Background
Schizophrenia is considered as a neurodevelopmental disorder that starts very early. Patients with schizophrenia have structural and functional brain abnormalities that are usually observed in elderly people, and associated with this increased incidence of brain ageing, cognitive decline. A new neuroimaging-based strategy, namely, brain-age prediction, may reveal the relationship between brain structural and functional changes and age. Also, it offers a novel way for searching for brain ageing biomarkers.
Previous work indicates that schizophrenia is associated with accelerated brain ageing when comparing the estimated brain age from brain images with chronological age.Reference Schnack, van Haren, Nieuwenhuis, Hulshoff Pol, Cahn and Kahn1,Reference Nenadic, Dietzek, Langbein, Sauer and Gaser2 The establishment of age prediction models based on brain magnetic resonance imaging (MRI) is a popular trend in neuroscience, which is used to recognise patterns in ageing-associated brain tissue.Reference Schnack, van Haren, Nieuwenhuis, Hulshoff Pol, Cahn and Kahn1,Reference Cole and Franke3,4 Predictive analytical models may be instrumental in increasing our understanding of brain structural and functional changes with age.
The difference between predicted and chronological age could serve as an important biomarker reflecting pathological processes in the brain. A higher brain-predicted age difference (PAD) score indicates an older brain age than chronological age, which might reflect how far a brain deviates from the normal ageing trajectory.Reference Schnack, van Haren, Nieuwenhuis, Hulshoff Pol, Cahn and Kahn1 Exploring this ‘gap’ between predicted and chronological age may help us better understand the mechanisms involved. The scores calculated from patients with schizophrenia and healthy individuals could further be used for regression analysis with clinical symptoms. Increases in brain-PAD indicate that the brain ageing process is accelerated and imply risks for neurodegeneration and other age-related issues, which have been widely revealed in individuals with psychiatric disorders and neurological diseases.Reference Koutsouleris, Davatzikos, Borgwardt, Gaser, Bottlender and Frodl5 The pathophysiology of schizophrenia needs to be elucidated for identification and well-directed interventions within the early-psychosis period. Therefore, we believe that exploring the difference between predicted and chronological age might be conducive to identifying a biomarker for early diagnosis and clinical outcome.
Aims
A series of studies have shown that schizophrenia is associated with accelerated brain ageing as estimated from brain images. However, whether it occurs in people with first-episode schizophrenia needs to be elucidated. Although existing studies have suggested that the age gap may serve as a biomarker for schizophrenia, it remains unclear which factors (for example severity of symptoms, cognitive function, medication) could affect the brain-age gap and what the relationships between the gap and those factors are. Thus, the aim of the current study was to explore these issues by assessing the characteristics of the brain-age difference between patients and healthy controls. With our brain-age prediction model, we not only compared the age gap between patients with schizophrenia and healthy controls, but also investigated the association between the age gap and symptoms and medication. We assumed the deviation from normal ageing may reflect important neurological changes relating to disease and medical treatment.
Method
Participants
The recruitment of first-episode schizophrenia patients could be conducive to minimising the confounding factors of research (e.g., prior therapeutic exposure and the potential influence of chronicity).Reference Cui, Wei, Xi, Griffa, De Lange and Kahn6 We included 60 patients (principal data-set) and 40 patients (replication data-set) with first-episode schizophrenia from the Department of Psychiatry at Xijing Hospital. In addition, 60 (principal data-set) and 40 (replication data-set) age- and gender-matched healthy controls were recruited by advertisement from the local community. The age range of all participants was 18–44 years. There was no history of neurological or psychiatric diseases and no use of medication affecting the central nervous system in the healthy participants.
All patients underwent a careful clinical diagnosis and met the Diagnostic and Statistical Manual of Mental Disorders, Fifth Edition (DSM-5) criteria.7 Only a small number of patients (15 patients in the principal data-set) had been exposed to antipsychotic treatment within 2 weeks, and the rest patients were medication-naïve. Two senior clinical psychiatrists performed clinical psychometric assessments. Patients were assessed with the Positive and Negative Syndrome Scale (PANSS)Reference Kay, Fiszbein and Opler8 on the day of scanning. Of the 60 patients in the principal data-set, 21 medication-naïve patients who were successfully followed up were included in the longitudinal study. All follow-up patients received second-generation antipsychotics, including paliperidone (4 patients), risperidone (10 patients), olanzapine (7 patients), amisulpride (2 patients) and quetiapine (1 patients) (partial patients received multi-therapy). They underwent clinical assessments and MRI scans at a mean of 4.7 months after recruitment. Dose of current antipsychotic medication at the time of MRI scan was converted to defined daily dose.Reference Leucht, Samara, Heres and Davis9
The authors assert that all procedures contributing to this work comply with the ethical standards of the relevant national and institutional committees on human experimentation and with the Helsinki Declaration of 1975, as revised in 2008. All procedures involving human patients were approved by the local Research Ethics Committee (Xijing Hospital, Fourth Military Medical University) with the approval number XJYYLL-2015047. All participants signed informed consent forms after a complete description of this study.
Demographic, clinical measures and neurocognitive assessment
Sociodemographic information was collected for all participants including age, gender and level of education. Symptom severity was characterised by the PANSS.Reference Kay, Fiszbein and Opler8 Cognition was assessed with the digit symbol and digit span test (including forward and backward) from the Wechsler Adult Intelligence Scale revised in China,Reference Gong, Dai, Li, Chen, Li and Jiang10 and semantic (category) fluency from the Beijing version of the Montreal Cognitive Assessment.
MRI image acquisition
In the test sets, diffusion tensor imaging (DTI) was acquired on General Electric Discovery MR750 3.0 T scanner (principal data-set) and on Siemens Magnetom Trio Tim MR 3.0 T scanner (replication data-set). The protocols of the two data-sets have been published. The participants wore a custom-built MRI-compatible head coil fitted with foam pads and earplugs to minimise head motion and dampen scanner noise. The diffusion sensitising gradients were applied along 64 non-parallel directions (b = 1000 s/mm2) and one without diffusion weighting (b = 0). The parameters were as follows.
(a) General Electric MR scanner: repetition time (TR)/echo time (TE), 10 000 ms/84.8 ms; flip angle, 90°; field of view (FOV), 240 mm × 240 mm; matrix, 128 × 128; 2.0 mm effective slice thickness with no gap; 70 slices; scanning time was about 22 min.
(b) Siemens MR scanner: TR/TE, 7000 ms/91 ms; flip angle, 90°; FOV, 256 mm × 256 mm; matrix, 128 × 128; 3.0 mm effective slice thickness with no gap; 50 slices; scanning time was about 8 min.
Image analysis
Raw diffusion tensor scans in the training set were drawn from the publicly accessible IXI cohort (http://brain-development.org/ixi-dataset/), the International Neuroimaging Data Sharing Initiative (INDI) online database (http://fcon_1000.projects.nitrc.org/), Cyclotron Research Centre (University of Liège) (https://www.nitrc.org/projects/parktdi/) and the First Affiliated Hospital of Xi'an Jiaotong University (non-public data).
In total, DTI scans from 523 healthy individuals with age ranging from 11 to 86.2 years were used to build a brain-age prediction model. Briefly, images both in the training set and test sets were preprocessed using the FSL (FMRIB Software Library v6.0, http://www.fmrib.ox.ac.uk/fsl). The preprocessing procedureReference Gan, Shi, Wang, Sun, Yin and Bai11 includes skull and non-brain tissue removal, head motion and eddy current correction. The individual spatial fractional anisotropy (FA) images were calculated by the diffusion tensor model. Then the FA images were registered to the FA template (FMRIB58_FA) using a non-linear registration routine.Reference Gan, Shi, Wang, Sun, Yin and Bai11 Average FA values of 50 white matter regions (i.e. 50 fibres) were extracted according to the rICBM_DTI_81_WMPM_FMRIB58 1 mm atlas.
Machine-learning brain-age estimation
Relevance vector regression (RVR)Reference Gan, Shi, Wang, Sun, Yin and Bai11 was introduced for model training of the relationship between FA values and chronological age in the training set, using the Scikit-learn Python library. In the feature selection, Pearson correlation (r) between the mean FA value of each fibre and the chronological age was calculated. The fibres survived when they were correlated with the chronological age (|r| > 0.25, P < 0.001). Then principal component analysis (PCA) was conducted to reduce the dimension, and then the top 90% of variance was taken as the input for the brain-age prediction model. The PCA components were registered into high-dimensional feature space by using the linear kernel function, obtaining the feature vector. Maximum likelihood estimation was used to estimate the weight in the regression model through the type-II maximum likelihood algorithm.
To avoid the possibility of overfitting, we used automatic correlation determination to converge the weights of some eigenvectors to zero. Relevance vectors were those feature vectors with non-zero weights. To assess the ability of the proposed regression model, we conducted internal validation in both data-sets. Tenfold cross-validation on the training set (i.e. 523 healthy individuals) was performed, with onefold in each iteration considered as the test set and the remaining folds considered as the training set. The final brain-age prediction model was determined by the relevant vectors and their corresponding weights. The Pearson correlation (r) between chronological age and predicted brain age, the proportion of variation (R 2), the mean absolute error (MAE), and root mean squared error (RMSE) of the brain-PAD scores were measured as the model performance. Based on the age-predicted model, we calculated each participant's brain-PAD in test sets (brain-PAD = predicted age − chronological age) (Fig. 1).
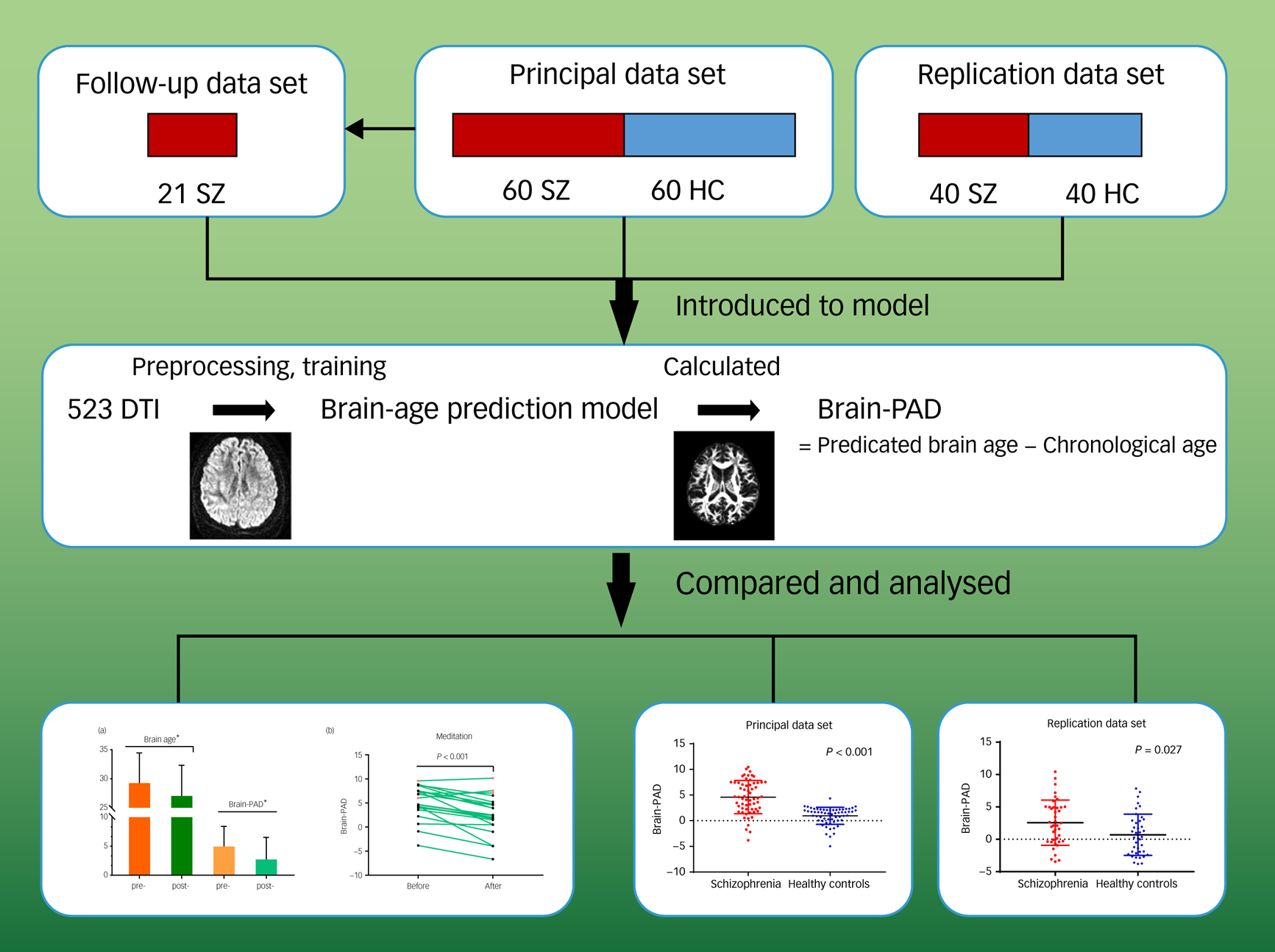
Fig. 1 Pipeline of analysis. Overview of data-sets, prediction model establishment, brain-predicted age difference (brain-PAD) calculation, and statistical analysis.
The images in the final row are shown full size in Figs 2 and 3. DTI, diffusion tensor imaging; HC, healthy controls; SZ, patients with schizophrenia.
Statistical analysis
Brain-PAD scores were statistically analysed in SPSS software (version 25.0) using the analysis of paired sample t-test (normal distribution) or Wilcoxon signed-rank test (non-normal distribution) to compare the differences between patients with schizophrenia and the healthy controls in the principal and replication data-sets. Pearson correlation, Spearman correlation and partial correlation analysis were used to examine the association between brain-PAD and clinical characteristics, such as PANSS scores and cognitive function. The chi-square test was conducted on the difference by gender between groups. Significance was set at P value < 0.05, with statistical rigidity tested by using the replication data-set. Finally, the paired t-test was used for observing the impact of early medication on brain age and clinical variables, and the differences compared with healthy controls. Between-group comparisons of brain-PAD scores for the schizophrenia group and healthy controls were conducted using ANCOVA. Multiple comparisons were corrected using the false discovery rate (FDR) method with a significance threshold of P < 0.05. Using linear mixed-effects model analysis to rule out the potential impact of illness duration on the comparison of brain age at baseline and after medication.
Results
Clinical characteristics of participants
Sociodemographic, clinical and cognitive characteristics of the patients and healthy controls are shown in Table 1. Except for education level in the principal data-set, there were no significant differences between the schizophrenia group and healthy controls for age, gender and education level.
Table 1 Demographic and clinical characteristics of the patients with schizophrenia and healthy controls
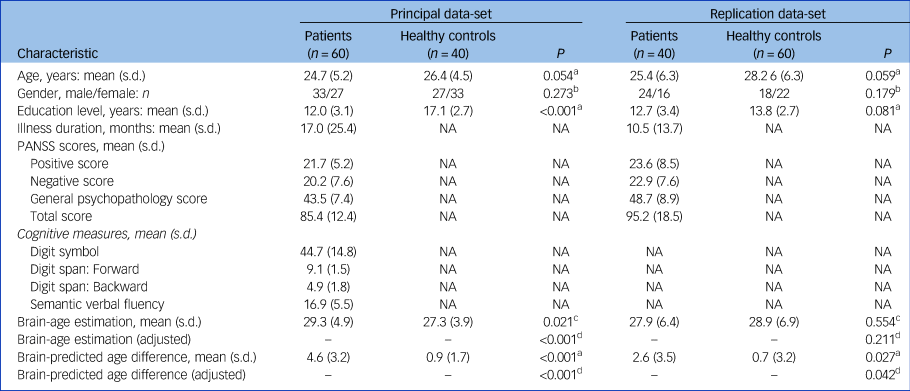
NA, not applicable; PANSS, the Positive and Negative Syndrome Scale.
a. Paired sample t-test.
b. Chi-square test.
c. Wilcoxon signed-rank test was performed owing to the non-normal distribution of samples.
d. ANCOVA, adjusting for education level, age.
Brain-age prediction
The accuracy of the model was assessed via the FA values (r = 0.96, R 2 = 0.93, MAE = 3.74, RMSE = 5.03) from the training set. The predicted ages of participants were highly correlated with their chronological age in the whole group (P < 0.001). In the principal data-set, the mean brain-PAD values in the schizophrenia group was 4.6 (s.d. = 3.2) years, whereas healthy controls showed a difference of only 0.9 (s.d. = 1.7) years. In the replication data-set, the mean brain-PAD value in the schizophrenia group and healthy controls were 2.6 (s.d. = 3.5) years and 0.7 (s.d. = 3.2) years, respectively (Table 1). The differences between the two patient groups were also compared (Supplementary Table 1 available at https://doi.org/10.1192/bjp.2021.169). The schizophrenia groups had significantly higher brain-PAD values than the healthy control groups in both data-sets (t = 7.933, P < 0.001; t = 2.295, P = 0.027) (Fig. 2); the difference in brain-age estimation was higher, with statistical significance, in the principal data-set (Z = −2.312, P = 0.021). After adjusting for education level and age, the differences still existed (Table 1).
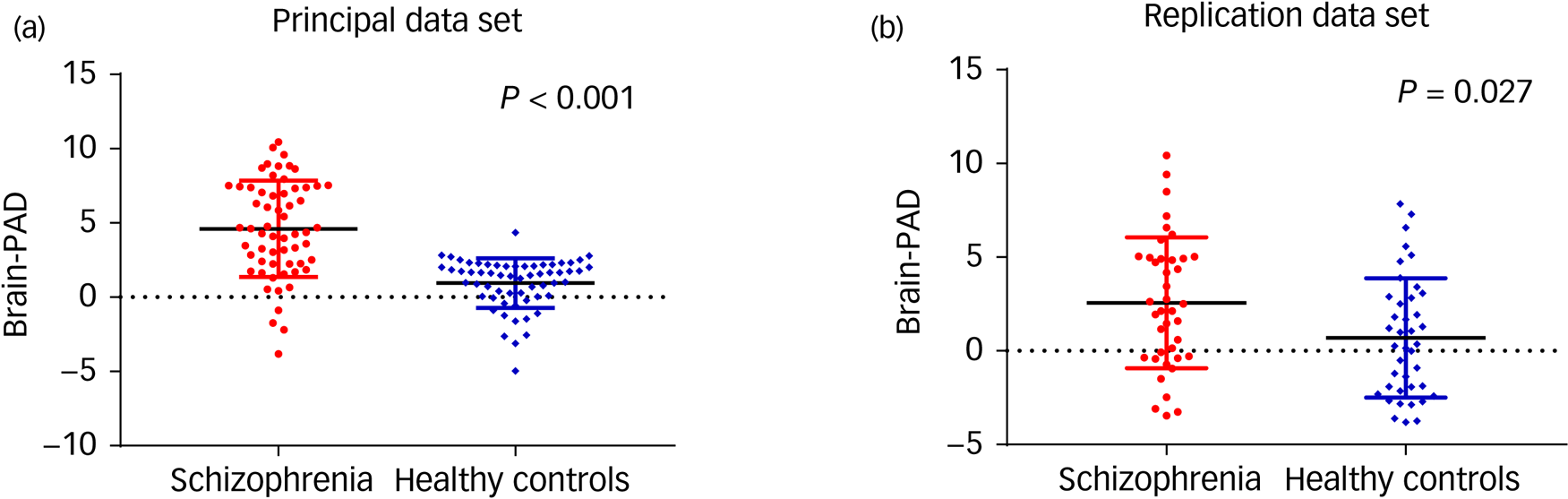
Fig. 2 Brain-predicted age difference (brain-PAD) in the patients with schizophrenia and healthy controls. Scatter plot of brain-PAD scores in the principal data-set (a) and replication data-set (b).
The brain-PAD was higher in patients with schizophrenia than in the healthy controls with P < 0.001 (a) and P = 0.027 (b). Solid black lines indicate group mean values of brain-PAD. The dotted lines refer to the brain-PAD of 0.
Changes with early medication
After early medication (4.743 months, s.d. = 1.535), 21 patients in the principal data-set had a significant reduction in brain-PAD compared with their baseline state (2.718 (s.d. = 3.818) v. 4.932 3 (s.d. = 3.486), t = 5.495, P < 0.001) (Fig. 3). Their clinical characteristics are shown in Table 2. The post-PANSS scores was significantly lower compared with pre-PANSS scores (P < 0.001), whereas early medication had no effect on the changes in cognitive measures (P > 0.05). In addition, the correlations were not statistically significant between the changes in brain-PAD and chronological age (r = 0.106, P = 0.676) and education level (r = 0.096, P = 0.705) (Supplementary Table 2). When ruled out the effect of illness duration, the difference of PAD before and after medication still existed (P = 0.165). In other words, the illness duration was not a factor affecting the changes in PAD after medication.

Fig. 3 (a) Brain age and brain-predicted age difference (PAD) were reduced after early medication with a statistical significance. (b) Changes in brain-PAD in 21 patients at baseline and follow-up.
Error bars represent standard deviations and asterisk indicates significance (P < 0.001). In (a), pre- represents before medication baseline, post- represents after medication. In (b), the line between the black dots represents the decrease in PAD after treatment, and the line between the red dots represents the increase in PAD after treatment.
Table 2 Clinical characteristics of 21 patients with first-episode schizophrenia before and after treatment
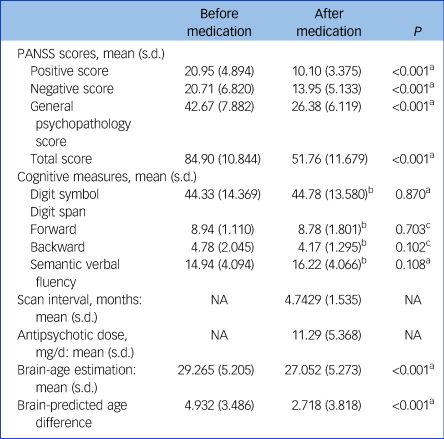
NA, not applicable; PANSS, the Positive and Negative Syndrome Scale.
a. Paired sample t-test.
b. Information available for 18 participants.
c. Wilcoxon signed-rank test was performed due to the non-normal distribution of samples.
Moreover, when comparing the follow-up patients with 21 healthy controls, there was no significant difference between the patients with schizophrenia and the healthy controls in chronological age (P = 0.300), gender (P = 0.757) but a significant difference in education (P < 0.001). Comparing the follow-up patients after medication for about 4 months with healthy controls, there were significantly differences in brain-PAD (F = 4.212, P = 0.047, adjusted), but not in the brain-age estimation (Supplementary Table 3).
Features associated with brain-PAD scores
In the model training, the r value between chronological age of healthy people and FA of white matter was calculated to evaluate the contribution of related white matter tracts to brain age. A total of 33 white matter tracts were selected and defined as features. The FA values in 31 white matter tracts (except for the right anterior corona radiata and the left fornix, Supplementary Table 4) that related to the brain-PAD scores, showed significant statistical differences between the two measurements (P < 0.05, FDR corrected).
Parameters affecting the brain-PAD
As for the parameters affecting the brain-PAD, correlation analysis was undertaken for chronological age, gender, educational level and illness duration (Supplementary Table 2). The brain-PAD value was negatively associated with the chronological age of the patients in the principal and pooled data-sets (P < 0.05), but not in the replication data-set. There were statistically significant correlations between brain-PAD and gender in the patient groups of the principal and pooled data-sets (P = 0.048 and P = 0.003). The brain-PAD were negatively associated with education level and illness duration in the replication patient group (P = 0.027 and P = 0.025).
Correlation with clinical measurements of patients with schizophrenia
As was shown in Supplementary Table 5, the brain-PAD value was negatively associated with the positive score in the principal data-set (r = −0.326, P = 0.014), whereas no statistically significant association was found in other symptoms of the PANSS assessment. In addition, there was a negative correlation between brain-PAD and semantic verbal fluency after medication for about 4 months (r = −0.632, P = 0.015). Nevertheless, correlation analysis showed that performances on cognitive measures (raw scores) were irrelevant to brain-PAD scores in patients with schizophrenia, including digit symbols, and forward and backward digit span. Moreover, the correlations were not statistically significant between the changes in brain-PAD and current antipsychotic dose and scan interval.
Discussion
Main findings
In this study, we aimed to confirm that predicted brain age extracted from structural imaging can be used as a biomarker for schizophrenia diagnosis and medication effect. We performed neuroimaging-based brain-age prediction in people with schizophrenia and healthy controls to assess whether there was accelerated brain ageing in patients with first-episode schizophrenia, the correlation between PAD and clinical characteristics, and the effect of medication on brain age estimation. Based on the advantage of MRI scans, the prediction model was highly accurate at estimating brain age in a relatively large cohort from the perspective of white matter structure. Brain age was estimated to be older than the patient's chronological age, which was verified in a replication data-set. The brain age of patients with schizophrenia might be altered by medication as we found that features (i.e. white matter fibres) have been significantly improved after treatment, namely, the degree of brain ageing assessed by white matter fiber characteristics was lightened.
Our study partially consolidated and extended previous findings showing the accelerated brain ageing in patients with schizophrenia, and further underscored that this feature may be the result of the disease itself. In addition, although accelerated ageing in schizophrenia has been reported in previous studies,Reference Nenadic, Dietzek, Langbein, Sauer and Gaser2,Reference Tonnesen, Kaufmann, de Lange, Richard, Doan and Alnaes4,Reference Sheffield, Rogers, Blackford, Heckers and Woodward12,Reference Shahab, Mulsant, Levesque, Calarco, Nazeri and Wheeler13 they did not focus on first-episode schizophrenia, where the brain ageing could be affected by illness duration and therapy.
Interpretation of our findings and comparison with findings from other studies
In the schizophrenia group the difference in the estimated brain age and their chronological age appeared to be 4.6 years older in the principal data-set and 2.6 years older in the replication data-set, which represented statistically significant differences compared with healthy controls. In the follow-up data-set, we found an average difference of 4.9 years between brain and chronological age at baseline, but only 2.7 years older after early medication. However, Schnack and colleagues reported that the brain age of patients with schizophrenia was progressively increased at a rate of about 1.24 years annually over the 3.5-year follow-up period.Reference Schnack, van Haren, Nieuwenhuis, Hulshoff Pol, Cahn and Kahn1 The difference between our findings and Schnack et al may be the result of dynamic changes with clinical course and long-term therapy, and further evidence is needed to verify the findings. Moreover, previous studies have verified a trend of accelerated brain ageing similar to those in schizophrenia in other psychiatric disorders.
A comparison of people with schizophrenia with people with bipolar disorder and healthy controls showed that the gap was more prominent in schizophrenia, and was found to a lesser extent in bipolar disorder.Reference Nenadic, Dietzek, Langbein, Sauer and Gaser2,Reference Shahab, Mulsant, Levesque, Calarco, Nazeri and Wheeler13 In a broader study using cross-diagnostic quantitative analysis, the results suggested that accelerated brain ageing might have a particular impact in schizophrenia with an increase of 5.5 years, which could also extend to major depression, borderline personality disorder and individuals with at-risk mental states.Reference Koutsouleris, Davatzikos, Borgwardt, Gaser, Bottlender and Frodl5 In brief, the gap between brain age and the chronological age of patients with schizophrenia was proved to make sense, and may represent an intrinsic feature of schizophrenia.
Combined predictors (such as brain atrophy, iron deposition and alterations of white matter microstructure) have great potential in capturing the various facets of brain ageing.Reference Cole and Franke3 As some researchers pointed out, schizophrenia is a progressive process with progressive brain tissue loss.Reference Schnack, van Haren, Nieuwenhuis, Hulshoff Pol, Cahn and Kahn1 Neuroimaging techniques have shown structuralReference Nenadic, Dietzek, Langbein, Sauer and Gaser2 and functional brain abnormalitiesReference Sheffield, Rogers, Blackford, Heckers and Woodward12,Reference Sheffield, Repovs, Harms, Carter, Gold and MacDonald14 in patients with schizophrenia, and ventricular enlargement and cortical atrophy have also proved to be progressive, which suggests the existence of brain atrophy. Ageing of the brain involves the shrinkage of the cortex and grey matter structures and loss of white matter. In the brain, the alterations found in schizophrenia are to some extent similar to those observed in healthy ageing, namely, atrophy is also observed in the schizophrenia brain. The current study revealed the existence of brain ageing in schizophrenia using neuroimaging; the DTI MRI sequence in patients with schizophrenia showed the relationship between brain ageing and characteristics of white matter, which was consistent with the results found of structural changes to white matter in our prior study.Reference Cui, Wei, Xi, Griffa, De Lange and Kahn6
Regarding brain-age prediction models, T 1-weighted MRI is commonly used for morphometric measures, such as regional cortical thickness, surface area and volume. Several studies have shown the robust prediction performance of schizophrenia using support vector machines,Reference Lancaster, Lorenz, Leech and Cole15 Gaussian Process regression,Reference Cole, Ritchie, Bastin, Valdes Hernandez, Munoz Maniega and Royle16 relevance vector machineReference Franke, Ziegler, Kloppel and Gaser17 and deep-learning approachesReference Jonsson, Bjornsdottir, Thorgeirsson, Ellingsen, Walters and Gudbjartsson18 with T 1-weighted MRI images. T 1-weighted and DTI sequences can describe distinct tissue classes, although both of them have the ability to assess and quantify white matter.Reference Richard, Kolskar, Sanders, Kaufmann, Petersen and Doan19 One hypothesis of accelerated brain ageing that has been reported in schizophrenia is disruption to white matter integrity.Reference Sheffield, Repovs, Harms, Carter, Gold and MacDonald14,Reference Mamah, Ji, Rutlin and Shimony20 Focusing on exploring the methodology of a brain-age model, Tonnesen et al indicated that brain-age prediction based on DTI providesReference Tonnesen, Kaufmann, de Lange, Richard, Doan and Alnaes4 the clinical application research with strong supporting evidence to study white matter abnormalities in patients of schizophrenia. In this study, we found that the FA values of most white matter fibre tracts improved after medication, also demonstrating the potential significance of white matter connection disruption in schizophrenia.
We used RVR with DTI sequences to establish the prediction model as DTI has an advantage in estimating white matter microstructures across the brain.Reference Richard, Kolskar, Sanders, Kaufmann, Petersen and Doan19 Compared with other parameters related to DTI, the brain-age prediction model based on FA showed higher sensitivity than mean diffusivity, radial diffusivity, or axial diffusivity.Reference Tonnesen, Kaufmann, de Lange, Richard, Doan and Alnaes4 To improve the accuracy of prediction, some investigators have combined DTI with T 1-weighted sequences to compare tissue-specific brain ages and their cognitive sensitivity in healthy controlsReference Richard, Kolskar, Sanders, Kaufmann, Petersen and Doan19 and delineated the trajectories of brain development in teenagers without mental disorders.Reference Erus, Battapady, Satterthwaite, Hakonarson, Gur and Davatzikos21 In the future, establishing a brain-age prediction model by integrating multiple MRI sequences from both functional and structural aspects of patients with schizophrenia will come closer to being realised.
Impaired white matter bundle connectivity between the brain regions plays a critical role in the neuropathology of schizophrenia.Reference Xiang, Wang, Lei, Li, Li and Zhao22 In this study, we did not find consistent significant factors that might influence the white matter connectivity in patients with schizophrenia, such as age, gender, education level, illness duration or symptoms. There was a trend to an increase in brain-PAD with younger age, which did not meet statistical significance in the replication data-set. It has been reported that psychiatric patients who are younger show more severe accelerated brain ageing, which indicates that abnormal white matter integrity may occur at a younger age rather than there being a progressive development with age.Reference Masuda, Okada, Takamura, Shibasaki, Yoshino and Yokoyama23,Reference Xiao, Sun, Shi, Jiang, Tao and Zhao24 In other words, early-onset schizophrenia is more symptomatic and has a worse prognosis. Therefore, quantitative studies based on brain age might be conducive in revealing the pathological mechanism involved. There was significant correlation between education level and brain-PAD in our replication patient group, but not in principal patient group and pooled group. A large cohort in a healthy participant brain-age study also reported that brain-PAD was not associated with years of education.Reference Cole, Ritchie, Bastin, Valdes Hernandez, Munoz Maniega and Royle16 Therefore, the effect of education on brain age needs to be further explored.
Cognitive impairments are commonly seen in individuals with schizophrenia, which might be in line with accelerated or premature ageing.Reference Harvey and Rosenthal25 The discrepancy between brain age and chronological age might have potential correlation with cognitive impairments. There may be no correlations between the discrepancy and some aspects of cognition impairments,Reference Jonsson, Bjornsdottir, Thorgeirsson, Ellingsen, Walters and Gudbjartsson18 or the correlation was negative, which indicated worse performance with higher brain age difference.Reference Richard, Kolskar, Sanders, Kaufmann, Petersen and Doan19 In the present study, we observed negative correlations between brain-PAD scores and semantic verbal fluency after medication, as well as the scores on the positive scale of the PANSS in the principal data-set. It has been reported that the FA value of white matter fibres are negatively associated with total PANSS scores in a never-treated schizophrenia group.Reference Xiao, Sun, Shi, Jiang, Tao and Zhao24 Nevertheless, no consistent results were found in replication group and pooled group of our study. Overall, patients with schizophrenia might have accelerated brain age and cognitive impairments that exist from an early stage of their illness and then follow a normal rate of age-related changes, which needs to be validated in larger longitudinal studies.
It was reported that antipsychotic treatment may confer benefits on white matter tracts in patient with schizophrenia.Reference Xiao, Sun, Shi, Jiang, Tao and Zhao24 In the longitudinal part of our study, we confirmed the effect of early medication on brain-age estimation, PANSS scores and cognitive measurements. The average predicted brain-age gap between baseline and follow-up was reduced by 2.2 years. The PANSS score, which indicates the severity of symptoms, had significantly reduced compared with the baseline assessment. Evidence showed that the brain-age acceleration rate was positively with the PANSS total score at follow-up and cumulative antipsychotic intake, while these were inconsistent with our findings.Reference Schnack, van Haren, Nieuwenhuis, Hulshoff Pol, Cahn and Kahn1 The differences found in brain age were not related to the current antipsychotic dose in our research. Furthermore, the significant changes in cognitive function were not found, which may be owing to the relatively slight effect of antipsychotics on cognitive function. Cognitive performance seems to be stable over the lifetime in most patients with schizophrenia, and accelerated ageing may occur very early in the illness.Reference Harvey and Rosenthal25 The results were coherent with that the early courses of psychiatric disorders are accompanied by accelerated ageing, and meanwhile, the cognitive function might be intact.Reference Sheffield, Rogers, Blackford, Heckers and Woodward12 That is to say, there is accelerated ageing in schizophrenia and the cognitive function of patients may not be related to this accelerated ageing. This study demonstrates that the estimated brain age of follow-up patients with first-episode schizophrenia became younger after early medication for about 4 months. Antipsychotic treatment might be beneficial to the integrity of white matter tracts. Further research is needed to confirm this effect and explore the neurobiological mechanism of antipsychotics on brain age in schizophrenia.
Limitations
The present findings should be considered with several limitations. First, the sample size is limited, and only a small number of participants were available for the follow-up data-set and this is likely to have limited statistical power. Second, cognitive measurements were not comprehensive and were only applied in patients in the principal data-set. Third, although we assessed whether the discrepancy of brain-PAD was associated with current medication exposure, it would be more precise to consider cumulative exposure to antipsychotics. Fourth, follow-up patients did not receive the same antipsychotics, which might affect the results. Fifth, the brain-age prediction model only used DTI MRI sequences. As a result, large collaborative longitudinal multimodal imaging studies of schizophrenia will be required to clarify the neurodevelopment trajectory and specific medication influences.
Implications
In conclusion, we found accelerated brain ageing in patients with schizophrenia and we also found that early medication had an effect on ameliorating it. In other words, our research supports the theory of accelerated ageing in patients with schizophrenia from the perspective of brain structural changes. Quantification of age discrepancy could be useful for screening for schizophrenia and searching for new targeted therapies. Given these findings, measuring brain ageing based on neuroimaging holds promise for establishing biomarkers that could contribute to clinical decision-making of psychiatric disorders.
Supplementary material
To view supplementary material for this article, please visit https://doi.org/10.1192/bjp.2021.169.
Data availability
The data that support the findings of this study are available from the corresponding author, H.Y., upon reasonable request.
Acknowledgements
We would like to acknowledge, with special thanks, Dr Jin-bo Sun at School of Life Sciences and Technology, Xidian University, for his help with this work. The authors would like to thank the people living with schizophrenia and the healthy controls who participated in this study for giving up so much of their time.
Author contributions
Y.-B.X. formulated the research questions, contributed to the design of the study, and supervised data collection and analysis. X.-S.W. ran the statistical analysis and edited the manuscript. L.-B.C. supervised the data collection, modified the original manuscript. L.-J.B. formulated the research questions, supervised data analysis. S.-Q.G. conducted the preliminary analysis and contributed the method of the manuscript. X.-Y.J. processed the data of the participants and conducted the preliminary analysis. X.L. supervised the data analysis and revised the final manuscript. Y.-Q.X. collected the data of the participants and analysed the data. X.-W.K. supervised the design of the study and modified the manuscript. F.G. supervised the design of the study and contributed to an early version of the manuscript. H.Y. formulated the research questions, supervised the design of the study and edited the final manuscript.
Funding
This work was supported by the National Natural Science Foundation of China (Y.-B.X., Grant number 81601474) (L.-B.C., Grant number 81801675) (L.-J.B., Grant number 81771914 and 82071993), the Youth Talent Lifting Project of Shaanxi province (F.G., Grant number 20210304), Fourth Military Medical University (L.-B.C., Grant number, 2019CYJH), Project funded by China Postdoctoral Science Foundation (L.-B.C., Grant number 2019TQ0130).
Declaration of interest
None
eLetters
No eLetters have been published for this article.