Overview, Rationale, and Objectives
Prior research has demonstrated that genetic and environmental factors play critical etiologic roles in the pathways to cannabis use disorder (CUD; Gillespie et al., Reference Gillespie, Kendler, Prescott, Aggen, Gardner, Jacobson and Neale2007a, Reference Gillespie, Neale, Prescott, Aggen and Kendler2007b, Reference Gillespie, Kendler and Neale2011, Reference Gillespie, Neale, Lubke and Kendler2012). Although the role of environmental risk factors is increasingly better understood (Gillespie et al., Reference Gillespie, Neale, Lubke and Kendler2012), very little is known about the putative genes involved because to date there have only been two published tests of genome-wide association for a CUD phenotype (Agrawal et al., Reference Agrawal, Lynskey, Hinrichs, Grucza, Saccone, Krueger and Bierut2011; Verweij et al., Reference Verweij, Vinkhuyzen, Benyamin, Lynskey, Quaye, Agrawal and Medland2012). This is despite the fact that cannabis use and CUD are major public health issues that have long attracted public concern and controversy in many developed countries (Schlosser, Reference Schlosser2004).
Improved phenotypic measurement, along with the identification of quantitative trait loci (QTL) responsible for variation in CUD, is still required to fill gaps in our knowledge and to develop targeted treatments, as well as to provide an empirical basis for addressing policy issues and public concerns about the putative effects of cannabis use. The US National Institutes of Health (NIH) and National Institute on Drug Abuse (NIDA) ‘Pathways to Cannabis Use, Abuse and Dependence’ project was funded to address these empirical needs, by beginning to elucidate the genetic and environmental risk factors in the pathways to CUD. This article describes in detail the twin sample, methods, and procedures involved in the data collection, along with preliminary findings and plans for future research and collaboration.
Project Aims, Ascertainment, and Sample Characteristics
Data for this project have been collected from Australian twins and their non-twin siblings as part of the ongoing Brisbane Longitudinal Twin Study (BLTS) at the Queensland Institute of Medical Research (QIMR). The BLTS began in 1992 when twins were recruited from primary and secondary schools in the greater Brisbane area via media appeals and by word of mouth. Twins were ascertained along with family members as part of a study examining the development of melanocytic naevi at ages 12 and 14, and of cognition at age 16 at the QIMR.
Currently, the BLTS sample comprises both adolescent and young adult twins (3,408 individuals) and their non-twin siblings (1,572), constituting 1,703 families. This includes both monozygotic (MZ) and dizygotic (DZ) twin pairs, including opposite-sex DZ twin pairs, along with singleton siblings of twins, and the twins’ parents. The proportions of twins by sex and zygosity in the sample closely mirror population expectations, further strengthening our confidence in its representativeness. The BLTS recruits approximately 100 new twins per year and is now a longitudinal collection of psychiatric phenotypes, environmental and psychological risk factors, as well as neurobiological correlates and endophenotypes for psychiatric disorders that are summarized in Figure 1.

FIGURE 1 Summary of Brisbane Longitudinal Twin Study measures and phenotypes.
The BLTS is the core resource on which this project is based. Data collection for the project began in 2009 and is funded by the NIH NIDA to explore the genetic and environmental pathways to cannabis use, abuse, and dependence on 1,000 BLTS twins and their siblings. These data are being supplemented with an additional 1,100 twins, with funding from the Australian National Health and Medical Research Council (NHMRC), along with seed funding from an NHMRC Australia grant to the co-author IH. Data collection is expected to be completed by mid-2013.
When the current project began, the average age of the BLTS sample was 22.8 years (SD = 4.3). Based on current sample size and factoring sample increases of 100 families per year, the project's sample will include a minimum of 2,100 twins and non-twin siblings who will be 22 years or older by 2013. This age is close enough to the ages when initiation of cannabis and other drug use occurs, but old enough to have passed through the maximum period of risk for onset of CUD and other drug use disorders (DUDs) (Gillespie et al., Reference Gillespie, Neale and Kendler2009b). The 2010 National Drug Strategy Household Survey found that the mean age of initiation of cannabis use in Australians is 18.5 years (Australian Institute of Health and Welfare, 2010). Typical response rates across the BLTS projects since 1992 range from 73% to 85% (Wright & Martin, Reference Wright and Martin2004).
Population Ancestry
The United Kingdom and Ireland were traditionally the principal countries of origin for the majority of immigrants to Australia, reflecting the colonial history of the country. Since World War II (1939–1945), Australia's population has become more ethnically diverse as people have emigrated from a wider range of countries. The proportion of residents born in other countries increased from 10% in 1947 to 24% in 2000. In 1947, 81% of new arrivals came principally from the United Kingdom and Ireland, and to a lesser extent from New Zealand, Canada, South Africa, and the United States. Although only 39% of new arrivals in 2000 came from these major English-speaking countries, people of European descent still constitute 91% of Australia's population. Most claim British or Irish heritage, but there are also Italian, Dutch, Greek, German, and other European groups. Moreover, twins and siblings from the BLTS who were recruited in South East Queensland are largely Anglo-Saxon or Anglo-Celtic background. The BLTS sample will reflect the population structure of Australia at the time this twin cohort were first recruited, and the few minority individuals who will be included in this cohort will be of predominantly Asian ancestry. The remainder of the sample will be of European ancestry. The under-representation of minorities is scientifically justified given the relative lack of genetic epidemiological data on cannabis use and related disorders.
Summary of all Available Measures and Phenotypes to Date
Since 1992, the BLTS data collection has been ongoing, with twins at varying ages and stages of participation. A key strength of this project will be the ability to tie the CUD and DUD data with a phenotypically rich, longitudinal collection of environmental and psychological risk factors, psychiatric phenotypes and diagnoses, as well as neurobiological correlates and endophenotypes such as brain imaging (see Figure 1).
Adolescents
In addition to measures of melanocytic naevi at ages 12 and 14 (see Zhu et al., Reference Zhu, Montgomery, James, Trent, Hayward, Martin and Duffy2007), BLTS twins and siblings have been measured with the Junior Eysenck Personality Questionnaire (Eysenck, Reference Eysenck1965, Reference Eysenck1972) at 12, 14, and 16 years, along with tests of cognition at 16 years (see Wright & Martin, Reference Wright and Martin2004). The proportions of twins by sex and zygosity in our sample closely mirror population expectations, further strengthening our confidence in its representativeness. In addition to these initial target variables, personality (most pertinently, neuroticism) is assessed.
Since 1999 we have also been collecting common symptoms of anxiety, depression, and somatic distress using the Somatic and Psychological Health Report (SPHERE; Hickie et al., Reference Hickie, Davenport, Hadzi-Pavlovic, Koschera, Naismith, Scott and Wilhelm2001a, Reference Hickie, Davenport, Naismith and Scott2001b) at ages 12, 14, and 16. The SPHERE is a 34-item self-report questionnaire (SRQ) incorporating items from other self-report and diagnostic instruments (Schedule of Fatigue and Anergia; Hickie et al., Reference Hickie, Hooker, Hadzi-Pavlovic, Bennett, Wilson and Lloyd1996); and General Health Questionnaire 30) and criteria for somatization disorder from DSM-III-R (American Psychiatric Association, 1987). The SPHERE was developed and validated in two samples of primary care attenders (N = 1,593; Hickie, et al., Reference Hickie, Hooker, Hadzi-Pavlovic, Bennett, Wilson and Lloyd1996, Reference Hickie, Davenport, Hadzi-Pavlovic, Koschera, Naismith, Scott and Wilhelm2001a). Further data on psychometric properties have been derived from other smaller specialist samples (breast cancer, chronic pain study, older patients, post-infective patients, specialist psychiatry practice) and other general practice samples (Bennett et al., Reference Bennett, Goldstein, Lloyd, Davenport and Hickie2004; Butow et al., Reference Butow, Meiser, Price, Bennett, Tucker, Davenport and Hickie2005; Clover et al., Reference Clover, Carter, Adams, Hickie and Davenport2009; Wilhelm et al., Reference Wilhelm, Finch, Davenport and Hickie2008). Adolescent-type anxiety and depression both show substantial heritability (~40%; Hansell et al., Reference Hansell, Wright, Medland, Davenport, Wray, Martin and Hickie2012).
For twins at age 12 we have recently introduced the novel Verbal and Spatial Reasoning Test for Children (VESPARCH; Mellanby & Langdon, Reference Mellanby and Langdon2010) to assess verbal and spatial abilities. At age 14, the twins complete a binocular rivalry task, which gives an estimate of the inter-hemispheric attentional switching rate, a putative endophenotype for bipolar disorder (Miller et al., Reference Miller, Hansell, Ngo, Liu, Pettigrew, Martin and Wright2010). At age 16, a battery of cognitive tasks is administered, including verbal and performance IQ (Multidimensional Aptitude Battery [Jackson, Reference Jackson1984]; and Wechsler Adult Intelligence Scale—Revised [Wechsler, Reference Wechsler1981]), relational processing, perceptual speed, choice reaction time, working memory (delayed response task) as well as reading and language tests and academic achievement. Electroencephalography is also recorded while at rest, and during an n-back task so that event-related potentials can be recorded.
Young Adults
At age 21, the twins return to participate in an NIH- and NHMRC-funded brain imaging study that includes structural and functional (at rest, and during an n-back task) Magnetic resonance imaging (MRI), as well as diffusion tensor imaging (DTI—HARDI). To date, these data (N = 560 so far, budgeted for 1,100 with current funding, and new funding is being applied for to expand this) are collected on the 4T imaging facility at the Wesley Hospital, Brisbane, Australia. Processing of these images is in collaboration with the University of Queensland and UCLA (Blokland et al., Reference Blokland, McMahon, Hoffman, Zhu, Meredith, Martin and Wright2008; de Zubicaray et al., Reference de Zubicaray, Chiang, McMahon, Shattuck, Toga, Martin and Thompson2008). With seed funding from the Brain and Mind Research Institute at the University of Sydney we have extended the same brain imaging protocol back to twins aged 16 and 12 years. To date, 80 twins in each age group have been collected as proof of principle.
Since 2007 we have also been collecting SPHERE data at age 20+ in those undergoing MRI scanning. Currently, we are systematically collecting SPHERE data on all subjects who have participated in any phase of the BLTS.
Detailed Description of Project Protocols
Since mid-2010 we have begun obtaining, under the NIH/NIDA Pathways to Cannabis Use, Abuse and Dependence project, DSM-IV and DSM-V item level data on cannabis abuse and dependence and diagnostic data for nicotine and alcohol, as well as pilot epidemiological data for ecstasy and methamphetamine use. Using the same protocol described below, we have also begun obtaining DSM-IV item-level data on mood, anxiety, and fatigue with funding from the NHMRC.
Between 2009 and 2011, data collection for the NIH/NIDA and NHMRC projects began via online survey and computer-assisted telephone interview (CATI) using Version 1 of the protocol. Beginning July 1, 2012, the online survey and CATI instruments were merged into an entirely online protocol (Version 2). Under both protocols, described in detail below, ascertainment began with adult twins and non-twin singleton siblings from the BLTS sample in order to obtain data from individuals who had passed through the age of maximum risk for the onset of cannabis use (typically 16–18 years) and cannabis-related problems (typically 19–21 years). Flowcharts of Versions 1 and 2 are illustrated in Figures 2 and 3, respectively.

FIGURE 2 Summary of the Version 1 data collection protocol.

FIGURE 3 Summary of the Version 2 data collection protocol.
Version 1
Version 1, the online survey (referred to as 19UP Online Survey), assessed a variety of phenotypes such as general health, relationships, migraine and headaches, attention, hair loss, sleep and waking, daily activity, personality, romantic preferences, and joint flexibility. The general health section measured lifetime use of alcohol, nicotine, cannabis (e.g., marijuana, hashish, tetrahydrocannabino (THC), or ganja), as well as cocaine (e.g., coke, crack), amphetamine-type stimulants (e.g., speed, ice, diet pills), inhalants (e.g., nitrous glue, petrol, paint thinner), sedatives or sleeping pills (e.g., Valium, Serepax, Rohypnol), hallucinogens (e.g., lysergic acid diethylamide aka LSD, acid, mushrooms, phencyclidine aka PCP), opioids (e.g., heroin morphine, methadone, codeine), Ecstasy, Ketamine, gamma-Hydroxybutyric acid aka GHB, party drugs (e.g., E or X aka Ecstasy, 3,4-methylenedioxy-N-methylamphetamine aka MDMA, Special K, Fantasy), and over-the-counter and prescription analgesics and stimulants for non-medical purposes. In addition to age of initiation, lifetime and past 3-month use for each substance, subjects were also asked if they had ever used or consumed any of the substances while drinking alcohol. Table 1 shows the prevalence of alcohol, nicotine, and cannabis use along with patterns of past 3-month use. Based on sample of 132 subjects who repeated the online survey, Spearman's r correlations when averaged across the sexes for lifetime alcohol, cannabis, and nicotine use were 0.70, 0.83, and 0.76, respectively. Table 2 shows the prevalence of illicit drug use along with patterns of past 3-month use. The prevalence varied widely according to drug; for example, from 5.2% for opioids to 34.4% for Ecstasy, Ketamine, GHB, or party drugs among males. For males and females alike, prescription painkillers and analgesics were the most likely drugs to be consumed in the past 3 months compared to opioids or inhalants for males (1.3%) or inhalants for females (0.5%). With the exception of opioids, prescription painkillers, and stimulants, more than 50% of self-reported drug users also reported using alcohol at the same time. With the exception of sedatives and prescription painkillers, the retest correlations were moderate to high for both males and females.
TABLE 1 Lifetime Prevalence (%) of Alcohol, Nicotine, and Cannabis Use and Patterns of Past 3-month Drug Use Based on 711 19UP Online Survey Responses
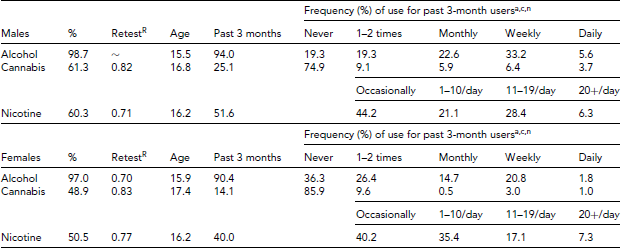
aWithin the past 3 months, how often have you had five or more drinks (if male), or four or more drinks (if female), within a day?
cIn the past 3 months, how often have you used cannabis (marijuana, pot, grass, or hash)?
nWithin the past 3 months, did you smoke cigarettes . . .?
RSpearman's ρ based on 132 subjects with repeated measures.
TABLE 2 Lifetime Prevalence (%) of Illicit Drug Use, Patterns of Past 3-Month Drug Use, and Prevalence of Drug Use with Alcohol Based on 709 19UP Online Survey Responses

nmpFor non-medical purposes and includes over-the-counter medications.
RTest–retest Spearman's ρ correlations based on N = 132.
Following the online survey, subjects were contacted within 6 weeks and scheduled for the CATI. The CATI required ~45–60 minutes to complete and began with an extensive demographic and background assessment, including measures of twin pair similarity. Central to the Pathways to Cannabis Use, Abuse and Dependence project, the CATI included DSM-IV-based criteria to assess alcohol, nicotine, cannabis (marijuana, hashish, THC, or ganja), methamphetamine (speed, base, d-meth, ice or crystal meth, shabu, batu, Tina, glass, or diet pills), and Ecstasy (aka E, XTC, eccy, bickies, & the love drug) use, abuse, and dependence. These sections began with basic screening items, initiation, and frequency of use measures. Table 3 summarizes the prevalence and patterns of cannabis use based on data from 626 CATIs. In this sample, males endorsed more lifetime and frequent cannabis use, and although tests of significance were not performed, the mean age of initiation was lower among males, whereas age of most frequent use was lower among females.
TABLE 3 Prevalence and Patterns of Cannabis Use Based on Data From 626 Computer-Assisted Telephone Interviews Comprising 259 Males and 367 Females

Administration of the Nicotine section, which was modeled on the Fagerstrom Test for Nicotine Dependence (Heatherton et al., Reference Heatherton, Kozlowski, Frecker and Fagerstrom1991), was contingent on responses to the online survey. Only subjects who endorsed having initiated (or who reported current smoking status) and who smoked 100 or more cigarettes (four to five packets) in their lifetime were eligible to be asked the CATI nicotine questions. For Alcohol, only subjects who endorsed five or more drinks for males or four or more drinks for females at least once a week for a month or more were subsequently asked the abuse and dependence items. For Cannabis, only subjects who reported having used marijuana at least six times in their lifetime were asked the abuse and dependence items. Craving was included for Alcohol and Cannabis in order to determine caseness for DSM-V Alcohol and Cannabis use disorder.
Table 4 lists the prevalence of each CUD criteria, along with average age of initiation for each symptom. A frequent desire or attempt to stop or cut down was the most commonly endorsed item compared to legal problems, and using cannabis to relieve symptoms of withdrawal, which were both rarely reported. Almost no women reported legal problems. Regarding onset, with the exception of withdrawal symptoms and legal problems for women, all symptoms were endorsed before age 20 across sexes.
TABLE 4 Prevalence (%), Age of Onset for Each DSM-IV and DSM-V Cannabis Use Disorder Criteria Along with Monozygotic (r MZ) and Dizygotic (r DZ) Polychoric Twin Pair Correlations Based on Data from 626 Computer-Assisted Telephone Interviews

All criteria were prefaced with: ‘During this time when you used cannabis the most did you . . .’. Data based on 162 MZ and 126 DZ twin pairs.
Table 4 also includes MZ and DZ polychoric correlations adjusted for the effects of age. Although the patterns of correlations suggest significant familial aggregation, explained by varying degrees of additive genetic and shared environmental risks, larger sample sizes, along with formal univariate model fitting methods, are required to estimate precise estimates of genetic and environmental components of variance.
The CATI screening items for the Alcohol, Nicotine, and Cannabis sections and their corresponding DSM-IV abuse and dependence criteria were purposely written to be isomorphic with items used in previous Mid Atlantic Twin Registry (Kendler & Prescott, Reference Kendler and Prescott2006) and Minnesota Family Twin Study (MFTS; Iacono et al., Reference Iacono, Carlson, Taylor, Elkins and McGue1999) surveys in order to facilitate future data merging and meta-analyses. When combined, these data will increase the power (1) to test cross-country phenotypic measurement invariance as well as (2) to increase the power detect QTL for CUD, DUDs, and correlated phenotypes. For Alcohol, Nicotine, Cannabis, and Ecstasy the age of initiation, age at most frequent use, and quantity of drug consumption during most frequent use were also asked.
The CATI also assessed the prevalence, onset, and patterns of Ecstasy and methamphetamine use. The rationale for including measures of Ecstasy and methamphetamine use was to obtain basic epidemiology data in order to inform pilot analyses as part of future project proposals.
As summarized in Table 5, one-third of males and over one-quarter of females have reported lifetime Ecstasy use before turning 21 years. A majority of users take Ecstasy at dance or rave parties, bars, pubs, and nightclubs, and typically once a year and in pill form. Although our preliminary data suggest that more than one-third of male and one-half of female users believe that Ecstasy is unsafe, almost half of the male lifetime users and over one-fifth of female users say that they would use the drug again. And although most users were unsure about the content of the Ecstasy they consumed, less than 10% reported having ever used a tester kit. Finally, despite concerns over drug safety and content awareness, over three quarters of users reported no psychological problems. Still, over 21% of males and 12% of females reported continual use despite psychological problems with similar numbers reporting use in dangerous situations. Finally, almost all subjects reported that Ecstasy was easy to obtain.
TABLE 5 Prevalence of Lifetime Ecstasy Use, Levels of Drug Awareness, Frequency of Psychological Problems as a Consequence of Use, and Perceived Ease of Availability

aAlso known as E, XTC, eccy, bickies, & the love drug.
bNo reported administration with/via liquid E, injection, or suppository.
cPills, snorts, injections, or liquid E.
dItem prefaced with statement, ‘Australian ecstasy is often cut with other substances’.
eIncluding energy drinks, antidepressants, or other drugs just before taking ecstasy to avoid or reduce the ‘comedown’ or negative effects.
fWhen you used ecstasy the most.
As shown in Table 6, amphetamine or methamphetamine use is also prevalent, with 21.6% of males and 13.9% of females reporting lifetime use before age 21. The most common type of amphetamine or methamphetamine is in powdered form typically taken by swallowing.
TABLE 6 Prevalence and Basic Epidemiology of Amphetamine (or Methamphetamine) Use Based on 259 Males and 367 Females With Complete Data

aSpeed, base, d-meth, ice, or crystal meth, shabu, batu, Tina, glass, or diet pills.
The remaining sections of the CATI assessed work and occupation history, depression, anxiety, mania and mania screening, psychosis, and Internet use. The mental health sections were based on a modified Composite International Diagnostic Interview (Kessler et al., Reference Kessler, Chiu, Demler, Merikangas and Walters2005) using item-based criteria to determine caseness for DSM-IV Major Depressive Disorder, Major Depressive Episode, Minor Depressive Disorder, Recurrent Brief Depression, Dysthymia, Hypomanic Episode, Manic Episode, Agoraphobia, Agoraphobia with/without panic, Generalized Anxiety Disorder, Panic Attack, Panic Disorder, Social Phobia, and Specific Phobia summarized in Table 6. Five items from the headspace National Telephone Survey (Burns et al., Reference Burns, Davenport, Durkin, Luscombe and Hickie2010) were also included to evaluate the prevalence of lifetime mental health diagnoses and treatment experiences. See Table 7 for summary of the prevalence of lifetime DSM-IV mental disorders.
TABLE 7 Prevalence (%) of Self-Reported DSM-IV Lifetime Mental Disorders Based on 625 Computer-Assisted Telephone Interviews

Column percentages have been reported. All diagnoses have been derived using the DSM-IV criteria for Major Depressive Episode (Depression), Social Anxiety Disorder and/or Panic Disorder (Anxiety), Psychotic Episode (Psychosis), Manic/Hypomanic Episode (Mania/ Hypomania), Alcohol Abuse and Alcohol Dependence (Alcohol Misuse).
Informed Consent, Reimbursement, and Ethical Approval
In Version 1 of the informed consent protocol, all subjects were given full information regarding the study aims, protocols, and requirements. Refusal, delays, and information changes were recorded and submitted for logging. Those who did not complete the online survey after repeated promptings were offered the option of receiving a paper copy, or having an interviewer complete it for them as they read out the questions over the phone. A recent survey of similar dimensions on this cohort achieved 79% compliance using these same procedures. All participants who completed the online survey and CATI were reimbursed with $25 and $50 gift vouchers, respectively. QIMR has found that this reimbursement strategy substantially increases compliance and interview participation rates, which is ~80%. All data provided were transferred by encryption and stored on secure QIMR servers. Prior to analysis, these data were stripped of identifying information linking individuals to their responses. Protocols for the online survey and CATI were extensively reviewed prior to approval by the QIMR Human Research and Ethics Committee (HREC) and the Virginia Commonwealth University (VCU) Institutional Review Board (IRB).
Interviewer Training and Quality Control
CATI interviewers used in the first protocol were selected from an experienced pool of QIMR staff who participated in a 2-week training session consisting of didactic instruction and supervised practice interviews. During the second week of training, interviewers conducted at least three interviews with community volunteer subjects under the supervision of a faculty trainer or senior staff member. Interviewees were told that they were participating in a training interview, and that a supervisor would be listening in on the call. Following consent, actual CATI interviews were recorded for editing and quality control. For quality control and to prevent interviewer drift, 5% of interviews were re-entered by an independent editor listening to the recorded interview, on a continuing basis throughout the project.
As of March 31, 2012, there were 626 twins and non-twin siblings from 444 families who had completed the CATI. This included 367 (58.6%) females as well as 127 complete and 205 incomplete twin pairs along with 167 non-twin siblings.
Version 2
In order to make data collection more cost-efficient, the 19UP Online Survey and CATI were merged into an entirely online series of SRQs and released in July 2012. All CATI questions were converted and transferred to an online protocol that was divided into a series of three smaller SRQs: SRQ-1 includes all NIH/NIDA-funded drug phenotypes; SRQ-2 includes a range of heritable phenotypes including migraine and headaches, inattention, hair loss, and joint flexibility; SRQ-3 assesses relationships, romantic preferences, personality, and Internet use. In terms of risk to participants, the QIMR HREC and VCU IRB approved procedures in the CATI protocol were operationalized and re-approved for the online automated protocol.
Ascertainment
Subject ascertainment for participation in the Version 2 protocol again began with adult twins and non-twin singleton siblings in order to obtain data from individuals who had passed through the age of maximum risk for the onset of cannabis use. Subjects are sent an approach letter describing the project's aims and protocols and inviting them to participate in a series of three online SRQs. For non-responders, follow-up telephone calls are then made every 2–4 weeks to monitor progress, note reasons for refusal to participate, answer questions, and offer provision to complete interview over telephone if required.
The SRQ-1 included identical demographic, occupational, and general health questions, followed by measures of licit and illicit drug use as well as psychiatric criteria for diagnosing mental health and DUDs as described in Version 1 above. At the beginning of SRQ-2 and SRQ-3, subjects are required to reconfirm their informed consent. SRQ-2 assesses a range of heritable phenotypes including height, weight, hair and skin color, hair texture, hair graying and balding, handedness, sunscreen use, moliness and melanoma, acne, herpes, age of death of close family members, asthma, wheezing and prevalence of atopic conditions, use of eyeglasses, travel sickness, migraines and recurrent headaches, pain tolerance, physical activity, joint flexibility, fibroids, polycystic ovarian syndrome, endometriosis, menstruation and menopause, anorexia and bulimia nervosa, and sleep patterns. SRQ-3 assesses relationship status, romantic relationships and romantic preferences, personality and Internet use. It should be noted that SRQ-2 and SRQ-3 are funded from different sources than SRQ-1.
Blood Ccollection, Siblings, and Informed Consent
Blood has been collected for DNA and various hematological and immunological measures. Where possible, any singleton siblings of the twins whose age was within 5 years of the twins are also recruited and tested in an identical protocol. For all studies, written, informed consent was obtained from a parent or guardian and ethics approval was obtained from the Human Research Ethics Committee at the QIMR.
Individual Genome-Wide Association, Gene Expression, and Methylation Data
All twins and siblings with 19UP Online Survey and CATI data have been or will be genotyped using the Illumina 610k SNP array. As of May 2012, ~2,639 (74% of the sample) have been genotyped using the Illumina 610k SNP array. Extensive quality control has already been performed using PLINK (Purcell et al., Reference Purcell, Neale, Todd-Brown, Thomas, Ferreira, Bender and Sham2007). This has included tests of Hardy Weinberg Equilibrium, analysis of missing genotype rates, inbreeding, identity by state, identity by descent statistics for individuals and pairs of individuals (to ensure that reported relationships are accurate and that distant relatives in the data set are properly accounted for), non-Mendelian transmission in family data (when available), sex checks based on X chromosome short nucleotide polymorphisms (SNPs), and tests of non-random genotyping failure. The data were then imputed to contain 2,428,106 SNPs. Imputation boosts the power of many chips toward levels obtained from hypothetical ‘complete’ arrays containing all HapMap SNPs (Howie et al., Reference Howie, Donnelly and Marchini2009; Spencer et al., Reference Spencer, Su, Donnelly and Marchini2009). Moreover, imputation, which is easily implemented in the software program PLINK (Purcell et al., Reference Purcell, Neale, Todd-Brown, Thomas, Ferreira, Bender and Sham2007), combines information across multiple reference panels, which will mean that genome-wide association study (GWAS) data obtained from different arrays such as the MFTS data (Iacono et al., Reference Iacono, Carlson, Taylor, Elkins and McGue1999) can be merged for future meta-analyses.
A subset of the BLTS subjects have participated in the Brisbane Systems Genetics Study funded by the Australian NHMRC grants to Drs P. Visscher and A. McRae. This is a family-based study aimed at elucidating the genetic factors affecting gene expression methylation and the role of gene regulation in mediating endophenotypes and complex diseases. To date, genome-wide expression has been assessed on 870 individuals using the Illumina HumanHT-12 v3.0 413 Beadchip. We are currently in the process of assessing methylation status on approximately 630 of the same subjects at ~485,000 CpG sites across the genome using the Infinium HM450 and HM27 BeadChips.
Zygosity Diagnosis
For BLTS same-sex twin pairs, zygosity has already been determined by typing nine independent DNA micro-satellite polymorphisms plus the X/Y amelogenin marker for sex determination by polymerase chain reaction (ABI Profiler system) and for most this has been (or will be) confirmed by GWAS.
Plans for Data Analysis
This section summarizes the statistical techniques for analyzing the 19UP Online Survey, CATI, SRQ, and genome-wide association data. Once phenotypic and diagnostic data become available, our intention is to begin running psychometric modeling and biometrical genetic analyses before turning to preliminary tests of genome-wide association.
The project's initial aim was to replicate previous findings based on psychometric modeling (latent factor, latent class, and factor mixture modeling) of cannabis use and CUD phenotypes using isomorphic twin data from Virginia and Minnesota (Gillespie et al., Reference Gillespie, Kendler, Prescott, Aggen, Gardner, Jacobson and Neale2007a, Reference Gillespie, Neale, Prescott, Aggen and Kendler2007b, Reference Gillespie, Neale, Jacobson and Kendler2009a, Reference Gillespie, Neale and Kendler2009b, Reference Gillespie, Kendler and Neale2011, Reference Gillespie, Neale, Lubke and Kendler2012; Iacono et al., Reference Iacono, Carlson, Taylor, Elkins and McGue1999). Employing the same model-fitting strategies in these reports, our aim is to derive and test empirical measures of cannabis use and CUD using the Australian BATS data. When combined with data from North America, tests of measurement invariance can be performed to identify sex, age, and cultural differences in the point prevalence of cannabis use and CUD as well as other illicit DUDs.
The next step will be to fit biometrical genetic models to the CUD, DUD, and correlated phenotypic data in order to identify phenotypes with largest genetic variance. Multivariate twin model fitting to the twin data will then enable us to identify the sources of genetic and environmental covariance between correlated and comorbid DUD and psychiatric phenotypes. Finally, the empirically best fitting CUD and DUD phenotypes will then be used as part of preliminary tests of genome-wide association involving combined SNP and genomic data available from the BLTS. Assuming between 7.8% and 15.1% of women and men will meet criteria for a lifetime diagnosis of cannabis abuse or dependence, then among the 2,100 subjects with complete phenotypic and genotypic information, between 164 and 321 individuals will meet traditional diagnostic criteria for a CUD. Analyses using the DSM-IV abuse and dependence diagnoses will be conducted in two ways: (1) all remaining subjects will be used as controls, and the GWAS analyses will make use of the traditional DSM diagnostic categories as well as an empirically derived quantitative CUD measure based on item responses; (2) only those who used cannabis but did not develop CUD will be used as controls. These complementary analyses will disentangle a potential confound arising because CUDs are conditional upon use, and the fact that for some individuals, liability to manifest a CUD remains a latent prospect because of lack of exposure opportunities. In other words, it will distinguish between those factors that influence both initiation and progression to substance use disorders from those that influence its use and progression only.
Additional GWAS analyses will be based on individual maximum-likelihood latent phenotypic and latent genetic factor scores calculated for the entire sample. The success of this proposed method for estimating factor scores that maximizes genetic variance has already been demonstrated by researchers at VCU with mood and anxiety disorders and related phenotypes (Hettema et al., Reference Hettema, An, Bukszar, van den Oord, Neale, Kendler and Chen2008). Additional analyses will model the association both at the factor level and at the level of the individual DSM-IV/V items (while accounting for the covariation among them). This approach can nest the test of association within the multivariate models that best fit the cannabis use and DSM-IV/V item level data. This will permit genome-wide tests of associations based on the best-fitting CUD phenotypes that may include two or more latent phenotypes to best explain the covariation.
Importance of the Project
Data collected from this population-based cohort of Brisbane twins using the (Version 1) 19UP Online Survey and CATI interviews clearly suggests that major mental disorders (notably anxiety and depressive disorders) as well as licit and illicit drug use are prevalent among young adults. We have identified high lifetime rates of alcohol, nicotine, and cannabis use, as well as cocaine, amphetamine-type stimulants, and Ecstasy among males and females alike. We have also shown in our pilot data that for Ecstasy users, their knowledge of drug content and safety is lacking, and this underscores the need for better epidemiological data to address an emerging public health concern. The project has the capacity to examine longitudinally the relationships between ages of onset of various substance misuse disorders and a range of other specific mental disorders.
Central to the project's focus, our data suggest that among lifetime cannabis users, the psychiatric criteria for CUD including craving are also prevalent (with the exception of legal problems). With data collection to be completed toward late 2013, our next step will be to model and derive an empirical CUD phenotype based on the DSM-IV and DSM-V psychiatric criteria. Currently, the sample is underpowered to determine the best-fitting empirical model to explain sources of symptoms covariation. However, the patterns of twin pair correlations suggest there is likely to be significant familial aggregation for these items (including craving) that can be explained by varying combinations of genetic and shared environmental risks. Increased samples will enable us to fit better powered biometrical genetical analyses (Neale & Cardon, Reference Neale and Cardon1992) in the near future.
As soon as empirical CUD phenotypes can be derived, these can be used as part tests of genome-wide association to identify QTLs for CUD. For multivariate association, we will also use methods (Medland & Neale, Reference Medland and Neale2010) that allow GWA tests at both the factor level and at the level of the individual DSM-IV/V items while accounting for the covariation among them. Using this approach we can nest tests of association within the multivariate models that best fit the cannabis use and item level abuse and dependence data. In other words, this will permit genome-wide tests of associations based on the best-fitting CUD phenotypes that may include two or more latent phenotypes to best explain the covariation between the DSM-IV item level data. The same approach, which can be applied to the remaining licit and illicit drug data, can also be used to identify pleiotropic effects across drug classes.
Advances that raise our understanding of CUDs and identify QTLs responsible for DUDs will have an important impact on society and public health. There remains a strong empirical need for psychometrically well-defined CUD phenotypes, a comprehensive model to explain the etiology of cannabis initiation and liability to CUDs, as well as association with sufficient resolution to identify the responsible QTLs. The identification of QTL responsible for CU and CUD will begin to fill gaps in our knowledge, open the way to developing better, targeted treatments, and to provide an empirical basis for addressing policy issues and public concerns about the effects of cannabis use.
Acknowledgments
NG was supported by the United States National Institute on Drug Abuse K99R00 award (R00DA023549). IH, DH, and TD were supported by an Australian National Health and Medical Research Council Australia Fellowship awarded to IH (No. 464914). DH was supported by a grant from the NSW Ministry of Health, Mental Health and Drug & Alcohol Office. The authors also acknowledge and thank the following project staff: Soad Hancock as Project Coordinator; David Smyth for IT; Lenore Sullivan as Research Editor; and our research interviewers Pieta-Marie Shertock and Jill Wood. The authors thank the twins and their siblings for their willing cooperation.