In the past 30 years, the worldwide prevalence of type 2 diabetes mellitus (T2DM) has increased substantially( Reference Danaei, Finucane and Lu 1 ) with an accompanying decrease in the age of onset, especially in black US women( Reference Everhart, Pettitt and Bennett 2 ). In the SEARCH for Diabetes in Youth Study, the incidence of diabetes mellitus was 24·3 (per 100 000 person-years) in 2002–2003, with T2DM most common in African Americans (19·4 %) and American Indians (49·4 %) aged 15–19 years( Reference Dabelea, Bell and D'Agostino 3 ). Although recent evidence suggests that the increase in childhood obesity might be abating in the USA( Reference Ogden, Carroll and Curtin 4 ) and some parts of Europe( Reference Sundblom, Petzold and Rasmussen 5 , Reference Kipping, Jago and Lawlor 6 ), the prevalence of obesity in childhood still remains high( Reference Han, Lawlor and Kimm 7 ) and is likely to fuel an increase in the prevalence of impaired fasting glucose (IFG) and T2DM in the future. To curb this potential increase, it is necessary that effective means of limiting the number of adolescents who become insulin resistant are identified.
Excess body fat is the primary risk factor for insulin resistance( Reference Pan and Pratt 8 ) and T2DM in youth( Reference Morrison, Glueck and Horn 9 , Reference Franks, Hanson and Knowler 10 ), but other factors have also been hypothesized. Frequent consumption of dietary fibre has been associated with a reduced risk of insulin resistance in two prospective cohorts with young adults( Reference Ludwig, Pereira and Kroenke 11 , Reference Pereira, Jacobs and Van Horn 12 ). For example, one 10-year cohort reported an inverse association between dietary fibre and fasting insulin and 2 h insulin levels after provision of a glucose supplement( Reference Ludwig, Pereira and Kroenke 11 ). A meta-analysis of nine cohort studies has also suggested that fibre from cereals may be particularly important in reducing the risk of T2DM in adults( Reference Schulze, Schulz and Heidemann 13 ). Beneficial effects of diets high in monounsaturated( Reference Parillo, Rivellese and Ciardullo 14 ) and polyunsaturated fats( Reference Karlström, Järvi and Byberg 15 , Reference Salmeron, Hu and Manson 16 ) have also been reported in adults with T2DM. In a 14-year prospective cohort of 84 204 middle-aged women, a 5 % increase in the intake of polyunsaturated fat was associated with a large reduction in the incidence of T2DM (relative risk = 0·63, 95 % CI 0·53, 0·76)Reference Salmeron, Hu and Manson (16) . However, support for the effect of monounsaturated and polyunsaturated fats has not been universal( Reference Garg 17 ), in part due to the inconsistent adjustment for the effect of different types of fats.
Other factors linked to the development of insulin resistance in adults have either not been investigated or very few studies exist in adolescents. For example, the Coronary Artery Risk Development in Young Adults (CARDIA) study reported a graded association between serum cotinine concentrations (a metabolite of exposure to tobacco) and the 15-year incidence of glucose intolerance( Reference Houston 18 ); yet no studies with younger groups exist. Similarly, in a small US study, levels of physical activity over an 8 d period were positively associated with efficient glucose metabolism in an intravenous glucose tolerance test( Reference Thomas, Greene and Ard 19 ); but existing investigations of physical activity as a risk factor have tended to be small( Reference Thomas, Greene and Ard 19 ) or assessed activity levels over short periods( Reference Thomas, Greene and Ard 19 – Reference Jago, Wedderkopp and Kristensen 21 ). It is also important to highlight that these associations have been estimated with incomplete adjustment for their mutual effects. This is important, as many are interrelated (e.g. obesity is associated with high SFA intake( Reference Gillis, Kennedy and Gillis 22 ) and low levels of physical activity( Reference Riddoch, Leary and Ness 23 )) and some foods high in both SFA and PUFA (e.g. margarine) may have opposing effects on insulin resistance( Reference Salmeron, Hu and Manson 16 ).
Against this background of a paucity of data on diet and the development of insulin resistance, we used data from the National Heart, Lung, and Blood Institute Growth and Health Study (NGHS), a 10-year study with adolescent girls. With concentrations of fasting blood glucose and insulin, objective assessments of BMI and tobacco exposure (serum cotinine) and detailed questions on the intake of different fats, fibre, sodium and alcohol, this cohort afforded us the opportunity to examine a range of risk factors for the development of insulin resistance. We sought to identify the independent effects of changes in risk factors, to address some of the weaknesses of the research with adults and provide information on viable targets for prevention in adolescence.
Materials and methods
We conducted secondary analyses of the NGHS. The NGHS was conducted according to the guidelines laid down in the Declaration of Helsinki and all procedures involving human subjects/patients were approved by the Institutional Review Boards at all participating centres (the Children's Medical Center, Cincinnati, OH; Westat, Inc., Rockville, MD; and the University of California, Berkeley, CA). Written informed consent was obtained from all participants and their parents.
A complete description of NGHS procedures, including the measurement of glucose and insulin( Reference Morrison, Glueck and Wang 24 ), BMI( Reference Kimm, Glynn and Obarzanek 25 , Reference Kimm, Obarzanek and Barton 26 ), nutrient intake( Reference Crawford, Obarzanek and Morrison 27 , Reference Obarzanek, Schreiber and Crawford 28 ) and physical activity( Reference Kimm, Glynn and Kriska 29 ), have been previously reported. Fasting blood samples were collected at ages 9 or 10 years (year 1), 16 or 17 years (year 7) and 18 or 19 years (year 10). Race was defined by self-report and eligible participants had to declare themselves as ‘black’ or ‘white’. We restricted our analysis to ages 16–17 years and 18–19 years, as fasting blood samples and cotinine were not assessed at other ages.
The present analyses are based on a sample of girls with complete information on each risk factor (% kJ from SFA, % kJ from MUFA, % kJ from PUFA; % kJ from carbohydrates; grams of: sucrose, starch, soluble fibre, insoluble fibre, sodium, alcohol; physical activity in MET-min/d (MET, metabolic equivalents of task); BMI; cotinine; smoking status), potential confounding variables (age at entry, race, level of parental education, and changes in height and menstrual status) and a fasting blood sample at ages 16–17 and 18–19 years. We used t tests and χ 2 tests to examine differences between continuous and categorical values, respectively, for girls who were and were not included in the final analytical sample.
Of the 2379 participants recruited at age 9–10 years, 1783 (74·9 %) took part at ages 16–17 and 18–19 years when fasting blood samples were collected. Of these, 935 (52·4 %) had fasting blood samples at ages 16–17 and 18–19 years and a concentration of serum cotinine determined at age 16–17 years. A total of 774 girls (43·4 %) provided valid estimates of all risk factors and potential confounders.
Assessment of fasting blood glucose, insulin and insulin resistance
Fasting serum insulin (competitive protein-binding RIA) and glucose concentrations were measured after an overnight fast (≥8 h) at the Endocrine Laboratory of the Michigan Diabetes Research and Training Center (Ann Arbor, MI, USA) at age 16–17 years and at the University of Cincinnati/Children's Medical Center (Cincinnati, OH, USA) at age 18–19 years. Glucose was measured using the glucose oxidase method with the Hitachi 704 chemistry analyser (Roche Diagnostics, Indianapolis, IN, USA). Homeostasis model assessment of insulin resistance (HOMA-IR) was used as an index of insulin resistance( Reference Matthews, Hosker and Rudenski 30 ). HOMA-IR was chosen instead of fasting glucose:insulin or the quantitative insulin sensitivity check index (QUICKI), as HOMA-IR has better sensitivity and specificity in identifying adolescents with insulin sensitivity than these measures( Reference Keskin, Kurtoglu and Kendirci 31 ).
Assessment of obesity
BMI (kg/m2) was derived from measurements of height and weight. Height was measured to the nearest 0·1 cm, using custom-made stadiometers. Weight was measured to the nearest 0·1 kg with calibrated Health-o-meter electronic scales (Sunbeam Products, Inc., Maitland, FL, USA). If the second measurement differed from the first by > 0·5 cm for height and 0·3 kg for weight, the same examiner took a third measurement. The mean of the two or three measurements was used for analysis. Obesity was coded using the International Obesity Taskforce cut-off point at 16–17 years (BMI > 29·43 kg/m2)( Reference Cole, Bellizzi and Flegal 32 ) and the WHO adult standard at 18–19 years (BMI ≥ 30·0 kg/m2)( 33 ). The International Obesity Taskforce criterion was applied, rather than other alternatives (e.g. ≥95th percentile on the US Centers for Disease Control and Prevention growth charts), because it was designed to identify age-appropriate cut-off points which pass through the commonly applied WHO adult standard; thus facilitating comparisons with adult studies.
Assessment of exposure to tobacco
Exposure to tobacco smoke was assessed at age 16–17 years using serum cotinine, a metabolite of nicotine. Each sample was analysed at the American Health Foundation (Valhalla, NY, USA) using a modification of the method by Langone et al.( Reference Langone, Gjika and Van Vunakis 34 , Reference Haley, Axelrad and Tilton 35 ) As cotinine concentrations showed strong negative skewness, exposure was re-coded as 0 (no exposure) v. >0 ng/ml. Information on smoking status (non-smoker or smoker) was also obtained by questionnaire at ages 16–17 and 18–19 years. Self-reports were used to identify if girls had started or stopped smoking by 18–19 years.
Dietary assessment
Nutrient intake was assessed using a food diary on three consecutive days (including one day in the weekend and two weekdays)( Reference Obarzanek, Schreiber and Crawford 28 ). Briefly, each girl was provided with measuring cups, spoons, rulers, a portion-size guide and age-appropriate instructions to record all food and drink, as well as the time of intake. The completed food diaries were reviewed individually by certified dietitians (all within 15 d of being completed) and supplementary information was sought using standard probes to clarify incomplete responses( Reference Obarzanek, Schreiber and Crawford 28 ). Each diary was reviewed in depth by a nutritionist through an interview and any inconsistencies or questionable items were clarified. Default values adapted from the Minnesota Nutrition Coordinating Center were used for missing information on food amounts or preparation methods. To minimize the use of defaults, staff had a notebook of labels and label pictures to help girls describe foods.
Nutrient intake was analysed using the Nutrient Data System software developed by the University of Minnesota( Reference Schakel, Sievert and Buzzard 36 ). Means for macronutrients and dietary components were averaged across the 3 d of diet records for each participant. In a validation study, this 3 d diary had a lower percentage of absolute errors, missing foods (i.e. observed food items not reported) and phantom foods (i.e. reported food items not observed) than a 5 d recall and a 24 h recall when compared with intake recorded unobtrusively, suggesting it provided a valid measure of dietary intake.
Assessment of physical activity, developmental status and other demographic variables
Habitual physical activity was assessed using a 3 d activity diary, in tandem with the 3 d food record( Reference Kimm, Glynn and Kriska 29 ). Mean energy expenditure was represented as the number of minutes engaged in different metabolic equivalent (MET) activities across the day, expressed as MET-min/d. Information on the occurrence of/date of menstrual periods and age was obtained by questionnaire at ages 16–17 and 18–19 years( Reference Kimm, Barton and Obarzanek 37 ). The highest level of parental education was collected at study entry from parents (or guardians) and taken as the maximum level of education achieved by either parent. Maximum parental education was categorized into: high school or less; college (some post high-school education); and college (≥4 years).
Statistical methods
Means and standard deviations were calculated for continuous variables and proportions were used for categorical variables. We used two-sided t tests to examine changes in risk factors between ages 16–17 and 18–19 years and the Wilcoxon signed rank test for changes in continuous variables that were skewed. The associations between risk factors (at age 16–17 years), and changes (Δ) in risk factors (between the ages of 16–17 and 18–19 years), with changes in fasting glucose, fasting insulin and HOMA-IR values were assessed using three multiple linear regression models. Each linear regression model included changes in all risk factors between 16–17 and 18–19 years and baseline values at 16–17 years. Models were also adjusted for the covariates of age, race, changes between 16–17 and 18–19 years in height and menstrual status, and parental education at entry as potential confounding factor. As the NGHS employed a nested design, we used a random-effects model with recruitment centre included as a random effect with robust standard errors to adjust for the effect of clustering.
We compared the distribution of study variables between our analytical sample and those who participated at ages 16–17 and 18–19 years but were excluded due to missing data. Girls in the excluded group were less likely to have parents who had attended ≥4 years of college than those in the analytical sample (32·0 % v. 41·5 %, P < 0·001); they were also less likely to start smoking by 18–19 years (11·5 % v. 16·1 %, P = 0·002); and had a slightly higher intake of MUFA (mean 12·74 (sd 2·97) % v. 12·25 (sd 3·20) %, P = 0·002) and SFA (mean 12·37 (sd 3·17) % v. 11·92 (sd 3·36) %, P = 0·005) as a percentage of energy, but a lower percentage of their energy intake came from carbohydrates (mean 52·72 (sd 8·31) % v. 53·88 (sd 8·88) %, P = 0·007). There were no differences between the groups in glucose, insulin and HOMA-IR levels, or other variables.
We conducted sensitivity analyses to explore the potential effect of excluding participants at age 16–17 or 18–19 years who had a fasting blood glucose ≥100–126 mg/dl indicative of IFG or ≥126 mg/dl as a diagnosis of T2DM( 38 ) (2012 definitions of the American Diabetes Association (ADA)), to focus on the role of these risk factors in prevention. All analyses were conducted using the Stata version 10 statistical software package.
Results
Characteristics of participants at ages 16–17 and 18–19 years are summarized in Tables 1 and 2. At baseline, most girls were around 16 years of age (mean 16·00 (sd 0·56) years), had a BMI in the normal range (mean 23·76 (sd 5·43) kg/m2; using the International Obesity Taskforce criterion) and fasting glucose levels which did not suggest IFG or T2DM (mean 76·58 (sd 17·30) mg/dl; using the 2012 ADA definition). Table 1 shows girls were more likely to become obese than normal weight (6·5 % v. 1·4 %, P < 0·001), start smoking than stop (16·1 % v. 3·4 %, P < 0·001), and all girls had started menstruating by age 18–19 years.
Table 1 Categorical measures in girls with assessments on glucose, insulin and HOMA-IR by modifiable risk factors at ages 16–17 and 18–19 years (n 774), NHLBI Growth and Health Study
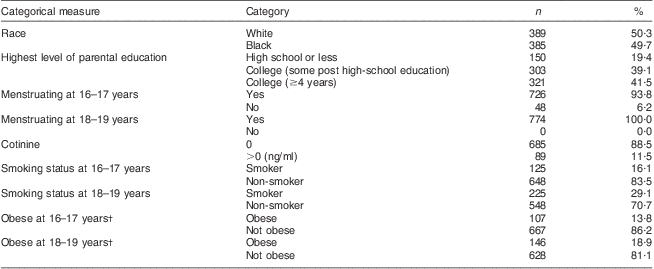
HOMA-IR, homeostasis model assessment of insulin resistance; NHLBI, National Heart, Lung, and Blood Institute.
†Age- and sex-standardized cut-off points of the International Obesity Taskforce applied.
Table 2 Continuous measures in girls with assessments on glucose, insulin and HOMA-IR by modifiable risk factors at ages 16–17 and 18–19 years (n 774), NHLBI Growth and Health Study
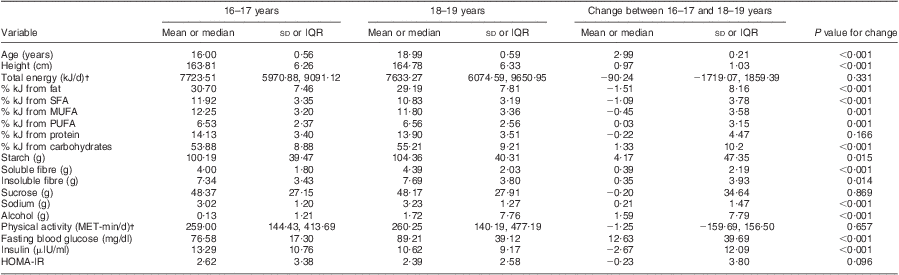
HOMA-IR, homeostasis model assessment of insulin resistance; NHLBI, National Heart, Lung, and Blood Institute; IQR, interquartile range; MET, metabolic equivalents of task.
†Median used for skewed variables.
Table 2 shows that between the ages of 16–17 and 18–19 years, concentrations of fasting blood glucose increased significantly (mean 76·58 (sd 17·30) mg/dl v. 89·21 (sd 39·12) mg/dl, P < 0·001), concentrations of fasting blood insulin decreased significantly (mean 13·29 (sd 10·76) μIU/ml v. 10·62 (sd 9·17) μIU/ml, P < 0·001), and a slight change was found in HOMA-IR values (mean 2·62 (sd 3·38) v. 2·39 (sd 2·58), P = 0·096). The average percentage of energy from fat decreased significantly (specifically % kJ from SFA and MUFA, P = 0·001) and an increase was observed in the percentage of energy from carbohydrates (P < 0·001); grams of soluble fibre, alcohol, sodium (P < 0·001), insoluble fibre (P = 0·014) and starch also increased (P = 0·015).
Table 3 shows that among dietary variables, after adjustment for potential confounders, the % kJ from PUFA at age 16–17 years (β = −0·84, 95 % CI −1·51, −0·18; P < 0·05) and increases between ages 16–17 and 18–19 years in the % kJ from PUFA (β = −0·58, 95 % CI −1·09, −0·07; P < 0·05) and grams of soluble fibre (β = –0·93, 95 % CI −1·73, −0·13; P < 0·05) were inversely associated with a change in fasting blood insulin concentrations between 16–17 and 18–19 years. Replicating these findings, increases in the % kJ from PUFA (β = −3·33, 95 % CI −6·28, −0·39; P < 0·05) and grams of soluble fibre (β = −5·20, 95 % CI −9·81, −0·59; P < 0·05) were also associated with a decrease in HOMA-IR values. The other dietary variables were not associated with changes in insulin resistance at conventional levels.
Table 3 Regression coefficients (95 % confidence intervals) for changes in glucose, insulin and HOMA-IR by modifiable risk factors (assessed at age 16–17 years) and change (Δ) in modifiable risk factors (between the ages of 16–17 and 18–19 years) in girls (n 774), NHLBI Growth and Health Study
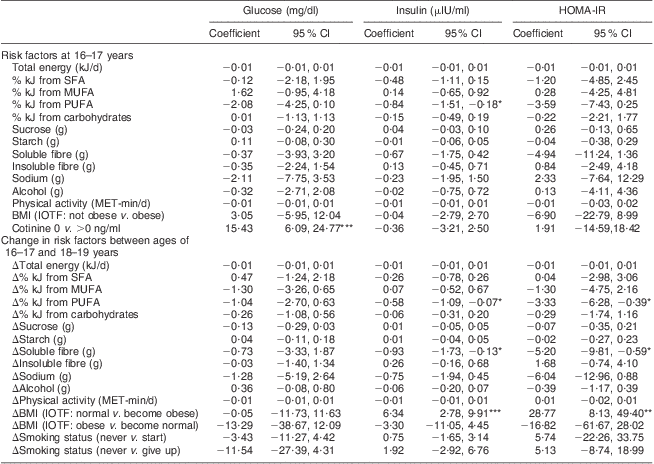
HOMA-IR, homeostasis model assessment of insulin resistance; NHLBI, National Heart, Lung, and Blood Institute; MET, metabolic equivalents of task; IOTF, International Obesity Taskforce (age- and sex-standardized classification applied).
All models are adjusted for the following covariates: age at entry, race, change between the ages of 16–17 and 18–19 years in height and menstrual status, and confounder of parental education.
Significance of coefficient (95 % CI): *P < 0·05, **P < 0·01, ***P < 0·001.
Serum cotinine concentrations at age 16–17 years were associated with a large increase in the concentration of fasting blood glucose between the ages of 16–17 and 18–19 years (β = 15·43, 95 % CI 6·09, 24·77; P < 0·001), whereas changes in smoking status were not. Transitioning over the 3-year follow-up period into obesity was positively associated with a large increase in fasting blood insulin (β = 6·34, 95 % CI 2·78, 9·91; P < 0·001) and HOMA-IR levels (β = 28·77, 95 % CI 8·13, 49·40; P < 0·01). Physical activity was not associated with insulin resistance.
To explore if the results were confounded by the inclusion of girls with pre-existing or developing insulin resistance, we excluded those with IFG (16–17 years: n 6, 0·78 %; 18–19 years: n 40, 5·16 %) or T2DM (16–17 years: n 4, 0·51 %; 18–19 years: n 5, 0·65 %) from the analyses. Following these exclusions, results were very similar with no loss in the strength of effects and slight reduction in precision (data not tabulated); we therefore present the results for the entire analytical cohort.
Discussion
In the present study into the development of insulin resistance in adolescence, improvements in fasting insulin and HOMA-IR levels were associated with increases in the intake of PUFA and soluble fibre, whereas exposure to tobacco smoke was associated with increases in concentrations of fasting glucose, and becoming obese predicted large increases in the concentration of insulin and HOMA-IR. These results are consistent with prospective cohorts which have assessed PUFA intake in middle-aged women( Reference Salmeron, Hu and Manson 16 ) and dietary fibre( Reference Pereira, Jacobs and Van Horn 12 ), obesity( Reference Pan and Pratt 39 ) and tobacco exposure in adults( Reference Houston 18 ). Our findings extend these results by suggesting that these associations operate independently in girls in late adolescence, after adjusting for variations in macronutrient intake, habitual physical activity and pubertal onset. We also showed that these associations were unaltered after excluding girls who had or were developing IFG and T2DM, suggesting these factors may be viable targets for prevention.
Consistent with previous findings, we found that diets high in soluble fibre were associated with improvements in insulin and HOMA-IR levels( Reference Pereira, Jacobs and Van Horn 12 , Reference Pan and Pratt 39 ). In the 10-year follow-up of the CARDIA study, there was an inverse relationship between dietary fibre and the risk of metabolic syndrome (OR = 0·66, 95 % CI 0·53, 0·80)( Reference Pereira, Jacobs and Van Horn 12 ). Our findings add to the growing body of epidemiological data linking high-fibre diets with lower insulin resistance( Reference McKeown, Meigs and Liu 40 , Reference Salmeron, Manson and Stampfer 41 ); although there is a need for randomized evaluation of the effect of these diets in adolescence.
Epidemiological data on the influence of dietary fats on insulin resistance in adolescence are sparse. In a previous analysis of the present cohort, an interaction between the percentage of energy from fat and HOMA-IR values at age 9–10 years was associated with an increased risk of IFG and T2DM at 18–19 years( Reference Morrison, Glueck and Horn 42 ). After adjustment for different types of fats, we found an inverse association between insulin resistance and PUFA intake. This is consistent with the findings from trials among adults with diabetes showing improved insulin resistance was associated with randomization to a diet with a high PUFA:SFA ratio( Reference Heine, Mulder and Popp-Snijders 43 ) or high linoleic acid content( Reference Houtsmuller, van Hal-Ferwerda and Zahn 44 ).
Our finding that increases in insulin and HOMA-IR were associated with becoming obese is consistent with cross-sectional( Reference Pan and Pratt 39 ) and prospective studies( Reference Pan and Pratt 8 , Reference Morrison, Glueck and Horn 42 ). Closely replicating our method of modelling obesity, a recent Israeli cohort found increases in BMI between 17 and 34 years were associated with an increased risk of T2DM, even after adjusting for baseline BMI( Reference Tirosh, Shai and Afek 45 ); and an intervention with adolescent girls demonstrated that a reduction in insulin resistance occurred after weight loss( Reference Nassis, Papantakou and Skenderi 46 ). The data presented here suggest that increases in BMI can adversely affect insulin resistance over shorter periods in adolescent girls.
To our knowledge, only one other prospective study has assessed the association between an objective measure of exposure to tobacco smoke and the development of metabolic risk( Reference Houston 18 ). In the CARDIA study, the 15-year incidence of insulin resistance was 11·5 % in non-smokers not exposed to environmental tobacco smoke, 14·4 % in former smokers, 17·2 % in non-smokers exposed to environmental tobacco smoke and 21·8 % among smokers. Interestingly, adjustment for waist-to-hip ratio did not alter this association, suggesting that this association, if causal, is not moderated by the effect of smoking on fat distribution. Our findings replicate this result in a younger cohort of adolescent girls, and suggest that environmental tobacco smoke is associated with significant increases in fasting blood glucose, after adjusting for changes in smoking and weight status.
Changes in the volume of habitual physical activity were unrelated to insulin resistance. This contrasts with results from a prospective study( Reference Jago, Wedderkopp and Kristensen 21 ), which found small associations between the volume of activity (assessed using accelerometry) and HOMA-IR. That prospective study also showed stronger associations between improvements in insulin resistance and minutes engaged in moderate-to-vigorous physical activity than with overall activity levels( Reference Jago, Wedderkopp and Kristensen 21 ). Our failure to replicate these associations suggests that our self-report measure might have not captured the true volume of activity; but also suggests that moderate-to-vigorous physical activity rather than overall volume of physical activity may be more closely related to changes in insulin resistance.
The use of an objective measure of environmental tobacco smoke exposure, the detailed nutritional profiling and the assessments of change in obesity, smoking status and physical activity, as well as other risk factors for insulin resistance, are major strengths of the present study. There are also some limitations. Cohort attrition and non-participation may have resulted in a less representative sample and participants were not randomly selected, thus the study results cannot be extrapolated to all adolescent girls. Second, we used fasting glucose and insulin values in the HOMA-IR equation to estimate insulin resistance, rather than the more accurate euglycaemic clamp( Reference Schwartz, Jacobs and Moran 47 ). Third, although the 3 d dietary diary was found to be more accurate than a 24 h recall or a 5 d recall( Reference Crawford, Obarzanek and Morrison 27 ), it is unlikely to reflect actual dietary intake as well as a 7 d record.
Conclusion
Our analysis of the NGHS suggests that a number of small changes in lifestyle, namely the adoption of a diet high in soluble fibre and PUFA, in addition to avoidance of excess weight gain and tobacco, could substantially reduce insulin resistance in adolescent girls.
Acknowledgements
Sources of funding: The NGHS was supported by a grant from the National Heart, Lung, and Blood Institute (NHLBI; HL/DK71122). Participating NGHS centres included: the Children's Medical Center, Cincinnati, OH (Stephen R. Daniels, MD, Principal Investigator; John A. Morrison, PhD, Co-Investigator); Westat, Inc., Rockville, MD (George B. Schreiber, ScD, Principal Investigator; Ruth Striegel-Moore, PhD, Co-Investigator); and the University of California, Berkeley, CA (Zak I. Sabry, PhD, Principal Investigator; Patricia B. Crawford, Dr PH, RD, Co-Investigator); the Maryland Medical Research Institute, Baltimore, MD (Bruce A. Barton, PhD, Principal Investigator) served as the data coordinating centre. Program Office: NHLBI (Eva Obarzanek, PhD, RD, Project Officer 1992–present; Gerald H. Payne, MD, Project Officer 1985–1991). The present work was undertaken at the Centre for the Development and Evaluation of Complex Interventions for Public Health Improvement, a United Kingdom Clinical Research Collaboration Public Health Research Centre of Excellence. Funding from the British Heart Foundation, Cancer Research UK, the Economic and Social Research Council (RES-590-28-0005), the Medical Research Council, the Welsh Assembly Government and the Wellcome Trust (WT087640MA), under the auspices of the UK Clinical Research Collaboration, is gratefully acknowledged. The present report is also research arising from a Career Development Fellowship (R.J.) supported by the National Institute for Health Research. The views expressed in this publication are those of the authors and not necessarily those of the UK National Health Service, the National Institute for Health Research or the Department of Health. Conflicts of interest: None of the authors had a conflict of interest. Authors’ contributions: J.W. obtained the NGHS data; J.W. analysed the data; J.W., R.J. and J.L.T. interpreted the data, participated in the writing of the manuscript, and read and approved the final manuscript.