1. Introduction
Pronunciation plays a key role in communication competence of foreign language learners as it is directly linked to the speech comprehensibility among interlocutors (Brinton, Celce-Murcia & Goodwin, Reference Brinton, Celce-Murcia and Goodwin2010; Goh & Burns, Reference Goh and Burns2012; Hismanoglu & Hismanoglu, Reference Hismanoglu and Hismanoglu2010; Sicola & Darcy, Reference Sicola, Darcy, Reed and Levis2015). In addition, second language (L2) learners often recognize the desire and need to improve their pronunciation (McCrocklin & Link, Reference McCrocklin and Link2016; LeVelle & Levis, Reference LeVelle, Levis, Levis and Moyer2014). Unfortunately, many language teachers choose not to teach pronunciation, and one of the important reasons for this neglect is due to the lack of adequate training and relevant pedagogical strategies in teaching pronunciation in L2 teachers (Henderson et al., Reference Henderson, Frost, Tergujeff, Kautzsch, Murphy, Kirkova-Naskova, Waniek-Klimczak, Cunningham and Curnick2012; Kirkova-Naskova et al., Reference Kirkova-Naskova, Tergujeff, Frost, Henderson, Kautzsch, Levey, Murphy, Waniek-Klimczak, Levis and LeVelle2013; Sicola & Darcy, Reference Sicola, Darcy, Reed and Levis2015).
According to Kirkova-Naskova (Reference Kirkova-Naskova2019), it was expected that L2 teachers may not have a clear understanding of the appropriate pedagogical approaches in teaching pronunciation since there existed contradictions and controversies in L2 pronunciation instructional methods. One of the prominent debates was whether pronunciation should be taught as a separate or integral language skill (Kirkova-Naskova, Reference Kirkova-Naskova2019). For example, some scholars believed that pronunciation should be taught as a separate language skill in which the focus of teaching was on the acquisition of L2 sounds and accurate pronunciation (Brown, Reference Brown1987). In contrast, others argued that pronunciation intelligibility rather than accuracy should be the main focus of pronunciation teaching, and that pronunciation was inseparable from communicative language skill (Pennington & Richards, Reference Pennington and Richards1986).
Despite the opposing viewpoints, there is a common consensus among scholars that it is important for teachers to help L2 learners understand the connection between sounds and their respective meanings, as well as the physical properties of sounds (i.e. how the sounds are produced, transmitted, and perceived) and their phonological concepts (i.e. how the sounds are organized in a language) (Kirkova-Naskova, Reference Kirkova-Naskova2019). Therefore, the investigations of efficient pronunciation teaching techniques are beneficial (Kirkova-Naskova, Reference Kirkova-Naskova2019; Lee, Jang & Plonsky, Reference Lee, Jang and Plonsky2015).
Some techniques for teaching L2 pronunciation have been widely acknowledged and promoted, such as covert rehearsal, reading poetry/jazz chants for rhythm, shadowing, and recording oneself to listen for errors. Although these techniques can be used to assist L2 students’ pronunciation practices, most of them do not provide opportunities for students to receive feedback on their oral production (McCrocklin, Reference McCrocklin2019). However, it is noticeable that some students may struggle to improve their L2 pronunciation due to influences from their first languages, and they often cannot recognize their own pronunciation errors (McCrocklin, Reference McCrocklin2019). Therefore, providing immediate and clear feedback may be necessary for students to develop their L2 pronunciation (Saito & Lyster, Reference Saito and Lyster2012).
With advancements in technology, automatic speech recognition (ASR) has been developed to deliver useful pronunciation feedback to students. The use of ASR is an advantage because of its capability to deliver individualized feedback to students, while teachers are unlikely to provide as much individual feedback to each student due to time constraints in teaching (Neri, Mich, Gerosa & Giuliani, Reference Neri, Mich, Gerosa and Giuliani2008; Offerman & Olson, Reference Offerman and Olson2016). Moreover, students tend to have stronger motivation and less anxiety when practicing pronunciation with computer-based ASR programs (Liakin, Cardoso & Liakina, Reference Liakin, Cardoso and Liakina2017; McCrocklin, Reference McCrocklin2019).
Although various ASR tools seem promising in training students’ pronunciation, the effects of using ASR in pronunciation training is not clear (Cucchiarini & Strik, Reference Cucchiarini, Strik, Kang, Thomson and Murphy2018; Golonka, Bowles, Frank, Richardson & Freynik, Reference Golonka, Bowles, Frank, Richardson and Freynik2014; Spring & Tabuchi, Reference Spring and Tabuchi2022). Thus, the present study attempts to meta-analyze the primary studies that focus on the effectiveness of ASR on ESL/EFL students’ pronunciation. The two main goals of the study are to (1) explore the overall effect size of using ASR in pronunciation training, and (2) investigate the influence of the moderator variables on the use of ASR in pronunciation training in terms of effect sizes.
The following section provides more background information on the effectiveness of ASR in ESL/EFL pronunciation, the potential factors affecting the effectiveness of ASR, and the motivation for conducting the present meta-analysis.
2. Literature review
2.1 The effectiveness of ASR in ESL/EFL pronunciation
Research on the effectiveness of ASR in facilitating ESL/EFL pronunciation has shown some inconsistent findings in the individual empirical studies. For instance, both Gorjian, Hayati and Pourkhoni (Reference Gorjian, Hayati and Pourkhoni2013) and Neri et al. (Reference Neri, Mich, Gerosa and Giuliani2008) compared the effects of an ASR program with a traditional method (e.g. textbook and teacher-fronted instruction) in ESL/EFL student pronunciation, but their findings were dissimilar. While Gorjian et al. (Reference Gorjian, Hayati and Pourkhoni2013) found that participants practicing pronunciation with the ASR program (e.g. Praat software) significantly outperformed those learning in the traditional method after 10 training sessions, Neri et al. (Reference Neri, Mich, Gerosa and Giuliani2008) showed the non-significant difference between the two groups (i.e. the group with the use of an ASR application PARLING and the group with the traditional teaching method) in their pronunciation performance after four weeks of training.
Apart from the inconsistent findings found between studies, the effect of ASR was also varied in within studies. For example, Evers and Chen (Reference Evers and Chen2022) investigated the effectiveness of an ASR dictation program (i.e. Speechnotes) on three different aspects of pronunciation (e.g. accentedness, comprehensibility, and spontaneous speech). The researchers found no significant difference between the experimental and control groups in terms of accentedness, but significant differences were found in comprehensibility and spontaneous speech. In another similar study, Evers and Chen (Reference Evers and Chen2021) found variations in the post-test effect size powers of the experimental groups, visual style and verbal style learners practicing pronunciation with Speechnotes, in their pronunciation performance in reading and spontaneous speech tasks.
The varying ASR programs, methods for measuring pronunciation, and treatment durations used in the studies seem to have led to the inconsistent results observed in the primary studies. Conducting a meta-analysis with robust evidence of effect sizes could help determine the overall effect size and explain the reasons for the inconsistent results (Lipsey & Wilson, Reference Lipsey and Wilson2001).
2.2 Potential moderating factors in ASR-assisted pronunciation
2.2.1 Treatment data
There are several treatment factors that potentially influence the effects of ASR, such as ASR feedback feature (e.g. explicit corrective, indirect), target measure (e.g. segmental, suprasegmental), treatment duration, and learning activity. First, the ASR feedback feature (e.g. explicit corrective, indirect) has been the subject of argument among scholars. On the one hand, some scholars believe that ASR programs with explicit corrective feedback were more helpful to students’ pronunciation development (Hincks, Reference Hincks, Reed and Levis2015; Neri, Cucchiarini & Strik, Reference Neri, Cucchiarini and Strik2006; Strik, Neri & Cucchiarini, Reference Strik, Neri and Cucchiarini2008). On the other hand, other scholars argued for the use of ASR programs with indirect feedback because those programs were also effective in pronunciation training and enjoyable to practice (Liakin et al., Reference Liakin, Cardoso and Liakina2017; McCrocklin, Reference McCrocklin2019; Mroz, Reference Mroz2018). Therefore, it could be useful to examine the extent to which different ASR feedback features could influence the pronunciation learning outcome differently to have some response to the aforementioned arguments.
In the present study, explicit corrective feedback refers to the ability of an ASR tool to provide some detailed feedback on the participants’ speech. For example, the programs (e.g. Speech Analyzer, Praat) could offer the illustration of speech waveform and its spectrogram on students’ pronunciation. These graphical representations of speech allowed students to understand why and how their pronunciation was similar to or different from a native speaker’s pronunciation. In addition, the teachers could also draw on these forms of feedback to give students objective evaluation and explanation that could help them during the practice process (Arunsirot, Reference Arunsirot2017; Gorjian et al., Reference Gorjian, Hayati and Pourkhoni2013). For another example, the ASR programs (e.g. SpeechAce, Fluent English) could provide some feedback messages, such as the scores for each word, each syllable, and each phoneme pronounced by the students. These messages helped students accurately understand the specific areas of their pronunciation mistakes regarding certain words, syllables or phoneme, and to what extent (e.g. good, not bad, wrong) did they perform on each specific pronunciation (Liu, Zhu, Jiao & Xu, Reference Liu, Zhu, Jiao, Xu, Cheung, Kwok, Kubota, Lee and Tokito2018; Moxon, Reference Moxon2021).
Indirect feedback refers to the type of feedback provided by ASR tools that only transcribes what students say and displays the text, or gives simple positive or negative responses (e.g. “correct” or “incorrect”) to the student’s voice input. For instance, some ASR programs, such as Speechnotes, Windows Speech Recognition, or PARLING, provide this type of feedback. Students can identify their mispronounced words from the displayed text or the feedback responses and try again (Evers & Chen, Reference Evers and Chen2021, Reference Evers and Chen2022; McCrocklin, Reference McCrocklin2019; Neri et al., Reference Neri, Mich, Gerosa and Giuliani2008).
Second, target measure (e.g. segmental, suprasegmental) was one of the most critical considerations in pronunciation instruction as well as the field of computer-assisted pronunciation training (CAPT) (Lee et al., Reference Lee, Jang and Plonsky2015; Mahdi & Al Khateeb, Reference Mahdi and Al Khateeb2019). While some scholars emphasize the importance of teaching segmental pronunciation features (Levis, Reference Levis2005; Saito, Reference Saito2014), others argue that teaching suprasegmental features is more effective (Hahn, Reference Hahn2004; Isaacs & Trofimovich, Reference Isaacs and Trofimovich2012; Kang, Reference Kang2010). Thus, studying the impact of ASR on different pronunciation features (i.e. target measure) may provide insights into which approach is more beneficial with the use of ASR programs.
Third, treatment duration could potentially affect the effectiveness of a given intervention, and the factor was examined across different meta-analyses in different study fields (Lee et al., Reference Lee, Jang and Plonsky2015). This factor is particularly important to examine as longer treatment durations are often expected to result in stronger effects of the intervention (Lee et al., Reference Lee, Jang and Plonsky2015; Mahdi & Al Khateeb, Reference Mahdi and Al Khateeb2019).
Fourth, the mode of learning activity (e.g. alone, with peers, or with a teacher) is a crucial factor to consider in ASR-assisted pronunciation instruction. There is some disagreement among scholars on this issue. For instance, McCrocklin (Reference McCrocklin2016) stressed the importance of exploring autonomous pronunciation development through ASR technology, without reliance on teacher feedback. However, the same author (McCrocklin, Reference McCrocklin2019) argued that teacher guidance is essential for successful learning outcomes with ASR. Additionally, some studies suggest that ASR-assisted pronunciation instruction with peers may be more effective than independent use of ASR (Evers & Chen, Reference Evers and Chen2021, Reference Evers and Chen2022; Tsai, Reference Tsai2015). To better understand the impact of different learning activities on ASR-assisted pronunciation instruction, a meta-analysis examining the differential effects of these approaches would be beneficial.
2.2.2 Population data
The participant factors were shown to greatly affect the effectiveness of an intervention across a large number of meta-analyses in different domains of second language acquisition (SLA) (Plonsky & Oswald, Reference Plonsky and Oswald2014). In addition, some participant factors such as age and proficiency were crucial to the effective use of CAPT in pronunciation learning (Mahdi & Al Khateeb, Reference Mahdi and Al Khateeb2019). Regarding participant age, it was predicted that young learners might receive larger benefit from pronunciation instruction given evidence from a critical period for phonological development (Flege, Yeni-Komshian & Liu, Reference Flege, Yeni-Komshian and Liu1999; Lee et al., Reference Lee, Jang and Plonsky2015; Trofimovich, Lightbown, Halter & Song, Reference Trofimovich, Lightbown, Halter and Song2009; Tsiartsioni, Reference Tsiartsioni, Psaltou-Joycey and Mattheoudakis2010). However, some studies have shown that ASR-assisted pronunciation programs may not be effective in recognizing speech produced by non-native young learners (Elenius & Blomberg, Reference Elenius and Blomberg2005; Gerosa & Giuliani, Reference Gerosa and Giuliani2004; Neri et al., Reference Neri, Mich, Gerosa and Giuliani2008). Given these conflicting results, examining the effects of participant age on the use of ASR-assisted pronunciation learning could be necessary.
In terms of participant proficiency, a common agreement in both pronunciation instruction and CAPT was that lower-level learners could yield larger improvement than higher-level learners (Derwing & Munro, Reference Derwing and Munro2005; Mahdi & Al Khateeb, Reference Mahdi and Al Khateeb2019). However, Lee et al. (Reference Lee, Jang and Plonsky2015) argued that higher-level learners may be more receptive to pronunciation instruction due to their stronger foundational knowledge and skills. Therefore, the effect power of different proficiency levels might be still of question and necessary to be explored.
2.3 Motivation for the present meta-analysis
To our knowledge, no meta-analysis on the effectiveness of ASR for pronunciation learning has been conducted. The most closely related topic to our investigation is the meta-analysis on the effectiveness of CAPT conducted by Mahdi and Al Khateeb (Reference Mahdi and Al Khateeb2019). The authors referred to CAPT as the use of computer or mobile devices for pronunciation learning. It is clear that their study synthesized the effects of various functions in technology (e.g. speech recognition technology, translation, or multimedia) and generated an overall effect size. In their moderating variables, there was no exploration on the effects of different technological functions on students’ pronunciation. Therefore, our present meta-analysis would serve as a more fine-grained study solely investigating the overall effectiveness and appropriate applications of ASR in assisting ESL/EFL students’ pronunciation performance.
Mahdi and Al Khateeb’s (2019) study has contributed much insight into the effectiveness and application of CAPT. However, a few limitations in their methodology would call for further investigations to complement the findings. First, the authors’ use of only three terms for searching primary studies (viz. “computer-assisted pronunciation teaching,” “CAPT” and “teaching pronunciation with technology”) may be problematic for the meta-analysis. Many relevant studies may have been omitted because the three searching terms are not comprehensive enough to cover as many studies related to CAPT. One of the reasons is that CAPT is an umbrella term referring to a wide range of technologies used for pronunciation purposes rather than a specific type (Cucchiarini & Strik, Reference Cucchiarini, Strik, Kang, Thomson and Murphy2018). The three aforementioned terms therefore would not include many primary studies focusing on one specific technology. For example, multiple relevant studies on ASR were omitted (see, e.g., Arunsirot, Reference Arunsirot2017; Elimat & AbuSeileek, Reference Elimat and AbuSeileek2014; Hyun, Reference Hyun2018; Liu et al., Reference Liu, Zhu, Jiao, Xu, Cheung, Kwok, Kubota, Lee and Tokito2018; Park, Reference Park2017; Zuberek, Reference Zuberek2016). An update for the meta-analysis in CAPT is thus necessary, in which researchers would need to use more comprehensive key terms (e.g. more hypernymies, hyponymies or synonyms of CAPT and/or pronunciation) to collect more qualified studies.
The second issue relates to the measurement used for effect sizes. Mahdi and Al Khateeb (Reference Mahdi and Al Khateeb2019) employed Cohen’s d for effect-size calculation, which may not be recommended for studies with sample sizes equal to or less than 20 because Cohen’s d will cause a larger upward bias in the calculated effect sizes compared to Hedges’s g (Hedges & Olkin, Reference Hedges and Olkin1985). In their meta-analysis, it could be noted that a large number of the collected studies (i.e. 13 out of 20 studies) had a sample size in the experimental group equal to or less than 20. Therefore, employing Hedges’s g instead of Cohen’s d to calculate the effect sizes should be more preferable. All things considered, Mahdi and Al Khateeb’s (2019) study needs an update to ameliorate the quality of the findings. Our present meta-analysis is a fine-grained investigation on ASR and the aforementioned issues in their methodology are taken into consideration to conduct the study in a fitting manner. The present study is guided by the following two research questions:
-
1. What is the overall effect size of using ASR in ESL/EFL pronunciation training?
-
2. To what extent do moderator variables show an influence on using ASR in ESL/EFL pronunciation training in terms of effect sizes?
3. Methodology
3.1 Inclusion and exclusion criteria
The present meta-analysis aimed to investigate the effectiveness of ASR on ESL/EFL students’ pronunciation performance. Therefore, the inclusion and exclusion criteria of the collected studies were as follows:
-
1. The primary studies were experimental or quasi-experimental and had both experimental and control groups.
-
2. ASR programs were applied in the experimental group during the pronunciation learning processes. The studies that combined the use of ASR with translation or stand-alone multimedia (e.g. videos, photos, animation) were excluded so that the effect of ASR could be singled out.
-
3. The pronunciation learning outcomes should be reported with means, standard deviation, or other statistical values, such as t-value, F-value or p-value, with sample sizes that allowed the possible transformation to the Hedges’s g effect size value.
-
4. ASR programs were designed for English pronunciation training, and students were from ESL/EFL contexts. Studies that used ASR programs for practicing pronunciation of other languages rather than English (e.g. Dutch, French, Chinese) or had participants whose first language was English were excluded.
-
5. The primary studies were written in English.
3.2 Literature search
The database for collecting primary studies include ProQuest, ERIC, Google Scholar, and some SSCI journals in the computer-assisted language learning field (viz. Computer Assisted Language Learning, ReCALL, British Journal of Educational Technology, Australasian Journal of Educational Technology, CALICO, Language Learning and Technology, Journal of Computer Assisted Learning, System). There were two sets of keywords for searching potentially relevant studies. The first set was ASR-related keywords: automatic speech recognition, ASR, speech to text, ASR dictation, AI and speech recognition, speech technology. The second set was other keywords related to learning contexts (e.g. EFL, ESL, SLA, L2), ASR-associated functions (e.g. feedback, errors, scoring), and outcome measures (e.g. pronunciation, English pronunciation, speaking, English speaking, speaking skills, speaking performance, oral assessment, oral skills). The search strategy was the use of the first set of keywords and/or the combination with the keywords in the second set. After the initial pool of potential eligible primary studies was collected, their references were also scanned to avoid missing qualified articles. Figure 1 (in the supplementary material) presents the outcomes of the literature search following the PRISMA statement (i.e. Preferred Reporting Items for Systematic Reviews and Meta-Analyses) designed by Moher, Liberati, Tetzlaff and Altman (Reference Moher, Liberati, Tetzlaff and Altman2009).
3.3 Effect-size calculation
The post-test effect sizes were calculated using Hedges’s g because it takes into consideration effect-size weighting in studies with small sample sizes involved in the present meta-analysis. The equations for effect-size calculation are as follows:
-
1. Hedges’s g = J correction factor x
${{Mea{n_T}{\rm{\;}} - {\rm{\;}}Mea{n_C}} \over {\sqrt {{{\left( {{n_T} - 1} \right)SD_T^2{\rm{\;}} + {\rm{\;}}\left( {{n_C} - 1} \right)SD_C^2} \over {{n_T}{\rm{\;}} + {\rm{\;}}{n_C}{\rm{\;}} - 2}}} }}$
-
2. SEg = J correction factor x
$\sqrt {{1 \over {{n_T}{\rm{\;}}}}{\rm{\;}} + {\rm{\;}}{1 \over {{n_C}{\rm{\;}}}}{\rm{\;}} + {\rm{\;}}{{Cohen's{\rm{\;}}{d^2}} \over {2{\rm{\;x\;}}\left( {{n_T}{\rm{\;}} + {\rm{\;}}{n_C}} \right)}}} $
in which J
correction factor = 1 -
${3 \over {4{\rm{\;x\;}}\left( {{n_T}{\rm{\;}} + {\rm{\;}}{n_C}{\rm{\;}} - 2} \right) - 1}}$
; Cohen’s d
2
=
${{Mea{n_T}{\rm{\;}} - {\rm{\;}}Mea{n_C}} \over {\sqrt {{{\left( {{n_T} - 1} \right)SD_T^2{\rm{\;}} + {\rm{\;}}\left( {{n_C} - 1} \right)SD_C^2} \over {{n_T}{\rm{\;}} + {\rm{\;}}{n_C}{\rm{\;}} - 2}}} }}$
;
Mean T , n T , and SD T respectively represented the mean, sample size, and standard deviation of the treated group; Mean C , n C , and SD C respectively represented those of the control group (see, e.g., Hedges & Olkin, Reference Hedges and Olkin1985; Lipsey & Wilson, Reference Lipsey and Wilson2001).
3.4 Analysis
The effect sizes of the primary studies were manually pre-calculated, then inputted to the R software for the meta-analysis. The random-effects model was adopted. The packages used were metafor (Viechtbauer, Reference Viechtbauer2010), meta (Balduzzi, Rücker & Schwarzer, Reference Balduzzi, Rücker and Schwarzer2019), tidyverse (Wickham et al., Reference Wickham, Averick, Bryan, Chang, McGowan, François, Grolemund, Hayes, Henry, Hester, Kuhn, Pedersen, Miller, Bache, Müller, Ooms, Robinson, Seidel, Spinu, Takahashi, Vaughan, Wilke, Woo and Yutani2019), and dmetar (Harrer, Cuijpers, Furukawa & Ebert, Reference Harrer, Cuijpers, Furukawa and Ebert2021). More information about the codes and the guidelines for conducting a meta-analysis in R can be found in Harrer et al. (Reference Harrer, Cuijpers, Furukawa and Ebert2021).
In the gathered studies, some studies produced a single outcome, whereas others produced multiple outcomes within the same studies. The current meta-analysis collected 15 studies with a total number of 38 effect sizes; on average, each study contributed three effect sizes (38/15 = 2.53). It was clear that the effect sizes were not completely independent of each other. In this respect, conducting a three-level meta-analysis is possible to tackle the issue of dependency in the effect sizes. However, because the three-level model is complex, it may not be necessary if the two-level conventional meta-analysis could provide a comparable fit to the data (Harrer et al., Reference Harrer, Cuijpers, Furukawa and Ebert2021). To make the decision on which meta-analysis model should be applied to analyze the effect sizes, we used the ANOVA function in R software to compare the fit of the two models. The analysis showed a significantly better fit for the three-level model (
$X_1^2$
= 11.98, p < 0.001). Therefore, the overall effect size and moderating effect sizes computed in the present study were in accordance with the three-level meta-analysis model.
3.5 Moderators and coding procedure
There were 11 groups of potential moderators examined in the present meta-analysis (viz. publication year, publication type, participant age, participant proficiency, ASR feedback feature, ASR platform, target measure, assessment type, treatment duration, learning activity, setting). The description of the moderators is presented in the supplementary material. These moderators represented publication data, population data, and treatment data. Variables under participant age, target measure, treatment duration, and setting were categorized similarly to Mahdi and Al Khateeb (Reference Mahdi and Al Khateeb2019). Variables under assessment type were categorized according to Lee et al. (Reference Lee, Jang and Plonsky2015). The examination of learning activity was motivated by Wang, Lan, Tseng, Lin and Gupta (Reference Wang, Lan, Tseng, Lin and Gupta2020), which followed the subcategories under the commonly designed activities of studies on ASR. Some moderators specific to ASR- and pronunciation-related topics were added to explore their potentially moderating effects on students’ pronunciation (e.g. ASR feedback feature, ASR platform). The remaining moderators (e.g. publication year, publication type, and participant proficiency) could be found across many meta-analyses in our field (see, e.g., Boulton & Cobb, Reference Boulton and Cobb2017; Lee, Warschauer & Lee, Reference Lee, Warschauer and Lee2019).
The 11 groups of moderators underwent multiple cycles of coding by two independent raters. The raters discussed and agreed on the coding scheme before independently coding the primary studies. The overall interrater reliability of the codes was measured by kappa statistic and equaled 98.57. A few disagreements that occurred between the two raters were further discussed to decide the final chosen codes for the analysis.
4. Results
This section presents the results of the present study. There are two subsections. The first subsection, overall effect size, aims to answer the first research question, “What is the overall effect size of using ASR in ESL/EFL pronunciation training?”. The second subsection, moderator analysis, aims to answer the second research question: “To what extent do moderator variables show an influence on using ASR in ESL/EFL pronunciation training in terms of effect sizes?”. In the second subsection, the moderators were divided into three groups (e.g. treatment data, population data, publication data) for the reports.
4.1 Overall effect size
Table 1 presents the overall effect of implementing ASR for ESL/EFL student pronunciation learning compared to the non-ASR condition. The pooled effect size was medium (g = 0.69), and the confidence interval was not across zero (95% CI = [0.31, 1.08]). This indicated the reliable positive effect of ASR. The Q-statistic was significant (Q = 227.70; p < .001), meaning that there was substantial variability in the outcomes of the collected studies, and further moderator analyses were needed to explore potential accounts for the variability. The estimated variance indexes were τ 2 level 3 = 0.36 and τ 2 level 2 = 0.19; I 2 level 3 = 56.56% of the total variance can be attributed to between-study heterogeneity; I 2 level 2 = 29.55% of the total variance to within-study heterogeneity.
Table 1. Overall effect size and the heterogeneity test

Note. ES = effect size; CI = confidence interval; n = the number of effect sizes; g = Hedges’s g standardized mean differences; SE = standard error.
4.2 Moderator analysis
To investigate the accounts for the variability in the overall effect, a series of multiple meta-regression were conducted in eight groups of moderators. Publication year was the only continuous variable in the present data and its effect would be presented in the form of the beta coefficient. All the other moderators were categorical variables, and their effects were presented by using Hedges’s g effect size. Tables 2–4 summarize all the results from the moderator analyses in treatment data, population data and publication data respectively.
Table 2. Moderator analyses in treatment data
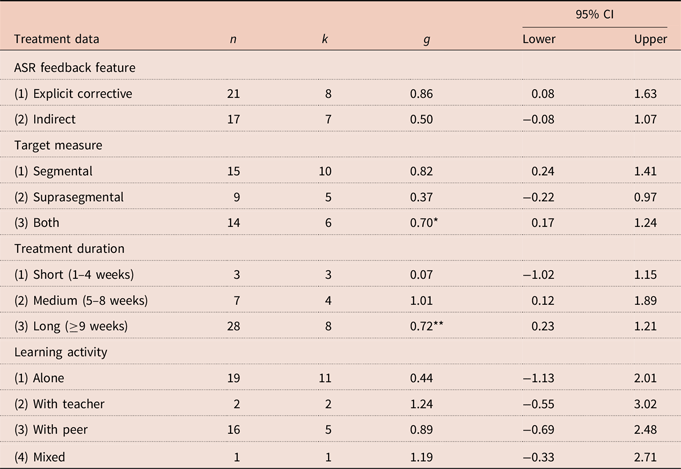
Note. n = the number of effect sizes; k = the number of studies; g = Hedges’s g standardized mean differences; CI = confidence interval.
*p < .05. **p < .01.
Table 3. Moderator analyses in population data
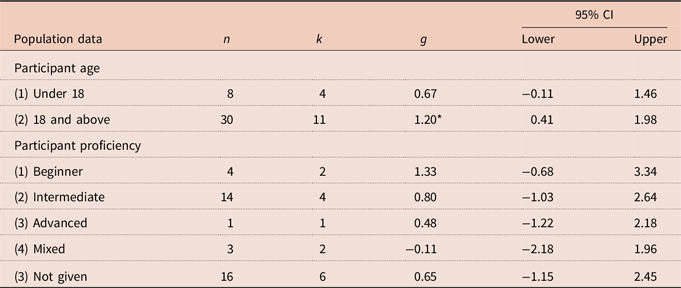
Note. n = the number of effect sizes; k = the number of studies; g = Hedges’s g standardized mean differences; CI = confidence interval.
*p < .05.
Table 4. Moderator analyses in publication data
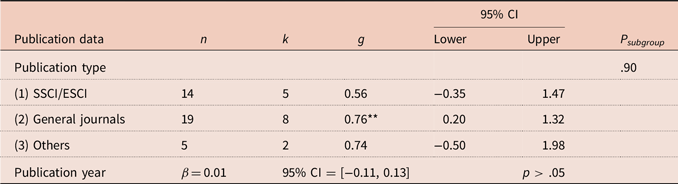
Note. n = the number of effect sizes; k = the number of studies; g = Hedges’s g standardized mean differences; CI = confidence interval.
**p < .01.
The first examined moderator in the treatment data explored in this meta-analysis was the ASR feedback feature. Based on the features of feedback offered by the ASR programs in the primary studies, two categories under the ASR feedback feature were classified including explicit corrective feedback (i.e. feedback offered by an ASR program such as providing the comparison of the speech input with the native speaker’s in terms of the speech waveform and its spectrogram and/or feedback messages on the pronunciation production) and indirect feedback (i.e. feedback offered by an ASR program that was simply a transcription of the speech input or the correct/incorrect responses to the input without any further clarification of the outcome). As shown in Table 2, eight studies with 21 numbers of effect sizes investigating explicit corrective feedback generated an overall large effect size (g = 0.86). Meanwhile, the overall effect size was medium (g = 0.50) in the case of indirect feedback, which was pooled from seven studies with 17 effect sizes.
The second examined moderator was target measure. The primary studies included in this meta-analysis either measured segmental and suprasegmental aspects of pronunciation separately or measured both aspects together. Most of these studies aimed at investigating the effect of ASR on segmental aspect of pronunciation (n = 10, k = 15), and the pooled effect size was large (g = 0.82). Meanwhile, the pooled effect size was small (g = 0.37) in suprasegmental pronunciation based on the synthesizing of five collected studies with nine effect sizes. Studies that measured both segmental and suprasegmental pronunciation (n = 14, k = 6) produced an overall medium effect size, and this effect size was significant (g = 0.70, p < .05).
The third moderator in the treatment data was treatment duration. Three categories under treatment duration were classified, including short (i.e. one to four weeks), medium (i.e. five to eight weeks), and long (i.e. equal to or more than nine weeks). The analysis indicated a negligible effect size in short duration (g = 0.07, n = 3, k = 3) and a large effect size in medium duration (g = 1.01, n = 7, k = 4). Most of the primary studies conducted the experiments in long duration (n = 28, k = 8), and generated a significant medium overall effect size (g = 0.72, p < .01).
The fourth moderator investigated in the present meta-analysis was learning activity. The collected studies had students practice ASR alone, with a teacher, with a peer, or having a mix of the three learning activity types. Most studies investigated the effectiveness of ASR with students practicing alone (n = 19, k = 11). However, the overall effect size was small (g = 0.44). In contrast, some studies investigating the effect of using ASR with a peer (n = 16, k = 5) produced a large overall effect size (g = 0.89). Two studies on using ASR with a teacher and one with the mix of the three learning activities showed a large overall effect size (g = 1.24 and g = 1.19 respectively).
In population data, the first investigated moderator was participant age. Age was divided into two subgroups (viz. under 18 years old; 18 years old and above) as similar to Mahdi and Al Khateeb (Reference Mahdi and Al Khateeb2019). Some collected studies investigating ESL/EFL participants who were under 18 years old (n = 8, k = 4) showed that ASR had a medium overall effect size (g = 0.67). In comparison, studies on those who were 18 years old and above showed a large overall effect size (g = 1.20), and the effect was also significant (p < .05).
The second moderator explored in the population data was participant proficiency levels. Many primary studies did not present the information about English proficiency levels of participants (n = 6, k = 16). Four studies with 14 effect sizes on students of intermediate proficiency generated a large overall effect size (g = 0.80). Two studies with four effect sizes on beginner proficiency level also demonstrated an initial large overall effect size (g = 1.33). However, the overall effect size was negligible (g = −0.11) based on two studies with three effect sizes on those participants who had different proficiency levels (i.e. mixed-proficiency ESL/EFL class). One study with one effect size on students of advanced proficiency showed a small effect size (g = 0.48).
Moderator analyses in publication data was to indirectly investigate the potential publication bias. As shown in Table 4, the overall effect sizes were medium in three different types of publications (g = 0.56 in SSCI/ESCI journals, g = 0.76 in general journals, and g = 0.74 in others). The difference among the three examined publication types was non-significant (p = .90). Moderator analysis on publication year demonstrated an unchanged relationship of the overall effect size with years (β = 0.01, p > .05).
5. Discussion
The main goals of the present meta-analysis study are to examine the overall effect of ASR use in ESL/EFL pronunciation training and to what extent do moderator variables show an influence on using ASR in ESL/EFL pronunciation training. In this section, we present our discussions regarding the findings following the above two research questions.
5.1 How effective is ASR for ESL/EFL pronunciation learning?
The result from the present meta-analysis showed a medium overall effect size (g = 0.69), meaning that ASR is moderately more effective on ESL/EFL pronunciation learning than the non-ASR condition. This provides support for the application of ASR to improve students’ pronunciation. The overall effect of ASR is in line with the overall effect of CAPT (d = 0.66) found in Mahdi and Al Khateeb (Reference Mahdi and Al Khateeb2019). Again, the present study is different for its specific consideration in ASR technology. To elaborate, only four out of the 20 collected studies (see, e.g., Gorjian et al., Reference Gorjian, Hayati and Pourkhoni2013; Neri et al., Reference Neri, Mich, Gerosa and Giuliani2008; Tsai, Reference Tsai2015; Young & Wang, Reference Young and Wang2014) in their study qualified to be included in our present meta-analysis, which comprised 15 studies. Therefore, the present study provides strong evidence solely for ASR application. In addition, the following moderator analyses can provide some recommendations on how to apply this specific technology (i.e. ASR) to improve students’ pronunciation.
5.2 To what extent do moderator variables show an influence on using ASR in ESL/EFL pronunciation training?
First, regarding the ASR feedback feature, findings show that ASR that provides explicit corrective feedback was found to be more effective in pronunciation learning than ASR that simply offers transcribed words or correct/incorrect responses in the speech input (g = 0.86 as opposed to g = 0.50). According to Coniam (Reference Coniam1999), Derwing, Munro and Carbonaro (Reference Derwing, Munro and Carbonaro2000), and Strik et al. (Reference Strik, Neri and Cucchiarini2008), ASR dictation programs that simply indicate mispronunciations are not sufficient for ESL/EFL learners to improve their pronunciation. Although these programs could be useful in helping students notice their mispronounced words, they were not able to illustrate the nature and the specific location of the errors. Furthermore, these researchers indicated that the dictation programs were originally developed for native speakers. Therefore, they had a lower recognition rate with non-native speech and could not provide non-native speakers (e.g. ESL/EFL learners) with satisfactory feedback. In contrast, research has shown that explicit error-highlighting and related feedback is more beneficial in pronunciation learning (Saito, Reference Saito2007, Reference Saito2011, Reference Saito2013) for which ASR programs with corrective feedback are more sufficient. All things considered, an ASR program with explicit corrective feedback is recommended in order to better facilitate L2 learners’ pronunciation (Hincks, Reference Hincks, Reed and Levis2015; Neri et al., Reference Neri, Cucchiarini and Strik2006; Strik et al., Reference Strik, Neri and Cucchiarini2008).
Second, regarding target measure, ASR is highly efficient in improving the segmental aspect of pronunciation (g = 0.82), but its effect on suprasegmental pronunciation is still rather small (g = 0.37). This result contradicts the finding of Mahdi and Al Khateeb (Reference Mahdi and Al Khateeb2019), which showed that CAPT has a large effect on suprasegmental pronunciation (d = 0.89) but a small effect on segmental pronunciation (d = 0.47). It should be noted that Mahdi and Al Khateeb’s (2019) meta-analysis included many primary studies implementing multimedia (e.g. videos, audios, animation, music, web) rather than ASR technology. It is very likely that other multimedia content may have played a role in these studies. According to Isaacs (Reference Isaacs, Kang, Thomson and Murphy2018), the limitation of ASR technology is that it predominantly focuses on the segmental aspect instead of suprasegmental aspect of pronunciation. This is because suprasegmental features are more difficult to target as they are amenable to sociolinguistic variables (e.g. age, gender, geography), which could be challenging to determine acceptable deviations from the norm for ASR technology (van Santen, Prud’hommeaux & Black, Reference van Santen, Prud’hommeaux and Black2009). As a result, ASR provides a higher effect on students’ improvement of segmental pronunciation and a lower effect on suprasegmental pronunciation.
However, in the studies that measured the two aspects of pronunciation (i.e. segmental and suprasegmental), a medium overall effect (g = 0.70) was found (see, e.g., Young & Wang, Reference Young and Wang2014; Evers & Chen, Reference Evers and Chen2021). This finding could be explained by the compensation in the effects of ASR on both aspects of pronunciation (i.e. the large effect on segmental pronunciation compensated for the small effect on suprasegmental pronunciation). Because the use of ASR has not provided the most desirable outcome (i.e. the large effect) in the instruction of both aspects of pronunciation, integrating other computer-assisted pronunciation training tools (e.g. multimedia) in pronunciation instruction could be considered (Mahdi & Al Khateeb, Reference Mahdi and Al Khateeb2019).
Third, regarding treatment duration, medium and long duration of ASR usage can result in desirable learning outcomes (g = 1.01 and g = 0.72 respectively), but a short duration (i.e. 1–4 weeks) is not effective (g = 0.07). The low effectiveness of short duration could be explained by DeKeyser’s (2007) skill acquisition theory. Initially, students gain awareness of the English pronunciation–related rules (i.e. acquisition of declarative knowledge) through the ASR feedback system. Then, students receive the opportunities to internalize the ASR feedback through thorough and deliberate training (i.e. transformation of declarative knowledge into procedural knowledge). Finally, continuous, long-term practice is required before students’ pronunciation skill can become more automatic (i.e. automatization) (DeKeyser, Reference DeKeyser, VanPatten and Williams2007; Foote & Trofimovich, Reference Foote, Trofimovich, Kang, Thomson and Murphy2018; Segalowitz, Reference Segalowitz2010). Hence, the effect of short-term ASR use is limited.
Another related but unexpected finding is that long duration showed a lower effect in segmental pronunciation when compared to medium duration. Referring back to the research design of the primary studies, one noticeable difference is that segmental pronunciation is mostly measured in studies with medium treatment duration (five out of seven numbers of examined effect sizes), while it only constitutes a small proportion in long treatment duration (seven out of 28 numbers of examined effect sizes) studies. In our previous discussion, it is clear that ASR has a larger effect on segmental pronunciation than suprasegmental pronunciation. Therefore, the relatively lower effect found in long duration studies could be attributed to their diverse measurements of pronunciation. On the other hand, medium duration studies mostly focused on measuring segmental pronunciation, which is found to be most effective for ASR use and, therefore, has a larger effect when compared to long duration studies. This interpretation can be further clarified in future research; nevertheless, confirmation of the overall effect sizes from the present meta-analysis indicates that a desirable positive learning outcome can be found in both medium and long duration of ASR usage.
Fourth, regarding learning activity, practicing pronunciation with peers in the ASR condition produces a large effect (g = 0.89), but practicing alone produces only a small effect (g = 0.44). First, in the ASR learning condition, research shows that peers can assist each other in interpreting the ASR feedback and modifying pronunciation errors (Evers & Chen, Reference Evers and Chen2022; Tsai, Reference Tsai2019). Students become more aware of their speech production (Tsai, Reference Tsai2019) and develop a sense of responsibility in accomplishing the task while practicing with peers in this learning condition (Dai & Wu, Reference Dai and Wu2021). Second, in a general computer-based environment, collaboration has also been long promoted as a necessary support for L2 learning (AbuSeileek, Reference AbuSeileek2007; Jones, Reference Jones2006). Therefore, the higher effect observed in the learning activity with peers could be expected since learners receive further benefits from their peers that they do not have when studying alone.
Moreover, practicing with teachers seemed to provide a large effect (g = 1.24), but the overall effect is pooled only from two primary studies; hence, the result cannot be conclusive. Furthermore, teachers usually have limited time for providing individual feedback. Considering other sources of feedback, such as peer feedback, could be more practical. This could be another reason as to why past research did not pay much attention to investigating practicing ASR with teachers.
Fifth, regarding participant age, ASR is more effective in students who are 18 years old and above (g = 1.20) than students who are under 18 years old (g = 0.67). According to Neri et al. (Reference Neri, Mich, Gerosa and Giuliani2008), ASR technology still has errors in recognizing and evaluating non-native speech, and the problem is more prominent in assessing children’s non-native speech as opposed to adult speech. The reason is that children’s speech produces higher variability in acoustic properties, which adds further challenges to the recognition technology. Moreover, many ASR speech models are built based on recordings of adult speech, which might explain why some researchers advocate for the creation of special databases for children’s speech (Elenius & Blomberg, Reference Elenius and Blomberg2005; Gerosa & Giuliani, Reference Gerosa and Giuliani2004). These issues may explain why the effectiveness of ASR is lower in young learners. More studies should be conducted to further develop suitable ASR engines for kids.
Sixth, regarding participant proficiency, ASR is largely effective for students at the intermediate English proficiency level (g = 0.80). According to Mahdi and Al Khateeb (Reference Mahdi and Al Khateeb2019), beginners and intermediate learners can learn foreign language pronunciation faster than advanced learners because they have not suffered from long-time language stabilization. The larger effect on the lower proficiency group could also be explained by the fact that students with lower proficiency have more room for improvement compared to those with higher proficiency who have reached a ceiling (Cucchiarini, Neri & Strik, Reference Cucchiarini, Neri and Strik2009). A meta-analysis with substantial evidence of effect sizes on the effectiveness of pronunciation training by Lee et al. (Reference Lee, Jang and Plonsky2015) also found large effect sizes on the lower proficiency group (e.g. beginner: d = 0.97; intermediate: d = 0.80) in contrast to the negligible effect size observed in the higher proficiency group (e.g. advanced: d = −0.01). In the present meta-analysis, two studies found in the beginner level generated an overall large effect size (g = 1.33), and one study in the advanced level showed a small effect size (g = 0.48). Those results seem to provide further evidence for the larger effect observed in the lower proficiency group, although more investigations targeting beginner and advanced levels are needed for the conclusiveness of their overall effect sizes in ASR use.
6. Pedagogical recommendations
The present study attempts to explore the effectiveness of ASR on ESL/EFL student pronunciation performance. Overall, ASR is moderately more effective in pronunciation learning compared to the non-ASR condition. This provides support for the use of ASR in facilitating students’ pronunciation development. However, the variation in the observed effects suggests that there is still room for ASR to develop. ESL/EFL practitioners could consider the following recommendations for an effective use of ASR.
First, ASR programs with explicit corrective feedback are recommended for use in the class to better support students’ pronunciation development (Hincks, Reference Hincks, Reed and Levis2015; Neri et al., Reference Neri, Cucchiarini and Strik2006; Strik et al., Reference Strik, Neri and Cucchiarini2008). This is also in line with research that found beneficial effects of improving pronunciation with explicit phonetic or pronunciation instruction (Saito, Reference Saito2007, Reference Saito2011, Reference Saito2013). In this explicit learning condition, students are exposed to the model pronunciation and rule presentation on the relevant phonetic characteristics of speech sounds (e.g. place and manner of articulation). ASR programs that can offer the above features could be most efficient for use (e.g. MyET). Notwithstanding, ASR programs with indirect feedback (e.g. Speechnotes) are not able to pinpoint the nature and location of learners’ pronunciation errors. Learners might need to carefully check back and forth to see the differences in the intended message of the transcribed outcomes, thus making it less efficient to pinpoint the errors (Hincks, Reference Hincks, Reed and Levis2015; Neri et al., Reference Neri, Cucchiarini and Strik2006; Strik et al., Reference Strik, Neri and Cucchiarini2008).
Second, ASR largely facilitates segmental pronunciation (see, e.g., Arunsirot, Reference Arunsirot2017; Liu et al., Reference Liu, Zhu, Jiao, Xu, Cheung, Kwok, Kubota, Lee and Tokito2018), but the effect is small in suprasegmental pronunciation (see, e.g., Evers & Chen, Reference Evers and Chen2022; Tsai, Reference Tsai2015). Although ASR is recommended to assist students’ development of segmental pronunciation (i.e. sound units in isolation), the integration of other computer-assisted pronunciation training technologies (e.g. multimedia) can be favorable in facilitating suprasegmental pronunciation (e.g. intonations, stress, rhythm) (Mahdi & Al Khateeb, Reference Mahdi and Al Khateeb2019). Moreover, pronunciation instruction has been supported to target both segmental and suprasegmental features to align with learners’ needs and backgrounds (Kang, Rubin & Pickering, Reference Kang, Rubin and Pickering2010; Lee et al., Reference Lee, Jang and Plonsky2015; Saito, Reference Saito2012). Therefore, L2 practitioners can consider the integration of ASR with other multimedia tools in assisting the pronunciation learning and teaching process.
Third, a medium to long-term use of ASR (e.g. more than four weeks) is encouraged before ASR can show a facilitative effect (see, e.g., Elimat & AbuSeileek, Reference Elimat and AbuSeileek2014; Gorjian et al., Reference Gorjian, Hayati and Pourkhoni2013; Liu et al., Reference Liu, Zhu, Jiao, Xu, Cheung, Kwok, Kubota, Lee and Tokito2018). To illustrate, the training program can be divided into many sessions, one period a day for several periods a week, and over a few months. In other words, a thorough and deliberate training is necessary for students to acquire the pronunciation skills supported by ASR programs. This is in line with DeKeyser’s (2007) skill acquisition theory.
Fourth, designing some peer learning activities in ASR-assisted pronunciation learning is recommended (Evers & Chen, Reference Evers and Chen2021; Tsai, Reference Tsai2019). Students can either work in pairs or in a group of approximately four members (Elimat & AbuSeileek, Reference Elimat and AbuSeileek2014; Evers & Chen, Reference Evers and Chen2021; Tsai, Reference Tsai2019). Peers could help each other in interpreting the feedback from ASR programs, exchange good learning strategies, and evaluate each other’s pronunciation problems. This cooperative learning environment has been shown to largely facilitate students’ learning motivation and outcome (Evers & Chen, Reference Evers and Chen2021; Tsai, Reference Tsai2019). However, caution should be taken if learners are young children who may be more distracted by their group members (Elimat & AbuSeileek, Reference Elimat and AbuSeileek2014).
Lastly, ASR is more effective for students 18 years old and above (see, e.g., Arunsirot, Reference Arunsirot2017; Gorjian et al., Reference Gorjian, Hayati and Pourkhoni2013) with an intermediate level of English proficiency (see, e.g., Gorjian et al., Reference Gorjian, Hayati and Pourkhoni2013; Park, Reference Park2017). Until more special databases for children’s speech are created in the development of ASR technology (Elenius & Blomberg, Reference Elenius and Blomberg2005; Gerosa & Giuliani, Reference Gerosa and Giuliani2004), and more experimental studies are conducted with the beginner and advanced levels, the present ASR programs are more suitable to be applied in a pronunciation learning class with the above groups of learners (i.e. adult, intermediate level).
This study provides several recommendations on the effective use of ASR for the following five aspects: feedback type (explicit corrective), language focus (segmental only), duration of use (medium to long), learning activity (with peer), and learner variables (intermediate, adult). These five recommendations should be valuable for teachers and researchers who want to implement ASR in ESL/EFL pronunciation training.
7. Limitations and future directions
The present study still has several limitations. The most important limitation is the small number of primary studies included in the meta-analysis, which may present some inconclusive findings for the study described here. A second limitation is that the moderator analysis on assessment type was not examined in this study (e.g. the potential moderating effects between the assessments that require a fixed response from all participants, such as read aloud given texts, pronounce given words) and the assessments that allow a variety of different responses from participants (e.g. open-ended measures, conversation, presentation). While this is a potential moderator to be examined in pronunciation instruction or CAPT topics (see, e.g., Lee et al., Reference Lee, Jang and Plonsky2015; Mahdi & Al Khateeb, Reference Mahdi and Al Khateeb2019), the present study in ASR found little evidence available for the latter assessment type (e.g. Evers & Chen, Reference Evers and Chen2021, Reference Evers and Chen2022; Zuberek, Reference Zuberek2016) for which a moderator analysis could not provide much strong findings. Lastly, we did not attempt to investigate the effect of missing data or biases in our study. The reason is that there are currently no official evaluation and guidelines on how to handle missing data and biases in a three-level meta-analysis from available tests such as Egger’s regression, fail-safe N, or the trim-and-fill method (Assink & Wibbelink, Reference Assink and Wibbelink2016; Pigott & Polanin, Reference Pigott and Polanin2020). A future three-level meta-analysis can be more rigorously conducted when a well-performed test for handling missing data and biases is made available.
In spite of the limitations, several possible directions for future research in ASR can be drawn from the present study. First of all, the retention effect of ASR on pronunciation has been scarcely explored in past research. Future research can consider including a delayed post-test design to examine the long-term effects of ASR to develop a more comprehensive understanding of its effectiveness. Second, attending to a variable such as students’ proficiency levels (beginners and advanced English learners) can be beneficial for ASR-related studies that have largely not been investigated before. Third, a meaningful direction for future meta-analyses or empirical studies on pronunciation learning is the examination of the moderating effect of linguistic-related properties (e.g. phoneme complexity, vowel reduction) on students’ pronunciation performance, as these are important factors that may affect students’ pronunciation learning (Celdrán & Elvira-García, Reference Celdrán, Elvira-García and Rao2019; Hoetjes & van Maastricht, Reference Hoetjes and van Maastricht2020). The present meta-analysis was unable to examine these linguistic-related properties because the report on target words used during treatment was often not available in the primary studies. Lastly, the assessment and development of children’s non-native speech in the current ASR technology has not been highly effective. Developing ASR models based on special databases from recordings of children’s speech is thus essential (Elenius & Blomberg, Reference Elenius and Blomberg2005; Gerosa & Giuliani, Reference Gerosa and Giuliani2004). In addition, the development of suprasegmental features in pronunciation may be inadequate. However, there are currently no feasible methods for the improvement of suprasegmental features in ASR (Isaacs, Reference Isaacs, Kang, Thomson and Murphy2018). Researchers in developing ASR technology can consider these issues and modify the programs in the near future.
Supplementary material
For supplementary material accompanying this paper visit https://doi.org/10.1017/S0958344023000113
Acknowledgements
This work was supported by the Ministry of Science and Technology in Taiwan under Grant MOST110-2923-H-003-002-MY2. The authors wish to acknowledge the anonymous reviewers for their critical reviews that helped improve the manuscript.
Ethical statement and competing interests
This study does not involve intervention or interaction with human participants. All the individual studies collected for the research can be accessed online. The authors declare no competing interests.
About the authors
Thuy Thi-Nhu Ngo is a doctoral student of the English Department at National Taiwan Normal University, Taipei, Taiwan. Her research interests include computer-assisted language learning and meta-analysis.
Howard Hao-Jan Chen is a distinguished professor of the English Department at National Taiwan Normal University, Taipei, Taiwan. Professor Chen has published several papers in Computer Assisted Language Learning, ReCALL, and several related language learning journals. His research interests include computer-assisted language learning, corpus research, and second language acquisition.
Kyle Kuo-Wei Lai is a doctoral student of the English Department at National Taiwan Normal University, Taipei, Taiwan. His research interests include computer-assisted language learning and digital game-based language learning.
Author ORCIDs
Thuy Thi-Nhu Ngo, https://orcid.org/0000-0002-6722-1188
Howard Hao-Jan Chen, https://orcid.org/0000-0002-8943-5689
Kyle Kuo-Wei Lai, https://orcid.org/0000-0001-9156-6744