“Facts do not cease to exist because they are ignored.”
(Aldous Huxley)Introduction: jumping on the bandwagon
As Zagzebski formulated it, knowledge is cognitive contact with reality (Zagzebski, Reference Zagzebski, Blake, Smeyers, Smith and Standish2017). The two sides of it are knowledge by acquaintance and knowledge by proposition. Comprehending the entirety of knowledge is an evergreen topic and a challenging undertaking. From the antique time until our post-modern time, it is continually in the focus of scientific, epistemological, cognitive, computational, industrial, and societal studies, debates, and speculations (Cassam, Reference Cassam2007). We know a lot about knowledge, but we cannot know everything about it due to its complexity and evolving nature. The perpetual evolution of knowledge involves exploration, structuring, consolidation, articulation, and rectification (Oeberst et al., Reference Oeberst, Kimmerle, Cress, Cress, Moskaliuk and Jeong2016). A unique area of studies is the classification and qualification of knowledge (De Jong and Ferguson-Hessler, Reference De Jong and Ferguson-Hessler1996). Above any disciplinary relatedness, Farnham-Diggory has identified: (i) declarative, (ii) procedural, (iii) conceptual, (iv) analogical, and (v) logical knowledge paradigms (Farnham-Diggory, Reference Farnham-Diggory1994). With a view to inquiry in scientific disciplines, Fergurson-Hessler and de Jong identified four types of domain-independent knowledge, namely (i) declarative (knowing that), (ii) procedural (knowing how), (iii) schematic (knowing why), and (iv) strategic (knowing when and where) knowledge (Fergurson-Hessler and de Jong, Reference Fergurson-Hessler and de Jong1990). Whatever categorization have been proposed, they focused on human knowledge and identified its fundamental constituents. In the last decades, computer-based collection, representation, processing, communication, and storage of human knowledge were the most important issues for knowledge science, knowledge engineering, and knowledge management. In turn, this has changed the status and the role of human knowledge. By now, the sophistication and intellectualization of knowledge-related and knowledge-based systems have reached a remarkable level. This process moving from debating philosophers to concrete knowledge machines was addressed in the work of Ahamed (Reference Ahamed2017).
In a nutshell, the essence of the changes is that in addition to (i) human ↔ knowledge relationships, also (ii) human ↔ knowledge ↔ system relationships, and (iii) system ↔ knowledge relationships have emerged. The human ↔ knowledge relationships mean that the creator, owner, and user of knowledge is human (involving individuals, groups, and populations). The human ↔ knowledge ↔ system relationships designate a transfer of human knowledge into artificial systems, so as knowledge creates a cognitive bridge between these parties. Human is the initial source of knowledge, which is structured and represented by humans for computational processing by systems, and the results of processing are availed for humans to harvest the benefits. In a next stage of technological development, systems become capable of generating knowledge on their own (Ahamed, Reference Ahamed2017). The system ↔ knowledge relationships mean that the creators of knowledge are some sort of intellectualized engineered systems (IESs), which commence with pre-programmed human knowledge and complement it with self-acquired synthetic system knowledge (SSK), and use this compound knowledge for decision-making in problem-solving or may avail it for humans as recommendation or problem solution (Brown, Reference Brown2020). This trend exemplifies the abovementioned changes in the status and the role of human knowledge. System knowledge is rapidly evolving due to the fast development of artificial intelligence applications and run-time self-adaptive operation of IESs (Ford, Reference Ford1983; Laird, Reference Laird2020).
With a view to its nature, this paper is a so-called academic position paper (APP). Typically, an APP addresses an observable particular phenomenon and recommends a course of action related to it. It tries to convince the audience that the presented view or opinion is valid and worth dealing with, without the need to present completed research work and/or validated results. The author provides an arguable opinion about the related issues as well as evidence to ensure the validity of his claims. The paper is structured accordingly. The next section further elaborates on the above developments and provides an account on their implications. The main observation is that, even if complemented with the knowledge of constructive disciplines, the generally accepted genres of disciplinary knowledge cannot cover SSK. Furthermore, it is argued that current theories of knowledge are not able to capture the specificities of system-generated knowledge. The research context and the addressed research questions are presented in the section “Presenting the research context and questions”. Sections “Alpha-knowledge” “Beta-knowledge”, and “Gamma-knowledge” discuss the nature and essence of the alpha, beta, and gamma (ABG) disciplines and genres of knowledge, while the section “Delta-knowledge” focuses on the delta-knowledge. The section “Epsilon-knowledge: what makes it legitimate?” concentrates on the emergence and the specificities of SSK and proposes to recognize it as an additional genre, that is epsilon-knowledge. The section “Computational ways of generating epsilon-knowledge” discusses the generic sources and typical ways of computationally generating SSK. The section “Action follows belief: issues for sympérasmology” approaches epsilon-knowledge from the perspectives of sympérasmology and identifies topics and issues for sympérasmological studies. The section “Ad perpetuam memoriam: reflections and conclusions” summarizes the findings, compares the features of epsilon-knowledge with the other genres, and formulates some implicative propositions.
Presenting the research context and questions
There were many efforts in the past to understand and rationally reconstruct human intellectual experience and to map the entire landscape of human knowledge onto a reasonable and useful chart (Stroud, Reference Stroud, Clay and Lehrer1989). This striving after exploring the structure of the whole of human knowledge, and making it transparent and qualified, required a robust identification of its genres and imposing some forms of categorization. A fundamental categorization was introduced by Machlup (Reference Machlup1980, Reference Machlup1982, Reference Machlup1984). This conceptualization of the genres of knowledge was considered as a model for the interpretation of academic disciplines. This can be traced back to the second half of the nineteenth century when the professionalization of science and organization of high-level educational institutions became a social concern. Machlup provided a comprehensive analysis of the essence, types, classes, forms, meaning, appearances, and qualities of knowledge. His reasoning model is visualized in Figure 1. In addition to general (or common sense) knowledge, he tentatively identified (i) humanistic knowledge, (ii) scientific knowledge, and (iii) social science knowledge as three fundamental genres of structured and learnable knowledge, giving no attention to other types of knowledge such as religious and technological knowledge. These genres of knowledge of disciplinary relevance have been adopted in various countries in Europe, in particular in the Netherlands, to articulate the educational structure (Lange et al., Reference Lange, De Bont, Filatova and Katsonis2018).
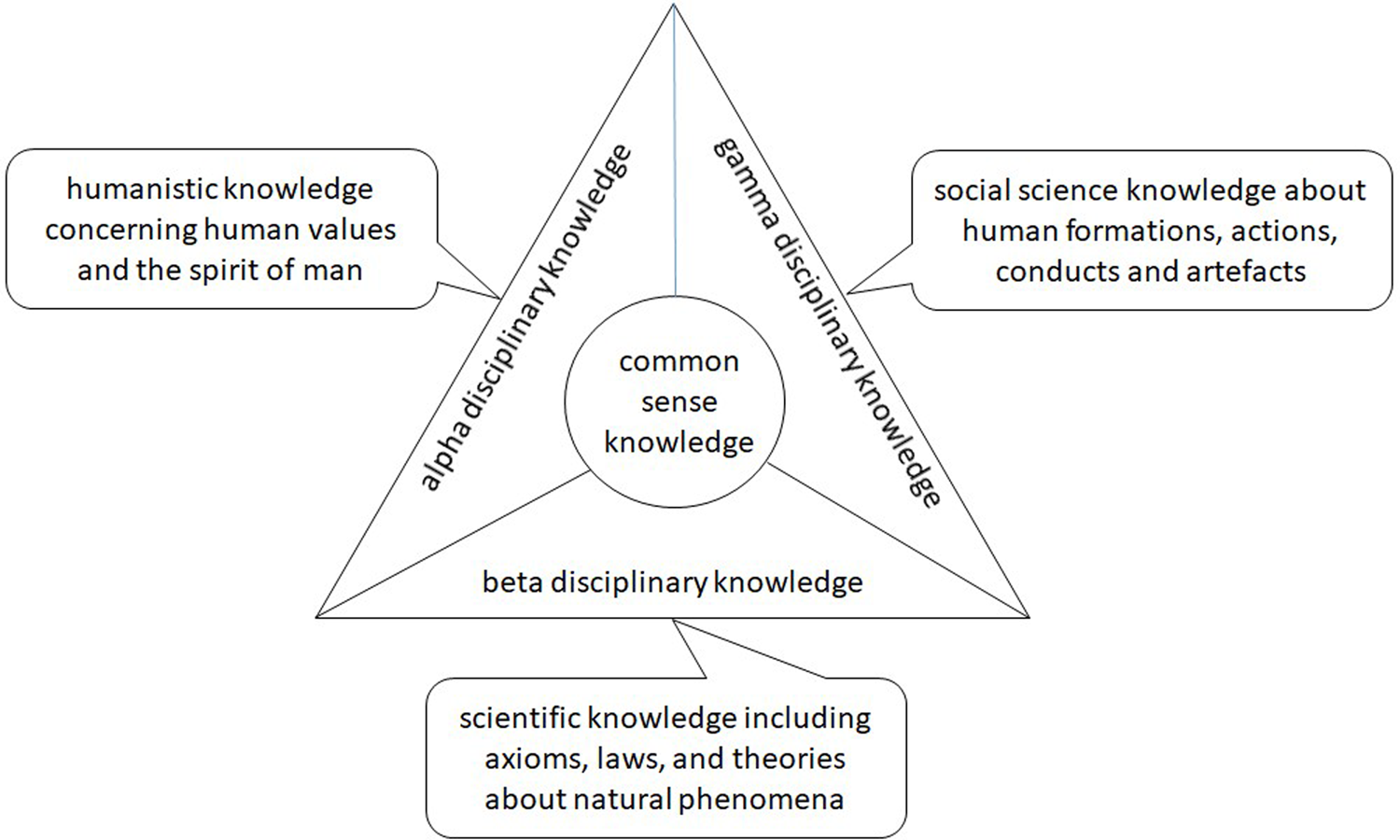
Fig. 1. Three genres of human knowledge according to Machlup. F.
Alpha-disciplines are in the domain of humanities such as languages, literature, philosophy, history, and archaeology (as products of human action). Concentrating on the nonhuman nature, the beta-disciplines include exact, physical, chemical, biological, engineering, computer sciences, and information technology. Gamma-disciplines study human activity and behavior including psychology, medicine, health, pharmaceutics, sociology, anthropology, law, politics, administration, economics, education, and media. It can be felt that this categorization of genres of human-inquired knowledge focuses primarily on the naturally prevailing and historically existing world, rather than on the human-created world. Although Machlup identified humanities, sciences, and social sciences as three distinct fields of academic learning and knowing, social sciences have many things in common with sciences as well as with humanities. Their methodological approaches resemble what is typical for beta-disciplines, while the subjects of their study coincide with those of humanities. In addition, many interdisciplinary, multi-disciplinary, and transdisciplinary disciplines have emerged over the years, which make the disciplinary boundaries blurred.
Toward the end of the last century, it was realized that the knowledge of practice-driven creative and decision-making disciplines does not trivially match the knowledge of the ABG disciplines. A strong and lasting academic debate was initiated in the field of design. The discussions were stimulated about “design science” and “science of designing, and the epistemology of design knowledge by papers such as Archer (Reference Archer1979), Toulmin (Reference Toulmin1988); Cross (Reference Cross1993); Sarlemijn (Reference Sarlemijn, de Vries, Cross and Grant1993), and Zack (Reference Zack1999). This resulted in an “identity movement” in this field that included both design practice and design research, and it has its influence on thinking about management and organization disciplines. It is a fact that the classical definition of knowledge excludes those forms that cannot be straightforwardly justified or need not necessarily be subject of justification such as design ideas, prospective decisions, or value judgments. The fact of the matter is that, besides the countless publications from British scholars, the rest of the world was also busy with issues related to design science, designerly knowledge, systematization of design processes, and consideration of stakeholders in design problem-solving. The works of Yoshikawa, Andreassen, Hubka and Eder, Roth, Rodenacker, Pahl and Beitz (just to mention a few) are also remarkable contributions (Roth, Reference Roth1981; Rodenacker, Reference Rodenacker1984; Hubka and Eder, Reference Hubka and Eder1987; Yoshikawa, Reference Yoshikawa1989; Beitz and Pahl, Reference Beitz and Pahl1996; Andreasen, Reference Andreasen2011). The disciplines of design belong to a wider abstract group of constructive disciplines, which is only vaguely defined (Mutanen, Reference Mutanen2019). In general, constructive disciplines deal with what is physically possible and feasible, while experimental disciplines study what is physically and logically probable. The latter study the world as is, while the former form it so as (believed) to be. Experimental disciplines are not absolutely free of constructing, but it typically concerns only the research models and the research designs. However, what differentiates construction in the constructive sciences is that it extends from constructing the research designs to embedded utilizations of the inquired knowledge. These together stimulated the author to seek for answer to the following question: if the genres of disciplinary knowledge, as introduced by Machlup, and the extension of this categorization with the knowledge of constructive sciences are given, can SSK be regarded as part of one of these or the whole formed by the genres, or does it represent a genuine new form of knowledge?
Nowadays, everybody knows that artificial intelligence refers to the physical, perceptive, and cognitive abilities of IESs to sense, interpret, reason, plan, act, and adapt smartly/intelligently, including making context-dependent decisions, and carry out problem-solving tasks (Rapaport, Reference Rapaport2020). They are considered smart or intelligent if their behavior resembles what a human does. It seems that, after some two decades of Artificial Intelligence-winter, a blossoming spring is out there and a never-experienced, but a justifiable hype is promised (Hendler, Reference Hendler2008). Most of the advocates argue with the novel data-driven nature, autonomous learning capabilities, and application-dependent reasoning mechanisms of AI-based systems and smart cyber-physical systems. The fact of the matter is that AI has become a prosperous industry. However, AI serves not only the empowering and automation of the industry, but also the well-being of the entire society. In addition to the inventions of the “Big Nine” [i.e. the large industrial corporations in the US or China including (in alphabetical order) Alibaba, Amazon, Apple, Baidu, Facebook, Google, IBM, Microsoft, and Tencent], smart cleaners, self-driving cars, grass cutters, recommendation systems, home robots, and so forth are appearing in everyday households (Brown, Reference Brown2020). These AI-based solutions not only “consume” data, but also generate and aggregate SSK of all kinds. What it means is that both the variety and the amount of SSK are growing rapidly due to the intense research in systelligence (Soni and Singh, Reference Soni and Singh2015).
To distinguish SSK from the other genres, it is proposed to call it “epsilon-knowledge”. In the next sections, three major research questions are addressed, namely:
• What does differentiate SSK from the other genres of knowledge?
• What are the reasons to identify it as a distinct genre?
• What sympérasmological studies are needed to investigate SSK?
The main argument of this paper is that the conversion of SSK into a productive asset needs, before everything such as (i) ontological clarification, (ii) a knowledge theoretical disposition, and (iii) tailored knowledge engineering methodologies. These all are important since the literature suggests that SSK has unique features in comparison with those of the ABGD genres of knowledge. The comparative analysis presented in the rest of the paper intended to show that SSK indeed represents a different genre. Starting out from the cognitive framework of sympérasmology, the paper will identify and propose some important issues for further studies (Horváth, Reference Horváth2020).
Alpha-knowledge
Representatives of humanities (also called alpha-disciplines) are such as art, archaeology, folklore, history, journalistic, linguistics, literature, logic, metaphysics, music, philosophy, ethics, and theology. Humanities conduct studies how people express, understand, process, document, and record human experience in various modalities. These modalities include beliefs, speculations, language, literature, music, faith, creative art, performing art, history memos, to name but the most evolved ones (Agresto, Reference Agresto1983). Knowledge delivered by humanities has both an expressional and a sensational perspective. They together create potential connections between humans uttering experience and humans perceiving the utterance, over space, time, and mind-sets. Humanities reflect not only experience, but also diverse ideologies, cultures, heritages, traditions, and histories (Levi, Reference Levi1983). They help understand what it is to be human and to make sense of the human experience in general and our individual experiences in particular. Myers emphasized that there is vagueness and uncertainty about the nature of the contribution of humanities to the academic community and to the wider world of human affairs (Myers, Reference Myers1967). Notwithstanding, humanities have significantly contributed to human social developments and formations by influencing purposes, values, norms, and meanings. In our post-modern time, the attitude toward traditional humanities and their role in the society and education have changed (Held, Reference Held2020). As early as 1944, it was observed that the weakest point in discussions of the role of the humanities is differentiation between (i) humanities as a distinct group of literary and artistic subjects and (ii) the humanistic spirit or ideal that is influencing the thinking and value system of broad-minded or narrow-minded individuals (MacKinney, Reference MacKinney1944).
For the reason that the humanities are not merely academic disciplines, but also important intellectual components of societies, the knowledge associated with them is rather versatile (Vaziri et al., Reference Vaziri, Tay, Keith and Pawelski2019). It entails that alpha-knowledge is varied because it belongs to various disciplines of humanities. Broderick argued that humanities embrace areas of human knowledge that feature the following characteristics: (i) central concern is human beings rather than the processes of nature or the structures of society, (ii) primary focus is on the individual rather than on the group, (iii) awareness (quite self-conscious awareness) of how we know what we know, (iv) attention to moral values, whether drawn from God, man, or nature, and (v) insistence that the process of intellectual growth calls for forthright moral judgments as an equal part (Broderick, Reference Broderick1983). Humanities work through four mechanisms: (i) immersion, (ii) embeddedness, (iii) socialization, and (iv) reflectiveness. Typical methods of dealing with alpha-knowledge are interpretational (criticism), comparative (affects), and speculative (contemplation) in nature, though anthropology, archaeology, and jurisprudence use methods, which have scientific flavor. One kind of knowledge is the result of storytelling – telling the story of human individuals, communities, and societies, and the story of humanity. This is a descriptive knowledge, which may appear in many alternative representations (text, movie, stage play, etc.). A part of humanities knowledge rests on facts and causalities, while the other part on ideas and visions, or the mixture of these (Boas, Reference Boas1957). Furthermore, many disciplines of humanities reflect the duality of art and craft (Smith, Reference Smith2015). It is also worth mentioning that several studies refuted the universality of knowledge in the humanities and social sciences, and that the contextuality of knowledge affirms “the social construction of knowledge” or “the enacted social knowledge”, though they do not object that belief is a necessary condition for knowledge (Wray, Reference Wray2007).
Beta-knowledge
It is well known that the scientific enterprise is a historical evolution – a result of the ever-continuing endeavor (i) to understand the natural world existing around us, (ii) to produce and accumulate scrutinized knowledge as an end, and (iii) to become able to do and make more (Losee, Reference Losee1987). Like humanities, science is also a philosophical category, which is related to the state or fact of knowing (Lakatos, Reference Lakatos1970). Eventually, science is the sum of what is known by the society, or the units and individuals of the society. Modern science has not only empirical and rational bases, but also computational inferences and massive data streams as bases (Džeroski et al., Reference Džeroski, Langley, Todorovski, Džeroski and Todorovski2007). It provides us with the epistemologically most warranted statements on the natural world, the human beings, human societies, human physical constructions, and human thought constructions that can be deemed as reliable at the time of being. In the Middle-Ages, the seven liberal arts represented what we call science nowadays. It constituted of (i) the trivium (grammar, logic, and rhetoric) and (ii) the quadrivium (arithmetic, music, geometry, and astronomy). After a long period of steady progression, the diversification and articulation of science accelerated in the last century (Popper, Reference Popper1962). In our time, typical representatives of scientific disciplines are such as agriculture, astronomy, behavioral science, biology, chemistry, computing, engineering, medicine, meteorology, oceanography, physics, physiology, and zoology.
The scientific approach of inquiry entails the embodiment of commitment to evidence in the process. In other words, the process of inquiry is featured by the quest for the “rationally agreeable and empirically evidential” truth in the scientific disciplines. The issue of truth appears differently in different scientific disciplines and contemplated differently in formal sciences (mathematics, logics), natural sciences (physics, chemistry, biology), and applied sciences (materials, technologies, energies, machines). Disciplines of formal science deal with proof, while natural and applied sciences seek evidence. In technical sciences (e.g., astronautics, aviation, electronics, computer science, and metallurgy), reliable knowledge is derived from the knowledge of natural and abstract sciences and is strongly contextualized by application-oriented thinking. Formal (also called abstract or exact) sciences (e.g., metaphysics, ontology, cosmology, mathematics, information theory) study abstract (intellectual) worlds in their own right and rationality (Pruzan, Reference Pruzan2016). Moreover, the meaning of truth varies in different times and according to philosophical positions (McCain, Reference McCain2016).
Machlup called the knowledge explored and aggregated by the abovementioned disciplines as beta-knowledge (Machlup, Reference Machlup1982). This genre of knowledge has a strong epistemology that clarifies not only its sources, but also the way of coming to know it, and the issues related to its justification, validation, and consolidation (French, Reference French2007). What makes various bodies of scientific knowledge scientific is that they share the following characteristics: (i) objective, (ii) rational, (iii) systematic, and (iv) universal. Objective means that: (i) phenomena are studied in an unbiased manner by alternative methods, (ii) the findings are scrutinized against empirical evidence, and (iii) the agreement of the concerned scientific community is sought to accept results as “properly justified correct belief” or “relative scientific truth”. Rational expresses the condition that any research work and its results should be reproducible either in a concrete or in an abstract way, or in both. Systematic implies that the results are supposed to be generated in a systematic way to match an existing paradigm or to create a new one. Universal means that the results of scientific research are expected to be universally applicable and the conditions of usability have to be precisely stated. These, together with commitment to evidence, are often referred to as foundational criteria of science.
Epistemologists typically distinguish three kinds of knowledge. These distinct kinds of knowledge are (i) acquaintance knowledge, (ii) knowledge-how, and (iii) propositional knowledge (French, Reference French2007). Beta-disciplines are concerned with propositional knowledge, which is considered historically as properly justified correct belief (Gordon, Reference Gordon2012). Schwitzgebel distinguished (i) representationalism, (ii) dispositionalism, (iii) interpretationism, (iv) functionalism, (v) eliminativism, and (vi) instrumentalism as main explanatory theories (philosophical stances) of beliefs (Schwitzgebel, Reference Schwitzgebel, Zalta, Nodelman, Allen and Perry2006). Based on the work of McCain, Ardourel elaborated on (i) representationalism (mental models), (ii) behavioralism (determination by characteristics), and (iii) eliminativism (folk psychology) (Ardourel, Reference Ardourel2017). Scientific research is supposed (i) to produce objective, general, testable, and complete scientific knowledge in various study (context) domains and (ii) to apply, consolidate, exploit, and monitor that knowledge. In line with the progression of studying a “scientific” phenomenon, scientific theories may convey (i) discovery, (ii) description, (iii) explanation, (iv) prediction, and (v) regulation (Horváth and Duhovnik, Reference Horváth and Duhovnik2005). A scientific theory must be based directly or indirectly on evidence from experiments, which supports or potentially falsifies the theory. Scientific theories are justified based on explanatory evidences. The lowest bound is that facts, laws, and theories should achieve significantly better accuracy of statistical prediction than random intuitive guesswork based on prior knowledge.
As mentioned above, scientific truth is a central issue of science philosophy (Mackinnon, Reference Mackinnon1982). It is usually seen as just one specific form of truth that is unconditionally accepted in a framework of reasoning based on evidences. Other forms of truth are (i) personal truth (true for one individual, no matter if can be proven, or if it has any meaning to anyone else), (ii) fictional truth (raising the feeling that something is true in a view, condition, and environment), and (iii) pseudo truth (stimulated by external influence, information, thinking, and behave differently). Facts, laws, and theories are the kernels of scientific knowledge. As empirical evidence, scientific fact is a category of the constituents of scientific knowledge and is derived by proper and repeatable observation, experimentation, and measurement processes.
Scientific theories include statements that (i) explain all the facts in a given context, (ii) logically relate the facts based on their content, (iii) make the applicability of the theory clear, (iv) indicate what is left over of the theory, and (v) trigger hypotheses that can extend the theory to cover a broader field. In the correspondence view of science, scientific laws capture the fundamental relationships concerning phenomenon and describes what happens, whereas scientific theories explain why and how a phenomenon happens. Science handles theories as comprehensive explanations of phenomena, which are based on observations and thoroughly tested against evidence from natural or man-made world, or alternatively from experiments conducted in laboratories and with predictive power. Utility of facts, laws, and theories is playing a crucial role in the (causal) model building and thinking capability of science (Homer, Reference Homer1996). Individuals and teams may initiate research data, hypotheses, and theories, but the consolidation of their scientific value is in the hand of the scientific community and the users. This is an element of the process of social construction of scientific knowledge (Mendelsohn, Reference Mendelsohn, Mendelsohn, Weingart and Whitely1977). In the consensus view of science, though based on empirical evidence, knowledge is tentative, theory-laden, view related, and technology dependent (Rosenberg, Reference Rosenberg2000).
While “truth-realists” argue that scientific theories are true or false, van Fraassen insisted that the appropriate contrast is between theories that are empirically adequate and those that are not (Van Fraassen, Reference Van Fraassen2002). Thus, an empirically adequate theory is a theory that is successful in saving the relevant phenomena. Siegel suggested that some contemporary philosophers of science challenge that validating a scientific belief is the hallmark of any rational scientific activity or, alternatively, that its rationality is not a function of its approach (Siegel, Reference Siegel1989). Hansson called everything that deviates substantially from the three major types of quality criteria of science – that is (i) reliability, (ii) fruitfulness, and (iii) practical usefulness – as pseudoscience (which puts forward a demonstrably false doctrine or information and claims it to be scientific) (Hansson, Reference Hansson, Pigliucci and Boudry2013). In his view, a statement is pseudo-scientific if it (i) pertains to a phenomenon investigated in a domain of science, (ii) is unreliable up to the level that it cannot be trusted, and (iii) represents a deviant doctrine from the mainstream approaches of a domain of science. In the view of these, nonscientific bodies of knowledge are not necessary unscientific, but their contribution to empirical scientific development is low.
Modern science is not only an exploratory cognitive activity of humans, but also the operation of the social and cultural institute toward consolidating the scientific activities and creating a system of scientific knowledge. Though both the traditional conceptualizations and the more recent interpretations of knowledge feature an individualistic focus (and methodological individualism), it is evidential that knowledge is created by collaboration of people and refined by a crowd of debating professionals in the overwhelming majority of cases. Science philosophy and epistemology have addressed many issues of synthesizing individualistic and social perspectives, as well as various social aspects of processes and developments related to scientific knowledge (Oeberst et al., Reference Oeberst, Kimmerle, Cress, Cress, Moskaliuk and Jeong2016). Although there are several studies on social construction of knowledge and enacted social knowledge, little has been done with respect to “social utilization of knowledge” (Stehr, Reference Stehr1996). As noticed by Saltykov et al., the advent of the Internet created new opportunities and technological capabilities for structuring and refinement of raw scientific data and knowledge (Saltykov et al., Reference Saltykov, Rusyaeva and Kravets2015). The scientific discipline and the social/cultural institute conjointly impose a conceptual system on scientific constructions. Luk proposed a theory of scientific study (including a contextual interaction model), which interprets it as a social learning process including activities such as scientific knowledge creation, revision, application, confirmation, and dissemination with the aim of safeguarding quality, completeness, and general relevance of knowledge (Luk, Reference Luk2017).
Gamma-knowledge
Among others, education, economics, environmental science, law, politics, sociology, and statistics represent present-day gamma-disciplines. These specific disciplines of social sciences are in different stages of their development – the arrow of which is pointing from an experimental character to an abstract character. Social sciences are orientated to study the human society and social relations. Social scientific realism assumes that social realities exist independently from thinking, observation, and behavior of observers. Mises discussed that the foundations of the modern social sciences were laid in the eighteenth century, starting with history, and that a radical change took place by picking up phenomena that belonged to political economy, human action/conduct, and social cognition/moral (Mises, Reference Mises1942). He indicated the restrictions in experiencing or formal modeling and argued: “What makes natural science possible is the power to experiment; what makes social science possible is the power to grasp or to comprehend the meaning of human action”. He is among the first ones whose work identified the interpretative nature of social sciences and the role of logical fallacy in justification. Later on, it has been extended by the introduction of the concepts of pragmatism, critical theory, and pluralist thinking (Bohman, Reference Bohman2002). Tang argued that there were 11 foundational paradigms in social sciences (Tang, Reference Tang2011). He identified nine bedrock paradigms: (i) materialism, (ii) ideationalism, (iii) individualism, (iv) collectivism, (v) anti-socialization, (vi) socialization, (vii) biological evolution, (viii) harmony, and (ix) conflict, and two integrative paradigms such as (x) social system paradigm and (xi) the social evolution paradigm. Each of these can only shed light on a limited area of human society. Therefore, in order to understand human society and its history adequately, all of them should be deployed.
Social sciences have always been multi-perspective and multi-cultural in nature. This gave floor to various claims about how it is the best to investigate and to understand the social world. Philosophy of social sciences deals with the generalized meaning of things and attempts to consider notions such as objectivism, normativity, replicability, quantifiability, explanation, demonstration, and prioritization. Social sciences are centered on the sharing of experience about the social world in which perspectives of people differ from one another. Valsiner stated that social sciences are crucial in our understanding of the increasingly globalizing ways of living in the twenty-first century, which is characterized by the conflict of rapid technological advancements and the resistance of the traditional social orders to them (Valsiner, Reference Valsiner and Valsiner2019).
Reber and Bullot discussed the difficulty of drawing a clear demarcation line between science and evidence-based advocacy in the social sciences and humanities (Reber and Bullot, Reference Reber and Bullot2019). They identified several open research issues such as (i) motivated testing, (ii) including and weighting values, (iii) side effects, (iv) intuitive judgments, (v) relativism and reductionism, and (vi) conditional objectivism. For instance, conditional objectivism claims that researchers have to (i) acknowledge value plurality, (ii) consider multiple standpoints at drawing practical conclusions, and (iii) reason based on counterfactual conditional statements. As philosophical positions with remarkable influence on community psychology, Tebes discussed: (i) perspectivism, (ii) pragmatism, (iii) feminism, and (iv) critical theory (Tebes, Reference Tebes, Bond, Serrano-García, Keys and Shinn2017). These positions (i) seek to base their claims on empirical evidence, which are accepted within a given scholarly community, (ii) accept constructivism as the basis for knowledge claims at varying degrees, (iii) recognize that knowledge claims are flawed and dependent on culture, history, and unique contexts, and (iv) seek to use knowledge claims variously as the basis for action.
Gamma-disciplines have things in common with sciences as well with humanities. The goal of social science is to understand and explain social phenomena around us, of which we ourselves are a part. Compared with the beta-disciplines, social sciences seek knowledge differently and offer different knowledge. For example, instrumentalists commit themselves to the view that social sciences, like engineering, should conduct only applied research and should devote itself to the creation of innovative solutions for real-world problems. Carre discussed that epistemology of social sciences is influenced by a recursive normativity. In fact, not only the way of investigating phenomena is influenced normatively, but also the studied phenomena are often normatively organized (Carre, Reference Carre and Valsiner2019). This complicates the epistemology of knowledge of social sciences. Sometimes, social scientists are criticized for taking the freedom to interpret the data so as to make recommendations that match their own opinion (Reed, Reference Reed2008).
Not only curiosity, but also subjective judgments play a role in recognition of influential phenomena of the human world and interpretation of the related problems. In many disciplines of social sciences, the issue is not if knowledge is a properly justified correct belief, but if it is “correct enough” or simply “good enough”. This issue is rooted in the endeavor of social sciences, for example business economics and behavioristic psychology, to solve societal problems as soon as possible by considering their all-unique features. Certain fields of social science were often criticized as unscientific because of their limitations to formulate general laws and universal theories governing human societies. Mayntz argued that natural science concepts and models have had significant influence on theoretical developments in the social sciences, especially on sociology, in terms of theory transfer, formal modeling, and theory building (Mayntz, Reference Mayntz1990).
Driven by the intention to establish a common physics foundation for all fields of social and natural science, Wayne made an attempt to formulate five new physics laws, which are qualitative, related to decision-making, and connects particular research domains of natural and social sciences (Wayne, Reference Wayne2013). Rittel and Webber argued that a significant difference between natural science and social science is that the former has developed to deal with “tame” problems, whereas the latter usually faces “wicked” problems that cannot be definitively described (Rittel and Webber, Reference Rittel and Webber1973).
Delta-knowledge
Some elements and levels of constructivism have appeared in gamma-disciplines and -knowledge. On the other hand, the operationalization and utilization of truly creative knowledge is rooted in intellectual aptitudes such as heuristics, intuition, instinct, serendipity, inception, trial-error, perception, clairvoyance, and karma. These words were not mentioned above and, more importantly, are not used in the context of ABG disciplines in the literature. Nevertheless, the knowledge that has to do with these needs attention since the above-listed nouns are not about what is, but about what might be. The word “might” used to express interrelatedness of the potency of creating and the intention of creating that are closely related to humans and their designerly behaviur (Gedenryd, Reference Gedenryd1998). Archer wrote that design is the area of human knowledge, understanding, skill, and experience that reflects the human concern with the appreciation and adaption to his surroundings in the light of material and spiritual needs and that the design discipline is equated with science and the humanities (Archer, Reference Archer1979). Though design is a broader concept, it is regarded as part of engineering (i.e. engineering and industrial design as the input for production). What makes engineering design different from science is (the necessity of) abandoning the explicit commitment to evidence, though not neglecting physical principles and critical thinking (Eekels and Roozenburg, Reference Eekels and Roozenburg1991). In the process of design, evidence about the properness of the outcome may be not logically possible since the design problem evolves with the design solution, and vice versa (Frayling, Reference Frayling1993). The challenges in the process of formulating the problem and conceiving a solution (or re-solution) are identical policies that respond to social problems and that cannot be meaningfully correct or false (van Aken, Reference van Aken, Helfert and Donnellan2012).
As a follow-up on the design movement, which has its roots in the early 1960s, an influential design lobby came to power in the UK and initiated a political and economic campaign in the years of 1980s. It was concerned with the disciplinary identity of design and the unique manifestation of “design science”. Because of this, as discussed in Archer (Reference Archer1990), design methodology and design research moved decisively from the academic and professional establishments of artistic, architectural, and industrial design to the engineering establishment. Therefore, design science has been defined as a body of valid generic knowledge produced by rigorous research about what, why, and how to design. Cross made a distinction between (i) research into design (producing understanding of the design process) and (ii) research for design (producing instructions on how to design) (Cross, Reference Cross1999). As a knowledge system, design science is a structured body of valid knowledge on all possible manifestations of designing. Willem posited that the goal of design (activity) is not to produce knowledge, but to take action to produce change in human's environment (Willem, Reference Willem1990). In his view, producing knowledge and creating change are distinctly different activities. However, it should be taken into consideration that producing knowledge needs knowledge and creating change needs knowledge. That is, a sharp separation is not sensible. Eventually it means that research should be designed, and design needs enabling research.
Among others, Gilles and Paquet suggested to differentiate the genre of socio-cognitive inventive knowledge, labeled by delta (Gilles and Paquet, Reference Gilles, Paquet, Paquet and von Zur-Muehlen1989). This fourth genre supplements the ABG genres of knowledge and includes the intellect of various creative disciplines such as fine arts, performing arts, industrial design, product design (customer durables), as well as that of sustainability, law, and economy (Giard and Gilles, Reference Giard and Gilles2001). Though it seems to be contradictory at first sight, this latter is easy to explain by the following facts: (i) no artifacts can be against the laws of nature and (ii) social science knowledge supports their implementation as socially proper products. Consequently, while there is a debate about the “scientistic” bias in the social sciences, there are much less questions about the role of specific bodies of natural sciences and social sciences in delta-knowledge. Another essential characteristic of this kind of knowledge is that it is closely related to disciplinary practice and appears as the result of doing, making, acting, and deciding. As posited by Friedman, practice is a significant method of knowledge creation and deepening existing knowledge, and mastering skills and knowledge application by practitioners (Friedman, Reference Friedman2017). Refection also contributes to this knowledge (Schön, Reference Schön1992). Appraising the academic work of Schön, the dilemma of rigor versus relevance was addressed by Waks (Reference Waks2001). He wrote: “The crisis of the professions arises because real-life problems do not present themselves neatly as cases to which scientific generalizations apply”.
Thus, delta-knowledge may exist in two forms. First, it can manifest in a noticeable higher sophistication (or quality) of the subject of making, doing, acting, and deciding, in particular, when the mentioned activities occur in a recurring manner. Second, it can also manifest in the mind-set and skills of the maker, doer, actor, and decision-maker and may enable a successful task completion. Delta-knowledge benefits from the bodies of ABG-knowledge, but it does conflict beta-knowledge neither on a fundamental nor on an applied level. On the way to a fully fledged innovation society, the whole of delta-knowledge is regaining its important place as transdisciplinary innovation assets of change-maker disciplines (Cross, Reference Cross2001). Therefore, the triplet presented in Figure 1 can be extended with this fourth genre without any contradiction, as shown in Figure 2.
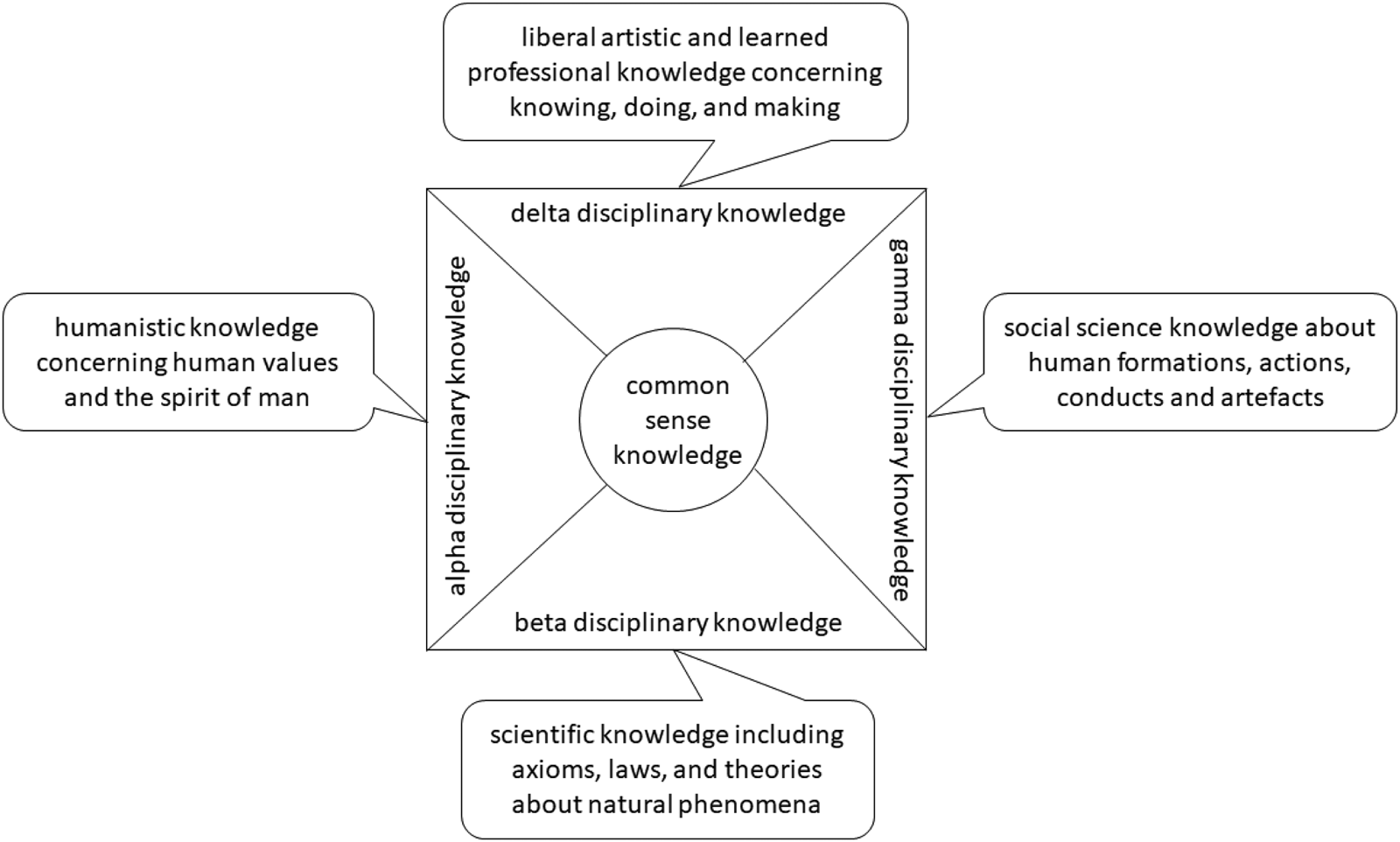
Fig. 2. Delta-knowledge included in the model of human knowledge.
Willem rightly observed that “while science is the striving for knowledge, designing is the expression of an innate human ability that pursues changing the environment presumably for the better. Perhaps because it involves an innate ability rather than knowable objects and phenomena, humans have shown decidedly less curiosity about design than about science.” (Willem, Reference Willem1990). Nevertheless, systematic inquiries toward design knowledge may address any phenomenon related to occurrences of design/designing (Cross, Reference Cross2001). The literature typically considers five categories of these phenomena, which are related to: (i) artifacts (products, systems, services, and experiences) created by design, (ii) people (involved in or influenced by design), (iii) processes (involving all creative, operational, use, and change activities), (iv) environments (in which design-related changes take place), and (v) intellect (cognition associated with intelligent behavior). In each of these categories, the knowledge may ideate, describe, explain, predict, and/or regulate natural or created phenomena. In addition to this, design knowledge can also be articulated (taxonomically decomposed) in terms of the pertaining notions, concepts, things, actors, etc. For instance, as shown in Figure 3, design (conceptualization, detailing, and planning) processes integrate bodies of knowledge about motives, objects, methods, place, time, and reasons.

Fig. 3. Knowledge embodied in design processes.
Considering everything, delta-knowledge differs from common sense knowledge since it has a rich professional content. It also differs from alpha-knowledge because of its dynamically evolving professional nature. With regard to beta-knowledge, the main difference lies in the different roles of and relationships to formal theories, and in the dominance of validation in context over logical justifications of proper beliefs. Delta-knowledge reflects the particular, rather than the general. Typically, inductive (i.e. generalization from particular observations), abductive (i.e. making a probable conclusion from what is known), or retroductive (i.e. making rational instinct driven probable conclusion) reasoning is applied to generalize from the particular toward the general. The difference with regard to gamma-knowledge is in the dominantly creative and predictive nature, and not in a descriptive and explanatory nature, though delta-knowledge shares the pragmatic nature of gamma-knowledge (Van Aken, Reference Van Aken2005). In addition to dedicated inquiries, a basic mechanism of acquiring delta-knowledge is generalization from dealing with practical things, cases, and situations as reflection in action (Schön, Reference Schön, Dowie and Elstein1988). Reflection may happen directly or indirectly. Direct reflection assumes a conversation with the subject and the situation, and an intuitive or systematic evaluation of the findings and experiences. For instance, this kind of intelligence can be (i) derived from cross-cases studies in the field of management, (ii) generated by comparing products with products of competitors in the field of design, and (iii) collected by surveying the notes in the visitors' book at an exhibition in the field of art. The second-generation systems-approach is needed to deal with wicked-problems that often happen in design, policy, and planning (Graham and Dickinson, Reference Graham and Dickinson2007).
As the above concise comparison shows, delta-knowledge reflects different epistemology, methodology, and axiology (Frey, Reference Frey2003). This is broadly analyzed in the related literature. The epistemology of delta-knowledge is practice-fed. The sources are the problems to be addressed in the respective disciplinary domains. A repeated addressing of the problems lends itself to some theories of general or multi-context relevance. The explanations about the solution and the process of solving problems are usually based on imperfect or even incomplete information and knowledge and are strongly influenced by the (evolving) context in which the problem is placed (Smith and Browne, Reference Smith and Browne1993). Therefore, generalization has restrictions. The reasoning about the solution and the way of solving the problems rely on intuitions and are strongly influenced by both practical experiences and retrospective knowledge. This latter body of knowledge is rooted in the productive past. It means that knowing the space of potential solutions and having the know-how needed for (i) navigating on this search space, (ii) synthesizing, analyzing, and crafting compositions, and (iii) conceiving (near)future situations are key elements of design problem-solving.
Looking at it from a structural perspective, delta-knowledge is a composite knowledge. It purposefully blends knowledge of (i) natural sciences, (ii) engineering sciences and technology, (iii) social and behavioral sciences, (iv) creative and applied arts, (v) humanities and liberal arts, and (vi) human professions and services. One part of the practical design knowledge is the result of learning by doing. Another part is formed by the interplaying elements of (i) tacit knowledge (e.g. attitude, vision, preferences, creativity, and leadership), (ii) codified knowledge (e.g. standards, principles, guidelines, protocols, norms, scenarios, and procedures), and (iii) factual knowledge (e.g. signals, data, events, evidences, patterns, and theories). There is a circular relationship between the delta-disciplines and the beta- and gamma-disciplines. Delta-disciplines import basic and applied knowledge of the latter disciplines and discover novel research phenomena and questions for them (Zdrahal et al., Reference Zdrahal, Mulholland, Valasek and Bernardi2007). The societal elements (populations, communities, crowds, and forums) play a significant role in the exploration, sharing, and consolidation of design knowledge. Together with the offered experiential values, the usefulness of the related artefactual and procedural knowledge and the sufficiency of the solutions are the major axiological concerns and measures. The axiological concerns (values and norms) are more specific in the typical delta-disciplines, but are also varied in them (Paterson, Reference Paterson1979). Physical, functional, utility, perceptive, aesthetic, mystic, rational, cognitive, emotional, financial, and educational values are all in the spectrum of values.
Other issues of delta-knowledge are related to understanding its typology and taxonomies. Since the 1960s, both design and management have received research attention from these perspectives (Margolin, Reference Margolin1998). For instance, Uluoğlu approached the type of design knowledge from the perspective of communication and distinguished (i) reflective, (ii) operative, (iii) contemplative, (iv) directive, and (v) associative types of knowledge (Uluoğlu, Reference Uluoğlu2000). As fundamental ones, Narváez considered (i) empirical–analytical, (ii) hermeneutical–historical, and (iii) socio-critical types of knowledge (Narváez, Reference Narváez2000). Cross distinguished three categories of design knowledge: (i) knowledge of people (of outstanding designers), (ii) knowledge in design processes, and (iii) knowledge in design artifacts (Cross, Reference Cross2001). Van Aken made a distinction among (i) object knowledge (knowledge about properties of the artifacts and technologies), (ii) ideation process knowledge (knowledge about the design processes to produce object or realization designs), and (iii) realization knowledge (knowledge about the processes to realize artifacts) (Van Aken, Reference Van Aken2005). Considering system cognition, Radermacher proposed a general knowledge framework that includes four levels: (i) physical, (ii) neuronal, (iii) symbolic, and (iv) model knowledge levels (Radermacher, Reference Radermacher1996).
A theoretical framework for typology of design knowledge was proposed by Müller and Thoring (Reference Müller and Thoring2010), building on the stratified framework of Radermacher and the results of their literature study. This conceptual framework interprets four types of design-specific knowledge: (i) conveyed by physical objects (design artifacts), (ii) tacit instinctive feelings (design intuition), (iii) codified knowledge (design rationale), and (iv) scientific theories (design theories). Wölfel and Prescher observed that a broad debate is going on concerning the functions, structures, and contents of design knowledge, since the term is fuzzy and comprises different purposes and applications (Wölfel and Prescher, Reference Wölfel, Prescher, Scheuermann, Minder and Aebersold2008). They proposed to use prescriptive object knowledge as design knowledge. Haynes interpreted design knowledge of information systems, which includes core algorithms, data structures, parameter data, and system content, as underutilized resource for active learning (Haynes, Reference Haynes2006). Wallace and Press casted light on the role of craft and skill-type design knowledge in the context of wearable digital communication devices (Wallace and Press, Reference Wallace and Press2003). Contrary to the diversity of interpretations and the obvious need for further research, it is apparent that the overwhelming majority of the characteristics and classifications are unique and do not represent ABG-knowledge.
Epsilon-knowledge: what makes it legitimate?
As the current trends indicate it, we can expect disrupting changes in the twenty-first century (Sheng et al., Reference Sheng, Amankwah-Amoah and Wang2019). They will be caused by IESs. We are at the dawn of the fifth industrial revolution that is aiming at the development of artificial narrow and generic intelligence as a society-level productive asset (Pathak et al., Reference Pathak, Pal, Shrivastava and Ora2019). The on-going blending of bits, atoms, neurons, genes, and memes (the so-called B.A.N.G.M. technologies) is a driver of this process (Canton, Reference Canton2004). As the B.A.N.G.M. technologies evolve, a true synthesis of the physical, biological, cognitive, digital, cyber, and social realms becomes possible (Wetter, Reference Wetter, Markus, Kühling and Henn2006). Through this synthesis, also intelligence becomes integrated into engineered systems. As a result, highly intellectualized engineered systems (HIESs) will be available for conventional and unconventional applications (Liu et al., Reference Liu, Tomizuka and Ulsoy2004). Current examples of these are artificial narrow intelligence systems, collaborative agent-based software system, and smart cyber–physical–social systems. What makes the HIESs different from the well-known knowledge-intensive systems is their (not fully pre-programmed) evolving system intelligence (ESI). Dubbed as “systelligence”, ESI is a self-managed operationalization of ampliative reasoning mechanisms (ARMs) on massive SSK (M-SSK) (Horváth, Reference Horváth2021b). Symbolically,

The above scenario forecasts that, as system technologies evolve, the intellect of systems will also evolve through the progression of ARMs and M-SSK. Combined with advanced system resource management, the growing intellect will lead to self-awareness and self-adaptation capabilities (Horváth, Reference Horváth2021a). Systems equipped with these will be able to aggregate, produce, learn, transform, employ, and experience M-SSK over time, in addition to using the existing intellect in system-level problem-solving (Sumari and Ahmad, Reference Sumari and Ahmad2017). The mentioned knowledge operations will be supported by (i) external up-dates, (ii) self-supplementing and/or self-improvement of the possessed of ARMs, or (iii) all of these.
The major claim of the author is that M-SSK (generated and aggregated by multiple systems, such as smart cyber-physical system of systems) is going to grow into a fifth genre of knowledge (Fig. 4). The knowledge acquisition procedures conducted by IESs are contributing to the formation of this new genre of knowledge, which complements the ABGD-genres and, therefore, can be called “epsilon-knowledge”. It includes the total of knowledge associated with the operation of IESs as system-level problem-solving and state-maintaining knowledge. It has its own features, methods, and appearances. Epsilon-knowledge is holistic, synergetic, tailored, and evolving. Holistic means that it involves constituents that enable addressing target problems on a system level. Synergetic means that the constituents are cognitively dependent on and inseparable from each other, while tailored means that the constituents of M-SSK are constructed with the intention to support real-life application problems. Lastly, evolving means that an iterative process, which includes application, evaluation, extension, correction, and refinement, is implemented to enhance the effectiveness and dependability of system-level behavior.

Fig. 4. Placing epsilon-knowledge among the genres of knowledge.
The main constituents of M-SSK are (i) codified human knowledge (pre-programmed in individual systems), (ii) illative/inferential knowledge (self-generated by individual systems), and (iii) aggregated meta-knowledge (generated based on the contributions of the linked individual systems). Codification of human knowledge includes aggregation, filtering, structuring, representation, and validation of the raw knowledge elicited from relevant human stakeholders. Codification establishes an implicit (interpretative) relationship between genuine human beliefs and the M-SSK. As shown in Figure 5, this is external knowledge engineering, which can be substituted or combined with internal knowledge engineering. This is a typical situation in the case of using digital twins related to IESs (Boschert et al., Reference Boschert, Heinrich and Rosen2018). When the internal knowledge engineering is intense, a rapid growth of the knowledge can be anticipated. It means that the acquired/actual M-SSK will be very different from the start-up knowledge after a period.

Fig. 5. Modes of knowledge engineering for IESs.
Eventually, the chunks and bodies of knowledge generated by ARMs are derivatives of codified human knowledge. The generated components of M-SSK can be of two kinds: (i) explained (explainable) and (ii) not explained (unexplainable). For instance, the results of numerical computations produced by the algorithms of a system and the conclusions derived based on them are explainable, while the abstract and hidden patterns constructed by multi-level machine learning algorithms are not or not completely explainable. Taking it into consideration, two forms of M-SSK representation need to be distinguished: (i) explicit representations and (ii) implicit representations (Aktharsha, Reference Aktharsha2011). Concrete knowledge representations capture the functional elements and the logical and semantic arrangement of knowledge by means of language constructs and procedural structures. Abstract knowledge representations are information patterns and models generated by computational learning and reasoning mechanisms (Ziori, Reference Ziori2004). It is worth mentioning that neither gnoseology nor epistemology has to do with this genre of knowledge since their focus is on human knowledge.
Another issue concerns the application-specific reasoning mechanisms (ASRMs) of IESs, such as needed for an automated parking assistant or a social distance warning system. Likewise the initial problem solving knowledge, the initial computational inferring/reasoning mechanisms are constructed based on human knowledge by experts. During their operation, IESs may learn and aggregate additional problem-solving knowledge and may change, adapt, or even replace the initial inferring/reasoning mechanisms that process this knowledge. Self-construction of enhanced ASRMs needs dedicated knowledge, which complements the problem-solving knowledge. The management of these self-adaptation (self-enhancement) processes assumes dedicated system capabilities. It can be expected that, in addition to intra-system mechanisms, also inter-system knowledge aggregation and processing mechanisms will be available. The self-managed cognitive mechanisms of IESs should include those context modeling, awareness building, situation analysis, decision-making, and communication management mechanisms that are naturally given in the case of human cognition. These system-level ASRMs of complex system-of-systems (SoSs) will extract, structure, consolidate, and store meta-knowledge in on-line warehouses. Their operation toward this end may resemble what is performed by collaborative multi-agent systems. These are research themes and phenomena for the currently booting up system science and engineering research.
Some simple examples of systems with genuine evolving systelligence are as follows: Case A: A smart parking assistance system, ASRMs can learn the features of the successfully chosen and applied motion paths, or can rank their appropriateness, and, based on this knowledge, the ASRMs can operate more efficiently in future cases. Case B: A smart in-house fire evacuation system, the ASRMs can learn the rate of obedience of the people to the obtained information/instructions and can use this information at constructing the messages sent to them real time during the evacuation process. Case C: A smart vertical greenhouse system, the ASRMs can learn the patterns of the variable sunlight, lighting, and humidity conditions and adjust the irrigation accordingly. It may advise if the placement of the greenhouse is proper or not.
Computational ways of generating epsilon-knowledge
The goal of this section is to provide an overview on (i) the variety of sources, (ii) the ways of obtaining, and (iii) the approaches of consolidation of the self-generated part of M-SSK. As mentioned above, the ways of creating M-SSK are (i) pre-programming of filtered and structured human knowledge by a team of knowledge engineers, (ii) acquiring, inferring, learning, and managing knowledge by cognitive mechanisms of a smart system, and (iii) generating and aggregating meta-knowledge over the contributions of the linked individual systems. The approaches of pre-processing and coding human knowledge are commonly known from the knowledge engineering practice of knowledge-intensive systems (Aikins, Reference Aikins1983). Though the pre-programmed part of M-SSK plays an important role in the initial intellectualization of engineered systems, its proportion with regard to the self-generated part will be decreasing over time. Therefore, it will be not discussed further in the rest of this section.
Concerning the sources of the self-acquired part of M-SSK, anything can actually be a source presumed it is accessible and can be processed by ASRMs. Figure 6 shows the most important general content sources (GCSs). The ampliative mechanisms of the system transform the contents (signals, data, and relations) obtained from these GCSs into problem/task specific or generic knowledge. The results of this transformation are the so-called derived knowledge chunks (DKCs), which may appear in explicit and implicit forms. The tested DKCs are placed in knowledge repositories, whose stored elements can be used immediately as input knowledge chunks. This direct coupling makes the content transformation process a loop and recurring. Figure 6 shows the original content sources in a random order. The reason is that they typically do not have logical priority and their future role may be different from the current one. Each of the mentioned sources necessitates the deployment of computer-readable representations (coding). Widely recognized challenges concerning fully automated knowledge elicitation and processing are (i) diversity and variability of the representations of the contents obtained from the mentioned sources, (ii) extracting the semantic meaning in a context-sensitive manner, (iii) efficient transformation of the input contents into useful chunks of knowledge, (iv) synthesizing the chunks into coherent bodies of knowledge, and (v) assigning meta-knowledge to the various bodies of knowledge. The reason behind these challenges is that each of them needs specific computational mechanisms, which may or may be not linked procedurally and semantically. The issue of interoperable ASRMs is addressed only scarcely and restrictedly in the current literature (Bezem and Mahboubi, Reference Bezem and Mahboubi2019).
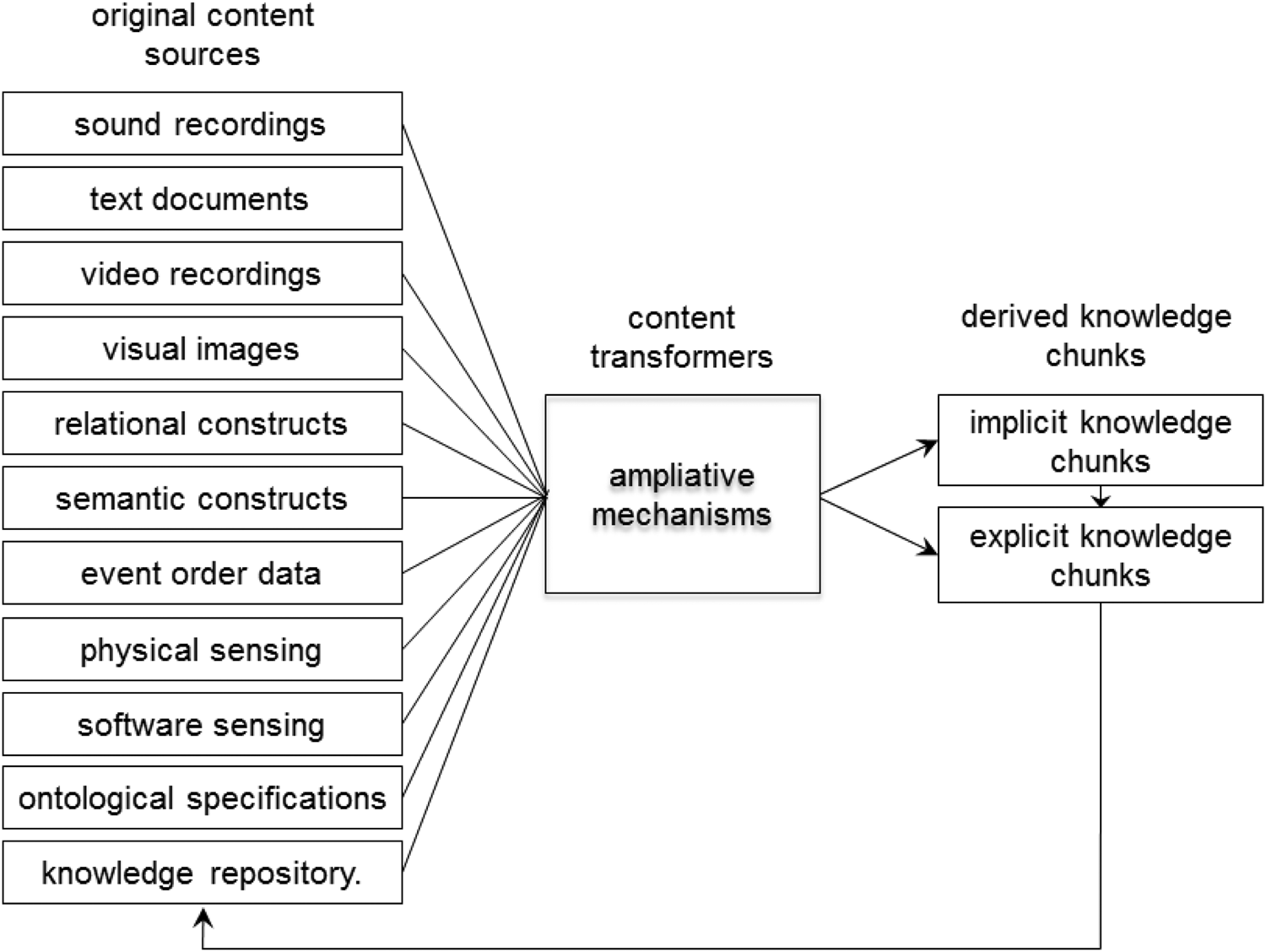
Fig. 6. The reasoning model on getting to chunks of SSK.
The GCSs offer a wide range of input for knowledge transformation. For example, analog and digital sound recordings include noise, speech, and music signals and data. Analog and digital text documents may include traditional text carriers and local digital or network hypertext files. Having both visual and auditory contents, video recordings may be real-time streams or stored recordings. Visual images include drawings, images, photos, and displayed contents. Relational constructs are entries of digital databases and digital models, while semantic constructs are arrangements such as scripts, frames, decision tables, agent intellect, and rule structures. Event order data represent logical and temporal (historical) relationships among events. As source of contents, physical sensing provides descriptive characteristics (signals and data) of physical phenomenon, while software sensing provides data associated with computational phenomena and actions. Ontological specifications carry descriptive and associative characteristics in structured (or standardized) language formats. Knowledge repositories are knowledge bases, warehouses, or silos that contain chunks and bodies of knowledge in some interchangeable format (Kohlhase et al., Reference Kohlhase, Rabe and Wenzel2020).
The previously mentioned challenges can be explained partially by the various epochs of developing artificial intelligence methods and tools for problem-solving (Li et al., Reference Li, Wang, Ji and Yang2020). Historically, five major families of ampliative computational mechanisms were proposed. First, symbolist approaches such as (i) imperative programming language-based (procedure-based) reasoning, (ii) declarative logical language-based reasoning, (iii) propositional logic-based inferring, (iv) production rule-based inferring, and (v) decision table/tree-based inferring. Symbolic structures are deemed more human friendly than sub-symbolic systems since they allow human readability. The literature discusses several modes of logical reasoning such as (i) deductive, (ii) inductive, (iii) abductive, and (iv) retrospective modes. Second, analogist approaches, such as (i) process-based reasoning, (ii) qualitative physics-based reasoning, (iii) case-based reasoning, (iv) analogical (natural analogy-based) reasoning, (v) temporal (time-based) reasoning, (vi) pattern-based reasoning, and (vii) similarity-based reasoning. Third, probabilistic approaches, such as (i) Bayesians reasoning, (ii) fuzzy reasoning, (iii) non-monotonic logic, and (iv) heuristic reasoning. Fourth, evolutionist approaches, such as (i) genetic algorithms, (ii) bio-mimicry techniques, and (iii) self-adaptation-based techniques. Fifth, connectionist approaches, such as (i) semantic network-based, (ii) swallow-learning neural networks, (iii) smart multi-agent networks, (iv) deep-learning neural networks, (v) convolutional neural networks, and (iv) extreme neural networks. These sub-symbolic structures are incomparably richer than standard propositional symbolic languages, where human readability is not a gold standard of information processing anymore. As discussed by Paschen et al., the current lack of standards leads to incompatible application programming interfaces and incompatible methods of communication between various software components and results in interoperability and usability gaps in AI applications (Paschen et al., Reference Paschen, Pitt and Kietzmann2020).
The computational mechanisms of an IES are the sort of cognitive equipment by which the problem-solving potential of M-SSK can be operationalized. The problem to be solved, the available knowledge, and the processing mechanisms are in transitive relations. In other words, the nature of problem determines what knowledge is appropriate and the knowledge determines what computational mechanisms are applicable for knowledge processing. A confounding variable is the representation of the knowledge in this context. In principle, both non-ampliative and ampliative mechanisms can be used for knowledge processing depending on the problem at hand. Non-ampliative mechanisms are such as (i) classification (placing into groups/classes), searching/looking up (selecting from a bulk), and contextualization (appending application context).
There is a clearly observable progression concerning the implementation of truly ampliative mechanisms. Typical tasks are (i) fusion, (ii) inferring, (iii) reasoning, (iv) abstraction, (v) learning, (vi) decision-making, and (vii) adaptation (of knowledge). Inferring is the operation of deriving information in context, whereas reasoning is about synthesis of knowledge. Inferences are not made always explicit in the process, but serve as invisible connectors between the claims in the argument. Reasoning is a complex and intricate cognitive phenomenon, which is more than just formal application logics – it extends to semantics, pragmatics, and even apobetics of human intellect. With regard to consolidation of M-SSK, there are some issues to consider. For instance, consolidation concerns not only the run-time generated part, but also the human pre-programmed part and the off-line constructed meta-knowledge (Yang et al., Reference Yang, Yang and Chen2020).
Action follows belief: issues for sympérasmology
A strong impression of the author states that the overall nature, identity, specificity, exploitation, possible impact, and future role of epsilon-knowledge are almost ignored, or at least not sufficiently addressed in the current literature. On the other hand, he believes that this is an important issue since the amount of epsilon-knowledge is rapidly growing and it needs to be used as a productive asset already in the near future. As discussed above, a characteristic manifestation of epsilon-knowledge is M-SSK that needs deep and comprehensive studies, and a philosophically underpinned theoretical framework. Figure 7 explains the current situation in term of scientific study of the categories of knowledge. Traditionally, gnoseology deals with common (non-general) human knowledge possessed by individuals. Epistemology investigates general human knowledge, typically that regarded as tested scientific knowledge. It also addresses composite engineering knowledge, most often in combination with human knowledge. Epistemology has been individualistic, that is, it has typically focused on how individual thinking or working alone can acquire knowledge. Neither gnoseology nor epistemology is interested in artificial knowledge that manifests in massive SSK. Simply for historical and teleological reasons, they are not ready to consider this emerging genre of knowledge.
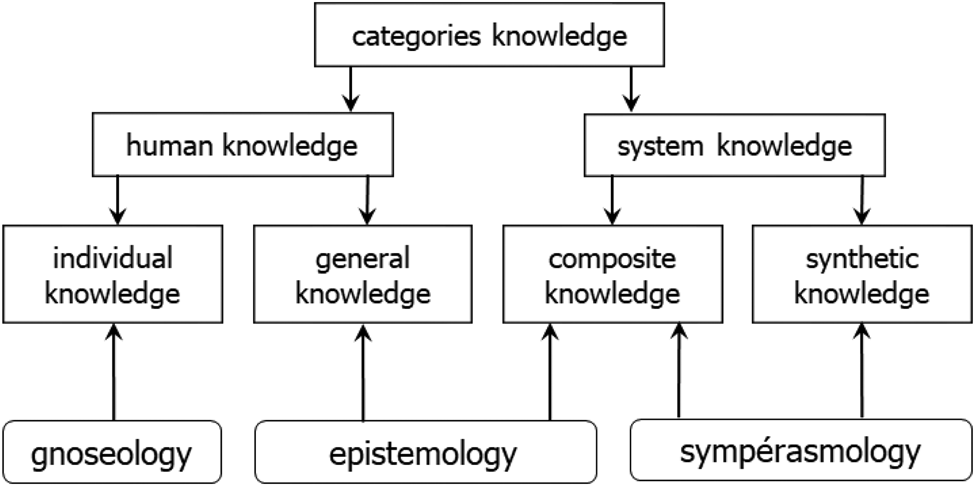
Fig. 7. Scientific study of the categories of knowledge.
Action follows belief: in order to initiate a dedicated theory of SSK, I proposed “sympérasmology” as the conceptual framework and a comprehensive field of research in epsilon-knowledge (Horváth, Reference Horváth2020, Reference Horváth2021b). This name has been derived by putting together the Greek term “sympérasma” that refers to inferred/reasoned knowledge, and the Greek term “logos” that is used to express the logic and reasoning in crafting a defendable piece of knowledge (and building demonstrative logical cases). Sympérasmological investigations can help separate the competing concepts of intellect (i.e. system intelligence by mimicking human intelligence and purposefully synthesized system intelligence). I took the liberty to propose the term “systelligence” to refer to various grades of self-managed intelligence in IESs.
A broad spectrum of investigation domains was proposed for sympérasmological studies, which are arranged in Figure 8. Digital management of M-SSK starts with modeling and structuring, and continues with mapping the structures to various conceptual constructs and representations. Sympérasmology is closely related to computing but has a different viewpoint. Sympérasmology is interested in theoretical and philosophical, descriptions, explanations, and predictions, although praxiological and pragmatic issues are also important for it. As an example, the main issue for sympérasmological representation (syntax) studies is not what representations exist for different purposes and how to represent a particular body of knowledge digitally, but how the various representations relate to the very nature and essence of M-SSK. From a methodological viewpoint, sympérasmological investigations apply both “a posterior” (experimental) and “a priori” (interpretative) methods similar to those of epistemology and gnoseology. Normative questions are also posed concerning what and how people should view and approach M-SSK. A typical experimental method is individual case or case ensemble implied reasoning.

Fig. 8. Domains of sympérasmological investigations.
An important study orientation of sympérasmology is the general characterization of M-SSK. As a first and rough iteration, the following key attributes were proposed as elements of the attribute profile: (i) synthetic, (ii) dependable, (iii) ampliative, (iv) codifiable, (v) compositional, (vi) inferable, and (vii) explainable (Fig. 9). From a philosophical perspective, key attributes can be seen as dispositions, which lead to abilities under certain conditions. Synthetic means that a dominant part or the whole of M-SSK is artificially created for a purpose. Dependability means that a system is trustworthy since it is collectively characterized by attributes such as (i) reliability operation, (ii) continuous availability, (iii) safety, (iv) confidentiality, (v) robust survivability, (vi) overall integrity, and (vii) preventive maintainability. Ampliative describes the potential (power) of deriving additional knowledge that is explicitly not included in a given body of M-SSK. Codifiability represents the extent to which a given knowledge item can be reduced to representable and communicable conceptual units by means of drawings, formulae, numbers, or words. Compositionality means that the whole of M-SSK is derived from the ultimate goal of system operation by complementing knowledge generation and fusion actions of the given system. Inferable means that the M-SSK complies with the principles of epistemic knowledge (logical rationality) and allows multiple forms of inferring without the need for knowing explicitly by the system what it knows implicitly. Explainability is a feature that makes possible to discover, analyze, and clarify implicit and nonfigurative relationships between unconnected, causal, and/or abstracted constituents of M-SSK. From the perspective of knowledge management, distinguishing attributes of importance are such as (i) volatility, (ii) location, (iii) abstraction, (iv) conceptuality, (v) resolution, (vi) transferability, (vii) measurability, and (recursion). From a computational dimension, the most significant ones are such as (i) transferability, (ii) traceability, (iii) duplicability, (iv) augmentability, (v) evolvability, (vi) tacitness, (vii) explicitness, (viii) experiencable, (ix) accountability, and (x) contextualization.

Fig. 9. Key attributes of M-SSK.
Sympérasmology cannot be implemented as simple augmentation of gnoseology or epistemology, since it is based on different fundamental concepts and principles originating in the very nature and processing mechanisms of SSK. The importance of sympérasmological studies of epsilon-knowledge comes from the vision of knowledge growing and harvesting systems (Sumari et al., Reference Sumari, Ahmad, Wuryandari, Sembiring and Sahlan2010). Sympérasmology is supposed to provide a constructive and pluralistic theory of epsilon-knowledge. It must investigate its entirety, as well as the bodies of knowledge acquired by various system-level processing mechanisms. Sympérasmological investigations may concern (i) rudiments, (ii) principles, (iii) faculties, and (implications). In this sense, they go beyond the methodological approaches (informed speculation and rational examinations) of gnoseology and epistemology. It may rely on methods such as (i) critical literature studies, (ii) experimental investigations, (iii) creative system thinking, (iv) cross-case practical studies, and (v) contemplative validations.
The benefits of conducting studies according to the principles of sympérasmology are not only in a clearer academic view, but also in the opportunity of more dependable innovation strategies and better engineering decisions concerning the proper intellectualization of systems for industrial and social applications. It may even have a disruptive influence on the design, engineering, application, and utilization processes of smart (and intelligent) systems. It is foreseen that the sympérasmological theory of M-SSK will condense and evolve in accordance with the progression of the B.A.N.G. technology, system science, and system engineering. The sympérasmological insights will be instrumental for forwarding epsilon-knowledge toward a scientific, technological, and commercial asset position (Weikum et al., Reference Weikum, Dong, Razniewski and Suchanek2020).
Ad perpetuam memoriam: reflections and conclusions
This position paper recognized the issue of growing SSK and argued that we must regard it as an emerging complement of Machlup's types of disciplinary knowledge. Called epsilon-knowledge, this knowledge enables the cognitive operation of IESs, such as smart cyber-physical systems. Initially, this knowledge is “triggered” by some pre-processed (filtered, structured, represented, and coded) chunks of structured and human knowledge that is usually largely extended and enriched by the results of the cognitive operation of the mentioned systems (Epstein et al., Reference Epstein, Payne, Shen, Dubey, Felbo, Groh and Rahwan2018). This is not the knowledge that is used by the developers at designing and architecting the computational components and ASRMs of these systems. This latter is beta-/delta-knowledge, rather than epsilon-knowledge. A simple practical example for this delta-knowledge is the data constructs describing the feature sets used for training a deep-learning network. The epsilon-knowledge is a growing system-acquired and self-learnt knowledge constituent, not designed by humans – though it may be influenced implicitly by human knowledge, decisions, and designs. The theories of epsilon-knowledge and the methodologies of its exploitation beyond solving concrete tasks and beyond the boundaries of systems are in a premature stage (Kastenhofer, Reference Kastenhofer2010). On the other hand, based on a proper understanding and with a dedicated management and exploitation strategy, it may become an additional productive asset (Woods and Roth, Reference Woods and Roth1988).
Struggling with the challenge of finding the most expressive phrases, Table 1 offers a comparison of the different genres of knowledge and identifies the distinguishing features of massive SSK. Based on this and the preceding discussion, the main conclusions are as follows:
• Epsilon-knowledge has different features than the bodies of knowledge that are related to human discoveries in the alpha-, beta-, and gamma-disciplines as well as to those related to the human inventions by the delta-disciplines.
• Using their ampliative reasoning and learning mechanisms, IESs can process digitally coded human knowledge and to acquire, synthesize, learn, extract, aggregate, and restructure knowledge on their own.
• Massive SSK represents the practical manifestation of epsilon-knowledge. Since it is not sufficiently explored and explained from ontological and methodological point of view, broadly based sympérasmological investigations are proposed.
• In the near future, IESs should be not seen only as AI-enabled problem-solving systems, but as knowledge growing and harvesting systems. This will increase their functional complexity, but it will most probably offer new affordances and business advantages.
• New technologies (inferring/reasoning techniques) are emerging, such as transfer learning that helps manifestation and exploitation of epsilon-knowledge. Transfer learning aims at improving the collective learning/knowing efficiency and problem-solving potential/power of interoperating IESs by synthesizing and sharing the previously acquired knowledge by any one of the included systems. Such recursively synthesized knowledge is getting far from being human designed knowledge.
• The eventual objective of sympérasmology is to become the staircase to the “mind” (the inferred intellect) of IESs. Toward this end, it must address theoretical and conceptual issues of dealing with epsilon-knowledge in the context of systelligence, rather than methodological, technological, and engineering ones. It must consider logical, computational, semantic, pragmatic, apobetic, human, and social discourses.
• Managing epsilon-knowledge implies the need for semantic and pragmatic knowledge fusion frameworks and system-independent methods and meta-methods, well beyond the issues of unification and oconversion of knowledge models and representations.
Table 1. Correlating the main features of epsilon-knowledge to those of the other genres

Acknowledgements
The author feels himself obliged to express his appreciation of and gratefulness to the three anonymous peer reviewers for their insightful, constructive, and useful comments, questions, and proposals. These largely contributed to the improvement of the initially submitted manuscript. The undiscussed professional issues, the proposed research questions, and the identified extension opportunities offer many important topics, as well as stimulation, for at least two more papers.
Dr. Imre Horváth is an emeritus professor of the Delft University of Technology, where he focused on cognitive engineering of smart cyber-physical systems. He promoted more than 25 Ph.D. students and has more than 440 publications. He is a fellow of ASME and a member of the Royal Dutch Institute of Engineers. He received two honorary doctor titles, the ASME lifetime achievement award, and the Pahl-Beitz ICONNN award. He has served several international journals as editor and is involved on advisory boards. He is the initiator of the International Tools and Methods of Competitive Engineering (TMCE) Symposia. His current research interests are in systematic design research methodologies, intellectualization of cyber-physical systems, and the science of synthetic knowledge of intellectualized systems.