Introduction
Although the importance of ecosystems is now central to discussions about biodiversity (Millennium Ecosystem Assessment, 2005; United Nations, 2015; Schultz et al., Reference Schultz, Tyrrell and Ebenhard2016), the distribution and habitat use of the many species that are essential components of these ecosystems remain poorly known (Meyer et al., Reference Meyer, Kreft, Guralnick and Jetz2015; Minin et al., Reference Minin, Slotow, Hunter, Pouzols, Toivonen and Peter2016). Within this context, an estimated 1 million species of animals and plants are threatened with extinction (Díaz et al., Reference Díaz, Settele, Brondizio, Ngo, Agard and Arneth2019). Mammalian carnivores have lost > 75% of their original range (Faurby & Svenning, Reference Faurby and Svenning2015; Díaz et al., Reference Díaz, Settele, Brondizio, Ngo, Agard and Arneth2019): e.g. the red wolf Canis rufus (> 99%), tiger Panthera tigris (95%), lion Panthera leo (94%) and leopard Panthera pardus (79.4%; Wolf & Ripple, Reference Wolf and Ripple2017). Approximately 25% of known mammalian species are believed to be at risk of extinction (Minin et al., Reference Minin, Slotow, Hunter, Pouzols, Toivonen and Peter2016).
Because of the urgent need for conservation action for threatened species, research has predominantly been based on proxies such as charismatic, endemic or threatened species (Ducarme et al., Reference Ducarme, Luque and Courchamp2013; Brum et al., Reference Brum, Graham, Costa, Hedges, Penone and Radeloff2017), with large-bodied, charismatic species studied preferentially (Brooke et al., Reference Brooke, Bielby, Nambiar and Carbone2014; Albert et al., Reference Albert, Luque and Courchamp2018). For example, of 4,351 peer-reviewed articles on canids and felids published during 2013–2017 there were 359 publications on the Endangered tiger and only one on the small, Near Threatened rusty-spotted cat Prionailurus rubiginosus (Tenson, Reference Tensen2018). Small wild cats receive only 1.2% of all funding for research on wild cats (Global Wildlife Conservation, 2020).
This focus on large, charismatic species ignores many medium-sized, common species. There are many common, little studied mesocarnivores that are important as predators of species such as rodents, as competitors with other medium-sized predators, and as omnivorous seed dispersers, and ‘may be fundamentally important drivers of ecosystem function, structure, or dynamics’ (Gittleman & Gompper, Reference Gittleman, Gompper, Barbosa and Castellanos2005; Roemer et al., Reference Roemer, Gompper and Van Valkenburgh2009, p. 171). In response to this concern there have been appeals to ensure common species remain common (Ramesh & McGowan, Reference Ramesh and McGowan2009; Redford et al., Reference Redford, Berger and Zack2013; Frimpong, Reference Frimpong2018; USGS, 2018; Baker et al., Reference Baker, Garnett, O'Connor, Ehmke, Clarke, Woinarski and McGeoch2019).
Here we describe how we used citizen science, with minimal financial investment, to document the occurrence of the jungle cat Felis chaus, a relatively common species in Sri Lanka but the least studied of the four wild cat species occurring in the country (Kittle & Watson, Reference Kittle and Watson2018; Miththapala, Reference Miththapala2018). The other three species are the fishing cat Prionailurus viverrinus, rusty-spotted cat and leopard Panthera pardus kotiya. The jungle cat is categorized as Least Concern on the IUCN Red List, although more information is needed on the general ecology of the species and its status across its range, and the population trend is decreasing (Gray et al., Reference Gray, Timmins, Jathana, Duckworth, Baral and Mukherjee2021). In Sri Lanka the jungle cat is categorized as Near Threatened (Ministry of Environment Sri Lanka, 2012), but little is known about its habitat use and distribution (Miththapala, Reference Miththapala2018). We aimed to model habitat suitability for this species in Sri Lanka and improve knowledge of its distribution. Based on our observations that the species may have a greater tolerance for, and perhaps benefit from, human activities than was previously believed, we hypothesized that the jungle cat is more widespread than described in the National Red List assessment.
Study area
Sri Lanka is a highly biodiverse tropical island nation in the Indian Ocean (Ministry of Mahaweli Development & Environment, 2016). The topography of the land mass of 65,610 km2 is a result of geological uplift and erosion that have led to the formation of three peneplains, with the highest (1,501–2,524 m), forming a central hill massif. The combination of these hills and the winds of the south-west and north-east monsoons result in three main climatic zones: the wet zone in the south-west (low and mid elevation and montane) with a mean annual rainfall of 2,500–5,000 mm; the dry zone extending over much of the lowlands, with a mean annual rainfall of 1,250–1,900 mm; and the intermediate zone, a narrow strip in the lowlands and on the eastern slopes of the central hills, between the wet and dry zones with a mean annual rainfall of 1,900–2,500 mm (Ministry of Mahaweli Development & Environment, 2016; Fig. 1a). Land cover is a mosaic of forests, agriculture, plantations (tea, rubber and coconut), homesteads, roads and scattered towns. Nearly 10,000 reservoirs, some up to 820 years old, provide perennial and seasonal sources of water to the large expanse of the lowland dry zone (Ministry of Mahaweli Development & Environment, 2016).
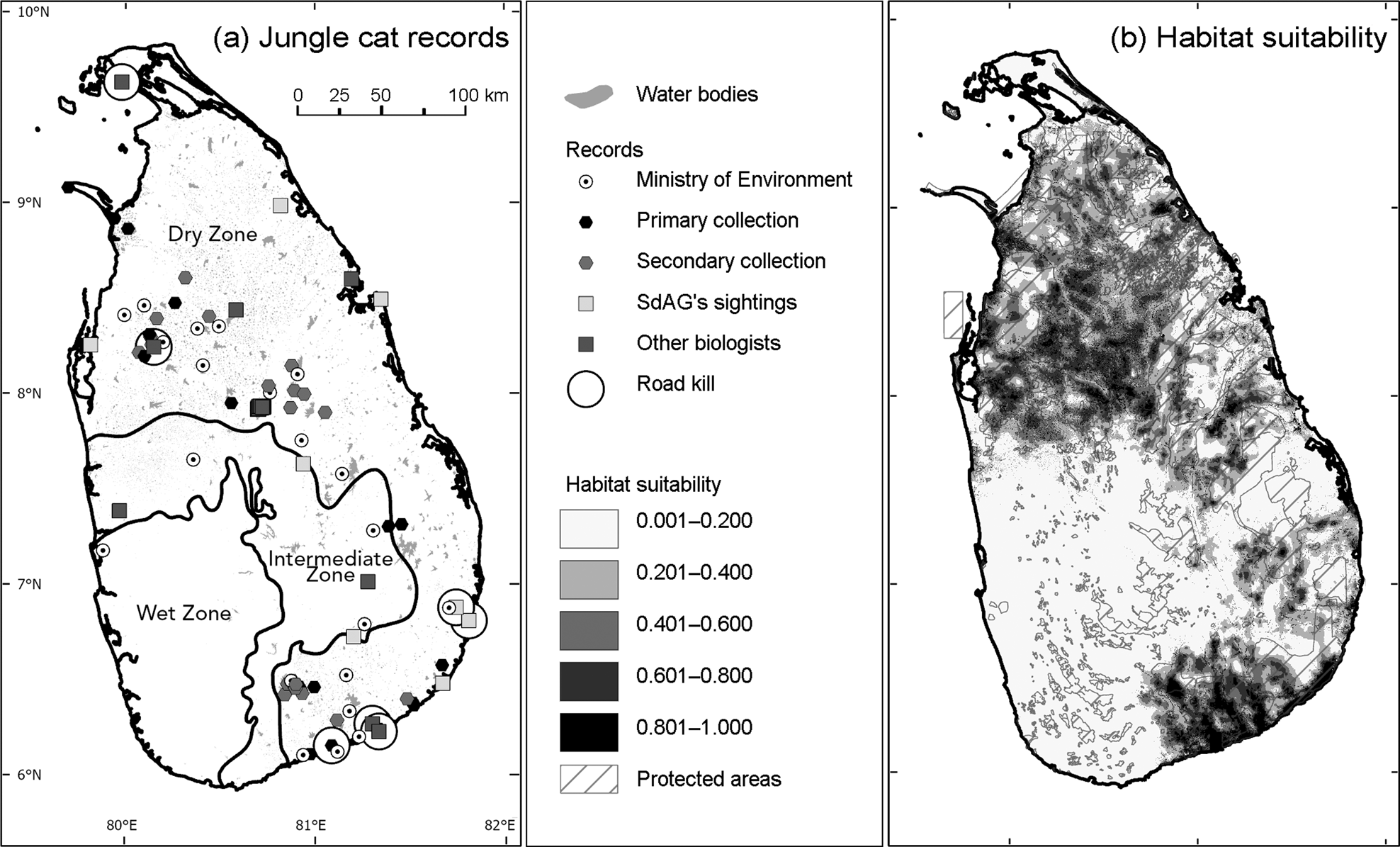
Fig. 1 (a) Water bodies in the study area, and records of the jungle cat Felis chaus from the Ministry of Environment Sri Lanka (2012), primary and secondary collections (see text for details), SdAG's sightings, other biologists and road kill. (b) Our model of predicted habitat suitability for the jungle cat in Sri Lanka. Protected areas from Ministry of Mahaweli Development and Environment (2016), and water bodies from Department of Agrarian Development (2011).
Methods
We conducted this study during 8 November 2016–18 March 2019, using three methods to obtain records of jungle cats. Firstly, we took advantage of increased interest in wildlife and wildlife photography, and the popularization of sharing wildlife-related photographs on social media. We created a website for this project, and through an e-mail campaign and advertisements and posts on Facebook (Meta Platforms, Menlo Park, USA), we invited wildlife and photography enthusiasts to submit geo-referenced photographs of the jungle cat and, if possible, to identify the habitat from among a given list of habitats (primary collection in Fig. 1; Miththapala, Reference Miththapala2016). Each submission was verified as a jungle cat by SM or SdAG, and uploaded onto an online map (Miththapala, Reference Miththapala2016). Secondly, we collected data on sightings from newspapers and social media pages (secondary collection in Fig. 1). We searched Instagram (Meta Platforms, Menlo Park, USA) for ‘#junglecat’, recording any sightings in Sri Lanka. We also used personal contacts made during fieldwork and through awareness programmes to request information (by phone or text) of any jungle cat sightings. We asked these contacts to use an online small wild cat sighting form, and verified records from submitted photographs. To enlist a wide audience, we made announcements on the organizational pages of Small Cat Advocacy & Research (2017), Urban Fishing Cat Conservation Project (2020), the Save Fishing Cats Conservation Project (2015) and other pages with an interest in wildlife news. Thirdly, we communicated with other field biologists, seeking additional sightings, and also included SdAG's own records of the jungle cat.
Using Arcmap 10.3.1 (Esri, Redlands, USA), we combined all new records for the jungle cat with those from the National Red List assessment for this species (Ministry of Environment Sri Lanka, 2012), obtained from the Biodiversity Secretariat of the Ministry of Mahaweli Development and Environment (Fig. 1a). We used these records to model habitat suitability for the species, using MaxEnt (Phillips et al., Reference Phillips, Anderson and Schapire2006). MaxEnt is a machine learning approach to modelling species habitat suitability using presence-only data and environmental covariates. MaxEnt models the probability density of environmental features at the locations of species records and at a large set of background points across the study area, and then attempts to find the broadest probability distribution to describe habitat suitability within the constraints of the environmental features (Elith et al., Reference Elith, Phillips, Hastie, Dudik, Chee and Yates2011).
We generated 13 environmental covariate layers to model habitat suitability (Table 1). We used a raster land-use layer of agricultural forest plantations, home gardens, grasslands, open forests/scrub forests, dense forests, marshes and paddy fields (Surveyor General, Survey Department, 2016), and a water features layer for reservoirs and streams/rivers (Department of Agrarian Development, 2011). To identify riverine forests, we trained a colleague to identify and digitize riverine forest habitat in Sri Lanka, using Google Earth (Google, Mountain View, USA), and we then visually checked the digitized areas (we were not able to ground-truth the data because of the lack of financial resources to do so). We calculated the Euclidean distance from each jungle cat record to the nearest riverine forest, marsh, paddy field, stream/river, and reservoir.
Table 1 Per cent contribution, in decreasing order, of 13 environmental covariates to the MaxEnt model of habitat suitability for the jungle cat Felis chaus in Sri Lanka. Density refers to the per cent coverage of a land cover within a 3.78 km radius (45 km2) of a particular location.

We transformed the land-use raster layer into a point layer and used a kernel density estimator to calculate the densities of plantation forests, home gardens, grasslands, open forests (which included arid-mixed evergreen forests, commonly called scrublands) and dense forests. We used a 45 km2 moving window with a radius (3.78 km) based on the approximate distance moved by individual jungle cats in a night (Sunquist & Sunquist, Reference Sunquist and Sunquist2002), the only information available on the species' home range. We followed a similar procedure to calculate road density using the Survey Department's database of Sri Lanka's roads (Surveyor General, Survey Department, 2016) but used linear rather than point density. All densities were transformed into km2, from which we calculated the per cent surface area of each land cover surrounding a point within a 45 km2 moving window. We used elevation data from the ASTER Global Digital Elevation Model (DEM) Version 2 to create a 30-m resolution raster layer of Sri Lanka. We obtained annual rainfall and temperature data for Sri Lanka from WorldClim 2.0 (Hijmans et al., Reference Hijmans, Cameron, Parra, Jones and Jarvis2005). Spatial correlations were assessed to reduce the multicollinearity of environmental covariates; one covariate was removed from a covariate pair if |r| ≤ 0.80. To reduce potential bias in the presence-only data as a result of unequal sampling effort, we used a spatial rarefication tool (SDMToolbox 2.4; Brown et al., Reference Brown, Bennett and French2017) to truncate one sighting from any pair of points that were within 1 km of each other. We used ArcGIS 2.2 (Esri, Redlands, USA) and R 3.6 (R Core Team, 2019) for processing spatial data and preparation for model input.
We ran MaxEnt models for a maximum of 1,000 iterations, including linear, quadratic, product and hinge features, and used default regularization settings (Elith et al., Reference Elith, Phillips, Hastie, Dudik, Chee and Yates2011). We used a 10-fold cross-validation method to estimate model error and predictive performance (Rodriguez et al., Reference Rodriguez, Perez and Lozano2010; Pascoe et al., Reference Pascoe, Marcantonio, Caminade and Foley2019). This procedure runs 10 iterations of the same set of model variable inputs, but with different training and testing subsets per iteration. We created and ran models with different sets of variable inputs. We assessed model performance and selected a model structure by using the highest mean area under the receiver operating characteristic curve (AUC) and if all variables had a positive influence on the regularized training gain of the model; AUC values range from 0 to 1, with 0.5 indicating that the model is no better than random at predicting habitat suitability and 1 indicating a perfect ability of the model to estimate species presence (Phillips et al., Reference Phillips, Anderson and Schapire2006). Regularized training gain is a measure of the increased model fit of the MaxEnt model distribution to the species locations versus the fit of a uniform distribution to the species locations, with a penalty factor. Higher values of regularized training gain for a model with one specific variable is an indication that variable is of greater importance in modelling habitat suitability (Kisssel & Poserina, Reference Kisssel and Poserina2017).
Results
We collated 112 jungle cat records spanning 2016–2019, a substantial increase compared to the 21 sightings used for the species assessment on the National Red List (Ministry of Environment Sri Lanka, 2012; Fig. 1a, Plate 1). We verified records from photographs received from the public and from photographs in newspapers and on social media, and accepted some records without supporting photographic evidence if the submitter's field identification knowledge and experience was known to us. These records expand the known range for this species in Sri Lanka and also show that it occurs outside protected areas (Fig. 1a). The jungle cat appears to be a habitat generalist, with records in a range of open habitats, including human-dominated areas such as paddy fields and near human-made water reservoirs, and in forests (S. Miththapala et al., unpubl. data, 2022). Eight of the 112 records (7.1%) were road kills.
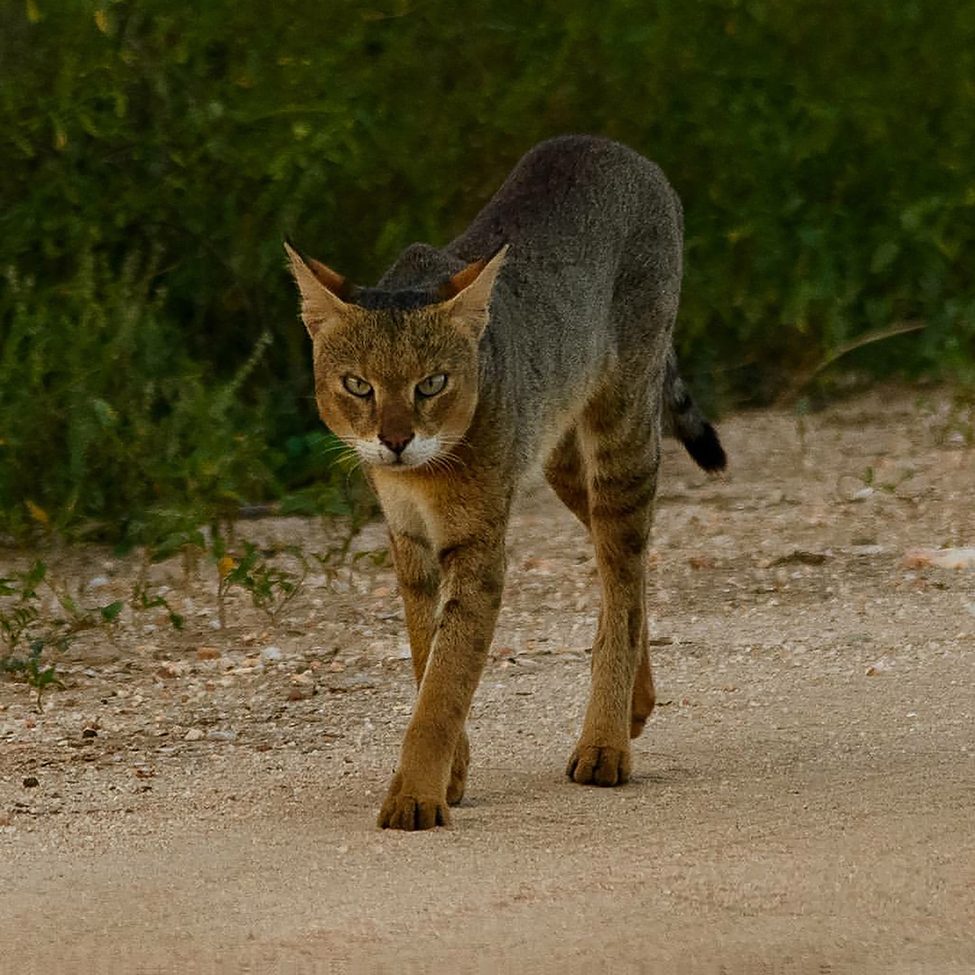
Plate 1 A sample photograph, provided by a member of the public, taken within Udawalawe National Park, away from any human habitation (Photo: Chitral Jayatilake, 2016). We identified this individual as a jungle cat by its long legs, ear tufts, tawny head and part of the legs, most of the body grey, black and white speckled, few stripes on the legs and tail, and plain coat. The practice of hybridizing domestic with wild cats is not known in Sri Lanka (Phillips, Reference Phillips1935; Yapa, Reference Yapa and Ratnavira2013) and therefore we are confident this is not a hybrid. Furthermore, this photograph was taken deep within the National Park, where it is unlikely that natural hybridization would occur.
The best MaxEnt model had a mean AUC of 0.873, indicating the model had a relatively strong diagnostic ability to predict habitat suitability (Fig. 1b, Supplementary Fig. 1). The model included 13 environmental covariates, with six of these having > 80% cumulative contribution to the model (Table 1, Supplementary Fig. 2). Distances to nearest riverine forest and reservoir contributed the most to the model and were both negatively associated with habitat suitability for jungle cats (i.e. increasing distances from these habitats resulted in decreased habitat suitability). The highest probability of presence was within 5 km of riverine forests and in proximity to a reservoir (Supplementary Fig. 2). Annual rainfall and the density of agricultural forests (rubber, palmyra, coconut, cashew, mixed-tree and other perennial crops) were also high contributors to the model (Table 1, Supplementary Fig. 3). The highest probability of presence was in areas that received 500–1,000 mm of rainfall per year and had the lowest densities of plantation forests.
Relative to other covariates, the densities of roads, open forest and home gardens contributed little to the final model. Road density showed a strong negative relationship with habitat suitability when road density was the only variable considered in the model but this became a positive relationship when all other variables in the model were included. Habitat suitability was negatively correlated with the density of home gardens. When the density of home gardens was > 33%, habitat suitability was < 0.50.
Discussion
Our findings validate our hypotheses that the jungle cat is more widespread than shown in the current Sri Lanka National Red List and than reported by Kittle & Watson (Reference Kittle and Watson2018), and that the species appears to have a tolerance for human activities as it was sighted in human-dominated landscapes. Figure 1a shows the jungle cat's preference for the drier parts of Sri Lanka, with most sightings in the dry zone. The habitat suitability model also indicated the species’ preference for the dry zone (Fig. 1b, Table 1, Supplementary Fig. 2).
The jungle cat has also been referred to as the swamp cat or reed cat because of its occurrence in swamps and among reeds (Sunquist & Sunquist, Reference Sunquist and Sunquist2002; Prisazhnyuk & Belousova, Reference Prisazhnyuk and Belousova2007; Sanei et al., Reference Sanei, Mousavi, Rabiee, Khosravi, Julaee and Gudarzi2016), and it has also been recorded near rivers or wetlands (Hatt, Reference Hatt1959; Harrison, Reference Harrison1968; Mendelssohn, Reference Mendelssohn1989; Dayan et al., Reference Dayan, Simberloff, Tchernov and Yom-Tov1990; Heptner & Sludskii, Reference Heptner and Sludskii1992; Sunquist & Sunquist, Reference Sunquist and Sunquist2002; Prisazhnyuk & Belousova, Reference Prisazhnyuk and Belousova2007; Avgan, Reference Avgan2009; Gerngross, Reference Gerngross2014; Sanei et al., Reference Sanei, Mousavi, Rabiee, Khosravi, Julaee and Gudarzi2016). We only recorded one sighting in a riverine habitat, but the habitat suitability modelling indicated a high probability of presence within 5 km of riverine forests and in proximity to a reservoir (Table 1, Fig. 1b, Supplementary Fig. 2). However, the habitat suitability model prediction must be interpreted with caution, as we were not able to ground-truth the digitized satellite data. The jungle cat also appears to use a range of open habitats, including human-dominated areas in Sri Lanka (S. Miththapala et al., unpubl. data, 2022), as shown in other parts of its range (Patel, Reference Patel2011; Kalle et al., Reference Kalle, Ramesh, Qureshi and Sankar2013; Sanei et al., Reference Sanei, Mousavi, Rabiee, Khosravi, Julaee and Gudarzi2016; Mishra et al., Reference Mishra, Gautam, Shah, Subedi, Pokheral and Lamichhane2020; Shrestha et al., Reference Shrestha, Subedi and Kandel2020).
Our finding that the jungle cat occurs both within and outside protected areas parallels studies in Sri Lanka of other carnivores such as the leopard (Kittle et al., Reference Kittle, Watson, Cushman and Macdonald2018), fishing cat (Urban Fishing Cat Conservation Project, 2020) and elephant Elephas maximus (Fernando et al., Reference Fernando, Silva, Jayasinghe, Janaka and Pastorini2021). However, the presence of these species in human-dominated landscapes can result in negative interactions with people. In 2019, for example, 405 elephants (Prakash et al., Reference Prakash, Wijeratne and Fernando2020), 12 leopards (A. Kittle, pers. comm., 2021) and 22 fishing cats (Small Cat Advocacy & Research, 2020) were killed as a result of human–wildlife interactions. Research is required to investigate whether the jungle cat is also affected in this way.
Eight of our records were road kills. Of these, six were along the south-east coastline, probably because there are good roads and little traffic in this area, facilitating fast driving. Road kills, and irresponsible driving within national parks, are threats to wildlife in Sri Lanka (Karunarathna et al., Reference Karunarathna, Ranwala, Surasinghe and Madawala2017) and elsewhere (Filius et al., Reference Filius, van der Hoek, Jarrín and van Hooft2020; Schwartz et al., Reference Schwartz, Shilling and Perkins2020; Ascensão et al., Reference Ascensão, Yogui, Alves, Alves, Abra and Desbiez2021). An additional threat to the jungle cat is the decline of its prey as a result of deforestation and consequent killing by farmers in retaliation for preying on livestock (de Alwis, Reference de Alwis and Eaton1973). Habitat destruction has not abated since 1973 and remains the prime driver of ecosystem change in Sri Lanka (Ministry of Mahaweli Development & Environment, 2016). An additional threat is the killing of jungle cats by feral dogs (Bambaradeniya & Amerasinghe, Reference Bambaradeniya and Amarasinghe2001).
Globally, funding for research on biodiversity is inadequate. In Sri Lanka most of the limited funds available are spent on exploration for new species (e.g. fish: Sudasinghe, Reference Sudasinghe2018; amphibians: Senevirathne et al., Reference Senevirathne, Samarawickrama, Wijayathilaka, Manamendra-Arachchi and Bowatte2018; reptiles: Mendis Wickramasinghe et al., Reference Mendis Wickramasinghe, Vidanapathirana and Wickramasinghe2020; mammals: Meegaskumbura, Reference Meegaskumbura, Meegaskumbura, Pethiyagoda, Manamendra-Arachchi and Schneider2007) or studies of threatened species (e.g. leopards: Kittle et al., Reference Kittle, Watson, Cushman and Macdonald2018; elephants: Fernando et al., Reference Fernando, Silva, Jayasinghe, Janaka and Pastorini2021). Studying common species is not a priority, even though by studying such species and planning for their needs before they become rare, future conservation funds can be conserved (Scott et al., Reference Scott, Davis, Csuti, Noss, Butterfield and Groves1993; Redford et al., Reference Redford, Berger and Zack2013). Our study provides a model for how ecological and behavioural information for common species could be obtained inexpensively and incorporated into species distribution models. From our findings we will be able to develop more precise hypotheses about habitat dependencies, and employ camera traps and GPS collars on individuals in specific locations (S. Miththapala et al., unpubl. data, 2022) to investigate the species’ ecology and behaviour. Studies of species such as the jungle cat, which are neither threatened nor charismatic, will help ensure that we are ‘keep […] common species common’ (Ramesh & McGowan, Reference Ramesh and McGowan2009, p. 108; Frimpong, Reference Frimpong2018; USGS, 2018; Baker et al., Reference Baker, Garnett, O'Connor, Ehmke, Clarke, Woinarski and McGeoch2019).
Acknowledgements
We thank the Mohamed bin Zayed Species Conservation Fund (grant number 160513505) for financial support; the Biodiversity Secretariat for providing us with the 2012 Red List map for Felis chaus; Isuru Dayananda, Saranga Dissanayake Suresh Ellawala, Wasiri Rasu Gajaman, Anton Gihan, Shelley Glass, Medhisha Pasan Gunawardena, Chaminda Jayasekera, Chitral Jayatileke, Eric Joseph, Namal Kamalgoda, Sunitha Kanthi, Moditha Hiranya Kodikara Arachchi, Dineth Mallikarachchy, Srilal Miththapala, Luxshmanan Nadaraja, Abhaya Indika Nettigama, Madduma Wellalage Tiron Premajith, Stuart Peoples, Madura Priyankara, Phil Rekret, Oliver Rook, Danushka Senadeera, Pasan Senevirathne, Aisha Uduman, Milinda Wattegedera, Mithra Weerakone, Rajiv Welikala Romesh Wijesiri and Christopher Young for data collection; Chandima Fernando, Manori Gunawardena, Andrew Kittle, Enoka Kudawidanage and Sampath Seneviratne for sharing their sighting data; Virginia Hayssen and Angie Appel for sharing references; Rob Baldwin, Janaki Galappatti and John Seidensticker for reviews; and Rob Baldwin for providing technical support for habitat suitability modelling.
Author contributions
Funding acquisition: SM, NM; study design, supervision: SM; data collection: SM, AAWR, AT, SdAG; data analysis: NL, JD, DW, SdAG; writing: SM, JD, AAWR, SdAG; website construction and maintenance: NM.
Conflicts of interest
None.
Ethical standards
No specimens were collected during this research, which otherwise abided by the Oryx guidelines on ethical standards.