have better access to litigation and settlement data, even one-shotters don’t operate entirely in the dark.Footnote 83 But a simple fact remains: Even a slow burn of marginally better information, and marginally greater negotiation leverage, can have large aggregate effects across thousands and even millions of cases.
6.3 What to Do?
As the litigation playing field tilts under legal tech’s weight, there are some possible responses. Below, we start by briefly sketching three possible reforms that are facially plausible but, nevertheless, in our view, somewhat infeasible. Then, we offer a less attractive option – judicial discretion applied to existing procedural rules – as the most likely, though bumpy, path forward.
6.3.1 Plausible but Unlikely Reforms
Rewrite substantive or procedural law. First, we could respond to the skew that legal tech brings by recalibrating substantive law. Some combination of state and federal courts and legislatures could, for example, relax liability standards (for instance, making disparate impact job discrimination easier to prove), loosen restrictions on punitive damages, repeal damage caps, return to a world of joint and several liability, restore aider-and-abettor liability, abolish qualified immunity, and resurrect the collateral source rule.
Whatever the merit of a substantive law renaissance, we are, however, quite bearish on the possibility, as the obstacles blocking such an effort are formidable and, over the near- to medium-term, overwhelming. Substantive laws are sticky and salient, especially in a political system increasingly characterized by polarization and legislative gridlock.Footnote 84 Even in less-polarized subfederal jurisdictions, it would be hard to convince state legislators and (often elected) judges to enact sweeping reforms without strong support from a public that often clings to enduring but often misguided beliefs about “jackpot justice” and frivolous claiming.Footnote 85
Nor are federal courts, or a Supreme Court, newly stocked with Trump-era appointees, likely to help; to the contrary, they are likely to place barriers in front of litigation-friendly legislative efforts.Footnote 86 And procedural rules, though less politically salient, will also be hard to change, particularly at the federal level given the stranglehold of conservative judges and defense-side lawyers on the process of court-supervised rulemaking.Footnote 87
Democratize the data. Second, we could try to recalibrate the playing field by expanding litigants’ access to currently confidential data. As it stands, when it comes to data regarding the civil justice system, judges, lawyers, litigants, and academics operate almost entirely in the dark. We do not know how many civil trials are conducted each year. We don’t know how many cases go to trial in each case category. And, we don’t know – even vaguely – the outcome of the trials that do take place.Footnote 88 Furthermore, even if we could know what happens at trial (which we don’t) or what happens after trial (which we don’t), that still wouldn’t tell us much about the much larger pool of claims that never make it to trial and instead are resolved consensually, often before official filing, by civil settlements.
This is crucial, for without information about those millions of below-the-radar settlements, the ability to “price” a claim – at least to “price” a claim using publicly available data, approaches zero. As Stephen Yeazell has aptly put it:
[I]n the U.S. at the start of the twenty-first century, citizens can get reliable pricing information for almost any lawful transaction. But not for civil settlements. We can quickly find out the going price of a ten-year old car, of a two-bedroom apartment, or a souvenir of the last Superbowl, but one cannot get a current “market” quote for a broken leg, three weeks of lost work, and a lifetime of residual restricted mobility. Nor for any of the other 7 million large or the additional 10 million smaller civil claims filed annually in the United States. We simply do not know what these are worth.Footnote 89
Recognizing this gap, Computer Sciences Corp. (the maker of Colossus) and Walmart and its tech and BigLaw collaborators are working to fill it. But, they have filled it for themselves – and, in fact, they have leveraged what amounts to their near-total monopoly on settlement data to do so. Indeed, some insurers’ apparent ability to “tune” Colossus rests entirely on the fact that plaintiffs cannot reliably check insurance companies’ work – and so insurers can, at least theoretically, “massage” the data with near impunity.
Seeing the status quo in this light, of course, suggests a solution: We could try to democratize the data. Taking this tack, Yeazell has advocated for the creation of electronic databases whereby basic information about settlements – including, for instance, the amount of damages claimed, the place suit was filed, and the ultimate settlement amount – would be compiled and made accessible online.Footnote 90 In the same vein, one of us has suggested that plaintiffs’ attorneys who work on a contingency fee basis and seek damages in cases for personal injury or wrongful death should be subject to significant public disclosure requirements.Footnote 91
Yet, as much as democratizing the data sounds promising, numerous impediments remain – some already introduced above. The first is that many “cases” are never actually cases at all. In the personal injury realm, for example, the majority of claims – in fact, approximately half of claims that involve represented claimants – are resolved before a lawsuit is ever filed.Footnote 92 Getting reliable data about these settlements is exceptionally difficult. Next, even when cases are filed, some high proportion of civil cases exit dockets via an uninformative voluntary dismissal under Rule 41 or its state-level equivalents.Footnote 93 Those filings, of course, may be “public,” but they reveal nothing about the settlement’s monetary terms.Footnote 94 Then, even on those relatively rare occasions when a document describing the parties’ terms of settlement is filed with the court, public access remains limited. Despite a brewing “open court data” movement, court records from the federal level on down sit behind “walls of cash and kludge.”Footnote 95 Breaking through – and getting meaningful access even to what is “public” – is easier said than done.
“Public option” legal tech. A third unlikely possibility is “public option” legal tech. Perhaps, that is, the government could fund the development of legal tech tools and make them widely available.
When it comes to TAR, public option legal tech is not hard to imagine. Indeed, state and federal judiciaries already feature magistrate judges who, on a day-to-day basis, mainly referee discovery disputes. It may only be a small step to create courthouse e-discovery arms, featuring tech-forward magistrate judges who work with staff technologists to perform discovery on behalf of the parties.
Public option outcome-prediction tools that can compete with Colossus or the Walmart Suite are harder to imagine. Judges, cautious Burkeans even compared to the ranks of lawyers from which they are drawn, are unlikely to relax norms of decisional independence or risk any whiff of prejudgment anytime soon. The bigger problem, however, will be structural, not just legal-cultural. The rub is that, like one-shot litigants and academics, courts lack access to outcome data that litigation’s repeat players possess. Short of a sea change in the treatment of both pre- and post-suit secret settlements, courts, no less than litigation’s have-nots, will lack the information needed to power potent legal tech tools.Footnote 96
6.3.2 Slouching Toward Equity: Judicial Procedural Management with an Eye to Technological Realities
Given the above obstacles, the more likely (though perhaps least attractive) outcome is that judges, applying existing procedural rules, will be the ones to manage legal tech’s incorporation into the civil justice system. And, as is often the case, judges will be asked to manage this tectonic transition with few rules and limited guidance, making it up mostly as they go.
The discussion of TAR’s contingent future, set forth above in Part 6.2.2 offers a vivid depiction of how courts, as legal tech’s frontline regulators, might do so adeptly; they might consider on-the-ground realities when addressing and applying existing procedural doctrines. But the TAR example also captures a wider truth about the challenges judges will face. As already noted, legal tech tools that cut litigation costs and hone information derive their value from their exclusivity – the fact that they are possessed by only one side. It follows that the procedural means available to judges to blunt legal tech’s distributive impacts will also reduce the tools’ value and, at the same time, dull incentives for litigants to adopt them, or tech companies to develop them, in the first instance. As a result, disparate judges applying proportionality and work product rules in individual cases will, inevitably, in the aggregate, create innovation policy. And, for better or worse, they will make this policy without the synoptic view that is typically thought essential to making wise, wide-angle judgments.
Judicial management of legal tech’s incorporation into the civil justice system will require a deft hand and a thorough understanding of changing on-the-ground realities. To offer just one example: As noted above, discovery cost concerns have fueled the creation of a number of doctrines that constrict discovery and, in so doing, tend to make life harder for plaintiffs. These include not just Rule 26’s “proportionality” requirement (described above), but also a slew of other tweaks and outright inventions, noted previously, from tightened pleading standards to court-created Lone Pine orders that compel plaintiffs to offer extensive proof of their claims, sometimes soon after filing.
Undergirding all these restrictive doctrines is a bedrock belief: that discovery is burdensome and too easily abused, so much so that it ought to be rationed and rationalized. Yet, as explained above, TAR has the potential to significantly reduce the burden of discovery (particularly, as noted above, if more expansive discovery, which might offset certain efficiency gains, is not forthcoming). As such, TAR, at least arguably, will steadily erode the very foundation on which Twombly, Iqbal, and Lone Pine orders rest – and this newly unsettled foundation might, therefore, demand the reexamination of those doctrines. As judges manage the incorporation of potent new legal tech tools into the civil justice system, we can only hope that they will exhibit the wisdom to reconsider, where relevant, this wider landscape.
6.4 Conclusion
This chapter has argued that the civil justice system sits at a contingent moment, as new digital technologies are ushered into it. While legal tech will bring many benefits and may even help to level the playing field in certain respects, some of the more potent and immediately available tools will likely tilt the playing field, skewing it ever further toward powerful players. One can imagine numerous fixes, but the reality is that, in typical common law fashion, the system’s future fairness will depend heavily on the action of judges, who, using an array of procedural rules built for an analog era will, for better or worse, make it up as they go. We’re not confident about the results of that process. But the future fairness of a fast-digitizing civil justice system might just hinge on it.
The United States has a serious and persistent civil justice gap. In 1994, an American Bar Association study found that half of low- and moderate-income households had faced at least one recent civil legal problem, but only one-quarter to one-third turned to the justice system.Footnote 1 Twenty-four years later, a 2017 study by the country’s largest civil legal aid funder found that 71 percent of low-income households surveyed had experienced a civil legal need in the past year, but 86 percent of those problems received “inadequate or no legal help.”Footnote 2 Studies in individual states tell a similar story.Footnote 3
Unmet civil legal needs include a variety of high-stakes case types that affect basic safety, stability, and well-being: domestic violence restraining orders; health insurance coverage disputes; debt collection and relief actions; evictions and foreclosures; child support and custody cases; and education- and disability-related claims.Footnote 4 There is generally no legal right to counsel in these cases, and there are too few lawyers willing and able to offer representation at prices that low- and middle-income clients can afford.Footnote 5 In my home state of Georgia, for example, five or six rural counties – depending on the year – have no resident attorneys, and eighteen counties have only one or two.Footnote 6 These counties’ upper-income residents travel to the state’s urban centers for legal representation. Lower-income residents seek help from rotating legal aid lawyers who “ride circuit,” meeting clients for, say, two hours at the public library on the first Wednesday of the month.Footnote 7 Or they go without.
Can computationally driven litigation outcome prediction tools fill the civil justice gap? Maybe.
This chapter reviews the current state of outcome prediction tools and maps the ways they might affect the civil justice system. In Section 7.1, I define “computationally driven litigation outcome prediction tools” and explain how they work to forecast outcomes in civil cases. Section 7.2 outlines the theory: the potential for such tools to reduce uncertainty, thereby reducing the cost of civil legal services and helping to address unmet legal needs. Section 7.3 surveys the work that has been done thus far by academics, in commercial applications, and in the specific context of civil legal services for low- and middle-income litigants. Litigation outcome prediction has not reached maturity as a field, and Section 7.4 catalogs the data, methodological, and financial limits that have impeded development in general and the potential to expand access to justice in particular.
Section 7.5 steps back and confronts the deeper effects and the possible unintended consequences of the tools’ continued proliferation. In particular, I suggest that, even if all the problems identified in Section 7.4 can be solved and litigation outcome prediction tools can be made to work perfectly, their use raises important endogeneity concerns. Computationally driven tools might reify previous patterns, lock out litigants whose claims are novel or boundary-pushing, and shut down the innovative and flexible nature of common law reasoning. Section 7.6 closes by offering a set of proposals to stave off these risks.
Admittedly, the field of litigation prediction is not yet revolutionizing civil justice, whether for good or ill. Empirical questions remain about the way(s) that outcome prediction might affect access to justice. Yet if developments continue, policy makers and practitioners should be ready to exploit the tools’ substantial potential to fill the civil justice gap while also guarding against the harms they might cause.
7.1. Litigation Outcome Prediction Defined
I define “computationally driven litigation outcome prediction tools” as statistical or machine learning methods used to forecast the outcome of a civil litigation event, claim, or case. A litigation event may be a motion filed by either party; the relevant predicted outcome would be the judge’s decision to grant or deny, in full or in part. A claim or case outcome, on the other hand, refers to the disposition of a lawsuit, again in full or in part. My scope is civil only, though much of the analysis that follows could apply equally to criminal proceedings.
“Computationally driven” here refers to the use of statistical or machine learning models to detect patterns in past civil litigation data and exploit those patterns to predict, and to some extent explain, future outcomes. Just as actuaries compute the future risk of loss for insurance companies based on past claims data, so do outcome prediction tools attempt to compute the likelihood of future litigation events based on data gleaned from past court records.
In broad strokes, such tools take as their inputs a set of characteristics, also known as predictors, independent variables, or features, that describe the facts, legal claims, arguments, and authority, the people (judge, lawyers, litigants, expert witnesses), and the setting (location, court) of a case. Features might also come from external sources or be “engineered” by combining data. For example, the judge’s gender and years on the bench might be features, as well as the number of times the lawyers in the case had previously appeared before the same judge, the judge’s caseload, and local economic or crime data. Such information might be manually or computationally extracted from the unstructured text of legal documents and other sources – necessitating upstream text mining or natural language processing tasks – or might already be available in structured form.
These various features or case characteristics then become the inputs into one of many types of statistical or predictive models; the particular litigation outcome of interest is the target variable to be predicted.Footnote 8 When using such a tool, a lawyer would plug in the requested case characteristics and would receive an outcome prediction along with some measurement of error.
7.2. Theory: Access-to-Justice Potential
In theory, computationally driven outcome prediction, if good enough, can supplement, stretch, and reduce the cost of legal services by reducing outcome uncertainty. As Gillian Hadfield summarizes, uncertainty comes from several sources.Footnote 9 Sometimes the law is simply unclear. Other times, actors, whether police officers, prosecutors, regulators, or courts, have deliberately been given discretion. Further, an individual may subjectively discount or increase the probability of liability due to “mistakes in the determination of factual issues, and errors in the identification of the applicable legal rule.”Footnote 10 One way to resolve these uncertainties is to pay a lawyer for advice – in particular, a liability estimate.
Given a large enough training set, a predictive model may detect patterns in how courts have previously resolved vagueness and how officials have previously exercised discretion. Further, such a tool could correct the information deficits and asymmetries that may produce mistaken liability estimates. Outcome prediction tools might also obviate the need for legal representation entirely, allowing potential and actual litigants to estimate their own chances of success and proceed pro se. This could be a substantial boon for access to justice. Of course, even an outcome-informed pro se litigant may fail to navigate complex court procedures and norms successfully.Footnote 11 Fully opening the courthouse doors to self-represented litigants might also require simplification of court procedures. Still, outcome prediction tools might go a long way toward expanding access to justice, whether by serving litigants directly or by acting as a kind of force multiplier for lawyers and legal organizations, particularly those squaring off against better-resourced adversaries.Footnote 12
A second way outcome prediction tools could, in theory, open up access to justice is by enhancing the ability of legal services providers to quantify, and manage, risk. Profit-driven lawyers, as distinguished from government-funded legal services lawyers, build portfolios of cases with an eye toward managing risk.Footnote 13 Outcome prediction tools may allow lawyers to allocate their resources more efficiently, wasting less money on losing cases and freeing up lawyer time and attention for more meritorious cases, or by constructing portfolios that balance lower- and higher-risk cases.
In addition, enterprising lawyers with a higher-risk appetite might use such tools to discover new areas of practice or potential claim types that folk wisdom would advise against.Footnote 14 To draw an example from my previous work, I studied the boom in wage-and-hour lawsuits in the early 2000s and identified as one driver of the litigation spike an influx of enterprising personal injury attorneys into wage-and-hour law.Footnote 15 One early mover was a South Florida personal injury attorney named Gregg Shavitz, who discovered his clients’ unpaid wage claims by accident, became an overtime specialist, and converted his firm into one of the highest-volume wage-and-hour shops in the country. This was before the wide usage of litigation outcome prediction tools. However, one might imagine that more discoveries like Gregg Shavitz’s could be enabled by computationally driven systems, rather than by happenstance, opening up representation for more clients with previously overlooked or under-resourced claim types.Footnote 16
I return to, and complicate, this possibility in Section 7.5, where I raise concerns about outcome prediction tools’ conservatism in defining winning and losing cases, which may reduce, rather than increase, access to justice – empirical questions that remain to be resolved.
7.3 Practice: Where Are We Now?
From theory, I now turn to practice, tracing the evolution and present state of litigation outcome prediction in scholarship, commercial applications, and tools developed specifically to serve low- and middle-income litigants. This Section also begins to introduce these tools’ limitations in their present form, a topic that I explore more fully in Section 7.4.
7.3.1 Scholarship
Litigation outcome prediction is an active scholarly research area, characterized by experimentation with an array of different data sets, modeling approaches, and performance measures. Thus far, no single dominant approach has emerged.
In a useful article, Kevin Ashley traces the history of the field to the work of two academics who used a machine learning algorithm called k-nearest neighbors in the 1970s to forecast the outcome of Canadian real estate tax disputes.Footnote 17 Since then, academic work has flourished. In the United States, academic interest has focused, variously, on decisions by the US Supreme Court,Footnote 18 federal appellate courts,Footnote 19 federal district courts,Footnote 20 immigration court,Footnote 21 state trial courts,Footnote 22 and administrative agencies.Footnote 23 Case types studied include employment,Footnote 24 asylum,Footnote 25 tort and vehicular,Footnote 26 and trade secret misappropriation.Footnote 27 Other scholars outside the United States have, in turn, developed outcome prediction tools focused on the European Court of Human Rights,Footnote 28 the International Criminal Court,Footnote 29 French appeals courts,Footnote 30 the Supreme Court of the Philippines,Footnote 31 lending cases in China,Footnote 32 labor cases in Brazil,Footnote 33 public morality and freedom of expression cases in Turkey’s Constitutional Court,Footnote 34 and Canadian employment and tax cases.Footnote 35 Some of this research has spun off into commercial products, discussed in the next section.
This scholarly work reflects all the strengths and weaknesses of the wider field. Though direct comparison among studies can be difficult given different datasets and performance measures, predictive performance has ranged from relatively modest marginal classification accuracyFootnote 36 to a very high F1 score of 98 percent in one study.Footnote 37
That said, some high-performing academic approaches may suffer from research design flaws, as they appear to use the text of a court’s description of the facts of a case and the laws cited to predict the court’s ruling.Footnote 38 This is problematic, as judges or their clerks often write case descriptions and choose legal citations with pre-existing knowledge of the ruling they will issue. It is no surprise that these case descriptions predict outcomes. Further, much academic work is limited in its generalizability by the narrow band of cases used to train and test predictive models. This is due to inaccessible or missing court data, especially in the United States, a problem discussed further in Section 7.4. Finally, some researchers give short shrift to explanation, in favor of prediction.Footnote 39 Though a model may perform well in forecasting results, its practical and tactical utility may be limited if lawyers seeking to make representation decisions do not know what drives the predictions and cannot square them with their mental models of the world. As discussed further in Section 7.4, explainable predictions are becoming the new norm, as interpretations are now available for even the most “black box” predictive models. For the moment, however, explainability remains a sticking point.
7.3.2 Commercial Applications
The commercial lay of the land is similar to the academic landscape, with substantial activity and disparate approaches focused on particular case types or litigation events.
The Big Three legal research companies – LexisNexis, Westlaw, and Bloomberg Law – have all developed outcome prediction tools that sit within their existing suites of research and analysis tools. LexisNexis offers what they label “judge and court analytics” as well as “attorney and law firm analytics.” In both spaces, the offerings are more descriptive than predictive – showing, for example, “a tally of total cases for a judge or court for a specific area of law to approximate experience on a motion like yours.”Footnote 40 The predictive jump is left to the user, who decides whether to adopt the approximation as a prediction or to distinguish it from the case at hand. LexisNexis provides further predictive firepower in the form of an acquired start-up, LexMachina, which provides, among other output, estimates of judges’ likelihood of granting or denying certain motions in certain case types.Footnote 41 Westlaw offers similar options in its litigation and precedent analytics tools,Footnote 42 as does Bloomberg Law in its litigation analytics suite.Footnote 43 Fastcase, a newer entrant into the space, offers a different approach, allowing subscribers to build their own bespoke predictive and descriptive analyses, using tools and methodologies drawn from a host of partner companies.Footnote 44
A collection of smaller companies offers litigation outcome prediction focused on particular practice areas or litigation events. Docket Alarm, now owned by Fastcase, offers patent litigation analytics that produce “the likelihood of winning given a particular judge, technology area, law firm or party.”Footnote 45 In Canada, Blue J Tax builds on the scholarly work described above to offer outcome prediction in tax disputes,Footnote 46 while in the United Kingdom companies like CourtQuant “predict [case] outcome and settlement probability.”Footnote 47
A final segment of the industry are law firms’ and other players’Footnote 48 homegrown, proprietary tools. On the plaintiffs’ side, giant personal injury firm Morgan & Morgan has developed “a ‘Google-style’ operation” in which the firm “evaluate[s] ‘actionable data points’ about personal injury settlements or court proceedings” and uses the insight to “work up a case accordingly – and … do that at scale.”Footnote 49 Defense-side firms are doing the same. Dentons, the world’s largest firm, even spun off an independent analytics lab and venture firm to fund development in outcome prediction and other AI-enabled approaches to law.Footnote 50
It is difficult to assess how well any of these tools performs, as access is expensive or unavailable, the feature sets used as inputs are not always clear, and the algorithms that power the predictions are hidden. I raise some concerns about commercial model design in Section 7.4 – in particular, reliance on lawyer identity as a predictor – and, as above, return to the perpetual problem of inaccessible and missing court data.
7.3.3 Outcome Prediction for Low- and Middle-Income Litigants
For reasons explored further below, examples are scarce of computationally driven litigation outcome prediction tools engineered specifically for the kinds of cases noted in this chapter’s opening. Philadelphia’s civil legal services provider, Community Legal Services, uses a tool called Expungement Generator (EG) to determine whether criminal record expungement is possible and assist in completing the paperwork.Footnote 51 The EG does not predict outcomes, but its automated approach enables efficiency gains for an organization that prepares thousands of expungement petitions per year.Footnote 52 Similarly, an application developed in the Family Law Clinic at Duquesne University School of Law prompts litigants in child support cases to answer a set of questions, which the tool then evaluates to determine “if there is a meritorious claim for appeal to be raised” under Pennsylvania law.Footnote 53 As with the EG, the Duquesne system does not appear to use machine learning techniques, but rather to apply a set of mechanical rules. The clinic plans prediction as a next step, however, and is developing a tool that analyzes winning arguments in appellate cases in order to guide users’ own arguments.Footnote 54
7.4. Present Limits
Having surveyed the state of the outcome prediction field, I now step back and assess its limits. As David Freeman Engstrom and Jonah Gelbach rightly concluded in earlier work: “[L]egal tech tools will arrive sooner, and advance most rapidly, in legal areas where data is abundant, regulated conduct takes repetitive and stereotypical forms, legal rules are inherently stable, and case volumes are such that a repeat player stands to gain financially by investing.”Footnote 55 Many of the commercial tools highlighted above fit this profile. Tax-oriented products exploit relatively stable rules; Morgan & Morgan’s internal case evaluation system exploits the firm’s extraordinarily high case volumes.
Yet, as noted above, data’s “abundance” is an open question, as is data quality. Methodological problems may also hinder these tools’ development. In the access to justice domain, the questions of investment incentives and financial gains loom large as well. The remainder of this Section addresses these limitations.
7.4.1 Data Limitations
Predictive algorithms require access to large amounts of data from previous court cases for model training, but such bulk data is not widely or freely available in the United States from the state or federal courts or from administrative agencies that have an adjudicatory function.Footnote 56 The Big Three have invested substantial funds in compiling private troves of court documents and judicial decisions, and jealously guard those resources with high user fees, restrictive terms and conditions, and threatened and actual litigation.Footnote 57
Data inaccessibility creates serious problems for outcome prediction tools designed to meet the legal needs of low- and middle-income litigants.Footnote 58 Much of this litigation occurs in state courts, where data is sometimes poorly managed and siloed in multiple systems.Footnote 59 Moreover, there is little money in practice areas like eviction defense and public benefits appeals, in which clients, by definition, are poor. Thus, data costs are high, and financial incentives for investment in research and development are low.
Even the products offered by the monied Big Three, however, suffer from data problems. With large companies separately assembling their own private data repositories, coverage varies widely, producing remarkable disagreement about basic facts. A recent study revealed that the answers supplied to the question “How many opinions on motions for summary judgment has Judge Barbara Lynn (N.D. Tex.) issued in patent cases?” ranged from nine to thirty-two, depending on the legal research product used.Footnote 60 This is an existential problem for the future of litigation outcome prediction, as predictions are only as good as the data on which they are built.Footnote 61
A final data limitation centers on the challenges of causal explanation. Even if explainable modeling approaches are used, the case characteristics that appear to be the strongest predictors of outcomes may not, in fact, be actionable. For instance, when a predictive tool relies on attorney identity as a feature, the model’s prediction may actually be free-riding on the attorney’s own screening and selection decisions. In other words, if the presence of Lawyer A in a case is strongly predictive of a win for her clients, Lawyer A’s skills as a litigator may not be the true cause. The omitted, more predictive variable is likely the strength of the merits, and Lawyer A’s skill at assessing those merits up-front. Better data could enable better model construction, avoiding these kinds of proxy variable traps.
7.4.2 Methodological Limitations
Sitting atop these data limitations are two important methodological limitations. First, as noted above, even if predictive tools do a good job of forecasting the probable outcome of a litigation event, they may only poorly explain why the predicted outcome is likely to occur. Explanation is important for a number of related reasons, among them engendering confidence in predictions, enabling bias and error detection, and respecting the dignity of people affected by prediction.Footnote 62 Indeed, the European Union’s General Data Protection Regulation (GDPR) has established what some scholars have labeled a “right to an explanation,” consisting of a right “not to be subject to a decision based solely on automated processing” and various rights to notice of data collection.Footnote 63 Though researchers are actively developing explainable AI that can identify features’ specific importance to a prediction and generate counterfactual predictions if features change value,Footnote 64 the field has yet to converge on a single set of explainability practices, and commercial approaches vary widely.
Second, outcome prediction is limited by machine and deep learning algorithms’ inability to reason by analogy. Legal reasoning depends on analogical thinking: the ability to align one set of facts to another and guess at the likely application of the law, given the factual divergences. However, teaching AI to reason by analogy is a cutting-edge area of computer science research, and it is far from well established. As computer scientist Melanie Mitchell explains, “‘Today’s state-of-the-art neural networks are very good at certain tasks … but they’re very bad at taking what they’ve learned in one kind of situation and transferring it to another’ – the essence of analogy.”Footnote 65 There is a famous analogical example in text analytics, where a natural language processing technique known as word embedding, when trained on an enormous corpus of real-world text, is able to produce the answer “queen” when presented with the formula “king minus man plus woman.”Footnote 66 The jump from this parlor trick to full-blown legal reasoning, though, is substantial.
In short, scaling up computationally driven litigation outcome prediction tools in a way that would fill the civil justice gap would require access to more and better data and methodological advances. Making bulk federal and state court and administrative agency data and records freely and easily accessible would be a very good step.Footnote 67 Marshaling resources to support methods and tool development would be another. Foundation funding is already a common ingredient in efforts to fill the civil justice gap. I propose that large law firms pitch in as well. All firms on the AmLaw 100 could pledge a portion of their pro bono budgets toward the development of litigation outcome prediction tools to be used in pro bono and low bono settings. The ABA Foundation might play a coordinating and convening role, as it is already committed to access-to-justice initiatives. Such an effort could have a much broader impact than firms’ existing pro bono activities, which tend to focus on representation in single cases. It might also jump-start additional interest from the Big Three and other commercial competitors, who might invest more money in improving algorithms’ predictive performance and spin off free or low-cost versions of their existing suites of tools.
7.5 Unintended Consequences
Time will tell whether, when, and how the data and methodological problems identified in Section 7.4 will be solved. Assuming that they are, and litigation outcome prediction tools can reliably generate highly reliable forecasts, there still may be reason for caution.
This Section identifies two possible unintended consequences of outcome prediction tools, which could develop alongside the salutatory access to justice effects described in Section 7.2: harm to would-be litigants denied representation whose claims are novel or less viable according to predictive tools, and harm to the common law system as a whole.
Here, the assumption is that such tools have access to ample data, account for all relevant variables, and are transparent and explainable – in other words, the tools work as intended to learn from existing patterns in civil litigation outcomes and reproduce those patterns as outcome predictions. Yet it is this very reproductive nature that is cause for concern.
7.5.1 Harms to Would-Be Litigants
Consider the facts of Elisa B. v. Superior Court,Footnote 68 a case decided by the California Supreme Court in 2005. Emily B. sought child support from her estranged partner, Elisa B., for twins whom Emily had conceived via artificial insemination of her eggs during her relationship with Elisa. If Emily walked into a lawyer’s office seeking help with her child support action, the lawyer might be interested in the case’s viability: How often have similar fact patterns come before California courts, and what was their outcome? The answers might inform the lawyer’s decision about whether to offer representation.
In real life, this case was one of first impression in California. The governing law, the Uniform Parentage Act, referred to “mother” and “father” as the potential parents.Footnote 69 Searching for relevant precedent, the Elisa B. court reasoned by analogy from previous cases that involved, variously, three potential parents (one man and two women), non-biological fathers, non-biological mothers, and a woman who raised her half-brother as her son.Footnote 70 From this and other precedent, the court cobbled together a new legal rule that required Elisa B. to pay child support for her and Emily B.’s children.
I am doubtful that an outcome prediction tool would have reached this same conclusion. The number of analogical jumps that the court made would seem to be outside the capabilities of machine and deep learning, even assuming methodological advancement.Footnote 71 Further, judges’ decisions about what prior caselaw to draw upon and how many analogical leaps to make may be influenced by factors like ideology and public opinion, which could be difficult to model well. Emily B.’s claim would likely receive a very low viability score.Footnote 72
A similar cautionary tale comes from my own previous work with Camille Gear Rich and Zev Eigen on attorneys’ non-computational assessments of claim viability. We documented plaintiffs’ employment attorneys’ dim view of the likelihood of success for employment discrimination claims and their shifting of case selection decisions away from discrimination and toward easier-to-prove wage-and-hour claims.Footnote 73 One result of this shift, we observed, was that even litigants with meritorious discrimination claims were unable to find legal representation. That work happened in 2014 and 2015, before litigation outcome prediction tools were widely available, and I am not aware of subsequent empirical studies on the effect of such tools on lawyers’ intake decisions. Yet if lawyers were already using their intuition to learn from past cases and predict future outcomes, pre-AI, machine and deep learning tools could just cement these same patterns in place.
Thus, in this view, as civil litigation outcomes become more predictable, claims become commoditized. Outlier claims and clients like Emily B. may become less representable, much like high-loss risks become less insurable. While access to justice on the whole may increase, the courthouse doors may be effectively closed to some classes of potential clients who seek representation for novel or disfavored legal claims or defenses.Footnote 74
Further, to the extent that representation is denied to would-be litigants because of their own negative personal histories, ingested by a model as data points, litigation outcome prediction tools can reduce people to their worst past acts and prevent them from changing course. Take as an example a tenant with an old criminal record trying to fight an eviction, whose past conviction reduces her chance of winning according to an algorithmic viability assessment. This may be factually accurate – her criminal record may actually make eviction defense more challenging – but a creative lawyer might see other aspects of her case that an algorithmic assessment might miss. By reducing people to feature sets and exploiting the features that are most predictive of outcomes, but perhaps least representative of people’s full selves, computational tools enact dignitary harm. In the context of low-income litigants facing serious and potentially destabilizing court proceedings, and who are algorithmically denied legal representation, such tools can also cause substantial economic and social harm, reducing social mobility and locking people into place.
Indeed, machine and deep learning methods are inherently prone to what some researchers have called “value lock-in.”Footnote 75 All data is historical in the sense that it captures points in time that have passed; all machine and deep learning algorithms find patterns in historical data as a way to predict the future. This methodological design reifies past practices and locks in past patterns. As machine learning researcher Abeba Birhane and her collaborators point out, then, machine learning is not “value-neutral.”Footnote 76 And as AI pioneer Joseph Weizenbaum observed, “the computer has from the beginning been a fundamentally conservative force which solidified existing power: in place of fundamental social changes … the computer renders technical solutions that allow existing power hierarchies to remain intact.”Footnote 77 It is no accident that the anecdotes above involve a lesbian couple, employment discrimination claimants, and a tenant with a criminal record: the fear is that would-be litigants like these with the least power historically become further disempowered at the hands of computational methods.
Yet as Section 7.2 suggested, a different story might also be possible: More accurate predictions might enable lawyers to fill their case portfolios with low-risk sure winners as hedges when taking on riskier cases like Elisa B., or might help them discover and invest in previously under-resourced practice areas. At this stage, whether predictive tools would increase or decrease representation for outlier claims and clients is an open empirical question, which researchers and policy makers should work to answer as data and methods improve and outcome prediction tools become more widely used.
7.5.2 Harms to the System
I turn now to the second potential harm caused by computationally driven litigation outcome prediction: harm to the common law system itself.Footnote 78 As Charles Barzun explains, common-law reasoning “contains seeds of radicalism [in that] the case-by-case process by which the law develops means it is always open to revision. And even though its official position is one of incremental change … doctrine [is] constantly vulnerable to being upended.”Footnote 79 Barzun points to Catharine MacKinnon’s invention of sexual harassment doctrine out of Title VII’s cloth as an example of a “two-way process of interaction” between litigants, representing their real-world experience, and the courts, interpreting the law, in a shared creative process “in which the meaning and scope of application of the statute changes over time.”Footnote 80
If lawyers rely too heavily on litigation outcome prediction tools, which reproduce past patterns, the stream of new fact presentations and legal arguments flowing into the courts dries up. Litigation outcome prediction tools may produce a sort of super stare decisis by narrowing lawyers’ case selection preferences to only those case, claim, and client types that have previously appeared and been successful in court. Yet stare decisis is only one aspect of our common law system. Another competing characteristic is flexibility: A regular influx of new cases with new fact patterns and legal arguments enables the law to innovate and adapt. In other words, noise – as differentiated from signal – is a feature of the common law, not a bug. Outcome prediction tools that are too good at picking up signals and ignoring noise eliminate the structural benefits of the noise, and privilege stare decisis over flexibility by shaping the flow of cases that make their way to court.
Others, particularly Engstrom and Gelbach, have made this point, suggesting that prediction
comes at a steep cost, draining the law of its capacity to adapt to new developments or to ventilate legal rules in formal, public interpretive exercises …. The system also loses its legitimacy as a way to manage social conflict when the process of enforcing collective value judgments plays out in server farms rather than a messy deliberative and adjudicatory process, even where machine predictions prove perfectly accurate.Footnote 81
The danger is that law becomes endogenous and ossified. “Endogenous,” to repurpose a concept introduced by Lauren Edelman, means that the law’s inputs become the same as its outputs and “the content and meaning of law is determined within the social field that it is designed to regulate.”Footnote 82 “Ossified,” to borrow from Cynthia Estlund, means that the law becomes “essentially sealed off … both from democratic revision and renewal from local experimentation and innovation.”Footnote 83
7.6 Next Steps
As noted above, whether any of the unintended consequences outlined above will come to pass – and, indeed, whether access to justice improvements will come to pass as well – turns on empirical questions. Given the problems and limitations identified in Section 7.4, will litigation outcome prediction tools actually work well enough to achieve either their potential benefits or cause their potential harms? My assessment of the present state of the field suggests there is a long way to go before we reach either set of outcomes. But as the field matures, we can build in safeguards against the endogeneity risks and harms I identify above through technical, organizational, and policy interventions.
First, on the technical side, computer and data scientists, and the funders who make their work possible, should invest heavily in improving algorithmic analogical reasoning. Without the ability to reason by analogy, outcome predictors not only will miss an array of possible positive predictions, but they will also be systematically biased against fact patterns like Emily B.’s, which present issues of first impression.
Further on the technical front, developers could purposefully over-train predictive algorithms on novel, but successful, fact patterns and legal arguments in order to nudge the system off its path and make positive predictions possible even for cases that fall outside the norm. This idea is adapted from OpenAI’s work in nudging its state-of-the-art language model, GPT-3, away from its “harmful biases, such as outputting discriminatory racial text” learned from its training corpus, by over-exposing it to counter texts.Footnote 84
Technical fixes focus on outcome prediction tools’ production side. Organizational fixes target the tools’ consumers: the lawyers, law firms, and other legal organizations that might use them to influence case selection. I propose here that no decision should be made exclusively on the basis of algorithmic output. This guards against the dignitary and other real harms described above, as would-be litigants are treated as full people rather than feature sets. This also parallels the GDPR’s explanation mandate, though I suggest it here as an organizational practice that is baked into legal organizations’ decision-making processes.Footnote 85
Finally, I turn to policy. The story above assumes a profit-driven lawyer as the user of outcome prediction tools. Of course, there are other possible motivations for a lawyer’s case selection decisions, such as seeking affirmatively to establish a new interpretation of the law or right a historic wrong. These cause lawyers, from all points on the ideological spectrum, may be particularly likely to take on seemingly high-risk claim or party types, which receive low computationally determined viability scores. Government lawyers, too, may function as cause lawyers, pushing legal arguments, in accordance with administration position, that diverge from courts’ past practices. Government agencies should study trends in private attorneys’ use of litigation outcome prediction tools in the areas in which they regulate, and should make their own case selection decisions to fill gaps in representation.Footnote 86
7.7 Conclusion
This chapter has explored the consequences of computationally driven litigation outcome prediction tools for the civil justice system, with a focus on increasing access to justice. It has mapped the current state of the outcome prediction field in academic work and commercial applications, as well as in pro bono and low bono practice settings. It has also raised concerns about unintended consequences for litigants and for our legal system as a whole.
I conclude that there is plenty of reason for “techno-optimism,” to use Tanina Rostain’s term, about the potential for computationally driven litigation outcome prediction tools to close the civil justice gap.Footnote 87 However, reaching that optimistic future, while also guarding against potential harms, will require substantially more money and data, continued methodological improvement, careful organizational implementation, and strategic deployment of government resources.
Debate about legal tech and the future of civil litigation typically focuses on high-technology innovations. This volume is no exception, and with good reason. Advanced technologies are spreading (or seem poised to spread) throughout the legal landscape, from discovery to online dispute resolution (ODR) to trials, and from individual lawsuits to aggregate litigation. These tools’ practical utility and social value are rightly contested.Footnote 1
But in some contexts, straightforward, low-tech solutions hold tremendous promise – and also demand attention. Here, we zero in on a modest tool that bears upon the management of multidistrict litigation, or MDL. In particular, we explore how improved online communication could enhance litigant autonomy, usher in a more “participatory” MDL, and supply a platform for further innovation.Footnote 2
The MDL statute – 28 U.S.C. §1407 – is a procedural vehicle through which filed federal cases involving common questions of fact, such as a mass tort involving asbestos or defective pharmaceuticals, are swept together into a single “transferee” court, ostensibly for pretrial proceedings (though very often, in reality, for pretrial adjudication or settlement).Footnote 3 Thirty years ago, MDLs were barely a blip on our collective radar. As of 1991, these actions made up only about 1 percent of pending civil cases.Footnote 4 Now, by contrast, MDLs make up fully half of all new federal civil filings.Footnote 5 This means that one out of every two litigants who files a claim in federal court might not really be fully represented by the lawyer she chose, get the venue she chose, or remain before the judge to whom her suit was initially assigned. Instead, her case will be fed into the MDL system and processed elsewhere, in a long, labyrinthian scheme that is often far afield and out of her sight.Footnote 6
Given these statistics, there’s no real question that the MDL has risen – and that its rise is significantly altering the American system of civil justice. There is little consensus, however, as to whether the MDL’s ascent is a good or bad thing. Some celebrate the MDL for promoting judicial efficiency, addressing harms that are national in scope, channeling claims to particularly able and expert advocates, creating economies of scale, and increasing access to justice – giving some judicial process to those who, without MDL, would have no ability to vindicate their essential rights.Footnote 7
Others, meanwhile, find much to dislike. Critics frequently seize on MDLs’ relatively slow speed,Footnote 8 their heavy reliance on repeat play,Footnote 9 and the free-wheeling judicial “ad hocery” that has become the device’s calling card.Footnote 10 Beyond that, critics worry that the device distorts the traditional attorney-client relationship and subverts litigant autonomy.Footnote 11 Critics fear that aggregation alters traditional screening patterns, which can unleash a “vacuum cleaner effect” and ultimately lead to the inclusion of claims of dubious merit.Footnote 12 And critics note that the device has seemingly deviated from its intended design: The MDL was supposed to aggregate cases for pretrial proceedings. So, the status quo – where trials are rare and transfer of a case back to a plaintiff’s home judicial district is exceptional – means, some say, that the MDL has strayed off script.Footnote 13
Stepping back, one can see: The MDL has certain advantages and disadvantages. Furthermore, and critically, many MDL drawbacks are baked in. There are certain compromises we must make if we want the efficiencies and access benefits MDLs supply. Aggregation (and, with it, some loss of litigant autonomy) is an essential and defining feature of the MDL paradigm. The same may be said for judicial innovation, the need to adapt the traditional attorney-client relationship, or the fact that some lawyers are tapped to lead MDLs in a selection process that will, inevitably, consign some able and eager advocates to the sidelines.
Recognizing these unavoidable trade-offs, in our own assessment, we ask subtly different and more targeted questions. We don’t hazard to assess whether MDLs, on balance, are good or bad. Nor do we even assess whether particular MDL features (such as procedural improvisation) are good or bad. Instead, we ask two more modest questions: (1) Do contemporary MDLs have avoidable drawbacks and (2) if so, can those be addressed? In this analysis, we zero in on just one MDL drawback that is both practically and doctrinally consequential: MDL’s restriction of litigant autonomy. And we further observe: Though some loss of litigant autonomy is an inevitable and inescapable by-product of aggregation and is therefore entirely understandable (the yin to aggregation’s yang), the present-day MDL may be more alienating and involve a larger loss of autonomy than is actually necessary. As explained in Section 8.1, that is a potentially large problem. But, we also argue, it is a problem that, with a little ingenuity, courts, policymakers, scholars, and litigators can practically mitigate.
The remainder of this chapter proceeds in three Parts. Section 8.1 sets the scene by focusing on individual autonomy. In particular, Section 8.1.1 explains why autonomy matters, while Section 8.1.2 draws on MDL plaintiff survey data recently compiled by Elizabeth Burch and Margaret Williams to query whether MDL procedures might compromise litigant autonomy more than is strictly necessary. Then, to assess whether transferee courts are currently doing what they practically can to promote autonomy by keeping litigants up-to-date and well-informed, Section 8.2 offers the results of our own systematic study of current court-run MDL websites. This analysis reveals that websites exist but are deficient in important respects. In particular, court websites are hard to find and often outdated. They lack digested, litigant-focused content and are laden with legalese. And they rarely offer litigants opportunities to attend hearings and status conferences remotely (from their home states). In light of these deficiencies, Section 8.3 proposes a modest set of changes that might practically improve matters. These tweaks will not revolutionize MDL processes. But they could further litigants’ legitimate interests in information, with little risk and at modest cost. In so doing, they seem poised to increase litigant autonomy – “low-tech tech,” to be sure, but with high potential reach.
8.1 Individual Autonomy, Even in the Aggregate: Why It Matters and What We Know
8.1.1 Why Individual Autonomy Matters
Litigant autonomy is a central and much-discussed concern of any adjudicatory design, be it individualized or aggregate. And, when assessing MDLs, individual autonomy is especially critical; indeed, its existence (or, conversely, its absence) goes to the heart of MDL’s legitimacy. That’s so because, if litigants swept into MDLs truly retain their individual autonomy – and preserve their ability meaningfully to participate in judicial processes – then the source of the MDL’s legitimacy is clear. On the other hand, to the extent consolidation into an MDL means that individual litigants necessarily and inevitably sacrifice their individual autonomy and forfeit their ability meaningfully to participate in judicial processes (and offer, or withhold, authentic consent to a settlement agreement), the MDL mechanism sits on much shakier ground.Footnote 14
On paper, that is not a problem: MDLs, as formally conceived, do little to undercut the autonomy of individual litigants. In theory, at least, MDLs serve only to streamline and expedite pretrial processes; they (again, in theory) interfere little, if at all, with lawyer-client communication, the allocation of authority within the lawyer-client relationship, or the client’s ability to accept or reject the defendant’s offer of settlement. That formal framework makes it acceptable to furnish MDL plaintiffs (unlike absent class members, say) with few special procedural protections.Footnote 15 It is thought that, even in an MDL, our old workhorses – Model Rules of Professional Conduct 1.4 (demanding candid attorney-client communication), 1.7 (policing conflicts), 1.2(a) (clarifying the allocation of authority and specifying “that a lawyer shall abide by a client’s decisions concerning the objectives of representation”), 1.16 (limiting attorneys’ ability to withdraw), and 1.8(g) (regulating aggregate settlements) – can ensure the adequate protection of clients.
In contemporary practice, however, MDLs are much more than a pretrial aggregation device.Footnote 16 And, it is not necessarily clear that in this system – characterized by infrequent remand to the transferor court, prescribed and cookie-cutter settlement advice, and heavy-handed attorney withdrawal provisions – our traditional ethics rules continue to cut it.Footnote 17 Indeed, some suggest that the status quo so thoroughly compromises litigant autonomy that it represents a denial of due process, as litigants are conscripted into a system “in which their substantive rights will be significantly affected, if not effectively resolved, by means of a shockingly sloppy, informal, and often secretive process in which they have little or no right to participate, and in which they have very little say.”Footnote 18
Individual autonomy is thus the hinge. To the extent it mostly endures, and to the extent individual litigants really can participate in judicial proceedings, authentically consent to settlement agreements, and control the resolution of their own claims, MDL’s legality and legitimacy is clearer. To the extent individual autonomy is a fiction, MDL’s legality and legitimacy is more doubtful.
The upshot? If judges, policymakers, scholars, and practitioners are concerned about – and want to shore up – MDL legitimacy, client autonomy should be fortified, at least where doing so is possible without major sacrifice.
8.1.2 Litigant Autonomy: What We Know
The above discussion underscores that in MDLs, litigant autonomy really matters. That insight tees up a clear – albeit hard-to-answer – real-world question: How much autonomy do contemporary MDL litigants actually have?
Context and caveats. That is the question to which we now turn, but before we do, a bit of context is necessary. The context is that, ideally, to gauge the autonomy of MDL litigants, we would know exactly how much autonomy is optimal and also how much is minimally sufficient – and how to measure it. Or, short of that, we could perhaps compare rigorous data that captures the experiences of MDL plaintiffs as against those of one-off “traditional” plaintiffs to understand whether, or to what extent, the former outperform or underperform the latter along relevant metrics.
Yet neither is remotely possible. Though litigant autonomy is an oft-cited ideal, we don’t know exactly what it would look like and mean, if fully realized, to litigants. Worse, decades into the empirical legal studies revolution, we continue to know shockingly little about litigants’ preferences, priorities, or lived experiences, whether in MDLs or otherwise.Footnote 19
These uncertainties prevent most sweeping claims about litigant autonomy. Nevertheless, one can, at least tentatively, identify several ingredients that are necessary, if not sufficient, to safeguard the autonomy interests of litigants. That list, we think, includes: Litigants can access case information and monitor judicial proceedings if they so choose; litigants can communicate with their attorneys and understand the signals of the court; litigants have a sense of where things stand, including with regard to the strength of their claim, their claim’s likelihood of success, and where the case is in the litigation life cycle; and litigants are empowered to accept or reject the defendant’s offer of settlement.Footnote 20 A system with these ingredients would seem to be fairly protective of individual autonomy. A system without seems the opposite.
Findings from the Burch-Williams study. How do MDL litigants fare on the above metrics? A survey, recently conducted by Elizabeth Burch and Margaret Williams, offers a partial answer.Footnote 21 The two scholars surveyed participants in recent MDLs, gathering confidential responses over multiple years.Footnote 22 In the end, 217 litigants (mostly women who had participated in the pelvic mesh litigation) weighed in, represented by 295 separate lawyers from 145 law firms.Footnote 23
The survey captures claimants’ perspectives on a wide range of subjects, including their reasons for initiating suit and their ultimate satisfaction with case outcomes. As relevant to litigant autonomy, information, and participation, the scholars found the following:
When asked if their lawyer “kept [them] informed about the status of [their] case,” 59 percent of respondents strongly or somewhat disagreed.Footnote 24
When offered the prompt: “While my case was pending, I felt like I understood what was happening,” 67.9 percent of respondents strongly or somewhat disagreed. Only 13.7 percent somewhat or strongly agreed.
When asked how their lawyers kept them informed and invited to list multiple options, more than a quarter of respondents – 26 percent – reported that their attorney did not update them at all.
Of the 111 respondents who reported on their attorneys’ methods of communication, only two indicated that their lawyer(s) utilized a website to communicate with them; only one indicated that her lawyer utilized social media for that purpose.
34 percent of respondents were unable or unwilling to identify their lawyer’s name.
Caveats apply: Respondents to the opt-in survey might not be representative, which stunts both reliability and generalizability.Footnote 25 The numbers, even if reliable, supply just one snapshot. And, with one data set, we can’t say whether litigant understanding is higher or lower than it would be if the litigants had never been swept into the MDL system and instead had their case litigated via traditional means. (Nor can we, alternatively, say whether, but for the MDL’s efficiencies, these litigants might have been shut out of the civil justice system entirely.Footnote 26) Nor can we even say whether MDL clients are communicated with more, or less, than those whose claims are “conventionally” litigated.Footnote 27
Even recognizing the study’s major caveats, however, five larger points seem clear. First, when surveyed, MDL litigants, represented by a broad range of lawyers (not just a few “bad apples”), reported infrequent attorney communication and persistent confusion.Footnote 28 Second, knowledgeable and independent experts echo litigants’ concerns, suggesting, for example, that “[p]laintiffs [within MDLs] have insufficient information and understanding to monitor effectively the course of the litigation and insufficient knowledge to assess independently the outcomes that are proposed for their approval if and when a time for settlement arrives.”Footnote 29 Third, plaintiffs’ lawyers in MDLs frequently have very large client inventories – of hundreds or thousands of clients.Footnote 30 When a lawyer has so many clients, real attorney-client communication and meaningful litigant participation is bound to suffer.Footnote 31 Fourth, when it comes to the promotion and protection of litigant autonomy, effective communication – and the provision of vital information – is not sufficient, but it is certainly necessary. Even well-informed litigants can be excluded from vital decision-making processes, but litigants, logically, cannot call the shots while operating in the dark.Footnote 32 And fifth, per Section 8.1, to the extent that individuals swept into MDLs unnecessarily forfeit their autonomy, that’s a real problem when it comes to MDL legitimacy and legality.Footnote 33
These five points paint a worrying portrait. Fortunately, however, alongside those five points, there is one further reality: Straightforward measures are available to promote litigants’ access to case information, their ability to monitor judicial proceedings, and their understanding of the litigation’s current path and likely trajectory. And, as we will argue in Section 8.3, these measures can be implemented by courts now, with little difficulty, and at reasonable cost.
8.2 Current Court Communication: MDL Websites and Their Deficiencies
Section 8.1 reviewed survey findings that indicate litigants within MDLs report substantial confusion and limited understanding. As noted, when given the prompt: “While my case was pending, I felt like I understood what was happening,” only 13.7 percent somewhat or strongly agreed.Footnote 34 These perceived communication failures are surprising. It’s 2023. MDL websites are common, and emails are easy; “the marginal cost of additional communication [is] approaching zero.”Footnote 35 What explains these reported gaps?
To gain analytic leverage on that question, we rolled up our sleeves and looked at where some MDL-relevant communication takes place.Footnote 36 In particular, we trained our gaze on MDL websites – resources that, per the Judicial Panel on Multidistrict Litigation (JPML) and Federal Judicial Center, “can be … invaluable tool[s] to keep parties … informed of the progress of the litigation.”Footnote 37 These sites are often described as key components of case management.Footnote 38 Scholars suggest that they facilitate litigants’ “due process rights to participate meaningfully in the proceedings.”Footnote 39 And, perhaps most notably, judges themselves have described these websites as key conduits of court-client communication.Footnote 40
Do MDL websites fulfill their promise of keeping “parties … informed of the progress of the litigation” by furnishing well-curated, up-to-date, user-friendly information? To answer that question, we reviewed each page of available websites for the twenty-five largest currently pending MDLs. Each of these MDLs contained at least 500 pending actions; together, they accounted for nearly 415,000 pending actions, encompassing the claims of hundreds of thousands of individual litigants, and constituted 98 percent of actions in all MDLs nationwide.Footnote 41 Thus, if judges are using court websites to engage in clear and frequent communication with individual litigants, we would have seen it.
We didn’t. Websites did exist. Of the twenty-five largest MDLs, all except one had a website that we could locate.Footnote 42 But, many of these sites were surprisingly limited and difficult to navigate. Indeed, the sites provided scant information, were not consistently updated, and often lacked straightforward content (like Zoom information or “plain English” summaries).
8.2.1 An Initial Example: The Zantac MDL
Take, as an initial example, the website that accompanies the Zantac MDL, pending in the Southern District of Florida.Footnote 43 We zero in on this website because it was one of the best, most user-friendly sites we analyzed. But even it contained serious deficiencies.
For starters, finding the website was challenging. A preliminary search – “Zantac lawsuit” – yielded over 1 million hits, and the official court website did not appear on the first several pages of Google results; rather, the first handful of results were attorney advertisements (mostly paid) or attorney and law firm websites.Footnote 44 A more targeted effort – “Zantac court website” – bumped the desired result to the first page, albeit below four paid advertisements.
Once we located the site, we were greeted with a description of the suit: “This matter concerns the heartburn medication Zantac. More specifically, this matter concerns the ranitidine molecule – the active ingredient of Zantac. The Judicial Panel for Multidistrict Litigation formed this MDL (number 2924) on February 6, 2020.”Footnote 45 We also were shown six links (Media Information, MDL Transfer Order, Docket Report, Operative Pleadings, Transcripts, and Calendar) and a curated list of PDF files (see Figure 8.1).

Figure 8.1 Zantac MDL docket
The “Calendar” led to a plain site listing basic information about an upcoming hearing, but with few details. The hearing in question was described only as “Status Conference – Case Mgt,” and it did not specify whether litigants could attend, either in person or remotely (see Figure 8.2).Footnote 46

Figure 8.2 Zantac MDL hearing notice
A litigant who clicked on the “Operative Pleadings” tab was taken to seven PDF documents (Pfizer, Inc. Answer; Class Economic Loss Complaint; etc.) described as those “of special interest,” plus a note that “the most accurate source for orders is PACER.”Footnote 47 (The site did not include information regarding what PACER is, though it did include a link; see Figure 8.3.)

Figure 8.3 Zantac operative pleadings
Finally, a search box allowed for a search of the case’s orders, again available as PDFs.
8.2.2 The Rest: Deficits along Five Key Dimensions
Within our broader sample, usability deficits were pervasive and very often worse than the Zantac MDL site. In the course of our inquiry, we reviewed websites along the following five dimensions: (1) searchability and identifiability; (2) plaintiff-focused content; (3) use of plain language; (4) whether the site supplied information to facilitate remote participation in, or attendance at, proceedings; and (5) timeliness. We found deficits along each.
Searchability and identifiability. A website is only useful if it can be located. As such, our first inquiry was whether MDL websites were easy, or alternatively difficult, to find. Here, we found that, as in Zantac, court sites were often buried under a thicket of advertisements for lawyers or lead generators (see Figure 8.4).Footnote 48 Commonsense search terms for the three largest MDLs yielded results on pages 13, 4, and 8, respectively.Footnote 49

Figure 8.4 Hernia mesh Google search
Litigant-focused content. Next, we evaluated whether websites featured custom content that was seemingly geared to orient individual litigants. Most didn’t. In particular, of the twenty-four sites we reviewed, only eleven contained any meaningful introductory content at all. Even then, those introductions focused primarily on the transfer process (including the relevant JPML proceeding) and a statement of the case’s overall topic – not its current status or its anticipated timeline. Meanwhile, only six of the twenty-four offered MDL-focused Frequently Asked Questions. And of those, most offered (and answered) questions at a general level (“What is multidistrict litigation?”) or that were clearly attorney-focused (regarding, for instance, motions to appear pro hac vice). Some others, while well intentioned, supplied limited help (see Figure 8.5).Footnote 50

Figure 8.5 Eastern District of Louisiana website link
Similarly, more than half of sites identified members of the cases’ leadership structure (e.g., by listing leadership or liaison counsel) and provided contact information for outreach. But none directed plaintiffs with questions to a specific point of contact among those attorneys.
Finally, materials that were presented – typically, a partial set of key documents, such as court orders or hearing transcripts – were often unadorned. For instance, seven of the twenty-four reviewed sites linked to orders, as PDFs, with essentially no description of what those documents contain (see Figure 8.6).Footnote 51
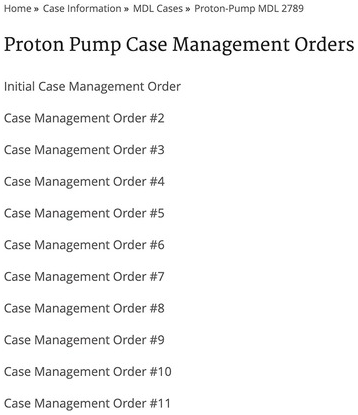
Figure 8.6 Proton Pump case management orders
Better: Sixteen of the sites offered some descriptions of posted PDFs. But only two included status updates that went much beyond one-line order summaries (see Figure 8.7).Footnote 52

Figure 8.7 “Current developments” listing
To a litigant, therefore, the average MDL site is best understood as a free, and often partial, PACER stand-in – not a source of curated, distilled, or intelligible information.
Jargon and legalese. We next assessed whether the websites were written in plain language – or at least translated legalese. Here, we found that the majority of sites relied on legal jargon when they described key developments.Footnote 53 For example, our review found websites touting privilege log protocols, an ESI order, and census implementation orders. Even case-specific Frequently Asked Questions – where one might most reasonably expect clear, litigant-friendly language – stopped short of “translating” key legal terms.Footnote 54 Put simply, site content was predominantly written in the language of lawyers, not litigants.
Information to facilitate remote attendance. We also gauged whether the websites offered teleconference or Zoom hearing information. This information is important because consolidated cases – and the geographic distance they entail – leave many litigants unable to attend judicial proceedings in person, which puts a premium on litigants’ ability to attend key proceedings remotely, via video or telephone.
Did the websites supply the logistical information a litigant needs in order to “attend” remotely? Not particularly. Of the twenty-four sites we reviewed, thirteen did not offer any case calendar that alerted litigants of upcoming hearings or conferences. Of the eleven that did:
Five listed events on their calendar (though some of the listed events had already occurred) without any Zoom or telephone information;
Two included Zoom or telephone information for some, but not all, past events;
Two included Zoom or telephone information for all events listed on the case calendar; and
Two included dedicated calendar pages but had no scheduled events.
Put another way, most sites did not include case calendars; of those that did, more than half lacked Zoom or other remote dial-in information for some or all listed hearings. That absence was particularly striking given that, in the wake of the COVID-19 pandemic, nearly all courts embraced remote proceedings.Footnote 55
Unsurprisingly, the sites’ presentation of upcoming hearings also varied widely. In some instances (as on the MDL-2775, MDL-3004, and MDL-2846 sites shown in Figure 8.8 a–c), virtual hearings were listed, but no dial-in information was provided.Footnote 56 In contrast, some MDL sites (like MDL-2741Footnote 57) linked to Zoom information (Figure 8.9).



Figure 8.8 a–c Link-less “Upcoming MDL Events” examples

Figure 8.9 Link-provided “Upcoming Proceedings” example
Timeliness. Lastly, recognizing that cases can move fast – and stale information is of limited utility – we evaluated the websites to see whether information was timely. Again, results were dispiriting. Of the sites that offered time-sensitive updates (e.g., calendars of upcoming events), several were not updated, meaning that a litigant or even an individually retained plaintiffs’ attorney who relied on the website for information was apt to be misinformed.Footnote 58 For instance, MDL-2913, involving Juul, was transferred to the Northern District of California on October 2, 2019. Its website included a calendar section and several “documents of special interest.”Footnote 59 The latest document upload involved a conditional transfer order from January 2020Footnote 60 – even though several major rulings had been issued more recently.Footnote 61 (The website’s source code indicates that it was last modified in May 2020.) Whether by conscious choice or oversight, the case’s online presence did not reflect its current status. Other sites, meanwhile, listed “upcoming” proceedings that had, in fact, occurred long before.Footnote 62 And, when we accessed archived, time-stamped versions of sites, we found several orders that were eventually posted – but not until months after they were handed down.Footnote 63
Nor were the sites set up to keep interested visitors repeatedly informed, as most of the sites did not themselves offer a direct “push” or sign-up feature, so that visitors could be notified via text or email when new material became available.Footnote 64
8.2.3 Explanations for the Above Deficits: Unspecified Audience and Insufficient Existing Guidance
What explains the above deficits? One possibility is that these websites were never intended to speak to, or otherwise benefit, actual litigants – and our analysis, then, is basically underscoring that websites, never meant to edify litigants, in fact, fail to edify them.Footnote 65 To some judges and attorney leaders, in other words, these sites may serve merely as internal or specialized resources, whether for state court judges involved in overlapping litigation, individually retained plaintiffs’ counsel, or even scholars and journalists.Footnote 66 Or, it could be that the “audience” question has never been carefully considered or seriously addressed. As a result, the websites may be trying to be all things to all people but actually serve none, as content is too general for members of the plaintiffs’ steering committee, too specialized and technical for litigants, and too partial or outdated for individually retained plaintiffs’ counsel or judges handling parallel state litigation.
A second culprit, in contrast, is crystal clear: Higher authorities have furnished transferee judges and court administrators with only limited public guidance.Footnote 67 In particular, current guidance tends to suggest categories for site content. But beyond that, it furnishes transferee judges only limited help. Illustrating this deficiency, the JPML and Federal Judicial Center’s Ten Steps to Better Case Management: A Guide for Multidistrict Litigation Transferee Court Clerks includes a discussion of recommended webpage content, but its relevant section provides only that:
Case name and master docket sheet case number
Brief description of the subject of the case
Name of the judge presiding over the case
List of court staff, along with their contact information
Names of liaison counsel, along with their contact information
In addition, it is useful to include the following types of orders in PDF:
Case management orders
Transfer orders from the Panel
Orders applicable to more than one case
Individual case orders affecting one case, but potentially pertinent to others
Suggestion of remand orders.Footnote 68
Several other pertinent resources are similarly circumscribed.Footnote 69 These publications have likely helped to spur websites’ creation, but they have stunted their meaningful evolution.
***
Whatever the reasons for the above deficiencies, the facts are these: Among the websites we reviewed, most suffered from basic deficits that could very well inhibit litigants’ access and engagement. And the deficits we identify could easily be addressed.
8.3. A Simple Path Forward: A “Low-Tech” Mechanism to Keep Litigants Better Informed
As noted in Section 8.1, MDLs rely, for legitimacy, on litigant autonomy, and while communication is not sufficient for litigant autonomy, it is necessary. Even well-informed litigants can be deprived of the capacity to make crucial decisions – but litigants, logically, cannot make crucial decisions if they are not reasonably well-informed. Meanwhile, while no one can currently prove that MDL litigants are underinformed, Section 8.2 compiled some evidence indicating information deficits are deep and pervasive. The Burch-Williams study paints a worrying portrait; knowledgeable scholars have long raised concerns; and our painstaking review of MDL websites reveals that one tool, theoretically poised to promote litigant understanding, is, in fact, poorly positioned to do so.
What can be done? Over the long run, the Federal Judicial Center (FJC), or another similar body, should furnish formal guidance to judges, court administrators, and lawyers on how to build effective and legible websites. This guidance would ideally be supplemented by a set of best practices around search engine optimization and language access. There is good reason to believe that such guidance would be effective. Noticeable similarities across existing websites suggest that transferee judges borrow heavily from one another. An implication of that cross-pollination is that better guidance from the FJC (or elsewhere) would (likely) rapidly spread.
In the meantime, we close with four concrete (though modest and partial) suggestions for transferee judges.
First, judges need to decide whom these sites are really for – and then need to ensure that the sites well-serve their intended audience. We suggest that MDL websites ought to be embraced as (among other things) a litigant-facing tool, and, as discussed below, they should be improved with that purpose in mind.Footnote 70 But, even if courts are not persuaded, they still need to do a better job tailoring sites to some particular audience. As long as the specific audience remains undetermined, courts are less likely to serve any particular audience adequately.
If courts agree that websites should speak directly to litigants, then a second recommendation follows: At least some clearly delineated website content should be customized for litigants. Courts should, as noted, avoid legalese and offer more digested (rather than just raw) material. For instance, judges might ask attorneys to supply monthly or quarterly updates; these updates, which should be approved by both parties and the court, should summarize the progress made in the preceding month and highlight what is on tap in the MDL in the immediate future. Here, the website should capture both in-court activity and noteworthy activity scheduled outside of the court’s four walls (e.g., depositions).
Third, irrespective of chosen audience, judges should take steps to ensure that MDL websites are visible and up-to-date. Regardless of whom the websites are meant to serve, websites cannot serve that audience if they cannot be quickly located.Footnote 71 And, because stale information is of limited utility, judges should ensure that the websites offer an accurate, timely snapshot of the case’s progress. The first steps are uncontroversial and straightforward; they include reliably adding hearings to the online calendar, removing them after they occur, and posting key documents within a reasonable time frame. Judges should also consider an opt-in sign-up that automatically emails or texts interested individuals when new content is added.
Fourth and finally, judges should ensure that websites clearly publicize hearings and status conferences, and, recognizing that MDLs necessarily and inescapably create distance between client and court, judges should facilitate remote participation whenever feasible. As noted above, many MDL judges have embraced remote hearings out of COVID-generated necessity; judges overseeing large MDLs should consider how the switching costs they have already paid can be invested to promote meaningful litigant access, even from afar.Footnote 72 Indeed, judges might cautiously pilot tools for two-way client-court communication, or even client-to-client communication – though, in so doing, judges must be attuned to various risks.Footnote 73
8.4. Conclusion: Zooming Out
We harbor no illusions about the role that better MDL websites can play. They’re no panacea, and vigorous debates about the merits and demerits of MDL will (and should) continue. But even so: Improved, refocused websites can keep litigants a bit more engaged; they can help litigants stay a bit better informed; and they can promote litigant participation in even distant MDL processes. More than that, improved websites can, however incrementally, promote litigant autonomy and, by extension, shore up the legitimacy of the MDL system. The day may come when some as-yet-unidentified high-tech innovation revolutionizes the MDL. Until then, low-tech changes can modestly improve the system, and just might serve as platforms for further reform.