Introduction
Mood disorders, encompassing depressive and bipolar disorder (BD), are prime contributors to the global burden of disease and are associated with a significant reduction in the quality of life of patients (Cramer, Torgersen, & Kringlen, Reference Cramer, Torgersen and Kringlen2010; Frey et al., Reference Frey, Vigod, de Azevedo Cardoso, Librenza-Garcia, Favotto, Perez and Kapczinski2020). This may, in part, be attributed to the cardiometabolic comorbidities to which individuals with mood disorders are predisposed, including type 2 diabetes (T2D), obesity, and metabolic syndrome (MetS) and its components (Qiu et al., Reference Qiu, Cai, Zheng, Qiu, Ke and Huang2021). These metabolic features are independently associated with insulin resistance, which on its own has been associated with more severe depressive symptoms (Singh et al., Reference Singh, Leslie, Packer, Zaiko, Phillips, Weisman and Rasgon2019). This is further reflected in the observation that the prevalence of depression in individuals with diabetes is two to three times greater than in a metabolically healthy population (Roy & Lloyd, Reference Roy and Lloyd2012). This evidence suggests a bidirectional relationship between metabolic dysfunction and mood disorders, such that it has been referred to as a ‘metabolic-mood syndrome’ (Mansur, Brietzke, & McIntyre, Reference Mansur, Brietzke and McIntyre2015).
Metabolic dysfunction and mood disorders have also, independently, been linked to cognitive impairment. Interestingly, these relationships are dynamic, whereby increases in symptom severity distinct to mood and metabolic disorders are associated with greater cognitive decline (Karlsson, Gatz, Arpawong, Dahl Aslan, & Reynolds, Reference Karlsson, Gatz, Arpawong, Dahl Aslan and Reynolds2021; Marvel & Paradiso, Reference Marvel and Paradiso2004). Understanding these interactions is of great importance due to the impact of cognitive function on symptom severity and functioning in individuals with mood disorders (Burdick, Goldberg, & Harrow, Reference Burdick, Goldberg and Harrow2010; Siegel-Ramsay et al., Reference Siegel-Ramsay, Wu, Bond, Spelber, Chiang, Lanza di Scalea and Almeida2022). A recent meta-analysis examined the association between depression and cognition in persons with diabetes mellitus and reported significant impairment in overall cognition, executive function, language, and memory among individuals with comorbid diabetes and depression in comparison to those with only diabetes (Chow, Verdonschot, McEvoy, & Peeters, Reference Chow, Verdonschot, McEvoy and Peeters2022). Another meta-analysis found obese/overweight BD patients to have more severe cognitive deficits v. normal-weight patients, especially in global cognition, executive function, and processing speed domains (Bora, McIntyre, & Ozerdem, Reference Bora, McIntyre and Ozerdem2019). However, there has been no synthesis of the association between metabolic dysfunction and cognitive outcomes in individuals across the mood disorder spectrum including major depressive disorder (MDD).
Therefore, this review sought to broadly explore the association between metabolic dysregulation and global cognition and individual cognitive domains in individuals with mood disorders. It was hypothesized that due to the bidirectional relationship observed between mood and metabolic disorders, and supporting evidence outlining its impact on cognition, that individuals with a mood and metabolic disorder comorbidity would exhibit poorer cognitive performance compared to those with only a mood disorder. Furthermore, exploratory analysis investigated the association between the severity of metabolic dysregulation and cognition.
Methods
This systematic review and meta-analysis was reported following the Preferred Reporting Items for Systematic Reviews and Meta-Analyses (PRISMA) guidelines (Page et al., Reference Page, McKenzie, Bossuyt, Boutron, Hoffmann, Mulrow and Moher2021). The protocol was registered in the International Prospective Register of Systematic Reviews (PROSPERO) with ID: CRD42022295765.
Inclusion criteria
Inclusion criteria were structured with the PICOS framework, as outlined.
(a) Population: Individuals with a diagnosed mood disorder (including depressive disorders and bipolar and related disorders) or those experiencing depressive symptoms as measured through validated scales and/or self-reported depression. Studies of participants with type 1 diabetes and those that were pregnant and/or breastfeeding were excluded to address potential confounding hormonal and genetic interactions with cognitive function. Similarly, studies of populations with predefined cognitive impairment and/or neurocognitive disorders at baseline were excluded.
(b) Intervention/exposure: Comorbid metabolic dysregulation, including MetS and/or at least one of its components such as abdominal obesity, high blood pressure, impaired fasting glucose, high triglycerides, reduced HDL cholesterol levels; T2D; insulin resistance; elevated body mass index (BMI) (overweight/obesity).
(c) Comparator: Individuals with the same mood disorder, but metabolically healthy in relation to the exposure/metabolic variable being studied.
(d) Outcome: Global cognition was explored as a single outcome when reported as an overall or composite score for assessments measuring various cognitive domains or as an average of the individual cognitive domains assessed in the study. The individual cognitive domains included verbal memory, attention, executive function, processing speed, visual memory, and working memory. The method of averaging scores and classifying assessments under distinct cognitive domains was adapted from Bora, Akdede and Alptekin (Reference Bora, Akdede and Alptekin2017) and Bora et al. Reference Bora, McIntyre and Ozerdem2019) reviews (Table S1). Measures of cognitive deficits/failures were not included in the meta-analysis (e.g. scales or self-report of perceived memory deficits) but summarized qualitatively. IQ and measures of crystallized or fluid intelligence were not assessed as cognitive outcomes (Dennis et al., Reference Dennis, Francis, Cirino, Schachar, Barnes and Fletcher2009).
(e) Study design: Randomized control trials, cross-sectional studies, observational studies, longitudinal studies. Longitudinal and intervention studies were excluded if the population and comparator groups were matched for cognitive function at baseline and not representative of the trends being explored.
Search strategy
A comprehensive search, encompassing mood disorder, metabolic dysregulation, and cognitive domains, was carried out in December 2021, and updated in January and September 2023. Six databases were used to conduct this search of published and grey literature: Ovid MEDLINE, APA PsycINFO, Embase, Scopus, Web of Science, and CINAHL. Limits were applied to English language and human studies in Ovid databases, but not to publication date or study design (Table S2). The reference lists of relevant reviews identified through the search were screened for additional articles. The grey literature search was supplemented by searching Google Scholar and contacting corresponding authors of included studies for unpublished data to synthesize in this review.
Study selection
The study selection process was managed using the Covidence software. Any combination of two reviewers involved in each step of the study selection process independently screened titles and abstracts (K. M., N. S., R. D., E. S., F. P., Z. H.), followed by full-text screening (K. M., N. S., J. G., A. H., F. P., Z. H., R. D., E. S.). Any disagreements in the study selection process were resolved through referencing studies and discussion between authors.
Data extraction
Data extraction was completed independently, and in duplicate, to verify the accuracy of information by any combination of two authors involved in data extraction (K. M., N. S., F. P., J. G., A. H., Z. H.). A standardized extraction form was utilized to collect information on study identifiers, population descriptors, and outcomes of interest. Discrepancies in data collection were resolved by referencing the original text and through discussion between authors. If applicable, data were extracted from figures using an online software, WebPlotDigitizer (https://automeris.io/WebPlotDigitizer/). Corresponding authors were contacted for any additional information that was not reported or could not be extracted from the included studies.
Risk of bias assessment
An adapted version of the Newcastle-Ottawa Scale (NOS) for cross-sectional data was utilized to perform a quality assessment of the studies to assess for risk of bias (Modesti et al., Reference Modesti, Reboldi, Cappuccio, Agyemang, Remuzzi, Rapi and Parati2016). Two authors (K. M. and Z. H.) completed these assessments and any differences in ratings were addressed through discussion. A high risk of bias was defined as a rating of equal to or less than four. Furthermore, funnel plots with 10 or greater studies were analyzed for reporting bias and supplemented with the Egger's test performed using Stata 16 (StataCorp. 2019. Stata Statistical Software: Release 16. College Station, TX, USA: StataCorp LLC.).
Data synthesis
Summaries of included studies were tabulated. A random-effects meta-analysis was conducted using Review Manager (RevMan) Version 5.4 by calculating the standardized mean difference (SMD) of continuous data reporting cognitive outcomes. A minimum of two studies were required for a meta-analysis. The effect sizes of individual cognitive domains/assessments used in studies were pooled to calculate global/total cognition when it was not reported as a single score (Bora et al., Reference Bora, Akdede and Alptekin2017, Reference Bora, McIntyre and Ozerdem2019). Only objective measures of cognitive function were included in these analyses. For studies with overlapping populations, only one with the largest population size experiencing the greatest metabolic burden was included in the meta-analysis. Mean effect sizes were multiplied by −1 if higher scores were associated with worse cognitive performance to ensure consistency in reporting the direction of exposure effects. All outcomes of all effect sizes were reported to ensure no reporting bias and completeness of data. Heterogeneity was assessed with the I 2 statistic. A Firepower plot was created to assist in the visualization of the power of effect sizes of cognitive outcomes in RStudio (R Foundation for Statistical Computing, Vienna, Austria, v 4.3.2) using the metameta package (Quintana, Reference Quintana2023).
Sub-analyses
A sensitivity analysis was conducted by removing studies of populations with a reported history of stroke, heart attack, and other comorbid conditions that have an independent association with cognition; outliers in funnel plots with a large effect size; and studies with a high risk of bias. A meta-regression was performed using Stata 16 (StataCorp. 2019. Stata Statistical Software: Release 16. StataCorp LLC.) to assess the association between age, BMI, and percentage of males in the comorbid study population; difference in BMI between the comorbid and control group; and SMD in depression severity between the comorbid and control group with global cognition. If separate characteristics were not provided for the male/female subgroups, the cognitive data for these subgroups was averaged and included in meta-regressions. Furthermore, if the average population BMI was not provided, the average height and weight were used to calculate BMI (kg/m2).
Certainty assessment
The Grading of Recommendations, Assessment, Development and Evaluations (GRADE) framework was used to identify the quality of evidence surrounding the association between metabolic dysregulation and cognition in individuals with mood disorders. Given the observational nature of this data, all evidence was rated starting from a very low level of certainty.
Results
Study selection
A summary of the study selection process is outlined in Fig. 1. A total of 26 708 articles were identified from the search. Following title and abstract screening, 1 970 articles were assessed at the full-text level for eligibility. Sixty-three studies met the pre-specified inclusion criteria and were included in the review.
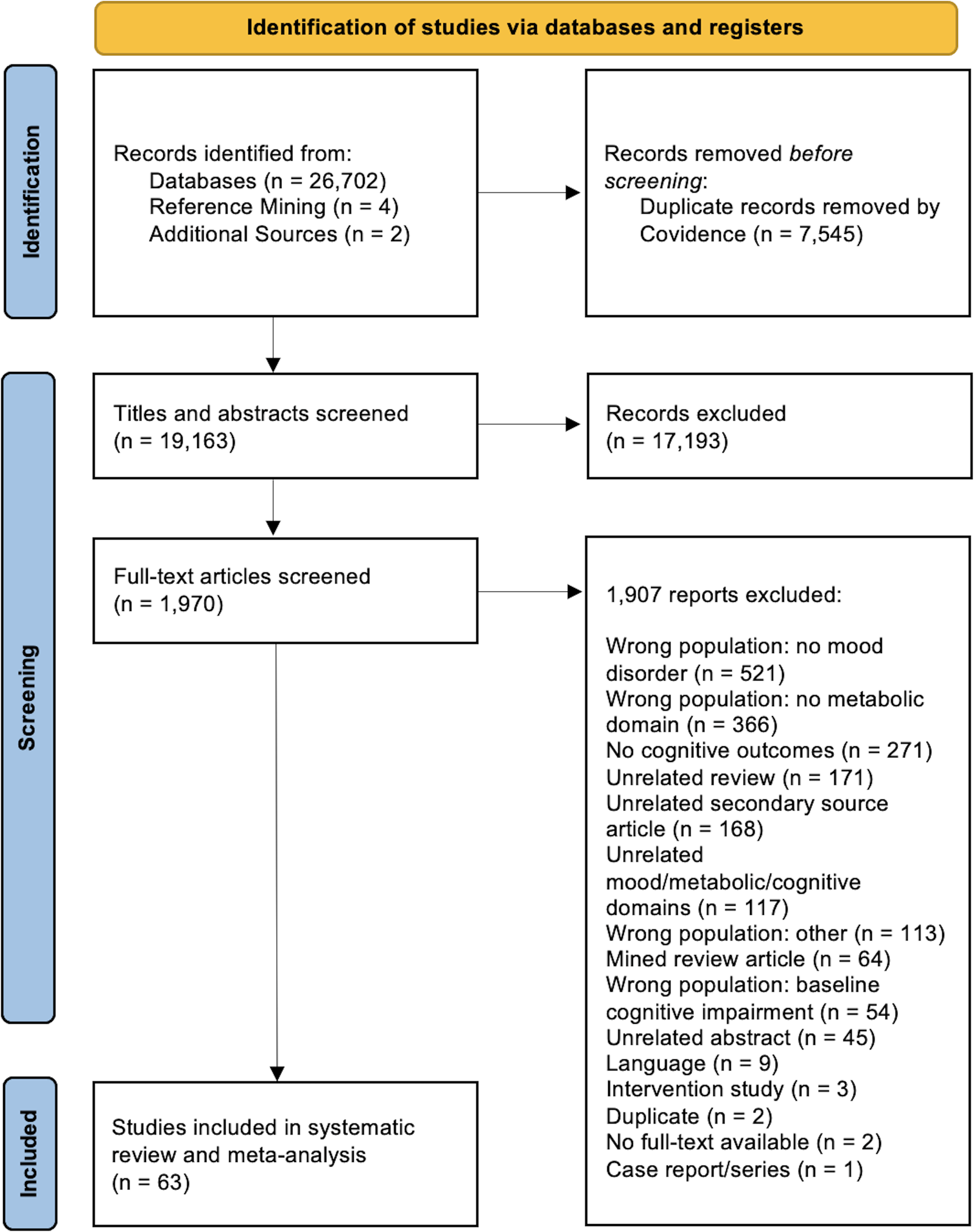
Figure 1. Preferred Reporting Items for Systematic Reviews and Meta-Analyses (PRISMA) flowchart of studies included in the systematic review and meta-analysis.
Study characteristics
The characteristics of included studies are summarized in Table 1. Twenty-seven studies explored the impact of metabolic dysregulation on cognitive outcomes in individuals with BD (Bai et al., Reference Bai, Li, Tsai, Tu, Chen and Su2016; Beunders et al., Reference Beunders, Kemp, Korten, Oudega, Beekman, Kupka and Dols2021; Bond et al., Reference Bond, Torres, Lee, Kozicky, Silveira, Dhanoa and Yatham2017; Chang et al., Reference Chang, Tseng, Chang, Huang, Lu, Yang and Chen2022; Dalkner et al., Reference Dalkner, Bengesser, Birner, Fellendorf, Fleischmann, Großschädl and Reininghaus2021a, Reference Dalkner, Bengesser, Birner, Rieger, Seebauer, Platzer and Reininghaus2021b; Depp et al., Reference Depp, Strassnig, Mausbach, Bowie, Wolyniec, Thornquist and Harvey2014; Hubenak, Tuma, & Bazant, Reference Hubenak, Tuma and Bazant2015; Hui et al., Reference Hui, Yin, Chen, Yin, Zhu, Li and Jia2019; Kadriu et al., Reference Kadriu, Deng, Kraus, Johnston, Fijtman, Henter and Zarate2023; La Montagna et al., Reference La Montagna, Stella, Ricci, Borraccino, Triggiani, Panza and Lozupone2017; Lackner et al., Reference Lackner, Bengesser, Birner, Painold, Fellendorf, Platzer and Reininghaus2016; Li et al., Reference Li, Bai, Hsieh, Lee, Yang, Chen and Su2015; Liebing et al., Reference Liebing, Dalkner, Ischebeck, Bengesser, Birner, Fellendorf and Reininghaus2023; Mehra, Jagota, Sahoo, & Grover, Reference Mehra, Jagota, Sahoo and Grover2022; Mora et al., Reference Mora, Portella, Martinez-Alonso, Teres, Forcada, Vieta and Mur2017; Naiberg, Newton, Collins, Bowie, & Goldstein, Reference Naiberg, Newton, Collins, Bowie and Goldstein2016a; Naiberg et al., Reference Naiberg, Newton, Collins, Dickstein, Bowie and Goldstein2016b; Qiu et al., Reference Qiu, Li, Teng, Tan, Xu, Yang and Wu2022; Reininghaus et al., Reference Reininghaus, Dalkner, Schörkhuber, Fleischmann, Fellendorf, Ratzenhofer and Reininghaus2022; Ringin et al., Reference Ringin, Dunstan, McIntyre, Berk, Owen, Rossell and Van Rheenen2023a, Reference Ringin, Dunstan, Meyer, McIntyre, Owen, Berk and Van Rheenen2023b; Salvi et al., Reference Salvi, Di Salvo, Korčáková, Torriero, Aragno, Kolenič and Hajek2020; Silveira et al., Reference Silveira, Kozicky, Muralidharan, Bücker, Torres, Bond and Yatham2014; Tsai, Lee, Chen, & Huang, Reference Tsai, Lee, Chen and Huang2007; Van Rheenen, McIntyre, Balanzá-Martínez, Berk, & Rossell, Reference Van Rheenen, McIntyre, Balanzá-Martínez, Berk and Rossell2021; Yim et al., Reference Yim, Soczynska, Kennedy, Woldeyohannes, Brietzke and McIntyre2012), 15 in MDD (Cao et al., Reference Cao, Yang, Cheng, Zhang, Shi and Li2023; Fourrier et al., Reference Fourrier, Sampson, Hori, Schubert, Clark, Mills and Baune2020; Geraets et al., Reference Geraets, Schram, Jansen, Backes, Schalkwijk, Stehouwer and Köhler2022; Guan et al., Reference Guan, Hou, Zhu, Cao, Tang, Yin and Hui2021; Hidese et al., Reference Hidese, Ota, Matsuo, Ishida, Hiraishi, Yoshida and Kunugi2018; Kloiber et al., Reference Kloiber, Ising, Reppermund, Horstmann, Dose, Majer and Lucae2007; Kopchak & Pulyk, Reference Kopchak and Pulyk2017; Kraus et al., Reference Kraus, Kautzky, Watzal, Gramser, Kadriu, Deng and Kasper2023; Lan et al., Reference Lan, Wang, Li, Chao, Lao, Wu and Zhou2022; Marijnissen et al., Reference Marijnissen, Vogelzangs, Mulder, van den Brink, Comijs and Oude Voshaar2017; Péterfalvi et al., Reference Péterfalvi, Németh, Herczeg, Tényi, Miseta, Czéh and Simon2019; Shao et al., Reference Shao, Yin, Yin, Wu, Du, Zhu and Hui2017; Smith et al., Reference Smith, Blumenthal, Hinderliter, Watkins, Hoffman and Sherwood2018; Wroolie, Kenna, Singh, & Rasgon, Reference Wroolie, Kenna, Singh and Rasgon2015; Zhang, Wang, Shi, & Li, Reference Zhang, Wang, Shi and Li2021), 17 in participants with measures of depressive symptoms/self-rated depression (Borda et al., Reference Borda, Santacruz, Aarsland, Camargo-Casas, Cano-Gutierrez, Suárez-Monsalve and Pérez-Zepeda2019; Borhaninejad & Saber, Reference Borhaninejad and Saber2022; Chang, Lung, & Yen, Reference Chang, Lung and Yen2015; Chen et al., Reference Chen, Siddarth, Ercoli, Merrill, Torres-Gil and Small2014; Choi et al., Reference Choi, Han, Jeon, Jang, Kim and Park2019; Demakakos, Muniz-Terrera, & Nouwen, Reference Demakakos, Muniz-Terrera and Nouwen2017; Downer, Vickers, Al Snih, Raji, & Markides, Reference Downer, Vickers, Al Snih, Raji and Markides2016; Ferri, Deschênes, Power, & Schmitz, Reference Ferri, Deschênes, Power and Schmitz2021; Janocha et al., Reference Janocha, Bolanowski, Pilecki, Małyszczak, Salomon, Woźniak and Sobieszczańska2010; Jia et al., Reference Jia, Yang, Zhuang, Yin, Zhu, Yuan and Hui2020; Johar, Schaefer, & Su, Reference Johar, Schaefer and Su2023; Kontari & Smith, Reference Kontari and Smith2019; Lin et al., Reference Lin, Jiang, Sun, Zhang, Shan, Zhao and Jiang2022; Liu et al., Reference Liu, Su, Huang, Li, Wei, He and Peng2023; Ng, Niti, Zaw, & Kua, Reference Ng, Niti, Zaw and Kua2009; Scuteri et al., Reference Scuteri, Spazzafumo, Cipriani, Gianni, Corsonello, Cravello and Sebastiani2011; Wei et al., Reference Wei, Ying, Xie, Chandrasekar, Lu, Wang and Li2019), and four in a mixed population of MDD and BD, of which some analyzed data independently for each mood disorder diagnosis (Chen et al., Reference Chen, Hsu, Huang, Tsai, Su, Li and Bai2021; Mansur et al., Reference Mansur, Lee, Subramaniapillai, Cha, Brietzke and McIntyre2020; McIntyre et al., Reference McIntyre, Soczynska, Cha, Woldeyohannes, Dale, Alsuwaidan and Kennedy2015; Zhuo et al., Reference Zhuo, Liu, Jiang, Li, Yu, Chen and Song2022). Specifically, BMI, MetS/comorbid metabolic risk factors, and T2D were studied most frequently in these populations.
Table 1. Summary of studies included in the systematic review

APs, antipsychotics; BACS, Brief Assessment of Cognition in Schizophrenia; BD, bipolar disorder; BMI, body mass index (kg/m2); BP, blood pressure; CANTAB, Cambridge Neuropsychological Test Automated Battery; CES-D, Center for Epidemiologic Studies-Depression; COWAT, Controlled Oral Word Association Test; CPT, Continuous Performance Test; CVLT, California Verbal Learning Test; DSM, Diagnostic and Statistical Manual of Mental Disorders; DSST, Digit Symbol Substitution Test; GDS, Geriatric Depression Scale; HDL, High-Density Lipoprotein; HOMA-IR, Homeostatic Model Assessment for Insulin Resistance; ICD, International Classification of Diseases; IR, insulin resistance; LDL, low-density lipoprotein; MADRS, Montgomery-Åsberg Depression Rating Scale; MDD, major depressive disorder; MetS, metabolic syndrome; MINI, Mini-International Neuropsychiatric Interview; MMSE, Mini Mental State Examination; MoCA, Montreal Cognitive Assessment; NaSSAs, Noradrenergic and Specific Serotonergic Antidepressants; NR, not reported; PHQ, Patient Health Questionnaire; RAVLT, Rey Auditory Verbal Learning Test; RBANS, Repeatable Battery for the Assessment of Neuropsychological Status; RCFT, Rey Complex Figure Test; SD, standard deviation; SNRI, serotonin/norepinephrine reuptake inhibitor; SSRI, selective serotonin reuptake inhibitor; T2D, type 2 diabetes; TCA, tricyclic antidepressants; TG, triglycerides; TMT-A/B, Trail-Making-Test-A/B; WC, waist circumference; WCST, Wisconsin Card Sorting Test; WHtR, waist-to-height ratio; ZVT, Zahlenverbindungstest.
*Included in meta-analysis.
In addition to the variety of mood and metabolic disorder subgroups, study populations ranged from an average of 17.21 (Naiberg et al., Reference Naiberg, Newton, Collins, Bowie and Goldstein2016a, Reference Naiberg, Newton, Collins, Dickstein, Bowie and Goldstein2016b) to ⩾80 years old (Wei et al., Reference Wei, Ying, Xie, Chandrasekar, Lu, Wang and Li2019). A range in the duration of illness was captured, including first-episode patients and those with a more chronic course of illness of up to 34.2 years (Beunders et al., Reference Beunders, Kemp, Korten, Oudega, Beekman, Kupka and Dols2021).
The use of psychotropic medication was reported in nearly all studies. This review included medication-free/naïve populations in the BD (Qiu et al., Reference Qiu, Li, Teng, Tan, Xu, Yang and Wu2022), MDD (Smith et al., Reference Smith, Blumenthal, Hinderliter, Watkins, Hoffman and Sherwood2018), and mixed BD/MDD (Zhuo et al., Reference Zhuo, Liu, Jiang, Li, Yu, Chen and Song2022) subgroups. Medication history was not reported for the depressive symptom/self-reported depression group likely because these were community-based samples.
No studies included in the meta-analysis met the criteria for high risk of bias as per evaluation of the quality of their sample selection, comparability of outcomes, and outcome assessment; however, most did not justify their sample size or outline non-respondents. Two studies identified through the grey literature search were summarized qualitatively and received a high risk of bias assessment as they were abstracts reporting limited information (La Montagna et al., Reference La Montagna, Stella, Ricci, Borraccino, Triggiani, Panza and Lozupone2017; Mehra et al., Reference Mehra, Jagota, Sahoo and Grover2022). A full summary of the study risk of bias is provided in Table S3.
Association between metabolic dysregulation and cognition within different mood disorders (meta-analysis)
Comorbid metabolic dysregulation was associated with significantly lower global cognition in individuals with mood disorders, compared to metabolically healthy individuals with mood disorders (−0.37 SMD, 95% confidence interval [CI] [−0.46 to −0.27], p < 0.00001, I 2 = 80%, n = 6593, k = 26) (Fig. 2). These associations were significant within each mood disorder group, with no significant differences in effect sizes between subgroups (p = 0.86, I 2 = 0%).

Figure 2. Forest plot of the association between metabolic dysregulation with global cognition in individuals with mood disorders.
Association between individual metabolic parameters and cognition across all mood disorder populations
Analysis of the relationship between individual metabolic parameters with cognition (Fig. 3) identified T2D to be associated with the greatest difference in cognitive performance among individuals with mood disorders (−0.56 SMD, 95% CI [−0.84 to −0.27], p = 0.0001, I 2 = 84%, n = 2478, k = 7). This medium effect size was followed by peripheral insulin resistance (−0.46 SMD, 95% CI [−0.62 to −0.30], p < 0.00001, I 2 = 0%, n = 157, k = 2), BMI ⩾25 (−0.35 SMD, 95% CI [−0.48 to −0.21], p < 0.00001, I 2 = 76%, n = 1268, k = 8) and MetS/multiple cardiometabolic risk factors (−0.25 SMD, 95% CI [−0.44 to −0.07], p = 0.006, I 2 = 84%, n = 2349, k = 7). The overall effect of triglycerides and hypertension could not be summarized across studies as these parameters were reported in only one study each. However, separating these populations by sex showed significant effects of high triglyceride levels in females, and hypertension in males (Guan et al., Reference Guan, Hou, Zhu, Cao, Tang, Yin and Hui2021; Ng et al., Reference Ng, Niti, Zaw and Kua2009).

Figure 3. Forest plot of the association between individual metabolic parameters with global cognition in individuals with mood disorders.
Association between metabolic dysregulation and individual cognitive domains across all mood disorder populations
Analyzing these effects further within individual cognitive subdomains demonstrated similar trends (Fig. 4; Fig. S1a–g). Comorbid metabolic dysregulation was associated with worse performance on attention tasks in the pooled mood disorder population (−0.42 SMD, 95% CI [−0.68 to −0.16], p = 0.002, I 2 = 81%, n = 1537, k = 8); however, not among all diagnosis subgroups. Executive function scores were significantly reduced in the pooled comorbid metabolic dysregulation group (−0.40 SMD, 95% CI [−0.53 to −0.27], p < 0.00001, I 2 = 44%, n = 1393, k = 11), and also in the BD and MDD subgroups in which this association was assessed. Similarly, small effect sizes were observed when analyzing processing speed (−0.40 SMD, 95% CI [−0.54 to −0.26], p < 0.00001, I 2 = 50%, n = 2834, k = 10) and verbal memory (−0.29 SMD, 95% CI [−0.43 to −0.14], p = 0.0001, I 2 = 70%, n = 1488, k = 11) performance in both the BD and MDD populations. Visual memory (−0.24 SMD, 95% CI [−0.43 to −0.05], p = 0.01, I 2 = 77%, n = 2735, k = 8) and working memory (−0.47 SMD, 95% CI [−0.63 to −0.30], p < 0.00001, I 2 = 55%, n = 1144, k = 9) scores were also lower in the pooled comorbid population, but not in all diagnosis subgroups. Furthermore, cognitive outcomes that were reported using mixed methods, such as by response time or performance score, showed similar small effect sizes highlighting worse performance in attention, executive function, and processing speed domains independent of the reporting method (Fig. S2a–f). The power of these associations is visually represented in Fig. S3.

Figure 4. Summary of the association between metabolic dysregulation and different cognitive domains in individuals with mood disorders.
Examining the association between other clinical variables and cognition using meta-regression
No significant association was discovered between the average age (β = 0.002; p = 0.704), percentage of males (β = 0.001; p = 0.816), or BMI (β = −0.004; p = 0.806) of the comorbid metabolic dysregulation group and global cognition. Due to significant heterogeneity between the clinical scales used to measure depression severity, the SMD was calculated to assess any differences between the comorbid and mood disorder only group, and no significant difference was found (0.08 SMD, 95% CI [−0.02 to 0.18], p = 0.12, I 2 = 14%, n = 2387, k = 13). Consequently, there were no significant trends between depression severity and cognitive outcomes (β = −0.062; p = 0.847). Similarly, the difference in BMI between the comorbid and mood disorder only group was not associated with the effect size of metabolic dysfunction on cognitive outcomes (β = −0.001; p = 0.951). Additional information on the meta-regressions can be found in Fig. S4a–e.
Publication bias
A visual funnel plot analysis of comparisons between 10 or more studies for all outcomes did not outline publication bias given equal distribution of studies reporting both positive and negative effect sizes (Fig. S5). However, supplementary Egger's test identified significant publication bias when assessing global cognition (p = 0.0002) and attention domains (p = 0.002) due to the large effect size of one outlier that was removed as part of the sensitivity analysis. No significant publication bias was reported for visual memory, verbal memory, executive function, processing speed, or working memory outcomes (p > 0.05).
Sensitivity analysis
A sensitivity analysis was performed by removing eight studies with an indication of a history of a confounding variable (Downer et al., Reference Downer, Vickers, Al Snih, Raji and Markides2016; Kontari & Smith, Reference Kontari and Smith2019; Marijnissen et al., Reference Marijnissen, Vogelzangs, Mulder, van den Brink, Comijs and Oude Voshaar2017; Ng et al., Reference Ng, Niti, Zaw and Kua2009; Salvi et al., Reference Salvi, Di Salvo, Korčáková, Torriero, Aragno, Kolenič and Hajek2020; Silveira et al., Reference Silveira, Kozicky, Muralidharan, Bücker, Torres, Bond and Yatham2014; Tsai et al., Reference Tsai, Lee, Chen and Huang2007) or large effect size (Janocha et al., Reference Janocha, Bolanowski, Pilecki, Małyszczak, Salomon, Woźniak and Sobieszczańska2010) (Fig. S6–7). The overall effect size remained significant for global cognition (−0.37 SMD, 95% CI [−0.47 to −0.27], p < 0.00001, I 2 = 77%, n = 4539, k = 17) in the pooled population and within the BD and MDD subgroups, but not in the depressive symptoms subgroup due to removal of many studies. Similar results were produced for the individual metabolic domain subgroups; however, peripheral insulin resistance and hypertension effect sizes could not be calculated due to fewer than two studies included in these subgroups. The sensitivity analysis for the attention subgroup remained significant (−0.27 SMD, 95% CI [−0.44 to −0.09], p < 0.003, I 2 = 58%, n = 1419, k = 6). Reassessment of publication bias using the Egger's test for the global cognition outcome was no longer significant (p = 0.383; Fig. S5g).
Certainty of evidence
The GRADE framework identified very low certainty of evidence for verbal and visual memory due to both positive and negative findings within individual studies and high overall heterogeneity (I 2>75%), and similarly for global cognition and attention due to publication bias. A low certainty of evidence was assigned to executive function, processing speed, and working memory outcomes due to residual confounding such as the impact of pharmacotherapy on the outcomes of interest, differences between family history/genetic predispositions to mood or metabolic disorders, variance in cognitive measures, and no measurement of state v. trait characteristics of mood disorders (Table S4).
Discussion
This review is the first of its scope to explore the association between metabolic dysfunction and various cognitive outcomes in a diverse mood disorder population. Global cognitive performance was significantly lower among individuals with BD, MDD, and depressive symptoms experiencing comorbid metabolic dysregulation in comparison to metabolically healthy mood disorder populations, and the effect size did not differ significantly among the mood disorder subgroups. These relationships with cognition were strongest (in descending order) in mood disorder populations with T2D, peripheral insulin resistance, a BMI ⩾25, and MetS/multiple cardiometabolic risk factors. In analyzing individual cognitive domains, worse cognitive performance was most prominent for working memory, followed by attention, executive function/processing speed, global cognition, verbal memory, and visual memory. The association between high triglyceride levels and hypertension with cognitive outcomes could not be assessed because of the limited number of studies reporting on these parameters.
Overall, these findings which explore the impact of several metabolic parameters in multiple mood disorders correspond to trends that are in line with what has been reported in BD (Bora et al., Reference Bora, McIntyre and Ozerdem2019), as well as beyond the mood disorder population, including schizophrenia (Bora et al., Reference Bora, Akdede and Alptekin2017). Our results parallel and complement the associations explored from an alternate perspective, whereby depressive symptoms are associated with cognitive impairment in individuals with diabetes (Chow et al., Reference Chow, Verdonschot, McEvoy and Peeters2022). Similar associations were reported for bariatric patients with MDD, whose cognitive performance was impaired compared to bariatric patients without psychiatric illness (Restivo et al., Reference Restivo, McKinnon, Frey, Hall, Syed and Taylor2017). Therefore, this bidirectional relationship between metabolic dysfunction and mental health disorders permeates multiple populations, suggesting shared mechanisms in relation to their effect on cognitive outcomes. Some proposed biological underpinnings of these complex interactions include inflammation, hyperactivity of the hypothalamic-pituitary-adrenal axis, disrupted insulin signaling, and dysregulated glucose homeostasis (Korczak, Pereira, Koulajian, Matejcek, & Giacca, Reference Korczak, Pereira, Koulajian, Matejcek and Giacca2011).
Identification of this multifaceted relationship between metabolic dysregulation, mood disorders, and cognition provides a novel framework for addressing cognitive decline in this population. For example, intervention studies have utilized anti-diabetic drugs such as liraglutide and metformin to revert metabolic dysfunction in MDD and BD patients, and demonstrated improvements in cognitive outcomes following the trials (Guo et al., Reference Guo, Mi, Jiang, Xu, Tang, Tian and Wang2014; Mansur et al., Reference Mansur, Ahmed, Cha, Woldeyohannes, Subramaniapillai, Lovshin and McIntyre2017). Therefore, targeting the metabolic domain may serve as an alternative treatment option as current first-line interventions often fail to treat cognitive deficits (Hamer et al., Reference Hamer, Testani, Mansur, Lee, Subramaniapillai and McIntyre2019). This is of importance as cognitive impairment is associated with worse adherence to treatment in individuals with mood disorders (Corréard et al., Reference Corréard, Consoloni, Raust, Etain, Guillot, Job and Belzeaux2017; Martinez-Aran et al., Reference Martinez-Aran, Scott, Colom, Torrent, Tabares-Seisdedos, Daban and Vieta2009). As a result, this may exacerbate pre-existing symptoms and cost the healthcare system an additional $3252–$19 363 per patient, annually (Cutler, Fernandez-Llimos, Frommer, Benrimoj, & Garcia-Cardenas, Reference Cutler, Fernandez-Llimos, Frommer, Benrimoj and Garcia-Cardenas2018). Furthermore, due to the strong socioeconomic influence on metabolic health (Mohammed et al., Reference Mohammed, Habtewold, Birhanu, Sissay, Tegegne, Abuzerr and Esmaillzadeh2019) and mental illness (Mann, Heesch, Rachele, Burton, & Turrell, Reference Mann, Heesch, Rachele, Burton and Turrell2022), this may disproportionately increase the risk for cognitive dysfunction among individuals of low socioeconomic status (Wang et al., Reference Wang, Hu, Ou, Wang, Ma, Tan and Yu2023). Therefore, when cognitive impairments persist despite the use of standard treatments in mood disorder populations, they may exacerbate the burden at both the individual patient and global healthcare level.
This review synthesizes evidence which outlines the significant negative association between metabolic dysregulation and cognition in the context of mood disorders, with low inter-subgroup variability. Furthermore, it does so in a representative population by being inclusive of those experiencing depression who may not have a formal diagnosis due to stigma or limited access to mental health services. Despite this, there are several limitations that must be considered.
The results of this review must be interpreted with care as the differences observed in cognitive performance do not confirm cognitive impairment. The comparisons drawn between cognitive outcomes in individuals with mood disorders, with or without metabolic dysfunction, rather highlight the importance of assessing cognition in this population, and the close relationship between these domains. Further studies must be conducted with the use of standardized cognitive score thresholds to assess the clinical relevance of these associations. Additionally, it must be noted that cognitive data used for this analysis were sourced from heterogeneous populations that vary in age, sex ratio, medical comorbidities, clinical severity, and medication use. Although the meta-regressions did not identify any significant trends between cognitive outcomes and some of these parameters, they still serve as confounding variables that may influence the associations that were reported. Specifically, the prevalence of psychotropic medication use within studies was high. Due to the independent effect of these medications on metabolic parameters (Pillinger et al., Reference Pillinger, McCutcheon, Vano, Mizuno, Arumuham, Hindley and Howes2020), it is difficult to discern their effects from other underlying physiological mechanisms. Although these elements are more representative of populations that are seen in clinic, they may dampen the effects reported in the results. However, the reported medications were also used by the control group, such that matching allows for better interpretation of these associations. Furthermore, the medication-free/naïve populations outlined in this review reported similar trends, whereby a multitude of metabolic parameters were associated with worse cognition/greater risk for cognitive impairment (Qiu et al., Reference Qiu, Li, Teng, Tan, Xu, Yang and Wu2022; Zhuo et al., Reference Zhuo, Liu, Jiang, Li, Yu, Chen and Song2022). Therefore, further validation of these associations in medication-free/naïve populations is necessary to eliminate confounding factors.
In addition to the variability between study populations, there was also significant heterogeneity in the cognitive assessment tools employed and domains assessed within studies. As there is no standardized method to assess this outcome, this limits comparability of cognition between studies. Similarly, different assessments were used to measure depression severity, but SMD was computed to be able to compare these elements. Since there was no significant difference in depression symptom severity between the two comparison groups, this suggests that the associations with cognitive outcomes were predominantly driven by the metabolic parameters being studied. However, similar regressions could not be performed for assessments of mania, apathy, and avolition due to limited reporting of these outcomes. Furthermore, many studies also did not report on race, ethnicity, and socioeconomic status making it unclear how results generalize across these variables. Finally, small sample sizes with large margins of errors may have affected the strength of evidence and inclusion of mostly cross-sectional study designs limits the interpretation of causality. Therefore, large-scale longitudinal studies are necessary to explore the directionality of these relationships.
In conclusion, this review and meta-analysis demonstrates the negative association between metabolic dysregulation and cognition in individuals with mood disorders. T2D was demonstrated to have the strongest association with cognitive outcomes in this population. Of all cognitive domains, working memory performance was lowest in mood disorder populations with comorbid metabolic dysfunction v. metabolically healthy comparators. Further research is required to understand the underlying mechanisms of this interplay between mood disorders, metabolism, and cognition.
Supplementary material
The supplementary material for this article can be found at https://doi.org/10.1017/S0033291724000345.
Acknowledgements
The authors would like to thank Dr Van Rheenen et al., Dr Hubenak et al., and Dr Geraets et al. for providing additional information to synthesize in this review. S. M. A. is supported by in part by an Academic Scholars Award from the Department of Psychiatry, University of Toronto and the CAMH Discovery Fund. B. I. G. acknowledges his position as RBC Investments Chair in Children's Mental Health and Developmental Psychopathology at CAMH, a joint Hospital-University Chair between the University of Toronto, CAMH, and the CAMH Foundation.
Author contributions
All authors contributed to the synthesis and revision of the final manuscript. K. M. and S. M. A. were involved in the concept and design of the study, and planning formal analysis. K. M. was involved in conducting the systematic search, screening articles, data extraction, risk of bias and GRADE assessment, and statistical analysis. Z. H. contributed to article screening, data extraction, and risk of bias assessment. N. S., F. P., and J. G. contributed to article screening and data extraction. R. D., E. S., and A. H. contributed to article screening. M. K. H. was involved in planning formal analysis and revision of the manuscript. B. I. G., D. K., and A. G.-G revised the work and offered feedback for improvement.
Funding statement
This research received no specific grant from any funding agency, commercial or not-for-profit sectors.
Competing interests
S. M. A. has received honoraria from HLS therapeutics and has served on the advisory board of Boehringer Ingelheim, Canada. M. K. H. has received consultation fees from Alkermes.