Paediatric obesity is a serious public health problem in the USA and throughout the world( Reference Afshin, Forouzanfar and Reitsma 1 ). In 2011–2012, 8·1 % of US children from birth to 2 years of age had high weight for recumbent length (weight-for-length ≥95th percentile) and in 2011–2014, 8·9 % of children aged 2–5 years were obese (BMI ≥95th percentile)( Reference Ogden, Carroll and Kit 2 , Reference Ogden, Carroll and Lawman 3 ). Obesity rates are significantly higher for older children and excess weight gained in the first 5 years is often maintained throughout life( Reference Gardner, Hosking and Metcalf 4 , Reference Cunningham, Kramer and Narayan 5 ). Worldwide in 2014, there were an estimated 41 million obese or overweight children under the age of 5 years( 6 ). Although the prevalence of overweight children under 5 years was highest in the more developed European regions, the majority of these overweight children live in low-income or middle-income countries. There is an urgent need for obesity prevention programmes targeting young children, yet there is limited evidence about home environment conditions associated with excessive weight gain.
Childhood eating habits are an important predictor of childhood obesity. Young children rely on caregivers to provide nutritious foods and to model healthy eating habits. Based on national recommendations, children aged 2–3 years should consume one cup of fruits and one cup of vegetables per day( 7 ), but many toddlers do not reach the recommended amounts( Reference Siega-Riz, Deming and Reidy 8 ). In addition to introducing more nutrient-dense foods into toddlers’ diets, recommendations are for caregivers to reduce unhealthy food and drink consumption to ensure that toddlers are maintaining a healthy weight and receiving proper nutrition. One type of unhealthy drink to be avoided is sugar-sweetened beverages (SSB); higher consumption of SSB is associated with higher BMI among young children( Reference DeBoer, Scharf and Demmer 9 ). The selection of healthy foods at meals and snacks is important for maintaining a healthy BMI among toddlers. Snacking is a normal part of the toddler diet, but often an opportunity for nutrient-poor options( 10 ). US dietary recommendations are consistent with recommendations from the British Nutrition Foundation (five toddler-sized portions of fruits and vegetables daily in addition to starches, dairy and protein)( 11 ) and the WHO( 6 ).
The Conceptual Model for Eating and Physical Activity Environmental Influences in the Home emphasizes that both the physical environment (food availability and food accessibility) and the social environment (parental role-modelling of healthy eating and parental policies around eating) can influence children’s eating habits( Reference Gattshall, Shoup and Marshall 12 ). This conceptual model serves as our framework for analysing relationships between the home environment and toddler diet.
Factors in the home environment that correlate with toddlers’ diet include electronic entertainment usage and meal setting. Mealtime television (TV) viewing has been associated with unhealthy food consumption among both mothers and toddlers( Reference Horodynski, Stommel and Brophy-Herb 13 ). A study of children in the UK showed that eating at a table, eating with the TV off and eating in a structured setting outside the home all improved the likelihood of fruit and vegetable consumption( Reference Mak, Prynne and Cole 14 ).
Previous studies have relied on maternal recall of toddler diet and home environment, subjecting the findings to recall bias. There is a need for real-time methods that do not rely on recall to collect information about toddler diet and the contextual cues associated with dietary behaviours. Ecological momentary assessment (EMA) is a method of real-time data collection in which study participants report their behaviour and environment at multiple time points, therefore eliminating recall bias. Using EMA to evaluate toddlers’ diet is a novel approach that can provide more information on the context of food intake behaviours. In one study of 642 children aged 1·5–10 years, caregivers recorded food and beverage intake of their child in real-time food diaries along with the eating context. This suggests that employing incident-based data collection focusing on food and context, as reported by a caregiver, is feasible with young children( Reference Mak, Prynne and Cole 14 ). Furthermore, EMA has also been used to study relationships between technology use and food cravings in adolescents( Reference Borgogna, Lockhart and Grenard 15 ). In addition to food diaries, EMA data have been collected using study-provided smart phones or palmtop computers. Multiple studies have used these electronic methods to collect data so as to analyse environmental factors associated with snacking or overeating among adult women( Reference Zenk, Horoi and McDonald 16 – Reference Elliston, Ferguson and Schüz 18 ). The use of EMA in a toddler population, with parents providing a proxy report, may provide important information on home environment factors associated with diet among very young children, which can inform future interventions aimed at improving toddler diet.
The present study aimed to identify factors in the home environment associated with toddler diet using real-time data collection methods. We hypothesize that, during eating and drinking episodes, toddlers are more likely to consume fruits and/or vegetables and less likely to consume SSB and snacks when: (i) eating with another individual (child or adult) compared with eating alone; (ii) interacting with their mother compared with not interacting; (iii) sitting in a high chair/chair at the table compared with walking around, sitting on someone’s lap, or another location; (iv) sitting or standing, compared with moving around; and (v) the TV is off compared with when the TV is on.
Methods
Participants
Biological mother–toddler dyads (n 277) were recruited to participate in the Toddler Overweight Prevention Study (TOPS). Baseline data from TOPS were used in the present study. Study participants were recruited from a Special Supplemental Nutrition Program for Women, Infants, and Children (WIC) clinic in a suburban location and from a paediatric clinic in an urban location. Mothers were recruited either in-person in the waiting room by study staff or through fliers and brochures disseminated at the clinic locations. Upon expressing interest in the study, mothers and toddlers were screened for eligibility (in-person or by telephone). Eligibility criteria included, for toddlers, age 12–32 months, born at term, birth weight >2500 g and able to walk independently, and for mothers, over 18 years of age, not currently pregnant, and no known health problems or disabilities that would impede ability to engage in physical activity. Eligible mothers provided written informed consent. Institutional review boards from both the university and the state Department of Health approved the study. Baseline data were collected over two study visits that took place either in the respondent’s home or at a location in the community (WIC clinic, local community centre or our laboratory) approximately one week apart, year-round from 2007 to 2010.
Measures
Ecological momentary assessment
During the first study visit, the mothers were given a personal digital assistant (PDA; Palm Z22), which beeped fifty-eight times over ≤8 d. The PDA beeped up to eight times per day between 08.30 and 20.30 hours; mothers did not know the beep schedule. Following a beep from the PDA, mothers were asked to complete an EMA questionnaire related to the mother and child’s environment, diet and physical activity. EMA responses submitted within 15 min of the beep were retained. The second study visit was scheduled about 8 d after the first visit and the PDA was returned at this time.
Each EMA questionnaire contained up to twenty-one items. Questions and responses from the EMA questionnaire that focused on diet and the home environment are summarized in Tables 1 and 2. Questions related to the home environment were chosen based on the existing literature about feeding and physical activity( Reference Horodynski, Stommel and Brophy-Herb 13 , Reference Hurley, Cross and Hughes 19 – Reference Dennison, Erb and Jenkins 23 ). Questions specific to the eating context were asked only if the child was eating at the time of the beep. Only data recorded when the child was eating or drinking were included in the current analyses.
Table 1 Questions and responses from the EMA questionnaire focusing on toddler diet and the proportion of dependent EMA responses among low-income mother–toddler dyads (n 249), urban and suburban Maryland, USA, 2007–2010
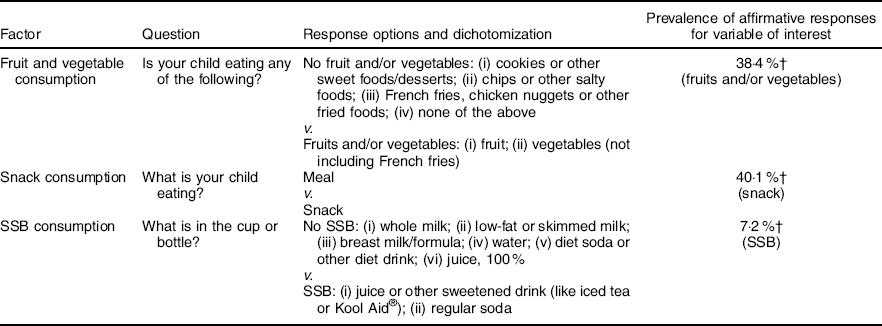
EMA, ecological momentary assessment; SSB, sugar-sweetened beverages.
† n 883 eating episodes and n 1586 drinking episodes.
Table 2 Questions and responses from the EMA questionnaire focusing on the home environment and the proportion of independent EMA responses among low-income mother–toddler dyads (n 249), urban and suburban Maryland, USA, 2007–2010

EMA, ecological momentary assessment; TV, television.
A detailed description of EMA compliance in the study, overall and by time of day, has been given elsewhere( Reference Hager, Tilton and Wang 24 ). The possible impact of maternal compliance (number of prompts answered out of maximum possible) on the study findings was examined by conducting a sensitivity analysis and adjusting for compliance in all regression models.
Dietary behaviours
The variables of fruit and/or vegetable consumption (combined to maximize the number of responses for analysis), snacking and SSB consumption obtained from the EMA questionnaire were binary. If the child was eating at the time of the beep, mothers were asked if the child was eating (i) fruit, (ii) vegetables (not including French fries), (iii) cookies or other sweet foods/desserts, (iv) chips or other salty foods, (v) French fries, chicken nuggets or other fried foods, or (vi) none of the above. Mothers could select all that apply. Choices (i) and (ii) were merged to create a variable representing ‘fruit and/or vegetable’ (first outcome variable). We chose to extract specific dietary behaviours from the list, even though an eating episode may have included more than one food (i.e. fruit and chips), to examine the context of the consumption of each food separately. During eating episodes, mothers were also asked if their child was eating a meal or a snack, and ‘snacking’ was selected as the second outcome variable. The third outcome variable was SSB consumption. If the child had a cup or bottle within reach, mothers were asked if the cup or bottle contained (i) whole milk, (ii) low-fat or skimmed milk, (iii) breast milk/formula, (iv) 100 % juice, (v) juice or other sweetened drink (like iced tea or Kool Aid®), (vi) regular soda, (vii) diet soda or other diet drink, or (viii) water. Choices (v) and (vi) were merged to create a variable representing ‘SSB’ (third outcome variable).
Home environment
The variables representing the home environment were obtained using the EMA questionnaire. ‘Interaction with mother’ was based on the question ‘Are you talking, singing, reading or playing with your toddler?’ ‘Movement/translocation’ was derived from responses that included walking, running or climbing at the time of the EMA beep, while a response of sitting or standing was considered ‘not moving’. These variables were dichotomized and used as binary outcome variables. Other binary home environment variables included TV on/off, eating in a high chair or chair at a table v. other, eating with another child or adult v. alone, and snacking v. eating a meal.
Anthropometrics
Toddler weight was measured in kilograms using a TANITA 1584 Baby Scale (TANITA, Tokyo, Japan), with the toddler in a clean diaper or underpants. Recumbent length was measured in centimetres using a Shorr Measuring Board (Shorr Productions, Olney, MD, USA). Gender-specific weight-for-length percentiles were generated based on the Centers for Disease Control and Prevention growth charts( Reference Grummer-Strawn, Reinold and Krebs 25 ). Obesity was defined as weight-for-length ≥95th percentile, and overweight was defined as weight-for-length ≥85th and <95th percentile( Reference Grummer-Strawn, Reinold and Krebs 25 ).
Demographics
Mothers reported their date of birth, marital status, education, employment status, number of household members and annual household income. They also reported their toddler’s date of birth, gender and race/ethnicity. Poverty status was calculated based on thresholds from the US Census Bureau in 2009( 26 ).
Statistical analysis
Analyses were performed using the statistical software package IBM SPSS Statistics version 22. Demographic information and EMA responses were summarized using simple proportions or means with standard deviations. Multilevel logistic regression models were used to examine associations of fruit/vegetable consumption, snacking and SSB consumption with the demographic characteristic. To examine the temporal relationship between home environment and dietary behaviours, we conducted a series of mixed-effects logistic regression models with random intercepts. These models were utilized to account for the clustering resulting from repeated measures within the same subjects, at multiple time points. Within- and between-subject findings were analysed to disentangle effects( Reference Kanning, Ebner-Priemer and Schlicht 27 ). Within-subject findings represent the relationship between home environment and dietary behaviours for an individual toddler over time (i.e. is toddler A more likely to eat snack when TV is on v. off), whereas between-subject findings represent the relationship between the home environment and dietary behaviours across toddlers (i.e. are toddlers more likely to eat snacks on average when TV is on v. off). All hypothesized home environment factors (independent variables) are time-varying. To disaggregate the within-subject and between-subject relationships, we used the group-mean centring method. Specifically, we included both the (i) person-centred mean and (ii) time-specific deviation from the person-centred mean of the time-varying predictors in the models( Reference Enders and Tofighi 28 , Reference Curran and Bauer 29 ). The mixed-effects logistic regression models were conducted separately for each outcome variable, adjusting for covariates. Statistical significance was rated at α<0·05.
A sensitivity analysis was conducted to examine if findings were driven by those with low maternal compliance rates. For this, all final analyses were conducted among a sample that excluded those with low compliance (responding to ≤10 beeps, approximately one-third of the sample). A side-by-side comparison of the direction and significance (set at P≤0·10, given the reduced sample size) of the regression coefficients was conducted to determine differences. In addition, the moderating effect of low compliance was examined by including interactions between compliance and the independent variables in relation to the outcomes of interest in the final models.
Results
Sample size
Of the 277 mother–toddler dyads recruited into the study, 259 provided at least one valid EMA response when with their toddler (assuming fifty-three prompts/mother, maximum possible prompts=13 727), with mothers responding 4924 times (35·9 % compliance). Of these, mothers answered for their toddlers on 3526 occasions.
Among mothers with EMA data, 249 provided at least one EMA response while the toddler was eating or drinking, yielding 1733 eating or drinking episodes (883 eating, 225 unique subjects and 1586 drinking, 240 unique subjects). Most eating/drinking EMA responses were collected on weekdays (73·9 %) compared with weekend days (26·1 %). About one-quarter of eating/drinking responses were collected in the morning (before 12.00 hours, 26·5 %), along with one-quarter in the afternoon (from 12.00 to 16.00 hours, 24·5 %), with nearly half of the responses collected in the evening (after 16.00 hours, 49·0 %).
Baseline demographic information
The sample characteristics for the 249 mother–toddler dyads are described in Table 3. The mean age of the participating toddlers was 20·0 months, 51·0 % were male, 67·9 % were Black and 18·4 % were overweight or obese. The mothers had a mean age of 27·1 years and 71·1 % were unmarried. The majority of the participants were living at or below the poverty line (67·5 %) and nearly all families (88·9 %) were living below 185 % of the federal poverty level (the threshold used for WIC eligibility( 30 )). Most participants were recruited from the urban location (59·4 %). On average, there were 2·4 children living in each of the households.
Table 3 Demographics of the sample of low-income mother–toddler dyads (n 249), urban and suburban Maryland, USA, 2007–2010
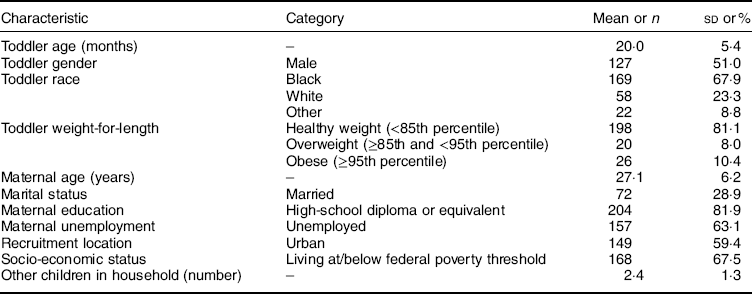
Toddler diet
During the 883 toddler eating episodes, the toddler was eating a fruit and/or vegetable 38·4 % of the time (n 339). More specifically, toddlers were eating fruit on 224 occasions, vegetables only on 165 occasions, and both fruits and vegetables on fifty occasions. Toddlers were eating a snack 40·1 % of the time (n354). During 34·5 % of snacking episodes, toddlers were consuming a fruit or vegetable. Among the 1586 toddler drinking episodes, the toddler was drinking an SSB in 7·2 % of these episodes (n 117). Specifically, mothers reported that their toddlers were drinking a sweetened drink (e.g. Kool-Aid) ninety-eight times and regular soda nineteen times. The toddlers were most likely to be drinking 100 % juice (31·1 %), water (28·2 %) or whole milk (22·9 %) when the EMA beeped. The proportions of responses are reported in Table 1.
Bivariate analyses
The demographic factors associated with increased odds of fruit and vegetable consumption were White race (v. Black: OR=2·08, P<0·001), older maternal age (OR=1·04, P=0·012), the mother being married (OR=1·79, P=0·002), living above the poverty line (OR=2·10, P<0·001) and living in a suburban location (v. urban: OR=1·55, P=0·016). In addition, mothers were more likely to report fruit and/or vegetable consumption in the evening compared with the morning (OR=1·50, P=0·013). The demographic factors associated with higher odds of SSB consumption were older toddler age (OR=1·06, P=0·021), Black race (v. White: OR=2·55, P=0·003), living at or below the poverty line (OR=2·23, P=0·014), living in an urban location (v. suburban: OR=2·77, P<0·001) and increased number of children in the household (OR=1·29, P=0·014). In addition, lower odds of SSB consumption were observed among toddlers with older mothers (OR=0·95, P=0·049) and married mothers (OR=0·55, P=0·047). None of the observed demographic factors had a significant association with snacking; however, snacking behaviours were more likely to be reported in the afternoon v. the morning (OR=1·71, P=0·006). Thus, toddler age, toddler race, maternal age, marital status, poverty, location (urban v. suburban), number of children in the household and time of day were chosen as covariates in regression models. Toddler gender and toddler weight-for-length category (obese, overweight or normal) were not significantly associated with the toddler dietary variables of interest and were not used as covariates in the final models. Day of the week was not independently associated with the outcome variables of interest; however, it was retained in the final models to fully account for the timing of the EMA responses.
Associations between home environment and diet
Fruit and/or vegetable consumption
During eating episodes, mothers reported that their toddlers were less likely, on average, to be eating a fruit or vegetable when walking, running or climbing (adjusted OR (aOR)=0·43, 95 % CI 0·19, 0·97, P=0·043) or watching TV (aOR=0·33, 95 % CI 0·18, 0·59, P<0·001) and were more likely to be eating a fruit or vegetable when eating in a chair at the table or a high chair (aOR=3·38, 95 % CI 1·78, 6·41, P<0·001; between-subject effects). No home environment factor had a significant within-subject relationship with fruit/vegetable consumption (P>0·05), indicating that for an individual toddler, variations in the home environment factors examined were not related to fruit or vegetable consumption. The findings are reported in Table 4.
Table 4 Toddlers’ consumption of fruits and/or vegetables in relation to the home environment among low-income mother–toddler dyads (n 225), urban and suburban Maryland, USA, 2007–2010
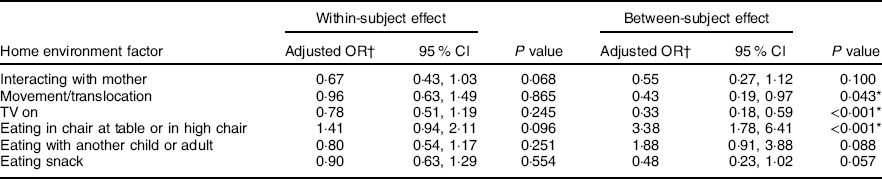
TV, television.
* Denotes a significant P value (P<0·05).
† Logistic mixed-effects regression models, adjusting for toddler age, toddler race, maternal age, marital status, poverty, recruitment location, the number of children in the household, time of day, day of week and compliance (number of responses).
Snack consumption
Both between- and within-subject effects revealed that toddlers on average and individual toddlers across time were less likely to be eating a snack (v. a meal) when eating in a chair at the table/in a high chair (aOR=0·13, 95 % CI 0·07, 0·23, P<0·001 and aOR=0·06, 95 % CI 0·04, 0·09, P<0·001, respectively) or eating with another child or adult (aOR=0·30, 95 % CI 0·17, 0·51, P<0·001 and aOR=0·31, 95 % CI 0·21, 0·45, P<0·001, respectively). An individual toddler was more likely to be eating a snack (v. a meal) when walking, running or climbing (aOR=3·61, 95 % CI 2·40, 5·41, P<0·001), based on within-subject findings only. These findings are reported in Table 5.
Table 5 Toddlers’ consumption of snacks in relation to the home environment among low-income mother–toddler dyads (n 225), urban and suburban Maryland, USA, 2007–2010

TV, television.
* Denotes a significant P value (P<0·05).
† Logistic mixed-effects regression models, adjusting for toddler age, toddler race, maternal age, marital status, poverty, recruitment location, the number of children in the household, time of day, day of week and compliance (number of responses).
Consumption of sugar-sweetened beverages
During times when mothers reported that their toddlers were drinking, toddlers were less likely, on average, to be drinking an SSB when in a chair at the table/in a highchair (v. not: aOR=0·21, 95 % CI 0·09, 0·50, P=0·001) and when with other children or adults (aOR=0·38, 95 % CI 0·14, 0·99, P=0·47), and were more likely to be drinking an SSB during a snack v. a meal (aOR=3·96, 95 % CI 1·35, 11·62, P=0·012), based on between-subject findings. For an individual toddler, variations in home environment were not significantly related to SSB consumption (within-subject findings). Results for SSB consumption in relation to home environment factors can be viewed in Table 6.
Table 6 Toddlers’ consumption of sugar-sweetened beverages in relation to the home environment among low-income mother–toddler dyads (n 240), urban and suburban Maryland, USA, 2007–2010
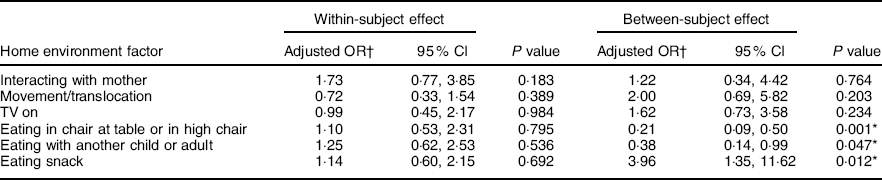
TV, television.
* Denotes a significant P value (P<0·05).
† Logistic mixed-effects regression models, adjusting for toddler age, toddler race, maternal age, marital status, poverty, recruitment location, the number of children in the household, time of day, day of week and compliance (number of responses).
Sensitivity analysis
Overall, seventy-five out of 261 (28·7 %) mothers responded to ten or fewer beeps, which was considered low compliance. When removing these participants from the data set, the direction and significance of the findings outlined above did not differ. In addition, the interaction term that included low compliance as a moderator was not significant in any model. Taken together, these findings suggest that low compliance did not impact the primary findings of the paper.
Discussion
In the present study, there were multiple findings regarding the connection between contextual factors in the home environment and toddler diet. Findings align with the Conceptual Model for Eating and Physical Activity Environmental Influences in the Home, which posits that both the physical and the social environment in the home influence a child’s eating habits( Reference Gattshall, Shoup and Marshall 12 ). With reference to eating location, toddlers ate more fruits and/or vegetables, fewer snacks and drank less SSB while sitting in a high chair or a chair at the table, compared with another location. For movement and translocation, toddlers on average consumed less fruit/vegetables when they were moving around. An individual toddler was more likely to consume snacks in motion compared with sitting or standing. Watching TV was associated with toddlers consuming fewer fruit/vegetables on average, although TV watching was not significantly associated with snacking. Odds of SSB consumption were higher when a toddler was snacking v. eating a meal. Lastly, eating alone was associated with more snacking, and greater SSB consumption, but was not significantly associated with fruit and vegetable consumption. There were no significant findings regarding toddlers’ diet while they were interacting with their mothers.
An important characteristic of a healthy toddler diet is a high intake of fruits and vegetables. The 2008 Feeding Infants and Toddlers Study showed that toddlers do not eat sufficient amounts of fruits and vegetables. Only about 70 % of toddlers consume vegetables at least once per day and about 90 % consume fruit or juice at least once per day( Reference Siega-Riz, Deming and Reidy 8 ). As we had hypothesized, toddlers in the present study were more likely to eat fruits and/or vegetables when they were sitting in a high chair or a chair at the table and when the TV was off. Additionally, we found that they were less likely to eat fruits and/or vegetables when they were moving around compared with when they were not ambulatory, and toddlers were more likely to consume fruits and/or vegetables in the evening v. the morning. This pattern suggests that eating in a structured setting, such as at the table during a family mealtime, with no competing activities (like TV), may be related to the likelihood of being served fruits and vegetables. Sit-down mealtime could be related to a healthier diet and promote better eating practices( Reference Porter, Shriver and Ramsay 31 ).
Snacking is a substantial part of most toddlers’ diets and about 40 % of the eating moments that we randomly captured using EMA were snacks rather than meals. Snacking was more likely to occur in the afternoon compared with the morning. A substantial portion of a child’s daily nutrients and energy typically comes from snacks, making it important to elucidate the nutritional quality of the snacks and the home environment factors associated with snacking. In the current study, the odds of consuming SSB were higher for snacks v. meals. As hypothesized, toddlers were more likely to eat a snack rather than a meal when they were eating alone and when they were not sitting in a high chair or a chair at a table. Furthermore, an individual toddler was more likely to be consuming a snack, as opposed to a meal, when moving around. This finding is consistent with our hypothesis and may be because mealtime is often more regimented and formal than snack time, requiring a child to be seated at a table or high chair. Contrary to our hypothesis, the odds of snacking did not change significantly depending on whether the TV was on or off. The TV was reported as ‘on’ for approximately half of the reported eating and drinking episodes, although we did not directly ask if children were engaged in TV viewing. The reported responses could include when the TV was on as background noise, in addition to when the participant was watching it; however, the EMA survey used herein does not parse this out.
Consumption of SSB can add extra energy to the diet and has been shown to increase the risk of obesity in young children( Reference Welsh, Cogswell and Rogers 32 ), although the exact link between SSB consumption and childhood obesity is controversial( Reference Morgan 33 ). Data from a nationwide study showed that SSB consumption may increase with toddler age and that the overall rate of SSB consumption among toddlers decreased between 2002 and 2008( Reference Siega-Riz, Deming and Reidy 8 ). In the present study, the toddlers were drinking SSB during 7·3 % of the drinking moments captured by EMA. They were much more likely to be drinking whole milk, 100 % juice or water at the time of the prompt, compared with an SSB. While whole milk and 100 % juice are also sources of energy, they can be a part of a healthy paediatric diet depending on the amount consumed and the child’s age( 34 ). When measuring SSB consumption in comparison to home environment factors, toddlers were less likely to consume SSB when they were eating a meal, at a table or high chair, or when eating with other children/adults, compared with when they were eating a snack, in another location or alone.
Most of the findings on the relationship between home environment factors and toddler diet occurred between subjects, particularly for fruits and/or vegetables and SSB, which is in contrast to our previous findings on home environment and physical activity( Reference Hager, Tilton and Wang 24 ). The previous study found more within-subject effects, compared with between-subject effects, in relation to toddler physical activity and the home environment when assessing physical location (inside/outside), interaction with the mother, being around other children and TV viewing( Reference Hager, Tilton and Wang 24 ). One possible explanation for more between-subject findings with respect to diet may be that dietary behaviours are more stable than physical activity among toddlers. The facets of the toddler diet analysed in the current study may have been influenced more by toddler or maternal food preferences or common characteristics of the mothers, instead of the home environmental factors. For example, if mothers do not buy sugary snacks or SSB, toddlers would not have had the opportunity to eat or drink them, or mothers who are less likely to put toddlers in high chairs may also be more likely to purchase snacks/SSB and less likely to purchase fruit/vegetables, both of which would lead to a greater likelihood of detecting between-subject findings.
The present study’s findings support the importance of both the physical and social environments in the home when considering a toddler’s diet (as described in the Conceptual Model for Eating and Physical Activity Environmental Influences in the Home). Given the cross-sectional design, additional studies are needed to inform potential interventions for future randomized studies. Ultimately, increased understanding of which factors in the home environment promote a healthier diet can shape future paediatric diet recommendations.
The present study also supports the use of EMA as an effective method for collecting real-time paediatric dietary data. Traditional dietary recall methods make it hard to accurately match foods and beverages consumed with the home environment context. Collecting information in real time allows us to avoid recall bias and capture the constantly changing features of the home environment.
The findings herein build on reports by Mak et al. in their analysis of home environment and diet in children aged 1·5–10 years living in the UK( Reference Mak, Prynne and Cole 14 ). The relationships they described between eating at the table with the TV off and increased fruit and vegetable consumption were found in our study of children aged 1–2·5 years living in the USA.
To date, EMA has been used primarily to study snacking in adults and adolescents. The present study is unique in applying EMA methods to study snacking in toddlers. Grenard et al. reported that high-school students were more likely to eat sweet or salty snacks at school, with friends, when they felt lonely or bored, when they had a food or beverage craving, and when exposed to food cues( Reference Grenard, Stacy and Shiffman 35 ). In addition, they found that watching TV was associated with eating sweet but not salty snacks. Borgogna et al. studied relationships between technology use and cravings for sweet or salty snacks and found that triggers for these cravings differed based on the subjects’ ethnicity and gender( Reference Borgogna, Lockhart and Grenard 15 ). In contrast to prior studies, our study focused on a much younger population and looked at all snacking rather than just sweet or salty snacks.
Our study has several limitations. Since we captured and analysed a small fraction of each toddler’s eating and drinking moments during the week, there is a potential for bias. It is not known if the episodes captured by EMA are representative of toddlers’ daily consumption of foods and beverages. There were also several limitations of the EMA survey and approach. For example, we examined snacking without differentiating between healthy and unhealthy snacks. To guide future interventions, it would be useful to identify home environment factors that are specifically related to unhealthy snacking. To ensure brevity of the survey, we were unable to capture the full context of the behaviours included (e.g. we inquired about drinks in a cup or bottle but failed to also include other options like cartons or packaged drinks). We also only asked about TV watching and did not expand to other screen-time opportunities. These data were collected between 2006 and 2010, prior to the common utilization of handheld devices with screens. Future studies should incorporate questions that include other devices with screens. Also, because the EMA schedule prompted data collection between 08.30 and 20.30 hours, breakfast may not have been captured and late-night snacking may have been missed. Other limitations include the choice to combine fruit and vegetable consumption to maximize our sample size in our analysis, which did not allow for an analysis of differences in the context of fruit v. vegetable consumption.
Compliance among mothers in the current study is lower than in prior studies among adolescents( Reference Dunton, Whalen and Jamner 22 , Reference Grenard, Stacy and Shiffman 35 ), college-aged women( Reference Thomas, Doshi and Crosby 17 ) and educated White women( Reference Elliston, Ferguson and Schüz 18 ). Low reporting is a common issue for studies involving low-income populations( Reference Kneipp, Lutz and Means 36 ). To examine whether low compliance introduced a bias, we adjusted for compliance in regression models and conducted a sensitivity analysis. Although there was no evidence of bias, low compliance is a limitation. A recent systematic review stated there is no definitive method for reporting compliance rates in EMA studies( Reference Liao, Skelton and Dunton 37 ). The inconsistency and lack of standardization in reporting makes it difficult to compare the present study with other similar studies. For future EMA studies, standard procedures for reporting participant response would benefit the field.
Finally, over two-thirds of the participants in the study were living at or below the poverty line and nearly all were below the threshold for WIC eligibility. Although this population is at particular risk of childhood obesity and is a prime target for future interventions, replication with samples representing other populations would be necessary before findings could be generalized to children living in other socio-economic statuses.
Conclusions
Using EMA, the present study found that a healthier toddler diet characterized by fruit and vegetable consumption was associated with the TV being off, eating in a high chair or a chair at the table, and not moving around while eating. In addition, toddlers were more likely to snack if they were eating alone, not eating in a high chair/chair at the table and moving around while eating. Reduction in SSB consumption was related with eating in a chair or high chair and when consuming a meal v. a snack in this study population. A greater understanding of the potential triggers in the home environment for dietary behaviours is needed to shape future toddler obesity prevention programmes and inform dietary recommendations.
Acknowledgements
Acknowledgements: The authors acknowledge the TOPS research team, especially Kristen Hurley, Brian Merry, Raquel Arbaiza, Adrienne McGill-Burroughs, Laura Latta and Falguni Patel. They are also grateful to the TOPS participants for their time and dedication. Financial support: Data analysis was supported by the Eunice Kennedy Shriver National Institute of Child Health and Human Development (NICHD) (E.R.H., grant number R03HD073802) and the NICHD–University of Maryland’s Organized Research Effort in Women’s Health BIRCWH K12 Scholar Program (E.R.H., grant number K12HD43489-8). Data collection and parent grant were supported by the US Department of Agriculture (M.M.B, grant number CREES 2005-04808) and the NICHD (M.M.B., grant number R01HD056099). The funding agencies had no role in the design, analysis or writing of this article. Conflict of interest: None. Authorship: E.R.H. conceived the study and worked with A.B., Y.W. and M.M.B. to design the study. K.L.C. and A.B. were the primary authors of the written content. K.L.C., A.B., N.A.T. and Y.W. conducted the statistical analyses and all authors interpreted findings. All authors revised the article for important intellectual content and read/approved the final version. Ethics of human subject participation: This study was conducted according to the guidelines laid down in the Declaration of Helsinki and all procedures involving human subjects/patients were approved by institutional review boards at the University of Maryland School of Medicine and the Maryland State Department of Health. Written informed consent was obtained from all mothers of toddlers.