Social media has changed the way that citizens communicate, both amongst themselves and with elites. In some contexts, most famously the Arab Spring in 2011, social media is reputed to have empowered citizens relative to established government regimes (Howard and Hussain Reference Hornik and Grün2011; Tufekci and Wilson Reference Tufekci2012). Despite the initial enthusiasm caused by these revolutions, the effect of the diffusion of social media on this balance of power is far from obvious. Technologically advanced and capable regimes in Russia and China have begun to actively and strategically engage in social media (Sanovich et al. Reference Roberts, M. Stewart and Tingley2018), rather than merely trying to ban its use, and it may be that by 2016, popular social media usage can actually enhances regime resilience.
The long-term use of social media by both pro- and anti-regime actors has received a good deal of scholarly attention, and its use by dissidents during acute protests has been even more studied. The fourth piece of the equation, the regime’s strategic social media response to protests, however, has yet to be fully explored. In part, this is due to a lack of appropriate cases. There have been few large-scale protests against tech-savvy regimes during what Deibert et al. (Reference Corrales and Penfold-Becerra2010) calls the “third generation” of social media use by regimes: active engagement. This engagement often takes the form of anonymous pro-government postings, but elite social media use offers an alternative avenue to flood and disrupt online forums. The largest and most protracted protests in the post-Arab Spring time period were in Turkey in 2013 and Ukraine in 2013 and 2014. Neither of these regimes had the capacity to use “third generation” strategies.Footnote 1
This article fills this gap by analyzing the Venezuelan La Salida protests of 2014. This case is particularly appropriate to exploring elite social media response to protests. Social media (in this case, Twitter) is widely used by both citizens and elites: Venezuela is among the top five countries in terms of Twitter penetration (Schoonderwoerd Reference Schoonderwoerd2013), and 2014 data suggests that 21 percent of internet users log on at least once a month (Friedman Reference Enikolopov, Makarin and Petrova2014). The “soft censorship” of mainstream media sources makes Twitter’s speech-enabling function especially important, and, as is common in Latin America, Venezuelan politicians are more likely to speak their minds directly on Twitter rather than have a media team craft a sanitized presence. The La Salida (“the exit”) protests of 2014 represented a serious effort to oust the regime of President Nicolás Maduro, and the protesters en guarimba (in a blockade) paralyzed parts of the capital of Caracas from February 12 to May 8.
We test a simple theoretical argument, inspired by descriptive accounts from qualitative research and by comparative statics from a related formal model, to analyze the strategic response of both pro- and anti-regime elites. Regime elites constitute the primary sample of interest, but any changes in their behavior can only be understood in contrast to that of opposition elites. We claim that the regime will try to distract attention away from the protesters’ narrative, ignoring the specific grievances of the dissidents and instead bringing up other issues or engaging in general pro-regime cheerleading so that the protesters’ narrative appears to be only one of several possible readings of current events. This strategy is similar to the one that King, Pan and Roberts (Reference King, Pan and Roberts2017) find employed by the Chinese regime in response to online criticism.
This strategy will have several observable implications. First, we expect that the opposition will focus on the protests and try to draw more attention to the narrative La Salida is promoting. Practically, this will entail discussing a smaller number of different topics each day and using Twitter’s “hashtag” function to mention people or events related to the protests in an effort to draw them into the conversation.Footnote 2 Conversely, the pro-Maduro coalition will bring up new issues and emphasize traditionally successful regime slogans, to give people the impression that La Salida’s narrative is only one of many ongoing political discussions. The regime will use Twitter’s “hashtag” function to insert explicitly crafted talking points into the public discussion, aiming to create conversations among their supporters.
We investigate the Twitter accounts of Venezuelan diputados, members of the unicameral Venezuelan National Assembly. The National Assembly is a powerful force in Venezuelan politics, as evidenced by the success of the opposition after winning a large majority in the 2015 parliamentary election. At the time of the 2014 protests, 121 of the 165 diputados had active Twitter accounts, and both regime and opposition diputados are well represented. These elites tweeted over 100,000 times during the five months under study, giving us ample analytical leverage.
We find evidence of the strategic use of Twitter by the two coalitions reflecting their proximate and ultimate goals, in accordance with the observable implications of our argument. By fitting a topic model on the text of the tweets, we find that opposition diputados focus their attention on the protesters and their demands, while regime diputados discuss a large number of different topics. Through analysis of the patterns of hashtag use, we find that the opposition mentions specific people and places, whereas the regime promotes new top-down narratives.
THE VENEZUELAN CONTEXT
Venezuela has been a democracy since 1958.Footnote 3 The first 40 years of Venezuelan democracy were dominated by the punto fijo system, with political power alternating between two clientelistic parties; 1998 saw the election of Hugo Chávez and a shift in Venezuelan economic and social policy.
Chávez won re-election in 2006 and 2012 with very high voter turnout, even as inflation and crime rates rose to high levels, as Chávez successfully but expensively expanded social services and consolidated support among poor Venezuelans (Corrales and Penfold-Becerra Reference Corrales2011). Chávez’s tenure also saw the transition of Venezuela from a representative democracy to what Mainwaring (Reference Leshchenko2012) calls “participatory competitive authoritarianism,” a system which could be subsumed in the general category of a “hybrid” regime. Chávez’s health declined rapidly in 2012, and he died in early 2013.
Vice president Nicolás Maduro won the 2013 special election against Henrique Capriles, a popular state governor and Chávez’s opponent in 2012, but his margin of victory was only 1.5 percent, and there were claims of fraud and illegitimacy made by the losing party.
Under Maduro, continuing inflation and high violent crime rates led to rising discontent (Corrales Reference Tufekci and Wilson2013). The spark that ignited the 2014 protests was the January 6 robbery–murder of a former Miss Venezuela. On January 23, María Corina Machado and Leopoldo López called for La Salida, a movement to protest in the street with the aim of removing the Maduro from power. The plan was for the protest to begin around the country on February 12 (National Youth Day in Venezuela) (Diaz Reference Deibert, Palfrey, Rohozinski, Zittrain and Haraszti2014). On February 5, the attempted rape of a university student on a campus in Táchira in the southeastern part of the country led to a small student protest that provoked a violent governmental response (Perez Reference Pan2014). This was publicized by La Salida, which hoped to use student anger to advance their movement. Importantly, the moderate opposition elites, including all of the diputados in this study, were not involved in the planning or execution of these early protests; thus, the large-scale protests we study were similarly exogenous to both the pro- and anti-regime diputados.
As planned, protests began in Caracas and 38 other cities on February 12, and escalated quickly. López became sufficiently outspoken and high-profile that the regime jailed him on February 18. His arrest was precipitated by him tweeting directly at Maduro: “.@Nicolasmaduro Don’t you have the guts to arrest me? Or are you waiting for orders from Havana? I tell you this: The truth is on our side” (authors’ translation).Footnote 4 Twitter allowed López to spread his message directly and quickly to a wide mass of people—note the almost 45,000 retweets—with no risk that it would be distorted or misrepresented.
The use of Twitter and other social media by the opposition was especially important because of the media environment. Although Venezuela’s constitution guarantees free speech, news outlets faced implicit threats, and engaged in significant self-censorship; Freedom House (Reference Hong and Davison2014)deemed Venezuelan media in 2014 “not free”. The regime lacked the technical ability to curtail speech online, however, because the most commonly used social messaging platforms (Twitter and Facebook) were controlled by US multinational corporations (Pan Reference Oates2017). Chávez used his Twitter account as an extension of his hours-long radio addresses, but this was not a major component of the regime’s overall media strategy.
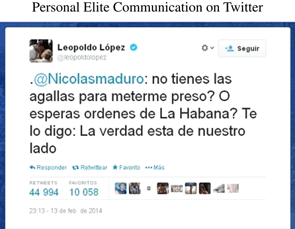
During the height of the protests, opposition groups advocating La Salida in Caracas set up permanent blockades (guarimbas) designed to paralyze the city and heighten the economic crisis. In response, the regime allowed armed paramilitary groups called colectivos to attack, rob, and kill opposition protesters. The violence led to thousands of injuries and at least 40 deaths.
The first major sit-down between the regime and the moderate opposition took place on April 10, though no conclusion or agreement was announced. April 19–20 were a major revolutionary holiday (Beginning of the Independence Movement Day) and Easter, respectively, and this weekend represented the high water mark of the protests. The guarimbas were permanently dismantled on May 8.
The protests ended with a compelling display of strength by the government, but they revealed deep divisions within the country and among the opposition factions, neither of which was truly unified. Capriles and the moderate opposition that includes all of the opposition diputados continued to push for democratic reforms and non-violent methods of progress, whereas La Salida wanted nothing less than the removal of the ruling party, through constitutional means if possible but through violent street protests if necessary (Ciccariello-Maher Reference Ciccariello-Maher2014). The moderates tried to draw as much attention to the protests as possible, but whether this was out of opposition solidarity or in order to improve their bargaining power or electoral chances in the future remains an open question. In either case, La Salida was never the first choice of the opposition diputados under study.
SOCIAL MEDIA IN NON-DEMOCRATIC CONTEXTS
Social media has had a pronounced impact in non-democratic regimes like Venezuela in 2014. Much of the initial enthusiasm about social media surrounded the way it empowered dissidents. It offered citizens affordances to become informed and engage in protest (Tufekci and Wilson Reference Tufekci2012; Christensen and Garfias Reference Christensen and Garfias2015) and for those protests to be better organized (Earl et al. Reference Diaz2013; Agarwal et al. Reference Agarwal, Bennett, Johnson and Walker2014; Bennett, Segerberg and Walker Reference Bennett, Segerberg and Walker2014).
However, dissidents are not the only actors who can use social media, and an acute political crisis is not the only context in which it can be used. Social media shapes the way that citizens communicate on a day-to-day basis, and tech-savvy regimes take an active role in trying to structure and engage with discussions online.
There is a healthy literature on this dynamic. Deibert et al. (Reference Corrales and Penfold-Becerra2010) develop a three-generation framework for how regimes reduce the threat of social media. The first generation is to restrict access to the internet (Howard, Agarwal and Hussain Reference Howard and Hussain2011), and the second is to censor certain kinds of information posted online (King, Pan and Roberts Reference Howard, Agarwal and Hussain2013). These two types of censorship vary significantly in both the technical sophistication required to execute them and their observed effectiveness. A crude, blanket internet shutdown is within the power of many authoritarian regimes. However, employing such a strategy during a protest can backfire: Hassanpour (Reference Griffiths and Steyvers2014) finds that Mubarak’s mass internet shutdown actually increased revolutionary mobilization during the 2011 Egyptian uprising. Targeted censorship of specific types of posts—those with the possibility of encouraging collective action—may be more effective, but they are beyond the reach of most national governments. As Pan (Reference Oates2017) argues, China is only able to execute this strategy because the Chinese internet market is dominated by domestic firms; Venezuela and other nations who primarily use US multinational firms are simply unable to dictate rules about content. First and second generation strategies for limiting the risk of the internet and social media are thus either ineffective or inaccessible to the Venezuelan regime.
This leaves the “third generation” strategies, which include information campaigns and actively paying supporters to post online. These strategies show how social media can be used as a tool for regime activism (Greitens 2013). For example, Oates (Reference Malesky and Schuler2013) details how post-Soviet regimes have taken advantage of weak civil societies to control the online discourse, providing a new, compelling narrative for people who felt directionless after the revelation of the emptiness of the Soviet project. Sanovich et al. (Reference Roberts, M. Stewart and Tingley2018) demonstrate the dramatic changes in online engagement strategy in Russia since 2000. Recent evidence suggests that Russian social media activity has offsetting effects: areas with higher social media penetration were both more supportive of the government and more likely to experience a protest (Enikolopov, Makarin and Petrova Reference Edmond2015).
A comprehensive discussion of social media’s potential to increase regime resilience using these “third generation” strategies is Gunitsky (Reference Greitens2015). Social media can help stave off revolution by giving the regime information about specific grievances that they can address without relying on local bureaucrats with an incentive to falsify information (Chen, Pan and Xu Reference Chen, Pan and Xu2015). Social media may be especially useful for predicting collective action events and aggregating allegations of corruption (Qin, Strömberg and Wu Reference Phan, Nguyen and Horiguchi2017).
However, there is little research specifically testing the “third generation” strategies employed by non-democratic regimes on social media during times of crisis. Before social media, regimes that controlled the mass media could either suppress all mention of a protest, or craft their own preferred narrative about it. Explicit propaganda produced by the state was an effective tool for enabling violent repression and preventing dissent.
Consider the case of the Tiananmen Square protest in 1989. Early in the occupation of the Square by student protesters, the Chinese regime used the media to promote the narrative that these students were agents of the United States, aiming to undermine China. The students were unable to broadcast their true goal and grievances, and the regime’s narrative was unchallenged throughout much of China. Once the regime found it necessary to begin shooting, they switched their media strategy, banning all mention of the protest and its repression.
Neither of these strategies is viable in a society—even one with control over mass media—in which social media use is widespread. Once a large protest has occupied space in a city, it will be able to broadcast its own narrative on social media, and the regime cannot physically remove or kill them without the whole country becoming aware.Footnote 5
Instead, a powerful strategy for the regime is to compete with the protesters on social media. They cannot fully silence the protesters, but they can hope to drown them out and show citizens that the there are other political conversations going on, either by bringing up other issues or engaging in general pro-regime cheerleading (King, Pan and Roberts Reference King, Pan and Roberts2017). La Salida’s narrative focused on high crime and inflation rates. These are trends that individuals can notice, and efforts by the regime to deny them outright would ring false. However, by bringing up many different issues or posting classic regime slogans rather than engaging with the protesters on their own terms, the regime can create ongoing discussions about their efforts to help the poor, improve healthcare, and stand up to the United States. Citizens using social media will thus see a political climate with multiple issues on the agenda and groups of competing regime boosters and detractors, rather than perceiving a one-issue debate with two sides arguing with each other about the fundamental legitimacy of the regime.
This theory has much in common with the strategic predictions made in section 6 of Edmond (Reference Earl, Hurwitz, Mesinas, Tolan and Arlotti2013). In this model, the predicted amount of noise that a regime will insert into their communications will vary with the strength of the regime. Strong regimes (and most regimes are strong in that they are not vulnerable to being overthrown) will decrease noise, to accurately signal their strength. On the other hand, weak regimes (like Venezuela, facing an existential threat) will want to make their communications “noisier” (less focused), in order to make it harder for imperfectly coordinated protesters with incomplete information to infer that the regime is in fact weak.Footnote 6 We do not claim to formally test the model in the current article, but the confluence of predictions from the descriptive and formal literatures is encouraging.
The strategy for the moderate opposition, however, is straightforward: they largely agree with the protesters, but would prefer to see the removal of Maduro and his party through electoral success. They will thus support the protests and try to coordinate attention on them on Twitter, to increase their bargaining power and chances at victory in the next election. The opposition diputados are of interest primarily so that the change in their Twitter behavior can be contrasted with changes in the media strategy of the regime diputados.
HYPOTHESES
We test the theory of active, strategic use of social media during an acute crisis developed above in the case of the 2014 Venezuelan protests.
We expect the pro-regime elites to attempt to distract the public’s attention from the protest’s narrative concerning the regime’s mishandling of the economy and failure to reign in violent crime. They will tweet about a variety of different subjects and engage in general pro-regime cheerleading, to make the opposition’s narrative less central to the national discourse.
In contrast, we expect the opposition elites to focus on the protests and their specific message, at least as long as they believe it to be in their interest. We expect to see a contrast in the strategic reactions of the two coalitions of diputados to the onset of the protests as they pursue their parties’ respective goals. Under normal conditions, politicians are expected to balance their personal interest against that of their party, but because these protests represented an existential threat to the government, the strategic incentives of each politician were closely aligned with that of their party.
The primary way we measure these strategies is by measuring what we call focus. Intuitively, this is a way of trying to get at how many different subjects are being addressed by each coalition’s Tweets: high focus refers to a small number of subjects, whereas low focus refers to Tweets spread out across many different topics. Technically, we measure focus using a two-step process. We employ an unsupervised topic modeling technique that uses the co-occurrence of words to find topics in a given series of texts: Latent Dirichlet Allocation (LDA). LDA gives us an estimate of the topics discussed on Twitter every day by each coalition. We then operationalize focus through the topic diversity of the tweets from each coalition on each day. Explained in more detail below, topic diversity summarizes the amount of different information a given text contains. Higher topic diversity corresponds with less focus.
Hypothesis 1: Compared to their pre-protest levels of focus, the opposition diputados’ tweets will become more focused and their topic diversity scores will decrease, while the regime diputados’ tweets will become less focused and their topic diversity scores will increase.
There are also important indicators of strategy that are unique to the medium of Twitter. The most relevant is the use of “hashtags” (#s), which structure discussion between people who might not know each other. There are two different strategies for using hashtags: include them in tweets to indicate the subject of your tweet, or invent specific, lengthy ones that themselves define subjects of discussion. The analysis of the use of hashtags does not easily generalize beyond the medium of Twitter, but we believe that Twitter plays a sufficiently large role in modern political communication to merit explicit study.
The former strategy has been shown to be useful in joining subjects together and connecting disparate networks (Bennett, Segerberg and Walker Reference Bennett, Segerberg and Walker2014), and is thus more useful for engaging with an existing narrative. There are two empirical implications of this strategy: using more short hashtags, and creating more tweets in which multiple hashtags co-occur. The more hashtags your tweet contains, the more (and potentially distinct) groups can be exposed to it. These implications are related because of Twitter’s then 140-character limit: longer hashtags limit the number of hashtags that can be used in a single tweet. Long hashtags are useful, though, to define and attempt to create engagement with a specific narrative. They constrain both the topic and the tone of a discussion.
We expect the opposition diputados to use more short, “labeling” hashtags and to send more tweets containing multiple hashtags. In contrast, we expect the regime diputados to use more long,Footnote 7 “discourse-structuring” hashtags and to send fewer tweets containing multiple hashtags.
Hypothesis 2: During the protests, the regime diputados will use long hashtags more frequently than the opposition.
Hypothesis 3: During the protests, the regime diputados will send tweets containing multiple hashtags less frequently than the opposition.
DATA COLLECTION
For each of the 99 regime and 66 opposition diputados in Venezuela’s unicameral legislature, we performed a manual search for an associated Twitter account. If there was any ambiguity as to whether a Twitter account belonged to a politician or an ordinary citizen with the same name, we checked to see if the account was followed by one of the party elites from either side. We were able to locate accounts for 139 of the 166 diputados (84 percent): 63 of 66 for the opposition (95 percent, similar to US Members of Congress), and 76 of 99 (77 percent) for the regime.Footnote 8 For the subsample whose tweets were analyzed in this article—those who tweeted after December 19, 2013—there were 121 accounts, 65 regime, and 56 opposition.
We used Twitter’s REST APIFootnote 9 via tweepy,Footnote 10 in the Python programming language, to collect the most recent tweets for each account. Using the /statuses/user_timelines endpoint,Footnote 11 Twitter’s API allows fetching the latest 3200 tweets for a given account.Footnote 12 We did this on April 19 and then again on May 29, at which point the protests had largely subsided. As a result, we obtained all of the tweets sent by these accounts during the time period under study. We entered each diputado’s party as an additional variable in the data set.
Table 1 summarizes of the number and distribution of the tweets collected. Although the regime had a higher number of individuals with active accounts, the opposition produced roughly 40 percent more total tweets during our period of observation, and this difference only became pronounced once the protests started. The difference is not just driven by a few prolific opposition accounts; comparing the first quartiles, medians, and third quartiles of the regime and opposition diputados indicates that the opposition diputados are more active throughout the distribution.
Table 1 Number of Tweets by Venezuelan Diputados

Note: Period of analysis: December 19, 2013 to May 29, 2014.
It is also important to note the distribution of tweets by each coalition over time. The timeline of our analysis can be divided into three periods: (1) December 19 to February 11, before the protests; (2) February 12 to May 8, protest blockades paralyze Caracas; (3) May 9 to May 28, after the protests. The beginning and end of the main protest period are noted by vertical lines in all of the figures on February 12 and May 8. For more details, see Appendix C, which contains an annotated visual timeline of the events.
Notice that before the protests, the tweet density was similar and low for both the government and opposition. Both sides saw a flare-up around the time of the murder of Miss Venezuela on January 6, which then subsided. On January 23, radical opposition leaders Leopoldo López and María Corina Machado began promoting La Salida, their plan to protest in the street and remove the regime from office. Both the regime and opposition diputados tweet most frequently in the time period between this announcement and the countrywide protests on National Youth Day, February 12. During the protest, both coalitions maintained a steady rate of tweeting. However, after the protests were effectively ended when the camps were bulldozed on May 8, there was a sharp decline in tweeting by both coalitions.
ANALYSIS
To estimate the number of overall topics being discussed on a given day by each coalition, we employed LDA (Blei, Ng and Jordan Reference Blei, Ng and Jordan2003) to generate topics and label each day’s worth of tweets with a certain distribution over those topics. LDA is an unsupervised, “bag-of-words” machine-learning algorithm used for topic modeling that is increasingly popular in the social sciences. This approach is well suited to analyzing the communication of a group of elites over time because it is unsupervised (i.e., the researcher does not decide which topics to study ex ante) and because it allows the entire corpus of information to be used.Footnote 13
The input to LDA is a corpus of documents composed of tweets aggregated as described below, each of which is a vector of N terms (within which order is irrelevant) taken from a vector of length V that contains all the terms in the corpus. LDA also requires the specification of two parameters: K, the number of topics to be modeled, and α, a concentration parameter that determines the shape of the probability distribution central to LDA. LDA analysis is conducted by treating each document as a probability distribution over latent topics and each topic as a distribution over words. That is, LDA assigns k weights to each word, one for each topic it is instructed to find and k weights to each document in the corpus.
In this case, the “documents” consist of the text of the diputados’ tweets. The start date of our analysis is December 19, 2013, two months before the start of the height of the protests as marked by López’s arrest, and the end is May 29, 2014, approximately one month after the protests subsided. We divided each day’s worth of tweets by each coalition into a separate document. This decision, to treat each diputado within a coalition as interchangeable, is premised on the idea that each coalition is coordinating (either explicitly, or through cues from the leaders) on a communication strategy, discussed below. There are 162 days included in the analysis, and thus 324 documents. For a visual depiction of this tweeting pattern, see Figure 1.Once aggregated into these documents, the terms comprising the tweets from that coalition-day are treated identically: order ceases to matter, as does the number of tweets. For example, a dozen tweets that each say only “Venezuela” spread over a single day by a dozen different diputados from the same coalition has the same impact as a single tweet that says “Venezuela” a dozen times.

Fig. 1 Tweets per day by each coalition Note: The number of tweets sent by dipudatos from each faction per day. The solid vertical lines correspond to February 12 (the beginning of the protests in Caracas on National Youth Day) and May 8 (protest camps are removed from Caracas). The dotted vertical line represents the April 10 televised sit-down between Maduro and Capriles. Trend lines for each coalition created with loess.
This approach does ignore potentially useful information by disregarding which individual diputado from a coalition produced each tweet. Given that our aim is to measure the different strategies between the coalitions, we are not concerned with which specific account sent each tweet. This assumption might not make sense if individuals within each coalition had substantially different incentives—for example, in the 2016 US election, some Republican candidates for Congress aligned themselves with the Trump presidential campaign, while others tried to distance themselves. However, in the relevant Venezuelan context, it would not make any sense for an individual PSUV diputado to distance herself from Maduro in the hope of holding office after the extralegal over-throw of the regime.Footnote 14 The tweet-pooling technique also loses information by conflating all the tweets from each day. This is unavoidable: there is not enough information in a single tweet to treat it as a document, and the machine-learning literature indicates that aggregating tweets leads to better performance for LDA (Hong and Davison Reference Hassanpour2010).
To determine the number of topics k, we performed tenfold cross-validation of both log-likelihood and perplexity analyses on the holdout sample.Footnote 15 Although the model fit improves monotonically in the number of topics, the gains from adding more topics diminish at around 50 topics (see Figure 2). There exist standard rules for choosing k, such as the conservative “one-standard-error” rule outlined in Hastie et al. (Reference Gunitsky2009), but this choice is contingent on the question LDA is being used to answer. LDA has been used most commonly to identify specific topics, prioritizing the recognizability of the topics created; in this context, the main priority is to avoid overfitting the data by choosing a conservative k. Our aim is to study the change in focus over time, so this concern is less relevant, and creating more topics allows for greater variation in the quantity of interest, even if those topics are sparsely represented and hard to identify. As a result, we follow a guideline of doubling the number of topics that the conservative approach recommends, and select k=100.Footnote 16 Our results are not an artifact of this choice; the central finding of different degrees of focus in Figure 3 is statistically significant for k=30 and k=50, though the time period for which this is true is narrower than for k=100.

Fig. 2 Testing different numbers of topics Note: The dotted line connects log-likelihood estimates for the model fitted with different number of topics, the dark line connects perplexity estimates for the model fitted with different number of topics. Vertical bars are 95 percent confidence intervals. Models fit on the entire corpus of tweets.

Fig. 3 Focus—as modeled by topic diversity—over time Note: Each point represents the topic diversity score for the opposition and regime tweets respectively, computed based on their tweets as described in the text. The solid vertical lines correspond to February 12 (the beginning of the protests in Caracas on National Youth Day) and May 8 (protest camps are removed from Caracas). The dotted vertical line represents the April 10 televised sit-down between Maduro and Capriles. Trend lines and 95 percent confidence intervals were created with loess.
Following standards in the social science literature, we use the collapsed Gibbs sampler (Griffiths and Steyvers Reference Friedman2004; Phan, Nguyen and Horiguchi Reference Perez2008). Using the R package “topicmodels” developed by Hornik and Grün (Reference Hastie, Tibshirani and Friedman2011), we ran LDA in a single chain for 1000 iterations. The text was pre-processed using “topicmodels” by removing numbers and punctuation, by converting all the text to lowercase, and by “stemming” the words so that different forms of the same word are not treated as entirely different words; stemming is especially important when dealing with Spanish objects that have four different endings depending on the number and gender of the subjects. After this pre-processing, the corpus consisted of N=50,902 terms.
In creating the topics, the algorithm estimates k=100γ for each document. For each of the 324 documents w, γ
wk
is the probability that document w pertains to topic k; note that
$\mathop{\sum}\nolimits_{k{\equals}1}^{100} {\gamma _{{wk}} {\equals}1} $
. There are 32,400 of these γs.
To analyze how focused the coalitions are over time, we measured the Shannon (Reference Schattschneider1948) Entropy of the γ distribution of each document. Commonly used in the natural sciences to measure the diversity of an ecosystem by the relative counts of each species in that ecosystem, Shannon Entropy (what we refer to throughout the article as topic diversity) is well suited to measuring how focused these documents are. It efficiently captures information about the entirety of the distribution while avoiding the imposition of arbitrary thresholds. The formula for Shannon Entropy is
$${\minus}\mathop{\sum}\limits_{i{\equals}1} {p_{i} \log _{2} (p_{i} )} $$
. In this case, because the γs in each document must sum to 1, p
i
=γ
wk
and the topic diversity score for each document is

The possible topic diversity scores range from 0 (if the γ distribution is unitary) to log2(k) (if the γ distribution is uniform). Generally, lower topic diversity scores mean a less uniform distribution, and in the case being analyzed here, a more focused message. LDA identifies some “topics” that are not semantically meaningful and assigns them non-zero weights. In order to address this issue, we analyzed only the most meaningful topics: we calculated the “semantic coherence” of each topic, following Roberts, Stewart and Tingley (Reference Qin, Strömberg and Wu2014). There was a clear clustering between the topics with “high” and “low” values of semantic coherence, so we kept only the 43 in the former category.
RESULTS
We divide the time period under study into three periods:(1) December 19 to February 11, before the protests; (2) February 12 to May 8, protest blockades paralyze Caracas; (3) May 9–28, after the protests. The beginning and end of the main protest period are noted by vertical lines in all of the figures on February 12 and May 8.
Tweet Focus: Topic Diversity
The central prediction in Hypothesis 1 is that the tweets sent by the regime will become less focused during the crisis. The results from the topic diversity scores are shown in Figure 3. Recall that lower topic diversity reflects more focused Twitter behavior (i.e., tweets are concentrated across a smaller number of topics). The absolute levels are quite different, as the opposition is far less focused than the regime. We did not explicitly predict this, but it concords with descriptive accounts of a generally well-coordinated regime and a comparatively disorganized opposition.
Importantly, the trends for the regime and opposition diputado diversities are similar in the first period: each coalition is becoming more focused during the regional protests over crime and inflation, though the opposition is consistently less focused.
Just before the planned, countrywide protests that shut down Caracas, the slope of the regime’s focus abruptly changes: after nearly two months of becoming more focused, the regime becomes significantly less focused during the protests.Footnote 17 On the other hand, the opposition dipudatos continue to become more focused even after the protest begins.
The topic diversity during the beginning of the protests is significantly higher than during the pre-protest time period for the regime, and significantly lower for the opposition, both at p<0.01, using a two-tailed t-test. However, the period for which this is the case is shorter than we had expected: beginning in mid-March, the regime begins to become more focused, reaching their pre-protest levels at around the April 10 sit-down with Maduro we had identified as a turning point for the protests. We thus find strong support for Hypothesis 1, with the caveat that the regime seems to have thought the threat of the protests had dissipated earlier than we anticipated.
The opposition, too, seems to have given up on their strategy of being focused on the protests earlier than anticipated. This suggests that the messaging strategy of the opposition may have changed. This was unexpected, and we cannot conclusively explain why this happened solely by looking at these data, a reasonable interpretation is that the moderate opposition “gave up” on supporting the aggressive strategy of La Salida to oust the Maduro government only a few weeks into the protest.
These results are supported by a brief inspection of the topics most discussed by each coalition. In addition to consistently drumming home the connection between Chávez, Maduro, President of the National Assembly (Cabello), the acronym of the regime’s party (PSUV), and the words for “the people” and “homeland” in Topic 44, their two most common top topics refer to specific events that happened at times other than the protest. Table 2 lists the top terms for each topic, sorted by how specific they are to that topic. All ten terms are common in that topic, and the terms earlier in each list are least likely to be common in other topics. Topic 76 is centered around a Chávez remembrance hashtag and mainly took place in May; many of the terms (“I will do”) are promises for what the regime will do in the future. Topic 77 is even more specific, referring to a meeting of the Community of Latin American and Caribbean States in Havana at the end of January which the regime touted as an opportunity to “make peace” with the opposition by heading off the planned protests on February 12.
Table 2 Top Terms for Relevant Topics

Note: The most common terms in the topics are the most commonly discussed by each coaltion. Each list of terms has been sorted so that the terms most specific to each topic are listed first. The terms have been translated from Spanish by the lead author; terms in italics refer to specific Twitter accounts. The original Spanish terms can be found in Appendix A.
Hashtags
Hashtags are a Twitter-specific linguistic tool used to coordinate discussion between strangers on a given subject. For example, the most common hashtag used by the regime elites in this sample was “#psuv,” the acronym for the ruling party. By including “#psuv” in a tweet, they ensure that their tweet will be shown in a list of all tweets containing “#psuv” to anyone who searches for or clicks on that hashtag. Tweets can also contain multiple hashtags, both increasing their overall visibility and allowing them to comment on multiple discussions. However, Twitter’s 140-character limit implies a tradeoff between characters used in hashtags and the amount of textual content in a tweet.
The final test of our theory of differential Twitter strategies comes from analyzing the use of hashtags. The opposition’s use of hashtags is predicted to be designed to connect with the dominant extant narrative and encourage different groups to join in on the ongoing conversation. In contrast, the regime is predicted to try to create their own narratives, giving the impression of multiple parallel conversations but limiting their ability to simultaneously engage with disparate groups.
The most straightforward way to examine hashtag use is to look at a summary of their frequencies; Appendix D presents the 100 most common hashtags for each coalition. Visual inspection suggests that the regime tweets more very long hashtags, and more often, supporting Hypothesis 2.
To understand why this is important, consider the following example: the most frequent opposition hashtag to mention the president is simply “#Nicolás,” but for the regime it is “#RodillaEnTierraConNicolásMaduro.” The latter is clearly intended as support for the president, in keeping with an explicitly designed narrative: “rodilla en tierra” is a term commonly used by the regime to connote dedication and resolve. We call this a “discourse-structuring” hashtag. “#Nicolás,” however, could be used as part of a general discussion of Maduro’s policies. Also, “#Nicolás” is only seven characters longer (compared to 31), so a tweet containing it has more room to include other hashtags or more discussion. We call this a “labeling” hashtag.
Figure 4(a) demonstrates that the regime was more likely to use discourse-structuring hashtags, but only once the protest started; this is evidence in support of Hypothesis 2, as the 95 percent confidence intervals created by non-parametric regression do not overlap for the duration of the protest. For each coalition-day, it shows the number of hashtags that were at least 20 characters long. This threshold is strongly indicative of a hashtag that consists of at least three substantive words, but see Appendix E for evidence that our results are robust to different choices for this cut-off. This effect is not being driven by a general increase in the use of hashtags by the regime. Indeed, as Figure 4(b) shows, both coalitions used a higher number of hashtags during the protest, and the increase is actually much more pronounced for the opposition.

Fig. 4 Hashtag use Note: (a) Plots the number of hashtags that were at least 20 characters long tweeted per day by the two colations. (b) expands this number to the total count of hashtags tweeted per day by the two coalitions. The solid vertical lines correspond to February 12 (the beginning of the protests in Caracas on National Youth Day) and May 8 (protest camps are removed from Caracas). The dotted vertical line represents the April 10 televised sit-down between Maduro and Capriles.

Fig. 5 Hashtag co-occurrences Note: (a) Shows the raw number of tweets containing multiple different hashtags tweeted per day by the two coalitions. (b) Weights this number by the number of hashtags each of those tweets contained. The solid vertical lines correspond to February 12 (the beginning of the protests in Caracas on National Youth Day) and May 8 (protest camps are removed from Caracas). The dotted vertical line represents the April 10 televised sit-down between Maduro and Capriles.
Before the protest, the two coalitions were equally (un)likely to use a discourse-structuring hashtag, but after the protests began, the regime started employing these lengthy hastags to develop alternative narratives to compete with the narrative of La Salida.
To illustrate, the regime diputados used the following hashtags at different times to encourage their followers to talk about specific topics. #DesconectateDeLaGuarimba explicitly encouraged people to stop participating in and paying attention to the protest camps in Caracas. #ChávezViveLaPatriaSigue was one of several discourse-structuring hashtags that claimed that the spirit of Chávez lived on and that patriots should support the ruling party. #GringosyFascistasRespeten emphasized the popular regime narrative calling their opponents facist US sympathizers who need to respect Venezuelan sovereignty. Relatedly, #VzlaBajoAtaqueMediatico claimed that the international press was being unfairly supportive of the opposition as part of an effort to unseat the anti-US regime. While each of these acknowledged that the protests were going on, each was an attempt to develop alternative narratives and to generate discussion of the situation that was unrelated to the demands and complaints of the protesters.
However, this strategy comes at a cost: the length of “discourse-structuring” hashtags makes them less useful than “labeling” hashtags for connecting different conversations by different groups. Following Bennett, Segerberg and Walker (Reference Bennett, Segerberg and Walker2014), Hypothesis 3 predicts that the opposition will be more likely to send tweets containing multiple hashtags during the protest.
In Figure 5, we find consistent support for this hypothesis. Panel (a) plots the total number of tweets sent by each coalition containing more than one hashtag, while panel (b) weights each occurrence of multiple hashtags by the number of hashtags in that tweet. In both cases, there were few tweets containing multiple hashtags before the protest started, and a much larger increase in this technique for the opposition than for the regime during the high point of the protests.
Regime Social Media Strategy During Crisis
We have found support for our claim that regimes employ “third generation” social media strategies during times of acute political crisis. In particular, in a context in which they could not silence or sufficiently discredit La Salida’s narrative, the regime diputados attempted to distract from the protesters by discussing many different issues and developing competing narratives and talking points. We have shown that the regime tended to discuss more distinct topics per day during the protest than before or after the protest, and the opposite is the case for the opposition. We have also shown that the regime used more “discourse-structuring” hashtags during the protest, each of which was designed to develop a conversation unrelated to the talking points of La Salida.
CONCLUSION
The stated goal of the La Salida movement, to remove the regime from power before the next election, was not accomplished during the summer of 2014. Although underlying macroeconomic and political concerns persisted, the regime effectively demonstrated their repressive capacity. Our findings show that part of their strategy involved actively engaging with Twitter to try and change the agenda away from the one driven by La Salida. The moderate opposition diputados, on the other hand, used Twitter to draw more attention to this established narrative, but only as long as it was in their interest—they seemed to have abruptly changed after the April 10 sit-down with Maduro. The moderate opposition was apprently not permanently committed to the strategy of La Salida.
This change in strategy could not have been detected without the use of social media data. The underlying theoretical argument about the powerful trying to limit the scope of conflict and the less powerful trying to expand it dates back at least to Schattschneider (Reference Sanovich, Stukal and Tucker1960). Social media allows researchers to more easily observe and test these kinds of theories. While this article has set out to establish that social media is used by authoritarian regimes as a part of a strategy of regime resilience, and thus that social media use by elites in these countries is intrinsically important, it also affords opportunities to learn about strategic goals in otherwise opaque political contexts.
We cannot measure whether or not the Twitter strategies employed by the regime were decisive or even effective; that question is outside the scope of this article, but would be an excellent avenue for future research.
The quantitative textual analysis developed in this article is a novel implementation of well-established machine-learning techniques. Although looking at the topic diversity scores and distribution of top topics is less qualitative than traditional methods, it has the advantages of being objective and easily replicable. Moreover, what this approach allows us to study—the amount of information being transmitted—is essentially impossible for humans to measure. Machine learning allows researchers to extract patterns from elite communication, even if the literal content of that communication is propagandistic.
Future research should take even more advantage of idiosyncratic social media use by elites to try to better understand the unobservable inner workings of non-democratic regimes. An excellent example of this approach is Malesky and Schuler (Reference Mainwaring2010), who use the behavior of Vietnamese legislators in questioning sessions to draw inferences about their incentives and that of the regime more generally. That institution is peculiar to Vietnam, but social media is not, and social media thus offers a similar avenue for analysis in many contexts.