There are different models of liaison psychiatry to deal with the high level of mental illness in general hospital populations. 1 It has been reported that about 27% of patients admitted to medical wards have mental illness fulfilling DSM-IV criteria. Reference Silverstone2 It has been recognised that the timing of psychiatric consultations could have an impact on patients’ length of stay in hospitals, Reference Lyons, Hammer, Strain and Fulop3-Reference De Jonge, Huyse, Ruinemans, Stiefel, Lyons and Slaets6 especially if psychosocial assessment is completed in the first 5 days of admission. Reference De Jonge, Latour and Huyse7,Reference Hall and Frankel8 Patients with severe psychiatric symptoms such as suicidal ideation tend to receive more timely consultations, as a result of less obvious psychiatric symptoms being undetected for greater lengths of time Reference Kishi, Meller, Kathol and Swigart9 which leads to poorer outcomes. Reference De Jonge, Latour and Huyse7 Moreover, the presence of physical illness has been found to delay the identification of mental health needs of patients to the extent that they may not even be recognised. Reference Creed, Morgan, Fiddler, Guthrie and House10
Psychological and psychiatric comorbidity has been correlated with longer length of hospital stay, particularly in patients with impaired cognition, delirium, dementia, anxiety and depression. Reference Saravay, Steinberg, Weinschel and Habert11,Reference Aoki, Sato and Hosaka12 However, the detection of these illnesses has been limited in both out-patient and in-patient care. Reference Kessler, Borges and Walters13 In addition, it was found that physicians who recognised mental health difficulties in patients with physical illness hesitated to discharge them, resulting in a prolonged admission. Reference Aoki, Sato and Hosaka12
Patients that frequently attend hospital have been estimated to cost health services approximately £2.3 billion every year. 14,Reference Syed and Congdon15 Research suggests that there are almost half a million frequent attendees in the UK and over a million emergency admissions to hospitals annually. This group of patients often have conditions that could be better managed with more appropriate treatment within the community. 14,Reference Sampson, Blanchard, Jones, Tookman and King16 Reports suggest that staff attitudes towards patients who attend hospital for reasons other than physical health may be negative. Reference Chan and Ovens17 This could be as a result of the difficulties that arise when attempting to address complex needs in an environment better suited for dealing with acute medical illnesses. Reference Chan and Ovens17-Reference Malone19
Although it is estimated that between 2001 and 2013 there will be a 53% increase in the number of people aged over 65 in the UK, 14 two-thirds of hospital beds are occupied by elderly patients. Moreover, patients over 65 years of age admitted to hospital with or acquiring superimposed mental health conditions have poorer outcomes and increased length of hospital stay. 1 The literature suggests that for older adults, delayed discharges and lengthy stays in hospital can result in anxiety related to: their future, low mood, frustration, anger, feeling unsupported, disempowered and feeling passive in the process. Reference Kydd20,Reference Swinkels and Mitchell21 Currently, 750 000 people live with dementia, a disease that carries a massive personal, family, social and economic cost. Reference Saad, Smith and Rochfort22,23 It is estimated that at any time, up to a quarter of older patients in general acute hospitals will have dementia. 1 The Royal College of Psychiatrists 1 reported that being admitted to a general hospital can often have a detrimental effect on the well-being of a person with dementia. The National Dementia Strategy has outlined several key objectives for patients with dementia, including good quality of care within general hospitals. 23
The RAID model
City Hospital, Birmingham, has 600 beds and an accident and emergency (A&E) department that receives over 200 patients per day. The hospital's statistics show that on average there are 4000 admissions every month: 3000 of them are classified as emergency admissions and the rest are elective or day cases.
The Rapid Assessment, Interface and Discharge (RAID) service provides a new model of liaison psychiatry (awarded the Health Service Journal 2010 award for innovation in mental health) in City Hospital that delivers a rapid response, 24-hour, 7 days a week, age-inclusive service and comprehensive range of mental health specialties, including old age, working age, postnatal mental health and substance misuse. The service aims at a 1-hour target for assessing patients who present to A&E and a 24-hour target for assessing patients on all wards, and is available to any patient aged 16 years or over. Additionally, RAID offers brief follow-up clinics within City Hospital. This multi-professional team provides close clinical involvement alongside clinical support and supervision in mental health interventions for general hospital professionals, patients and carers. The RAID team provided formal and informal training to the acute hospital staff. The formal training included 2 days’ training on dementia, depression, delirium and dignity every 3 months as well as a 1-hour weekly session on different mental health issues.
The RAID model was officially launched in December 2009 as a pilot project with extra investment of £0.8 million per year, although it currently receives recurring funding.
Aim
The aim of this study was to evaluate whether the implementation of an integrated rapid response mental health service improves access to psychiatric assessments and services, as well as reducing cost of health service provision in acute hospitals. The study focused on two areas of possible savings: length of hospital stay and readmission rates, including length of survival in the community after discharge. However, we also aimed to calculate savings from older adult services as a separate group.
Method
Data collection
This study used data already held by City Hospital. Participants were identified via a numerical coding system. The study examined two groups of patients. The RAID group consisted of all emergency admissions with a mental health diagnosis (category F, ICD-10 24 ) that were referred to and managed by the RAID team between 1 December 2009 and 31 July 2010, measuring the RAID direct effect. The RAID-influence group consisted of all emergency admissions with a mental health diagnosis (category F, ICD-10) that were not referred to RAID but had a discharge date between 1 December 2009 and 31 July 2010, measuring RAID indirect effect. The RAID-influence group was identified because the RAID team provided training and support to the City Hospital staff that helped to manage these patients during the period of the study. Also, the study included a retrospective control group (pre-RAID), which consisted of all emergency admissions with a mental health diagnosis (category F, ICD-10) that were admitted between 1 December 2008 and 31 July 2009. The study included all patients over the age of 16 who were admitted to City Hospital during the study period and who had a length of hospital stay of more than 1 day.
Statistical assessment
The statistical assessment of the RAID team intervention assumed a latent effect that could not be measured directly but could be inferred from other observed variables as patient length of stay, readmission rates and patient survival post discharge. The model compared the patients with a retrospective control (pre-RAID) from the same hospital, controlling for any other possible latent effects within the hospital, assuming no other major interventions for mental health patients from the previous year except for the introduction of RAID team. This evaluation was focused mainly on measuring the effect of the service on length of stay and readmissions. Patients’ data were added to an analysis database, which was used to match patients across groups. The statistical analysis was conducted mainly using R statistical package version 2.12.2 on Mac (www.r-project.org).
Length of hospital stay
Patient's length of stay indicates the number of days the patient stayed in the hospital. Since the length of stay variable is affected by fixed and random effects, a case-by-case matched control design was necessary to control the underlying confounding sources of variations except for length of stay. Reference Sorensen and Gillman25 Therefore pairs were matched on ‘exact’ and ‘fuzzy’ criteria. Exact rules match gender, Health Resource Group, main diagnosis, age band (16-55, 56-69, 70+ years) and seasonal variability. Fuzzy rules use probabilistic matching to match secondary diagnosis in the RAID/RAID-influence group with the control group (pre-RAID). Reference Winkler26 The cut-off limit for the lowest possible probability is identified within the RAID and RAID-influence group as the lowest possible secondary diagnosis matching probability with a minimum difference in the length of stay. However, with this sort of matching the marginal probability of finding matching patients between the two groups decreases with every criteria being considered
The result of this matching is a smaller subset of RAID v. pre-RAID and another subset of RAID-influence v. pre-RAID. Every pair of sets represents only the RAID latent effect and hence can be used as a direct empirical measure of the savings in the length of stay. As length of stay was found to generally follow a negative binomial distribution Reference Hilbe27 (as shown in Fig. 1), significance of the savings was assessed using parametric Monte Carlo negative binomial likelihood ratios and non-parametric permutation tests using Wilcoxon paired test. The small matched sample was tested for selection bias using a Monte Carlo randomisation approach to calculate the confidence interval of the main population mean.
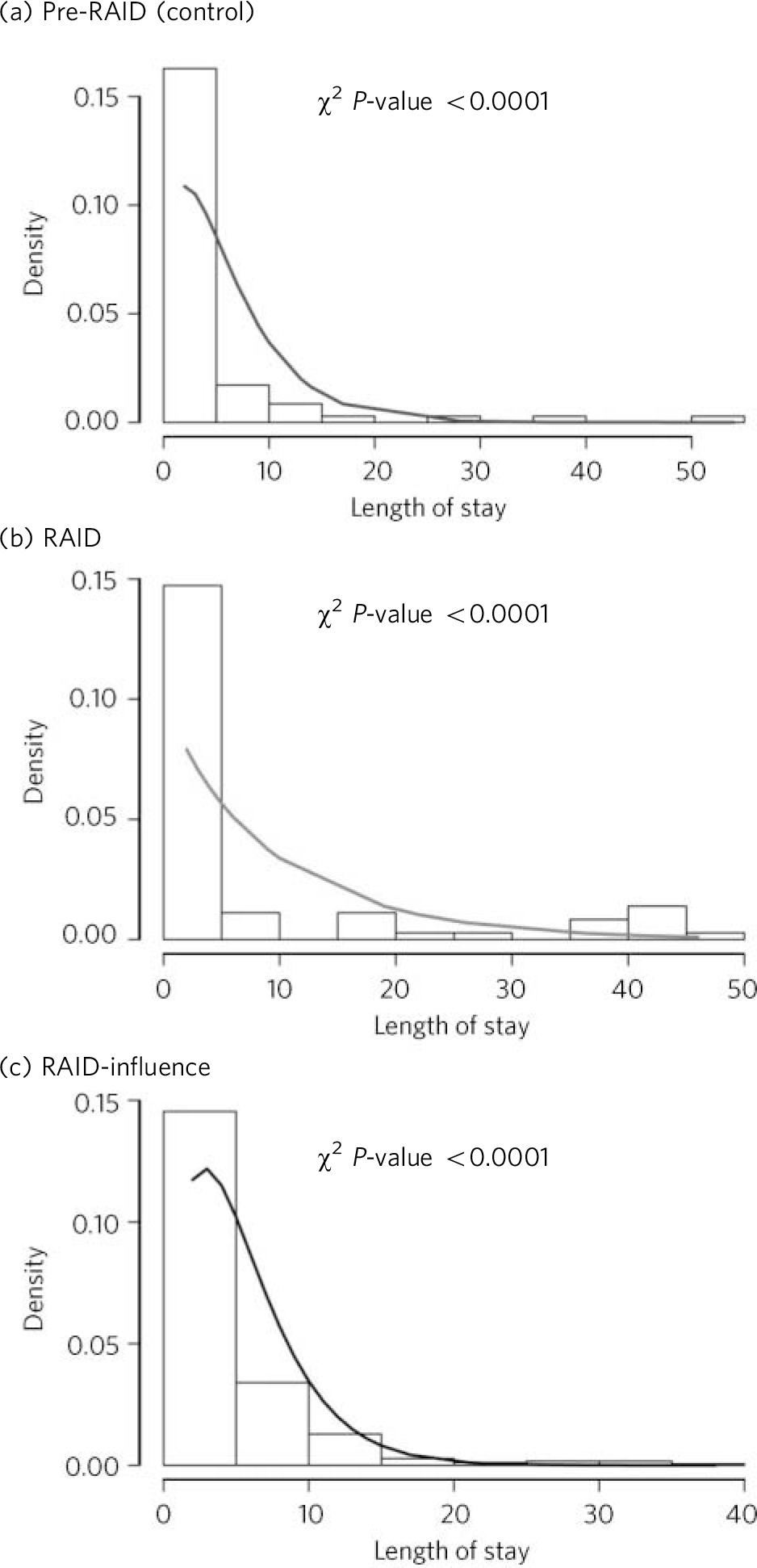
Fig 1 Negative binomial fit of patients’ length of stay across matched samples. RAID, Rapid Assessment, Interface and Discharge.
Discharge effect
The effect of the team to discharge patients was examined based on data measuring the time between the RAID intervention and the patients’ discharge. A major covariate with the patient discharge is the patient's length of stay. To prove the independence between the length of stay and the discharge effect of RAID, the two events were hypothesised to be independent and the Chi-squared test was used to test the null hypothesis of dependence.
Patient survival and readmission
The endurance of all patients in the community after discharge and before another admission was calculated using survival analysis methods Reference Parmar and Machin28 across the RAID, RAID-influence and pre-RAID groups. In the survival analysis, all patients across the three groups were included. The survival of the patient was measured as time to patient readmission. A categorical variable was introduced to identify patient group. A Kaplan-Meier estimate was used to estimate patient survival post discharge. To analyse the effect of any other measured covariate in a multivariate model, we used Cox proportional hazards model to fit the right-censored survival data. The proportionality of the covariates was tested using scaled Schoenfeld residues correlation with the time to readmission. The initial proportional hazards model of patients was fit to age, gender, all admissions during a 3-month period within 1 year, Health Resource Group, main diagnosis and group categorical variable. The final fit model is selected using stepwise selection, where Bayesian Information Criterion (BIC) is used to evaluate step models. We assessed the significance of the categorical variable in the best model using Chi-squared test. The analysis was done using R Stats and Survival library on Mac.
Results
The RAID team received, on average, 300 referrals a month, with 41.2% coming from the A&E department. The mean age of patients referred from A&E was 36.4 years, (95.6% aged 16-64, 4.4% aged 65+). The mean age of patients who were admitted because of self-harm was 34.5 years (95.9% aged 16-64, 4.1% aged 65+). The mean age of referrals from all wards was 65.7 years (40.4% aged 16-64, 59.6% aged 65+). During the period of the evaluation, in total there were 2258 referrals to RAID, including 886 patients who were referred from all the wards.
The RAID group consisted of 886 in-patients (407 male, 479 female), RAID-influence 2654 in-patients (1592 male, 1062 female) and pre-RAID 2873 patients (1686 male, 1187 female) (Table 1). Using paired Wilcoxon test, the P-value between all groups showed non-significant differences based on gender and age. It was noticed that patients in the RAID group had nine ICD-10 diagnostic codes on average (the most complex patients), whereas the RAID-influence group had three ICD-10 diagnostic codes on average.
Table 1 Demographics of the Rapid Assessment, Interface and Discharge (RAID), RAID-influence and pre-RAID groups

RAID, % | RAID-influence, % | Pre-RAID (control), % | |
---|---|---|---|
Male | 46 | 60 | 58 |
Female | 54 | 40 | 41 |
Age Band 1 (16–64 years) | 67 | 69 | 67 |
Age Band 2 (65–74 years) | 10 | 11 | 11 |
Age Band 3 (75+ years) | 23 | 20 | 22 |
RAID response times
Results indicate that 91% of A&E referrals were assessed by RAID within an hour; the average time was 24 min. In total, 89% of ward referrals were assessed within 24 h, with an average of 16 h.
Detection of mental health
Involvement of RAID also led to an increase in the detection and diagnosis of mental illness. The reasons for referral to the RAID team were: self-harm (32%), depression (18%), dementia (18%), alcohol-related problems (13%), psychosis (9%), anxiety (4%), drug misuse (4%) and others (2%). There was a noticeable increase in mental health diagnosis in City Hospital; the biggest increase was seen in the coding of eating disorders (46%), other anxiety disorders (44%), dementia (22%), mental and behavioural disorders due to the use of alcohol (21%), depression and related disorders (17%) and schizophrenia (8%).
Effect of RAID on length of hospital stay
The mean length of stay after matching for RAID group (79 cases) was 9.4 days compared with 10.3 days for the pre-RAID group, and the mean for the RAID-influence group (359 cases) was 5.2 days compared with 8.4 days for the pre-RAID matched group. The RAID-influence group reduction of 3.2 days from the matched pre-RAID group was highly significant (P<0.001), and the RAID group reduction of 0.9 day was of low statistical significance (P = 0.31) (Table 2).
Table 2 Length of stay savings in the RAID, RAID-influence and pre-RAID groups

Average length of stay, days | Saved days per patient | Saved days over 8 months | |
---|---|---|---|
RAID matched cohort | |||
Pre-RAID matched cohortFootnote a | 9.4 | 0.9 | 797 |
RAID-influence matched cohort | |||
Pre-RAID matched cohortFootnote b | 5.2 | 3.2 | 8493 |
RAID, Rapid Assessment, Interface and Discharge.
a. Average length of stay, 10.3 days.
b. Average length of stay, 8.4 days.
Selection bias of the matched groups was examined, as mentioned in the Method. It was found that the mean of 10.3 of the matched sample is within the 95% confidence interval of the population mean (7.5-27.7).
Total savings over 8 months were 9290 bed days in City Hospital. Consequently, estimated savings over 12 months will be 13 935 bed days, which will equate to 38 beds per day. It was also noticed that within the saved 38 beds, 35 beds came out of the geriatric wards. Using the Monte Carlo randomisation approach, we estimated the 95% confidence interval of the mean savings and calculated the minimum savings as 21 beds per day, and the maximum saving was 42 beds per day.
Discharge effect
In total, 75% of patients were discharged within 1 week and 65% were discharged within 3 days of seeing RAID independent of their length of stay. Figure 2 shows the cumulative distribution of the days from RAID referral to the discharge of the patient.
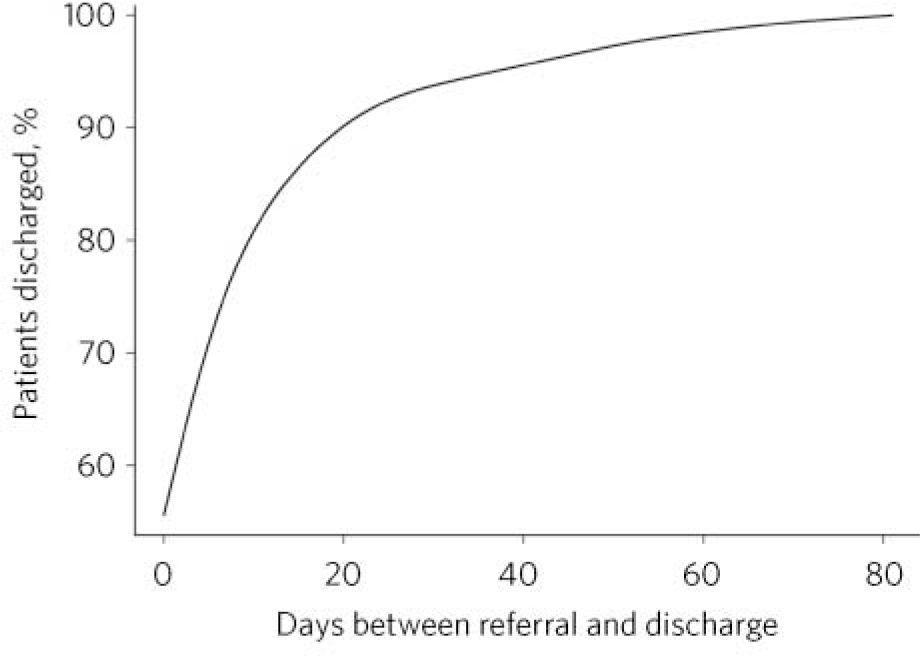
Fig 2 Cumulative distribution of days between RAID referral and discharge.
Patient survival
The readmission of RAID patients v. RAID-influence and pre-RAID patients in the same hospital was estimated using Kaplan-Meier (Fig. 3). Using log rank test, the RAID group had a highly significant patient survival than the control group and the RAID-influence group (P<0.0001), whereas the survival of the RAID-influence group was non-significant v. the pre-RAID group (P = 0.21). The final proportional hazards model post step selection included only the RAID group categorical variable and the main diagnosis, as shown in Table 3.
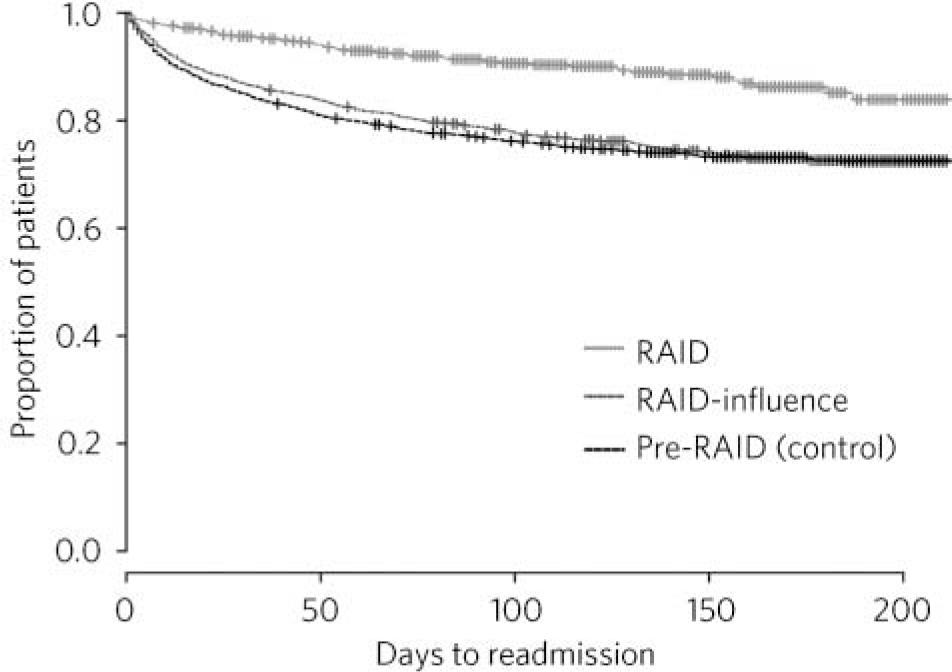
Fig 3 Kaplan-Meier estimate of patients’ readmission for each patient group.
Table 3 Fitted Cox proportional hazards modelFootnote a

RAID categorical variable | Relative risk (95% CI) | P |
---|---|---|
RAID v. pre-RAID/control | ||
RAID | 1.00 | <0.0001 |
Pre-RAID | 2.45 (2.33–2.57) | |
RAID-influence | 2.80 (2.68–2.92) | |
Main diagnosis | 0.99 (0.98–1.00) | <50.0001 |
a. Likelihood ratio test <0.0001.
The hazard ratio calculated for the categorical variable for the RAID-influence v. the RAID group was 2.8 and for the pre-RAID v. RAID group was 2.45. This indicates that the RAID model decreases the hazard of readmission by 60% from the RAID-influence group and by 65% from the pre-RAID group. The RAID group reduced the readmission of patients with all mental illness codes to only 4 readmissions for every 100 patients as opposed to 15 readmissions for every 100 patients for the pre-RAID group, and 12 readmissions for every 100 patients for the RAID-influence group. Calculations for admissions per day that would have potentially occurred prior to the RAID intervention accumulated to 1200 saved admissions over an 8-month period (estimated 1800 saved admissions over 12 months) for both the RAID and RAID-influence group. The average length of hospital stay for this group of readmitted patients was 4.5 days, i.e. the total annual saved bed-days was 8100 and the daily saved bed days was 22 beds. It was observed that 20 out of those 22 beds came out of the geriatric wards.
Discussion
The RAID model evaluation focused on three areas: response time, quality improvement and cost savings. This evaluation showed that the team achieved the rapid response targets for A&E and all wards in the vast majority of cases. The team also improved the detection of mental illness in City Hospital and access to mental health services. Quality improvement is reflected in increasing detection of mental illness as well as reducing length of stay and readmission; patients and providers welcome both. However, measuring cost savings is more challenging because of the complexity and variations of patients’ individual features and the known paucity of clinical information and diagnostic coding concerning retrospective patient groups with mental health issues in general hospitals; hence, the choice of the used methodology rather than simple group comparison.
The RAID model emphasises training for acute hospital staff in assessment, detection and intervention for mental health challenges. Research suggests that education can help to both eliminate discrimination of those with mental health difficulties Reference Mukherjee, Fialho, Wijetunge, Checinski and Surgenor29 and up-skill acute hospital staff. In particular, research suggests that health professionals feel unskilled in dealing with patients with mental health challenges due to a lack of training, and hold stigmatised and negative attitudes towards this patient group. Reference Friedman, Newton, Coggan, Hooley, Patel and Pickard30-Reference Arvaniti, Samakouri, Kalamara, Bochtsou, Bikos and Livaditis32 This is particularly applicable to older persons with mental illnesses, including dementia. 33 This study included a RAID-influence group in order to capture the effects of providing training and support to hospital staff, whereas the RAID group was designed to measure the direct effect of RAID. Despite using a retrospective case-by-case matched control group (pre-RAID) to compare the effect of RAID and RAID-influence groups on length of stay, the small number in the pre-RAID group might have affected the outcome due to missing diagnostic codes in the control group and strict matching criteria. It must be emphasised that there were no major changes in the clinical services, managerial structural or discharge support in City Hospital during the study period. However, we acknowledge that it was not possible to control for all external changes in the community during the time period in which RAID cost savings were examined.
The RAID-influence group had a greater impact than RAID in reducing length of stay but both RAID and RAID-influence groups were more effective than the pre-RAID control group. However, the RAID group included the most complex cases in comparison to the RAID-influence group and suggests that nurses and junior doctors on the wards were able, after receiving training on mental health, to develop clinical skills to decide which patients could be managed by the general hospital staff and who should be referred to specialised psychiatric services. The RAID group was more effective than the RAID-influence group and the pre-RAID group in reducing readmissions through linking patients to the right care pathways into the community. Reducing readmission to general hospital is a new target by the Department of Health, 34 as readmissions often do not lead to any improvement in health outcomes and are sometimes associated with negative attitudes. Reference Chan and Ovens17-Reference Malone19
This evaluation showed that most of the savings through reduced length of stay (35 out of 38 beds), and reducing readmissions (20 out of total of 22 beds) came out of geriatric wards. The hospital was able to close down 60 beds by incorporating the reduction in bed use resulting from RAID. The RAID model savings were reviewed by the London School of Economics (LSE), who chose to adopt a very conservative estimate rather than the central estimate used in this paper, and concluded that the RAID model saved at least 44 beds per day, which correlates with the estimate of £1: £4 as reported by LSE. 35
Conclusions
Patients who are occupying hospital beds waiting to be assessed by psychiatric services is not only an example of financial waste in our health system, but it also increases dissatisfaction by both patients and staff, which in turn leads to poorer outcomes. Mental health issues in acute hospitals have a negative effect on outcome measures for health and economy. The total savings in bed-days reported in this study through reducing length of stay (21-42 beds per day) and readmissions (22 beds per day) is estimated at 43-64 beds per day, which is equivalent to approximately 2-3 wards. The London School of Economics estimated that the team was able to save at least 44 beds per day. Most of the bed savings came from geriatric wards. We estimate the financial savings to be £4-6 million (based only on saved beds), but LSE estimated the saving to be around £3.5 million using a conservative calculation. During the period of the RAID intervention City Hospital managed to close 60 beds without cutting down on services.
We emphasise that rapid response to psychiatric patients in acute hospitals in a comprehensive and integrated model can lead to a reduction in readmissions and length of stay. Reference De Jonge, Huyse, Ruinemans, Stiefel, Lyons and Slaets6 Savings offer shared benefits between acute hospitals and primary care trusts (savings to the total economy, including social care cost, are not calculated in our study but can be viewed in the LSE report 35 ). We argue that improving mental health services in an acute hospital by enhancing the quality of care and increasing efficiency of psychiatric assessments in an integrated approach, can lead to achieving the required savings and improving quality. However, measuring the outcomes of liaison psychiatry remains a great challenge to research projects and service developments.
Acknowledgements
The authors thank all those who helped bring this project off the drawing board and into the wards. The success and achievements of the RAID team would not have been possible without the hard work and dedication of Sue Turner (CEO) who played a pivotal role in the creation and promotion of the model. Thanks also to Kate Phipps, who invested time and expertise in ensuring the flourishing of the team, alongside Dr George Georgiou (Clinical Director), Kerry Webb (Operational Lead), Wayne Harrison (Public Health Consultant), Mike Preece (Team Manager) and every other member of the team.
eLetters
No eLetters have been published for this article.