Background
As one of the leading causes of death worldwide, suicide accounts for nearly one million deaths each year. Suicide attempts are more frequent still.Reference Crosby, Han, Ortega, Parks and Gfroerer1, 2 The scope and gravity of this public health problem has prompted substantial increases in research. Despite this, suicide rates have not abated.3
Effective prevention rests on accurate prediction. Accordingly, identifying the risk factors for suicidal thoughts and behaviours has been a major focus of suicide research. Risk factors are longitudinal predictors of an outcome that divide a population into low- and high-risk groups. These are distinct from correlates, which are associated with an outcome but lack evidence of temporal precedence; as such, correlates are not very useful in prediction efforts.Reference Kraemer, Kazdin, Offord, Kessler, Jensen and Kupfer4 Although cross-sectional studies can identify correlates, establishing risk factors requires longitudinal designs.
Unipolar depression and hopelessness are among the most commonly cited risk factors for suicidal thoughts and behaviours. As evidence of this, depression and hopelessness are consistently featured within risk-factor guidelines developed by major national and international organisations and commonly integrated into structured suicide-risk assessments. In fact, the US Preventive Task ForceReference LeFevre5 recommendations suggest that suicide-risk assessments only be conducted in the context of depression screenings. Supporting these practices, a number of prominent suicide theories incorporate hopelessness and depression as either necessary (for example Interpersonal–Psychological Theory of SuicideReference Joiner6, Reference Van Orden, Witte, Cukrowicz, Braithwaite, Selby and Joiner7) or sufficient (for example Hopelessness Theory of SuicideReference Beck, Kovacs and Weissman8, Reference Abramson, Alloy, Hogan, Whitehouse, Gibb, Hankin, Joiner and Rudd9) causes of suicidal thoughts and behaviours. Moreover, suicidal thoughts and behaviours are symptoms of a depressive episode,10 large epidemiological studies have linked non-fatal suicide attempts with the presence of a mood disorderReference Nock, Hwang, Sampson and Kessler11 and psychological autopsy studies have repeatedly reported depression as the most common mental illness among suicide decedents.Reference Conwell, Duberstein, Cox, Herrmann, Forbes and Caine12, Reference Harwood, Hawton, Hope and Jacoby13
Consistent with theories and practice recommendations, many prospective studies have found that depression and hopelessness do confer substantial risk for later suicide ideation,Reference Sareen, Cox, Afifi, de Graaf, Asmundson and ten Have14, Reference Miranda, Gallagher, Bauchner, Vaysman and Marroquín15 attemptsReference Klein, Schwartz, Rose and Leader16, Reference O'Connor, Smyth, Ferguson, Ryan and Williams17 and death,Reference O'Connor, Smyth, Ferguson, Ryan and Williams17, Reference Kuo, Tsai, Lo, Wang and Chen18 with some studies even reporting 20- to 30-fold increases in risk (for example Beck et al Reference Beck, Steer, Kovacs and Garrison19 Juon et al Reference Juon and Ensminger20). Yet, many other studies report substantially weakerReference Holma, Haukka, Suominen, Valtonen, Mantere and Melartin21–Reference Ribeiro, Pease, Gutierrez, Silva, Bernert and Rudd23 or even non-significant effects.Reference Nock and Banaji24–Reference Sher, Carballo, Grunebaum, Burke, Zalsman and Huang27 These inconsistencies raise questions about whether depression and hopelessness indeed confer risk for future suicidal thoughts and behaviours, and, if so, to what degree.
Objectives
The objective of this study is to address these questions. To this end, we conducted a quantitative meta-analysis of all longitudinal studies examining the prospective effects of depression and hopelessness on suicide ideation, attempts and death. We address four aims. First, we provide a descriptive summary of the existing longitudinal literature. Second, we examine the predictive power of depression and hopelessness as risk factors for future suicide ideation, attempts and death. Third, we evaluate the potential moderating effects of sample severity, sample age and study follow-up length, as variability in these methodological factors may influence the effects of depression and hopelessness. Fourth, we consider the clinical utility of these results by examining effects in terms of the absolute risk of our outcomes (i.e. suicide ideation, attempt and death). Beyond depression per se, a large body of literature also exists exploring the effects of factors commonly studied in the context of depression (such as social isolation, rumination) on suicidal thoughts and behaviours; although slightly outside the scope of the present investigation, we include analyses examining the effects of these factors within the supplementary material (See Supplementary Table 1, available at https://doi.org/10.1192/bjp.2018.27).
Of note, the present study represents an extension and specification of a larger meta-analysis conducted by Franklin et al,Reference Franklin, Ribeiro, Fox, Bentley, Kleiman and Huang28 which examined broad trends in suicide prediction since the inception of suicide research. The approach applied by Franklin and colleaguesReference Franklin, Ribeiro, Fox, Bentley, Kleiman and Huang28 was too coarse to speak to the effects of specific constructs or potentially important moderation effects for specific constructs. As depression and hopelessness have played pivotal roles in suicide research as well as clinical policy and practice, it is prudent to further investigate the effects of these constructs and the potential moderators of their effects. One possibility is that, consistent with Franklin et al,Reference Franklin, Ribeiro, Fox, Bentley, Kleiman and Huang28 analyses reveal weak effects of depression and hopelessness. An alternate possibility, however, is that results of finer-grained analyses indicate stronger effects of these constructs, which would be more consistent with existing theories, policies and practice. This may be possible particularly in the context of moderation analyses. Results would stand to have important implications moving forward, and these implications may diverge from those delineated by Franklin et al.Reference Franklin, Ribeiro, Fox, Bentley, Kleiman and Huang28 As such, the present study was designed to address this existing gap in knowledge.
Method
Sources and searches
We conducted literature searches using PubMed, PsycINFO and Google Scholar up to 31 December 2014. As search terms, we used variants of the terms longitudinal (for example longitudinal, predicts, prediction, prospective, future, later) and suicide (for example suicide, suicidality, suicidal behavior, suicidal, suicide death, suicide plan, suicide thoughts, suicide ideation, suicide gesture, suicide threat, non-suicidal self-injury, self-injury, self-harm, self-mutilation, deliberate self-harm, self-cutting, cutting, self-burning, self-poisoning). We also searched the reference sections of all papers identified through database searches.
Study selection, inclusion criteria
Inclusion criteria required that papers include at least one longitudinal analysis predicting suicide ideation, attempts or death using any variable related to depression or hopelessness. Papers were required to be peer-reviewed publications published in English. We elected to use only published studies as we wanted to summarise the literature used to inform research, policy and practice and relying only on published studies provides some safeguards for study quality. Also, unpublished studies located could fail to be a complete or representative sample of unpublished data, which can in turn bias results. Papers were excluded if:
(a) no longitudinal analyses were reported
(b) no analyses examined the discrete effects on suicide ideation, attempts or death (as we were interested in understanding the specificity of effects on discrete suicide-relevant outcomes, outcomes that combined suicidal thoughts and behaviours and/or were not specific to suicidal thoughts or behaviours were not considered as outcomes);
(c) analyses were conducted within a primary treatment study.
No other criteria were used to exclude studies. Our initial searches yielded 2541 peer-reviewed publications. Based on abstracts, 2372 did not meet inclusion criteria. The remaining articles were read in full; 166 studies were retained (see Fig. 1 for PRISMA flow chart and Supplementary Data 1 for the references for the studies included in the meta-analysis.).
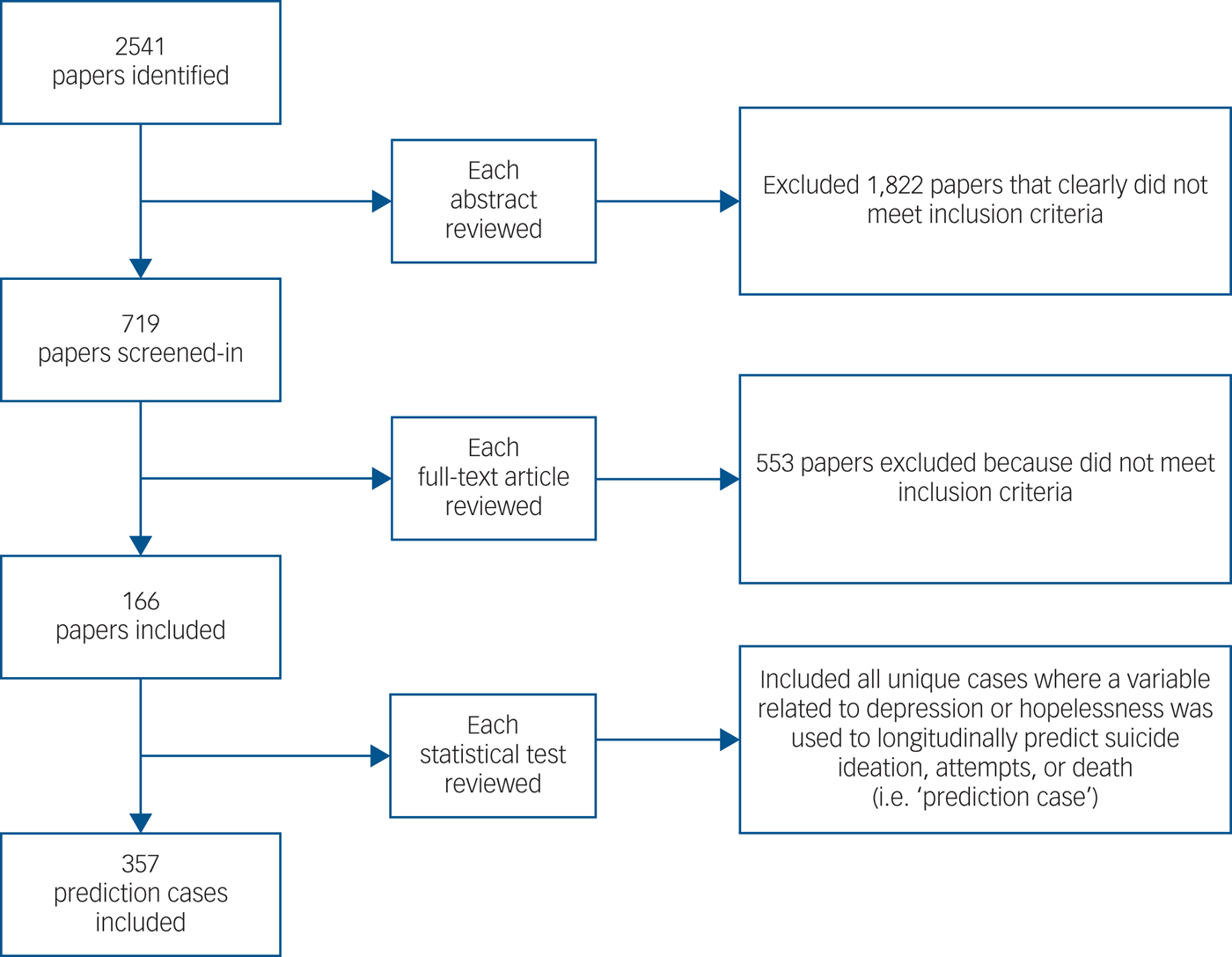
Fig. 1 PRISMA diagram.
Extraction and coding
Any statistical test that used a variable related to depression or hopelessness to predict suicide ideation, attempt or death was retained for analysis and termed a ‘prediction case’. To ensure case independence, we removed duplicate cases (n = 7), which occurred when the same data were re-analysed across multiple publications and/or multiple follow-up assessments using the same predictors were included in a single study. In the first case, we retained the most inclusive study; in the latter, we retained estimates at the final assessment. Our rationale for doing so was that the furthest time point was often most inclusive; however, results remained virtually unchanged when we retained the shortest time point instead. We identified a total of 357 unique prediction cases.
Data extracted from each study included: authors, publication year, follow-up length, number of participants with histories of self-injurious thoughts or behaviours, sample type (general population (i.e. samples in which no participant was selected on the basis of psychopathology or self-injurious thoughts or behaviour history), clinical (i.e. samples in which participants were selected on the basis of psychopathology history), self-injurious (i.e. samples in which participants were selected on the basis of self-injurious thoughts or behaviour history), sample age group (i.e. adult, adolescent, mixed)), predictor variable, outcome variable and any relevant statistics from each prediction case.
The process for selecting and coding prediction cases involved several iterative steps to ensure the validity and completeness of our meta-analysis. Each prediction case was assigned risk-factor categories to ensure meaningful summary of effects. Initial codes were independently determined by one of the authors with an advanced degree in psychology (i.e. masters or doctoral level). Each code was subsequently examined by two additional authors with advanced degrees in psychology. Discrepancies were resolved through discussion until consensus.
Study quality
Methodological variability across studies may influence the accuracy of results in meta-analyses. The inclusion criteria in the present meta-analysis, however, required that studies share a common core design (i.e. longitudinal) and outcome (i.e. suicide ideation, attempt or death), thereby substantially constraining the study pool. As a result, relative to typical treatment meta-analyses, this meta-analysis includes studies that are highly methodologically uniform.
Nonetheless, finer-grained methodological differences between studies exist; however, no criteria have been established to definitely inform a priori hypotheses about how these differences may have an impact on study quality. For example, no objective guidelines exist about how length of follow-up (for example 1 month v. 1 decade) or sample severity (for example general population v. self-injuring) influence prediction. As such, to empirically evaluate the effects of these methodological differences, we conducted moderator analyses. We also accounted for study heterogeneity by using random-effects models.
Statistical analyses
Meta-analyses were performed using Comprehensive Meta-Analysis, Version 3.Reference Thompson and Becker29 When provided, zero-order (i.e. unadjusted) effects were used as this provided the purest effect estimate (results were highly consistent across analyses using only unadjusted estimates v. only adjusted v. both unadjusted and adjusted). When odds ratios (ORs) were not provided, we calculated them based on 2 × 2 contingency tables, correlations, independent group means and group sample size and t-statistics or P-value. As hazard ratios cannot be converted into ORs, they were analysed separately (see Supplementary Data 2). Outliers were defined as values greater than 5 s.d. from the mean (n = 3, 0.01%). Results were nearly identical when outliers were retained. To ensure reliability of effects, we required a minimum of four prediction cases to report an estimate.
Between-study heterogeneity was quantified using I 2-tests. Between-study variance is common across studies because of differences in methodology; as such, a random-effects model was used for all meta-analyses. Random-effects models assume a distribution of effects across studies. Thus, the combined effect estimated using a random-effects model represents the mean of the distribution of true effects rather than a single true effect (as in fixed-effects models). Given that between-study variance is common, fixed-effects models are rarely indicated. Using random-effects models, heterogeneity across studies is accounted for in the weighting and calculation of each prediction case.
To quantify publication bias, we considered multiple indices. Specifically, we evaluated Duval and Tweedie's trim and fill test, Egger's regression test, Begg and Mazumdar rank correlation test, Classic fail-safe N, Orwin's fail-safe N and funnel plot symmetry.
In this meta-analysis, we assumed case independence. However, some studies contributed multiple prediction cases for which some of the predictors may have been correlated (for example number of episodes; symptom severity). Simulation studies suggest that not accounting for existing dependence has very little effect on point estimates, but can result in slight underestimates of variance and confidence intervals, which increases type 1 error.Reference Thompson and Becker29 Fully accounting for dependence requires modelling the covariance structure of each case; however, published studies rarely reported this information. Given this, we examined the potential effects of dependence by running analyses that assumed complete dependence of cases within each study (compared with Scammacca et al Reference Scammacca, Roberts and Stuebing30). Although these analyses represent a level of dependence that far exceeds the actual levels, they provide insight into the upper limit of the effects of dependence on our results. Due to space limitations, we provide only results from these models for overall prediction. Results from these analyses were highly consistent with those assuming complete independence.
For moderator analyses, we employed meta-regression using a random-effects model and unrestricted maximum likelihood. In meta-analyses, moderator analyses test whether the variation in effect size across studies is associated with differences in moderators. In this meta-analysis, we were interested in the potential moderating effects of sample age group, sample severity and study follow-up length. We examined these potential moderation effects on overall prediction as well as on the effects of depression and hopelessness.
Results
Descriptive summary of existing literature
Publication dates ranged from 1971 to 2014. The number of prediction cases has sharply increased over time, with 2.0% of cases published before 1985, 14.1% published in 1984–1994, 32.5% in 1995–2004 and 51.4% in 2005–2014. Suicide attempt was the most common outcome (47.7%) followed by death (33.6%) and ideation (18.7%). The majority of cases used adult samples (69.8%); 23.4% used adolescent samples and 6.8% used mixed adult–adolescent samples. Among adolescent samples, the most common outcome was attempt (63.9%) followed by ideation (33.7%); death cases were rare (2.4%).
Only 16.4% of cases used self-injurious samples; clinical (52.5%) and general (31.1%) samples were much more common. Clinical samples were the most common sample type within suicide death (60.5%) and attempt (56.8%) cases, followed by general (death: 17.6%; attempt: 27.2%) and then self-injurious samples (death: 21.8%; attempt: 16.0%). Among ideation prediction cases, general samples were predominant (65.2%), and clinical (27.3%) and self-injurious (7.6%) were less common.
The average follow-up length was about 9 years (median 60 months; s.d. = 122.20 months; range: 1–708 months). Death by suicide prediction cases had substantially longer follow-ups (median 156 months; mean 181.35 months; s.d. = 156.35 months) than suicide attempt (median 36 months; mean 67.31 months; s.d. 68.40 months) and ideation (median 24 months; mean 70.79 months; s.d. = 95.03 months).
Effects on subsequent suicide ideation, attempt and death
Publication bias and overall prediction
Overall prediction estimates reflect the pooled effect of all predictors on the outcome of interest, regardless of category type.
Ideation
OR analyses included 66 cases, generating a weighted mean OR (wOR) of 1.96 (95% CI 1.81–2.13). Publication bias was evident across several indices (Table 1; Fig. 2). Between-study heterogeneity was high (I 2 = 95.13%). Results were similar when complete case dependence was assumed (wOR = 2.01, 95% CI 1.84–2.20).

Fig. 2 Funnel plots. (a) suicide ideation, (b) suicide attempt and (c) suicide death.
Table 1 Publication biasa

wOR, weighted mean odds ratio.
a. Classic and Orwin's fail-safe N values represent the number of studies required to nullify the observed effects; Begg & Mazumdar rank correlation test computes the rank-order correlation between effect estimates and standard error; Egger's test of the intercept uses precision (i.e. the inverse of the standard error) to predict the standardised effect (i.e. effect size divided by the standard error). The size of the effect is reflected in the slope and bias is reflected in the intercept (B 0); missing cases under Duval & Tweedie's trim and fill are the number of cases estimated as missing below the mean.
Attempt
A total of 166 prediction cases were included, yielding a wOR of 1.63 (95% CI 1.55–1.72). Heterogeneity was high (I 2 = 88.98%). Within models assuming complete dependence, the wOR was 1.64 (95% CI 1.54–1.73). Publication bias was evident across multiple indices (Table 1; Fig. 2).
Death
Analyses included 116 prediction cases, producing a wOR of 1.33 (95% CI 1.18–1.49). Publication bias was detected across multiple indices (Table 2; Fig. 1). Heterogeneity was high (I 2 = 89.21%). Results were consistent within models assuming complete case dependence (wOR = 1.58, 95% CI 1.34–1.86).
Table 2 Risk-factor category analyses across outcomes

n, number of prediction cases; wOR, weighted mean odds ratio; –, indicates that information was not available.
a. Estimates are not reported for analyses involving three or fewer cases, as the small number of cases compromise the reliability of estimates; risk-factor categories with fewer than three cases across all three outcomes are not listed in the table.
Risk-factor category analyses
To ensure reliability of estimates, more than three prediction cases were required for each risk-factor category analysis. See Table 2 for results of all risk-factor category analyses.
Hopelessness
Hopelessness significantly predicted all outcomes. The strongest effect was on ideation (wOR = 2.19, 95% CI 1.60– 3.00); estimates were weaker predicting attempt (wOR = 1.95, 95% CI 1.59–2.39) and death (wOR = 1.98, 95% CI 1.46–2.69).
Depression-related disorders and symptoms
A diagnosis of major depressive disorder (MDD) was associated with significantly increased odds of ideation (wOR = 2.48, 95% CI 1.32–4.67), attempt (wOR = 2.38, 95% CI 1.84–3.07) and death (wOR = 1.50, 95% CI 1.04– 2.17).
Depressive symptoms were significant predictors of ideation (wOR = 1.57, 95% CI 1.45–1.70) and attempt (wOR = 1.30, 95% CI 1.23–1.37) but not death. Several self-report inventories were particularly common, allowing for additional analyses examining the effects of particular self-reports. The Beck Depression Inventory (BDI) and Center for Epidemiologic Studies Depression Scale significantly predicted ideation; there were too few cases of the Hamilton Rating Scale for Depression predicting ideation to produce a reliable estimate. Although all three significantly predicted attempts, none predicted suicide death (Table 2).
The subcategory, clinical features of depression, included predictors used to specify the nature of depression, such as age and type of onset (for example insidious) as well as the number, severity, duration and specific type (for example melancholic) of episodes. Considered jointly, clinical features were only significant predictors for attempts (wOR = 1.57, 95% CI 1.23–2.01) but not death; not enough cases were available in the prediction of ideation.
We also tested the effects of specific clinical features of depression. Number of episodes (wOR = 1.46, 95% CI 1.02–2.11) and course (wOR = 2.40, 95% CI 1.35–4.26) significantly increased the odds of attempt. Type of depressive episode was the only feature to significantly predict suicide death (wOR = 1.45, 95% CI 1.08–1.96). Cases were insufficient to examine finer-grained subcategories of course and episode type. However, most cases in the ‘course’ label reflect longer or more chronic illness. As such, comparisons examine longer illness duration v. shorter illness duration. The ‘episode type' label includes a range of specifiers characterizing the particular type (such as psychotic, atypical) of depressive episode. Comparisons on this level examine the presence v. absence of an episode-type specifier. Although other features have been tested (for example age of onset, comorbid presentation), too few cases existed in each category to produce reliable estimates.
The category, unspecified mood disorder diagnosis, included all prediction cases that used unspecified ‘mood disorder’ or ‘affective disorder’ as predictors. Results indicated that unspecified mood disorder diagnosis was only a significant predictor of death (wOR = 1.64, 95% CI 1.11–2.42). Several cases examined the effects of dysthymia; however, only attempt cases had a sufficient number of cases to produce a reliable estimate, which was not significant.
Too few cases were available for any outcome for family history of depression.
Moderator analyses: overall prediction
Sample age
Predicting ideation, adult samples produced statistically equivalent effects (wOR = 2.15, 95% CI 1.89–2.46) relative to adolescent samples (wOR = 1.95, 95% CI 1.66–2.30, χ2(1) = 0.84, P = 0.34); not enough cases were available to reliably report the effect on ideation for mixed samples (n = 3). Effects were statistically equivalent across age groups in the prediction of attempts (adults: wOR = 1.62, 95% CI 1.50–1.75); adolescents: wOR = 1.71, 95% CI 1.58–1.86; mixed: wOR = 1.95, 95% CI 1.12–3.39, χ2(2) = 1.21, P = 0.55) and death (adults: wOR = 1.30, 95% CI 1.14–1.48; mixed: wOR = 1.41, 95% CI 1.18–1.68), χ2(1) = 0.54, P = 0.46); there were too few cases among adolescents predicting suicide death.
Follow-up length
There was no significant effect of follow-up length on the prediction of ideation (b = 0.0003, P = 0.79), attempt (b = 0.0003, P = 0.72) or death (b = −0.0005, P = 0.36).
Sample severity
Predicting ideation, effects were significantly stronger among general population samples (wOR = 2.60, 95% CI 2.21–3.05) than clinical (wOR = 1.31, 95% CI 1.17–1.47; χ2(1) = 45.96, P < 0.001) and self-injurious samples (wOR = 1.12, 95% CI 1.00–1.25; χ2(1) = 69.19, P < 0.001). Prediction of ideation was significantly stronger among clinical relative to self-injurious samples (χ2(1) = 3.85, P = 0.05). Predicting attempts, effects were significantly stronger among general (wOR = 2.00, 95% CI 1.82–2.20) than clinical (wOR = 1.52, 95% CI 1.42–1.64, χ2(1) = 20.50, P < 0.001) and self-injurious samples (wOR = 1.49, 95% CI 1.15–1.94, χ2(1) = 4.28, P < 0.04); prediction was statistically equivalent among self-injurious compared with clinical samples (χ2(1) = 0.03, P = 0.88). Suicide death prediction was also significantly stronger among general (wOR = 2.01, 95% CI 1.40–2.87) compared with clinical (wOR = 1.17, 95% CI 1.03–1.34, χ2(1) = 7.66, P < 0.01) samples, but statistically equivalent to self-injurious samples (wOR = 1.43, 95% CI 1.12–1.82, χ2(1) = 2.42, P = 0.12); clinical and self-injurious samples were also statistically equivalent (χ2(1) = 1.76, P = 0.18).
Moderator analyses: depression effects
Sample age
Effects of depression-related predictors were statistically equivalent across age groups in the prediction of ideation (adults: wOR = 2.25, 95% CI 1.93–2.63; adolescents: 1.95, 95% CI 1.66–2.30; mixed: insufficient cases; χ2(1) = 3.72, P = 0.05) and attempts (adults: wOR = 1.53, 95% CI 1.42–1.66; adolescents: wOR = 1.62, 95% CI 1.50–1.76; mixed: wOR = 1.64, 95% CI 0.98–2.74; χ2(2) = 0.96, P = 0.62). Prediction was statistically equivalent among mixed samples (wOR = 1.41, 95% CI 1.18–1.68) relative to adult samples (wOR = 1.24, 95% CI 1.09–1.42; χ2(1) = 1.27, P = 0.26) in the prediction of suicide death; there were too few cases among adolescent samples.
Follow-up length
There was no significant effect of follow-up length on the predictive power of depression-related factors across any outcome (ideation: b = −0.0003, P = 0.54; attempt: b = 0.0007, P = 0.50; death: b = −0.0003, P = 0.55).
Sample severity
Predicting ideation, effects of depression-related factors were significantly stronger among general (wOR = 2.47, 95% CI 2.10–2.90) than clinical samples (wOR = 1.39, 95% CI 1.21–1.59, χ2(1) = 27.74, P < 0.001) and self-injurious samples (wOR = 1.61, 95% CI 0.72–3.62); χ2(2) = 28.53, P < 0.001). Effects were also significantly stronger among general population samples (wOR = 2.12, 95% CI 1.91–2.34) in the prediction of attempts (clinical: wOR = 1.38, 95% CI 1.29–1.47; χ2(1) = 49.73, P < 0.001; self-injurious: wOR = 1.37, 95% CI 1.03–1.81, χ2(1) = 8.25, P < 0.01). In the prediction of suicide death, general samples demonstrated stronger effects (wOR = 1.94, 95% CI 1.34–2.81) relative to clinical samples (wOR = 1.15, 95% CI 1.01–1.32; χ2(1) = 6.83, P < 0.01) and statistically equivalent effects relative to self-injurious samples (wOR = 1.32, 95% CI 1.04–1.68, χ2(1) = 2.92, P = 0.09).
Moderator analyses: hopelessness effects
Sample age
Potential moderating effects of sample age on the effects of hopelessness on ideation and death prediction were not examined, given an insufficient number of cases. In the prediction of ideation, all cases were among adult samples; in the prediction of suicide death, all cases were among adult samples. Effects were statistically equivalent among adult (wOR = 1.93, 95% CI 1.48–2.51) and adolescent (wOR = 1.92, 95% CI 1.48–2.48; χ2(1) = 0.001, P = 0.98) samples in the prediction of attempts; too few cases existed using mixed samples (n = 1).
Follow-up length
There were no significant moderating effects of follow-up length on the prediction of ideation (b = −0.006, P = 0.30), attempt (b = −0.002, P = 0.40) or death (b = −0.004, P = 0.30).
Sample severity
Moderating effects of sample severity for ideation were not conducted as there were too few cases in each group. There were no significant effects of sample severity in the prediction of suicide attempt (general: wOR = 1.91, 95% CI 1.34–2.73; clinical: wOR = 1.98, 95% CI 1.51–2.59; self-injurious: wOR = 2.35, 95% CI 1.02–5.40, χ2(2) = 0.20, P = 0.91) or death (general: too few cases (n = 2); clinical: wOR = 1.69, 95% CI 1.07–2.66; self-injurious: wOR = 2.38, , 95% CI 1.34–4.24, χ2(1) = 0.84, P = 0.36)).
Discussion
Main findings
Depression and hopelessness do confer risk for suicide ideation, attempt and death. At least when studied within the narrow methodological constraints of the existing literature, overall prediction estimates did not exceed wORs of 2.0 for any outcome, with the weakest effects produced in the prediction of death by suicide. Effects remained weak regardless of sample age or severity, or study follow-up length. Publication bias was evident across all outcomes, and most pronounced for ideation and attempt prediction. Accounting for publication bias estimates further reduced effects, suggesting the actual effects of depression and hopelessness are likely smaller than published research indicates.
Prediction of ideation, attempts and death varied slightly across risk-factor categories. The strongest predictors of suicide ideation were hopelessness, BDI scores and an MDD diagnosis. For suicide attempts, an MDD diagnosis and course yielded the strongest effects. Among the few factors that predicted suicide death, hopelessness, unspecified mood disorder diagnosis and an MDD diagnosis were the most robust.
Although some moderator effects emerged, results remained largely unchanged. One consistent notable finding, however, was that effects appeared stronger among general relative to clinical and self-injurious samples. We reason that this is likely a methodological artefact. Study designs that include clinical and self-injurious samples typically involve more stringent comparison groups; consequently, these designs necessarily control for a host of risk factors not usually accounted for in designs using general samples. Selecting more homogenous samples (for example, clinical or self-injurious) can also result in a restricted range of the predictors and outcomes. This in turn can limit the ability to detect significant associations. Of note, this pattern of findings echoes findings from prior meta-analyses (for example Bentley et al Reference Bentley, Franklin, Ribeiro, Kleiman, Fox and Nock31 and Ribeiro et al 32).
To evaluate clinical significance, we considered the magnitude of effects given the absolute risk of our outcomes. Suicidal thoughts and behaviours are rare. In a given year, the prevalence of suicide deaths in the USA is 0.00013. The strongest predictor of suicide death in this meta-analysis was hopelessness (wOR = 1.98). Doubling the odds of suicide death only marginally improves our ability to detect risk of death by suicide, given its extremely low prevalence. Moreover, most clinicians are tasked with predicting risk over the course of days or weeks, making suicidal behaviours even rarer still. Therefore, although hopelessness is strong relative to other risk factors, it is still weak in an absolute sense. This raises concerns about relying solely on depression and hopelessness to detect risk.
Interpretation of our results and methodological constraints
At first glance, our results appear to stand at odds with decades of prominent suicide theories and clinical wisdom. We caution against equating findings from this meta-analysis to the true nature of these phenomena, however. Results of this meta-analysis may or may not reflect how these constructs actually relate to suicidal thoughts and behaviours; instead, results reflect how these constructs have been studied to date. The findings of this meta-analysis also necessarily reflect any methodological constraints shared across the existing literature. We identified three major methodological issues.
First, most studies involved extremely long follow-ups. The average follow-up was 9 years, with very few studies focusing on short-term prediction. This represents a critical gap in knowledge, given existing clinical demands to assess risk over the course of days or weeks. It is notable, though, that the effects of these factors, although modest, do appear to be long lasting. As such, these factors may have stronger effects over more clinically useful (i.e. shorter) time frames.
Second, risk factors were typically measured as static, trait-like phenomena. As a result, virtually no studies examined how changes in these predictors could influence risk. Suicide risk is often conceptualised as transient and fluctuating, however. Assessing and studying these risk factors as state-like phenomena may be fruitful.
Third, most studies examined the effects of risk factors in isolation. Advancing prediction accuracy will likely require simultaneous consideration of many risk factors and the complex relationships between those factors.33 Recent efforts applying machine learning to prediction efforts support this approach.3Reference Kraemer, Kazdin, Offord, Kessler, Jensen and Kupfer4, 3Reference LeFevre5 Thus, when considered in combination with many other factors in an optimal fashion, depression and hopelessness may be very important predictors of suicidal behaviour.
Implications
In closing, depression and hopelessness do appear to increase suicide risk. Effects were weaker than expected; however, this may be an artefact of methodological constraints shared across the existing literature. Results from this investigation highlight a need to re-evaluate our current approaches to risk-detection strategies, especially those that rely solely on the presence of depression or hopelessness. Recent machine learning efforts indicate that accurate prediction is possible; however, it will likely require consideration of complex combinations of many risk factors. The present work also highlights substantive gaps in knowledge. Research designs that examine the potential protean nature of these factors, especially in the context of other sources of risk, to predict suicidal thoughts and behaviours in the short term will likely be particularly valuable. We look forward to future work that advances beyond the methodological constraints of the existing literature in order to make meaningful inroads in the prediction and prevention of suicidal thoughts and behaviours.
Supplementary material
Supplementary material is available online at https://doi.org/10.1192/bjp.2018.27.
eLetters
No eLetters have been published for this article.