Introduction
Tourette disorder (TD) is a neurodevelopmental disorder characterized by motor and vocal tics (American Psychological Association, 2013). It is often associated with psychiatric comorbidities, of which obsessive–compulsive disorders (OCDs), attention-deficit hyperactivity disorders (ADHDs), intermittent explosive disorders (IEDs) and depression represent the most common ones (Hirschtritt et al., Reference Hirschtritt, Lee, Pauls, Dion, Grados, Illmann and Lyon2015).
A dysfunction of cortico-striato-thalamo-cortical (CSTC) loops might account for this clinical spectrum (Singer, Reference Singer2005), as structural and functional abnormalities have been found in the basal ganglia (Worbe et al., Reference Worbe, Gerardin, Hartmann, Valabrégue, Chupin, Tremblay and Lehéricy2010, Reference Worbe, Malherbe, Hartmann, Pélégrini-Issac, Messé, Vidailhet and Benali2012), the sensory-motor areas (Fahim et al., Reference Fahim, Yoon, Das, Lyttelton, Chen, Arnaoutelis and Brandner2010; Worbe et al., Reference Worbe, Gerardin, Hartmann, Valabrégue, Chupin, Tremblay and Lehéricy2010), the dorsolateral prefrontal cortex and more generally the frontal cortex (Fredericksen et al., Reference Fredericksen, Cutting, Kates, Mostofsky, Singer, Cooper and Kaufmann2002; Kates et al., Reference Kates, Frederikse, Mostofsky, Folley, Cooper, Mazur-Hopkins and Pearlson2002), fronto-parietal networks (Atkinson-Clement et al., Reference Atkinson-Clement, Porte, de Liege, Wattiez, Klein, Beranger and Pouget2020; Eddy, Cavanna, Rickards, & Hansen, Reference Eddy, Cavanna, Rickards and Hansen2016) and the cerebellum (Lerner et al., Reference Lerner, Bagic, Boudreau, Hanakawa, Pagan, Mari and Simmons2007). Some of these abnormalities have been related to the disorder itself, but others have been associated with comorbidities, as well as with compensatory strategies to reduce the tics (Mazzone et al., Reference Mazzone, Yu, Blair, Gunter, Wang, Marsh and Peterson2010). Despite an extensive research, no reliable brain biomarker of this disorder has been found, and the precise pathophysiological mechanisms of TD are still poorly understood.
Multivariate approaches applied to neuroimaging, such as functional magnetic resonance imaging (fMRI), represent a valuable method to address the complex aspects of TD, due to their ability to (i) detect subtle and distributed patterns of activity throughout the brain in a fully data-driven manner, (ii) make predictions that have the potential to interrogate neurophysiological mechanisms, and (iii) aid in diagnosis and treatment (Nielsen, Barch, Petersen, Schlaggar, & Greene, Reference Nielsen, Barch, Petersen, Schlaggar and Greene2019). Recent studies have used multivariate approaches, in particular support vector machine (SVM), to discriminate children with TD from age-matched healthy controls (HCs) (Greene et al., Reference Greene, Church, Dosenbach, Nielsen, Adeyemo, Nardos and Schlaggar2016), as well as young TD patients from older ones (Nielsen et al., Reference Nielsen, Gratton, Church, Dosenbach, Black, Petersen and Greene2020). They have shown that functional brain abnormalities allow for the identification of TD with ~70% accuracy, and that delayed brain maturation may explain the atypical functional connectivity in adults with TD. However, they did not search a neural signature of the disorder in relation to pharmacological treatment. This is relevant, as patients with TD are often treated with antipsychotics and, even if their effects on the symptoms are documented, their specific action on large-scale brain networks is still unknown (Handley et al., Reference Handley, Zelaya, Reinders, Marques, Mehta, O'Gorman and Williams2013).
We employed a multivariate approach to predict patterns of resting state functional connectivity (rs-FC) in TD which: (i) inform on the neurophysiological mechanisms of adult TD, (ii) address the differences between TD patients under antipsychotic medication and unmedicated ones and (iii) correlate with symptoms' severity. We applied SVM to identify differences in connectivity patterns between TD patients and HCs, as well as between patients with and without medication. We also implemented a support vector regression (SVR) model that investigates whether rs-FC carries information about symptoms' severity. In addition to SVM, we performed a standard univariate analysis to evaluate specific differences in rs-FC across groups.
Our ultimate goal was to shed light on the altered brain functions underpinning TD in adults, as well as their link to medication status, and to study the potential of SVM as a predictive tool to support the diagnosis of TD.
Materials and methods
Participants and general procedure
We recruited 55 patients with TD and 55 sex- and age-matched HCs. Seven patients and four HCs were not able to perform the MRI (due to e.g. excessive movements) and were excluded from the analysis. The final sample consisted of 48 patients with TD (39 male, mean age: 30.5 ± 10.3 years) and 51 HCs (33 male, mean age: 30.9 ± 10.4 years).
Patients were recruited through the National Tourette Disorder reference center at the Pitié-Salpêtrière Hospital in Paris. Inclusion criteria for patients were: a diagnosis of TD according to DSM-5 (American Psychological Association, 2013), capability to control tics for at least 10 min during the MRI acquisition. Exclusion criteria for both HCs and patients with TD were: incompatibility with MR acquisition (e.g. claustrophobia and metallic body implants), history of alcohol or drug addiction (except for nicotine and recreational cannabis use for less than once per week), history of psychosis and learning disability. We also excluded HCs who experienced childhood tics and any neurological disorders.
In patients, tic severity was assessed using the Yale Global Tic Severity Scale (YGTSS50) (Leckman et al., Reference Leckman, Riddle, Hardin, Ort, Swartz, Stevenson and Cohen1989). The life-long diagnosis of psychiatric comorbidities, such as OCDs, ADHDs and IEDs, typically observed in TD (Hirschtritt et al., Reference Hirschtritt, Lee, Pauls, Dion, Grados, Illmann and Lyon2015), was evaluated using patients' medical records and psychiatric evaluations available at the inclusion in the study. Eighteen patients with TD were under stable medication for at least 3 years at the time of examination (Table 1).
Table 1. Clinical and demographic data

YGTSS, Yale global tic severity score; IED, intermittent explosive disorder; ADHD, attention deficit hyperactivity disorder; OCD, obsessive–compulsive disorder; HC, healthy control; TD, Tourette disorder.
Comparison of clinical and demographic scores across groups.
a All medications were prescribed for at least 3 years.
Standard protocol approvals, registrations and patients consent
The study was carried out in accordance with the latest version of the Declaration of Helsinki and approved by the local Ethics Committee (approval number: CCP16163/C16-07). All participants gave written informed consent prior to the study. The study was registered in ClinicalTials.gov (ID number: NCT02960698).
Clinical groups' analysis
We performed two analyses investigating: (i) between-group differences in 51 HCs and 48 patients with TD, and (ii) between-TD patients' subgroup differences in 18 patients with medication and 18 patients without medication. For the latter, as SVM achieves best performance when the two discriminated groups contain the same number of samples (Wu & Chang, Reference Wu and Chang2003), we analyzed data from all patients with TD under medication (n = 18) and a subset of 18 unmedicated patients that matched the group of medicated TD for age, sex, symptom severity and comorbidities. This ensured balanced groups, and excluded potential confounds due to between-group differences other than in rs-FC.
Differences in age were assessed with independent-sample t tests, whereas differences in the ratio between male and female participants were assessed with χ2 tests. Moreover, for the between-TD patients' subgroup analysis, differences in the YGTSS50 were assessed with independent-sample t tests, and differences in the ratio of patients with and without IED, ADHD and OCD were assessed with χ2 tests. The significance level was set at 0.05. Data analysis was performed with SPSS 25 (IBM Statistics, USA).
Neuroimaging acquisition parameters and pre-processing
During the MR session, participants were asked to lie still with the eyes open, while fixating a cross on a screen. Eye movements were monitored with an eye-tracking device. Neuroimaging data were acquired using a 3T Magnetom Prisma (Siemens, DE) with a 64-channel head coil. Resting state fMRI and structural images were acquired in one session using the following parameters: (i) echo-planar imaging sequences performed with a multi-slice, multi-echo acquisition, repetition time (TR) = 1.9 s, echo time (TE) = 17.2/36.62/56.04 ms, Ipat acceleration factor = 2, multi-band = 2, isotropic voxel size = 3 mm, dimensions = 66 × 66 in plane × 46 slices, 350 volumes, duration = 11 min; (ii) a T1-weighted MP2RAGE sequence with TR = 5 s, inversion time (TI) = 700/2500 ms, field of view (FOV) = 232 × 256 in plane × 176 slices, 1 mm isotropic, Ipat acceleration factor = 3.
T1-weighted images were first background denoised (O'Brien et al., Reference O'Brien, Kober, Hagmann, Maeder, Marques, Lazeyras and Roche2014) using https://github.com/benoitberanger/mp2rage, which is based on Reference MarquesMarques. This step improved the quality of the subsequent segmentation. Images were then pre-processed (segmentation, normalization to Montreal Neurological Institute – MNI – space) using the Computational Anatomy Toolbox (http://www.neuro.uni-jena.de/cat/) extension for SPM12 (https://www.fil.ion.ucl.ac.uk/spm/).
Functional data were pre-processed with AFNI using afni_proc.py script (https://afni.nimh.nih.gov/) according to standard procedures (despiking, slice timing correction and realignment to the volume with the minimum outlier fraction driven by the first echo). A brain mask was computed on the realigned shortest echo temporal mean using FSL BET (Jenkinson, Beckmann, Behrens, Woolrich, & Smith, Reference Jenkinson, Beckmann, Behrens, Woolrich and Smith2012). This step increased the robustness against strong signal bias intensity. Afterward, the TEDANA toolbox (Kundu et al., Reference Kundu, Voon, Balchandani, Lombardo, Poser and Bandettini2017) version 0.0.7 was used to optimally combine the realigned echoes, to reduce the dimensionality of the dataset by applying principal component analysis, and to perform an independent component analysis (ICA) decomposition which separated BOLD from non-BOLD components, based on the TE dependence of the ICA components (Kundu, Inati, Evans, Luh, & Bandettini, Reference Kundu, Inati, Evans, Luh and Bandettini2012). This step ensured robust artifact removal of non-BOLD components, such as movement, respiration or heartbeat. Previous research with resting state fMRI has already confirmed the superiority of this method in regressing out motion over standard denoising techniques (Kundu et al., Reference Kundu, Voon, Balchandani, Lombardo, Poser and Bandettini2017). To further confirm this, framewise displacement (FD) was computed according to standard methods (Power et al., Reference Power, Mitra, Laumann, Snyder, Schlaggar and Petersen2014), and compared between the groups. No motion artifacts affected the quality of the signal, the FD in TD (0.016 ± 0.007 mm) did not statistically differ from HC (0.136 ± 0.006 mm) [t(97) = 1.91, p = 0.059], and FD in patients with medication (0.016 ± 0.009 mm) did not statistically differ from patients without medication (0.016 ± 0.005 mm) [t(34) = 0.21, p = 0.830]. Finally, using SPM12, images were co-registered to the anatomical scan, normalized to MNI space, then smoothed with a Gaussian kernel with full width at half maximum of 5 × 5 × 5 mm.
Brain images were parcellated into 116 functional regions, based on the automatic anatomical labeling (AAL) atlas (Tzourio-Mazoyer et al., Reference Tzourio-Mazoyer, Landeau, Papathanassiou, Crivello, Etard, Delcroix and Joliot2002), and the region-averaged time series were extracted. The first 10 time points were discarded to ensure magnetization equilibrium. Motion parameters and the average signal of white matter and cerebrospinal fluid obtained during the segmentation, were regressed out. Time series were finally band-pass filtered at 0.009 < f < 0.08 Hz, according to previous studies (Greene et al., Reference Greene, Church, Dosenbach, Nielsen, Adeyemo, Nardos and Schlaggar2016; Nielsen et al., Reference Nielsen, Gratton, Church, Dosenbach, Black, Petersen and Greene2020). We computed pairwise Pearson correlation coefficients between all pairs of brain regions as indicators of their functional connectivity, and we obtained a symmetric correlation matrix of 116 × 116 coefficients for each participant, i.e. 6670 correlation coefficients. Next, we converted the correlation coefficients to z-scores using Fisher-Z transformation, in order to normalize them to a Gaussian distribution (Wegrzyk et al., Reference Wegrzyk, Kebets, Richiardi, Galli, Van de Ville and Aybek2018).
Multivariate analysis for neuroimaging data
The 6670 correlation coefficients were used as features for linear SVM models. We implemented three predictive models to: (i) distinguish HCs from patients with TD, (ii) distinguish patients with and without medication and (iii) predict symptoms' severity in patients with TD. The first two models were initially optimized with an automatic grid search algorithm based on Bayesian optimization (Hastie, Tibshirani, & Friedman, Reference Hastie, Tibshirani and Friedman2009). The optimization minimized the cross-validation loss (error) by iteratively varying the C parameter and Kernel Scale. In line with previous research (Nielsen et al., Reference Nielsen, Gratton, Church, Dosenbach, Black, Petersen and Greene2020; Wegrzyk et al., Reference Wegrzyk, Kebets, Richiardi, Galli, Van de Ville and Aybek2018), the best values were found to be C = 1 and Kernel Scale = 1. The models were then trained to learn a function that separates the two groups, based on the differences in their rs-FC. The models were trained on a known dataset of participants belonging to the two groups, and mathematically assigned weights to each connection, based on its contribution to the discrimination. Once the models were built, they used these weights to predict the group where a new and unknown participant belongs to. We applied leave-one-subject-out-cross-validation (LOSOCV) to estimate the generalization of the models. The statistical significance of the classification accuracy was assessed using its null distribution under permutation testing, where group labels were randomly permuted 1000 times. We finally trained the SVMs with all HCs and patients, and identified the most discriminative connections as the ones holding the highest weights (Wegrzyk et al., Reference Wegrzyk, Kebets, Richiardi, Galli, Van de Ville and Aybek2018). This step was performed after evaluating that the most discriminative connections obtained following training the SVM with the entire dataset were consistent with the ones calculated by the single LOSOCV folds (online Supplementary Fig. S1). We studied whether rs-FC contains information about symptom severity by implementing a SVR model [C = ∞, Kernel Scale = 1, ε = 0.00001, according to previous research (Nielsen et al., Reference Nielsen, Gratton, Church, Dosenbach, Black, Petersen and Greene2020) and optimized with Bayesian optimization, as described above] to predict YGTSS50 (Greene et al., Reference Greene, Church, Dosenbach, Nielsen, Adeyemo, Nardos and Schlaggar2016), and estimated it with LOSOCV. We studied the performance of the SVR model with r 2. The SVM and SVR were implemented with the Statistics and Machine Learning Toolbox in Matlab R2018a (The MathWorks, USA).
Univariate analysis for neuroimaging data
We performed a standard univariate analysis to study specific differences in rs-FC across groups. We compared the 6670 correlation coefficients between HCs and patients with TD, and between patients with and without medication, respectively, with multiple independent t tests. Moreover, in patients with TD, we computed Pearson's correlation coefficients between each connection and the YGTSS50. All tests were corrected for multiple comparisons with 1000 permutations. The significance level was set to 0.05 after correction. Data analysis was implemented in Matlab R2018a.
Results
Subjects
No statistically significant differences were found in sex and age between TD and HC. No statistically significant differences were found in tic severity and in the occurrence of comorbidities between medicated and unmedicated patients. Demographic and clinical data are presented in Table 1.
Multivariate analysis of rs-FC between the groups
The performance of the first two classifiers is shown in Table 2. The classifiers discriminated TD from HC (p = 0.004), as well as TD with and without medication (p = 0.019), with accuracy, specificity and sensitivity well above chance.
Table 2. Results of the multivariate analysis

HC, healthy control; TD, Tourette disorder.
Predictive performance of the SVM classifiers. p values represent the significance of the results, compared to chance.
For the between-group classification analysis, the SVM identified the most discriminative connections, i.e. the connections holding the highest weights, in fronto-cerebellar, fronto-parietal, parieto-cerebellar and subcortico-subcortical networks (Fig. 1a and online Supplementary Fig. S1A). In particular, Fig. 1a shows that the classification accuracy was driven by the connectivity between (i) the cerebellar lobule 7b and the superior parietal gyrus, (ii) the orbito-frontal cortex (OFC) and the angular gyrus, (iii) the putamen and the caudate, (iv) the caudate and the cerebellar lobule 10 and (v) the cerebellar vermis 9 and the OFC (online Supplementary Table S1).
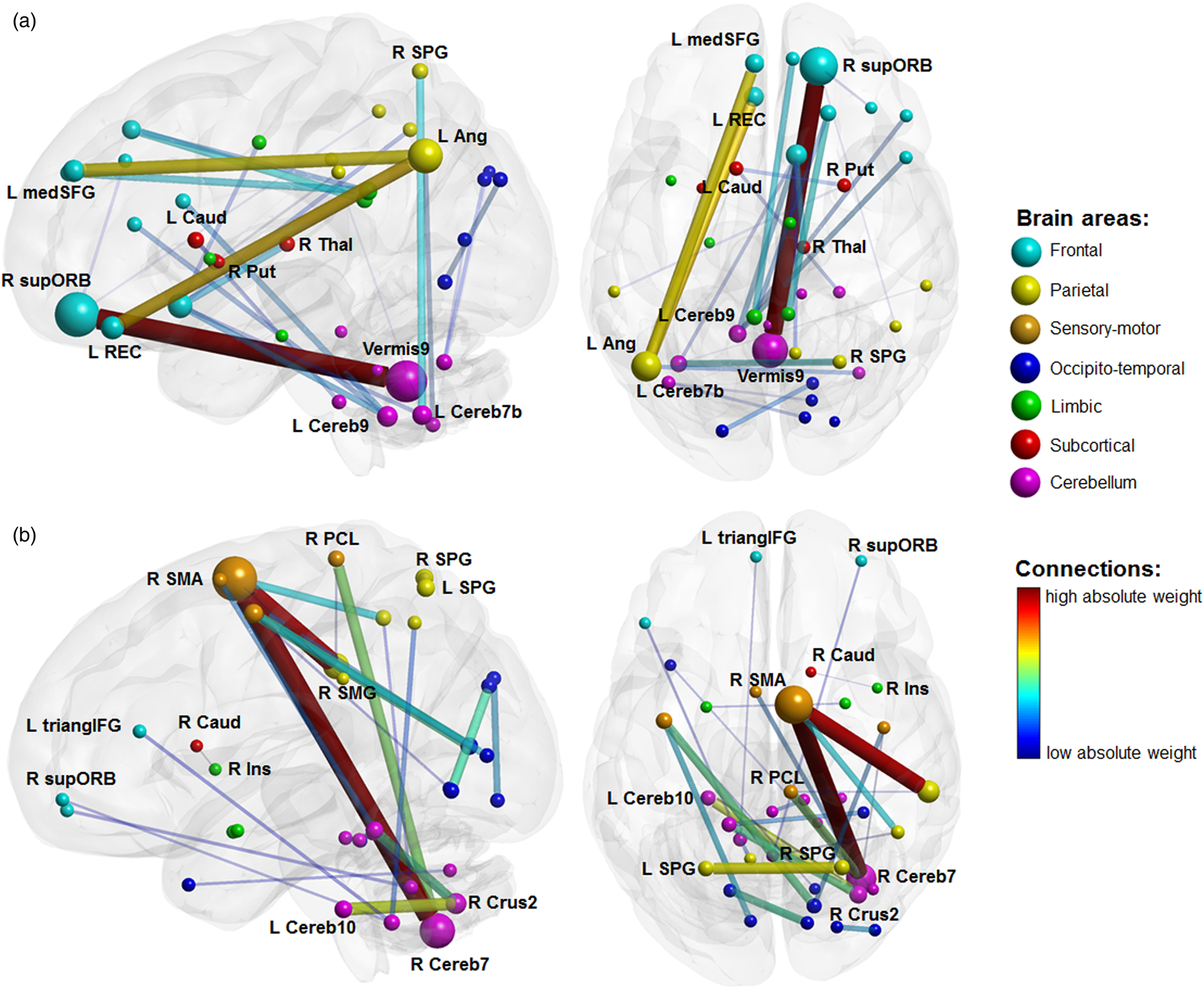
Fig. 1. SVM classifier with all HC and patients, most discriminative connections. Color code represents the absolute weights assigned to the connections. Node size represents the mean weighted number of connections entering the node over the entire set of 6670 weights. Line thickness represents the absolute mean weight of the connection over the entire set. For graphical purposes, the figure is truncated so that only the top 30 connections are displayed. (a) SVM for TD v. HC. (b) SVM for medicated v. unmedicated TD. R, right; L, left; Ang, angular gyrus; Caud, nucleus caudate; Cereb(N), Nth cerebellar lobule; Ins, insula; medSFG, medial segment of the superior frontal gyrus; PCL, paracentral lobule; Put, putamen; REC, rectus gyrus; SMA, supplementary motor area; SMG, supramarginal gyrus; SPG, superior parietal gyrus; supORB, superior segment of the orbital gyrus; Thal, thalamus; triangIFG, pars triangularis of the inferior frontal gyrus.
For the between-TD patients' subgroup classification analysis, the SVM identified the most discriminative connections in fronto-cerebellar, cerebello-limbic, parieto-cerebellar and cerebello-subcortical networks (Fig. 1b and online Supplementary Fig. S1B). Figure 1b shows that the performance of the classifier was driven by the connectivity (i) of the supplementary motor area (SMA) with the cerebellar lobule 7b and the supramarginal gyrus, respectively, (ii) within the cerebellar regions, namely between crus 2 and, respectively, 4th, 5th, and 10th lobules, (iii) between the right caudate and the right insula and (iv) between the cerebellum (vermis 9 and 9th lobule) and the OFC and the inferior frontal gyrus, respectively (online Supplementary Table S2). These patterns were independent of the number of top feature weights considered, as shown in online Supplementary Fig. S2.
The analysis of the SVR showed that rs-FC was not able to predict symptom severity in patients with TD (r 2 = 0.05, p = 0.114).
Univariate analysis of rs-FC
The univariate analysis showed increased functional connectivity, in patients with TD compared to HCs (Fig. 2a), of the right caudate with the right and left putamen, respectively [t(97) = 5.06, p corrected = 0.003 and t(97) = 5.29, p corrected = 0.001], and of the left caudate with the right and left putamen, respectively [t(97) = 4.21, p corrected = 0.050 and t(97) = 4.38, p corrected = 0.037].
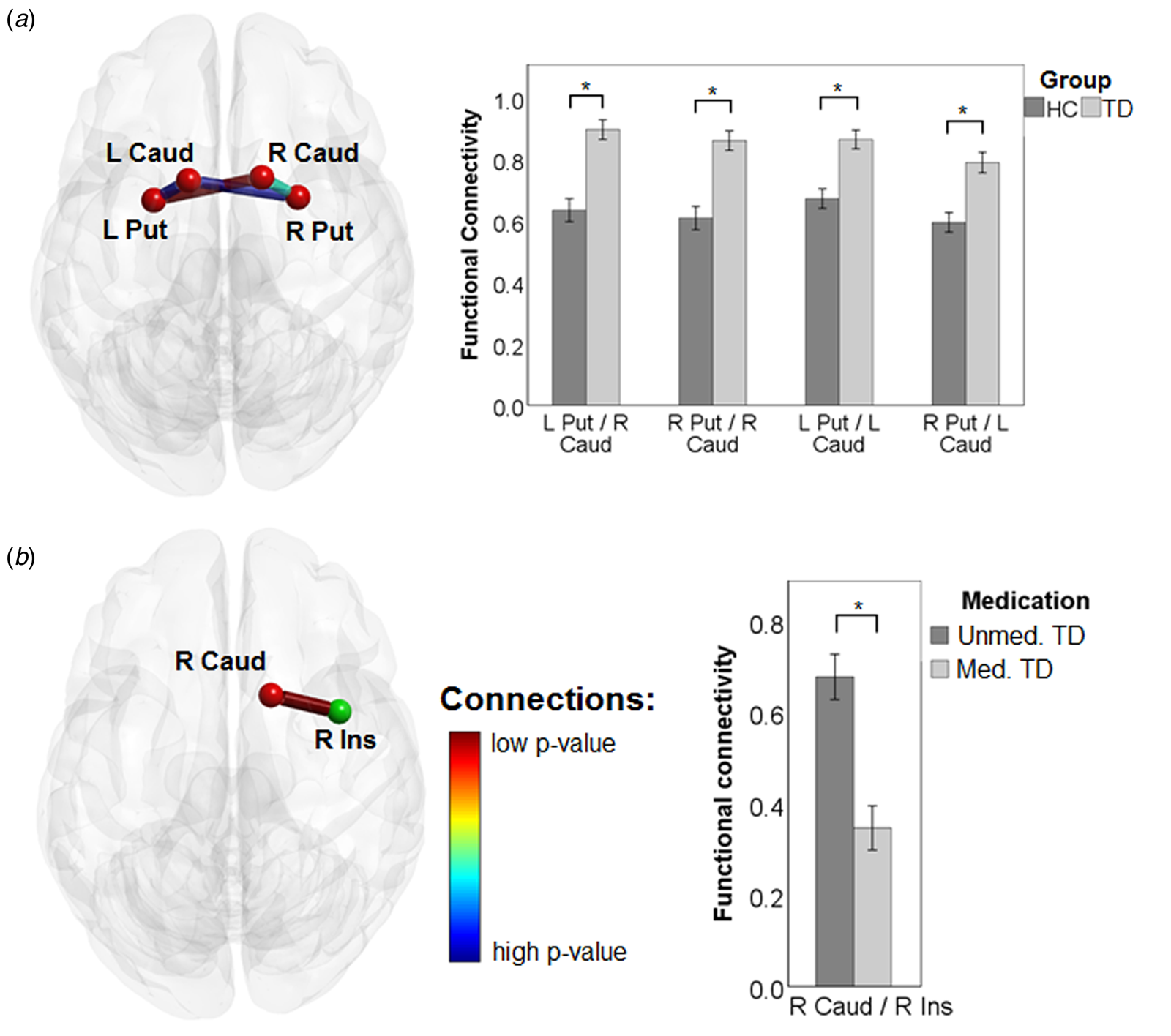
Fig. 2. Results of the univariate analysis. (a) Functional connectivity between TD and HC. (b) Functional connectivity between medicated and unmedicated TD. Bars represent the mean values ± s.e. of the mean. * depicts significant differences at independent sample t tests.
In the between-TD patients' subgroup analysis, the connectivity between the right caudate and the right insula was lower in medicated v. unmedicated patients [t(34) = 4.77, p corrected = 0.050] (Fig. 2b).
No correlation between YGTSS50 and any of the connections was found in patients with TD (p corrected > 0.05).
Discussion
Using rs-FC and a multivariate approach in a fully data-driven manner, we were able to significantly discriminate adult patients with TD from HCs, and patients with and without medication. Compared to HCs, patients with TD showed abnormal rs-FC among widespread brain areas, including striatum and cerebellum. Functional connectivity of the SMA, the OFC, the insula and the posterior parietal cortex, as well as the striatum and the cerebellum discriminated the patients under conventional medication with antipsychotics, such as aripiprazole (APZ), from the unmedicated patients. The univariate analysis found significant differences in connectivity between HCs and patients with TD within the striatum, and between medicated and unmedicated patients with TD in the connection between the caudate nucleus and the insula.
Our study has some limitations. First, we chose the AAL atlas based on existing literature on classification of rs-FC using a similar pipeline as in our study (Lee & Frangou, Reference Lee and Frangou2017; Richiardi et al., Reference Richiardi, Gschwind, Simioni, Annoni, Greco, Hagmann and Van De Ville2012; Wegrzyk et al., Reference Wegrzyk, Kebets, Richiardi, Galli, Van de Ville and Aybek2018). The choice of the atlas was crucial for our approach, as the brain parcellation might have a major impact on the definition of the regions of interest, hence on the connectivity patterns, and ultimately on the results. However, previous research has compared the rs-FC classification performance across different atlases (Wegrzyk et al., Reference Wegrzyk, Kebets, Richiardi, Galli, Van de Ville and Aybek2018), and found similar accuracy of ~70% when using, for instance, the AAL, the Hammers (Hammers et al., Reference Hammers, Allom, Koepp, Free, Myers, Lemieux and Duncan2003) and the Shirer (Shirer, Ryali, Rykhlevskaia, Menon, & Greicius, Reference Shirer, Ryali, Rykhlevskaia, Menon and Greicius2012) atlases. Second, we compared the medicated and non-medicated patients with TD in a parallel design. It is possible that patients under medication have substantial differences from the group of unmedicated patients. Thus, to fully address the question of APZ effect on brain networks, the same patients with TD should be assessed before and after the beginning of pharmacological treatment.
Overall, our results strengthen previous knowledge of altered brain networks in adult TD, and provide new evidence of specific patterns of functional connectivity in TD patients with pharmacological treatment.
Differences in functional connectivity between TD and controls
The results indicated large-scale networks' alteration in adult TD, and specifically in the connectivity between cortical areas, the cerebellum and the striatum. Other studies in patients with TD have confirmed functional and structural abnormalities between the striatum and sensory-motor cortices, OFC, parietal and temporal regions, similar to our results (Martino, Ganos, & Worbe, Reference Martino, Ganos and Worbe2018).
The connectivity within the striatum was among the most discriminative features of our multivariate analysis. This structure, as central part of the CSTC network (Singer, Reference Singer2005; Worbe, Lehericy, & Hartmann, Reference Worbe, Lehericy and Hartmann2015), has been suggested by various animal models to account for the wide spectrum of TD symptoms (Bronfeld, Yael, Belelovsky, & Bar-Gad, Reference Bronfeld, Yael, Belelovsky and Bar-Gad2013; Worbe et al., Reference Worbe, Sgambato-Faure, Epinat, Chaigneau, Tandé, François and Tremblay2013). Recent computational models of pathophysiology of TD have also suggested that tics and premonitory urges result from the abnormal computation of the sensation and action within sensory-motor regions of the striatum (Rae, Critchley, & Seth, Reference Rae, Critchley and Seth2019).
The connectivity of cerebello-cortical and cerebello-cerebellar networks was also among the most discriminative features of patients with TD compared to HCs. These results are in line with data obtained from animal models of TD, suggesting that tics result from the global neuronal rhythms abnormalities of cerebro-basal ganglia-cerebellar networks, due to striatal disinhibition (McCairn, Iriki, & Isoda, Reference McCairn, Iriki and Isoda2013). In particular, there is evidence that a cerebellar-prefrontal network is implicated in motor execution specific to Go events in Go-no-Go tasks (Mostofsky et al., Reference Mostofsky, Schafer, Abrams, Goldberg, Flower, Boyce and Denckla2003), and our results showed an impairment in such a network, which may lead to an alteration of unwanted movement suppression and, in turn, to tic release. Numerous studies have further confirmed abnormal structural and functional connectivity of the cerebellum with cortical areas and basal ganglia, namely the striatum (Ramkiran, Heidemeyer, Gaebler, Shah, & Neuner, Reference Ramkiran, Heidemeyer, Gaebler, Shah and Neuner2019; Sigurdsson, Jackson, Jolley, Mitchell, & Jackson, Reference Sigurdsson, Jackson, Jolley, Mitchell and Jackson2020) in patients with TD.
Overall, our results point to the pivotal role of the striatum and cerebellum in the pathophysiology of TD. They also suggest that functional connectivity of the striatum, cerebello-cerebellar and cerebello-cortical networks might be considered as potential imaging biomarkers of this disorder. However, and similar to previous research (Greene et al., Reference Greene, Church, Dosenbach, Nielsen, Adeyemo, Nardos and Schlaggar2016), SVR was not able to predict tic severity. This could result from the fact that only patients with low-to-moderate tic severity were included in the study, to guarantee the quality of MRI acquisitions. Further studies combining structural and functional connectivity are warranted to address this question. For instance, a larger hippocampal volume in children with TD predicted the persistence of tics in follow-up visits after onset (Sigurdsson et al., Reference Sigurdsson, Jackson, Jolley, Mitchell and Jackson2020). Moreover, our sample did not allow us to study the effects of comorbid disorders on rs-FC. Future research with a large number of patients will allow for the stratification of TD according to comorbidities, in order to disentangle their contribution to networks dysfunction. One potential limitation of this study is that we included only patients with low-to-moderate tics that did not impact the quality of the images. Also, even if the patients were not explicitly instructed to suppress their tics, some of them might have still performed this voluntary suppression, and this could have had an impact on the results.
Differences in functional connectivity between medicated and unmedicated TD
The most common drug used to treat TD in this study was APZ, taken by 83% of medicated patients. This antipsychotic acts on the dopaminergic and serotonergic function as a partial agonist of the dopamine D2 receptor and 5-HT1A, and antagonism at 5-HT2A receptors (Jordan et al., Reference Jordan, Koprivica, Dunn, Tottori, Kikuchi and Altar2004). It has shown a positive effect on tics in TD (Bubl, Perlov, & Tebartz Van Elst, Reference Bubl, Perlov and Tebartz Van Elst2006; Kastrup, Schlotter, Plewnia, & Bartels, Reference Kastrup, Schlotter, Plewnia and Bartels2005).
The empirical model of APZ action postulates that brain areas with high density of neurons with dopamine D2 receptors (D2R) might be more sensitive to this drug, and might, in turn, influence the activity of other regions innervated by the D2R neurons (Handley et al., Reference Handley, Zelaya, Reinders, Marques, Mehta, O'Gorman and Williams2013). Previous research has shown that, compared to placebo, APZ intake in healthy volunteers modulates activity in a network including the putamen, the insula, the caudate and the cerebellum, as well as in the superior frontal gyrus, the superior and inferior parietal lobes and the OFC (Handley et al., Reference Handley, Zelaya, Reinders, Marques, Mehta, O'Gorman and Williams2013), all regions found discriminative of medicated compared to unmediated patients in the current study. The univariate approach pointed to differences in the connectivity between the caudate and the insula in medicated compared to unmedicated patients. The insula has been related to the ‘urge-for-action’ (Worbe et al., Reference Worbe, Lehericy and Hartmann2015), i.e. suppression of natural urges (such as blinking), in healthy participants (Lerner et al., Reference Lerner, Bagic, Hanakawa, Boudreau, Pagan, Mari and Murphy2009), but also to uncomfortable feeling associated with the premonitory urges in TD (Jackson, Parkinson, Kim, Schüermann, & Eickhoff, Reference Jackson, Parkinson, Kim, Schüermann and Eickhoff2011). In particular, a brain network encompassing the insular cortex has been found active prior to tic onset, and concomitant with the subjective experience of the premonitory urge (Bohlhalter et al., Reference Bohlhalter, Goldfine, Matteson, Garraux, Hanakawa, Kansaku and Hallett2006). Similarly, functional connectivity (Tinaz, Malone, Hallett, & Horovitz, Reference Tinaz, Malone, Hallett and Horovitz2015) and cortical thickness (Draper, Jackson, Morgan, & Jackson, Reference Draper, Jackson, Morgan and Jackson2016) of the insula have been correlated with the urge to tic in TD. Altogether, these findings support a key role of the insula in the perception of bodily urges, linking the sensory and emotional character of premonitory urges with their translation into tics (Cavanna, Black, Hallett, & Voon, Reference Cavanna, Black, Hallett and Voon2017; Conceição, Dias, Farinha, & Maia, Reference Conceição, Dias, Farinha and Maia2017; Cox, Seri, & Cavanna, Reference Cox, Seri and Cavanna2018). In this study we have not monitored premonitory urges in patients with TD, however, our results indicate that antipsychotics might act on insular and striatal loops, and the tics improvement might result from premonitory urges reduction. This points to a potential effect of APZ on striatal, insular and cerebellar networks, and the activity of these areas might be used in future research to monitor the effects of medication.
One potential confound is the use of concomitant medications other than APZ, which may have an impact on rs-FC. Due to our sample size, we did not stratify according to medication type, however, most of our patients was under APZ, and our findings are in line with existing evidence of altered cortical and subcortical activity in healthy participants after APZ (Handley et al., Reference Handley, Zelaya, Reinders, Marques, Mehta, O'Gorman and Williams2013). It is, therefore, unlikely that the other drugs biased our predictions.
Overall, these results suggest that antipsychotic medication might affect the activity of areas within the CSTC loop implicated in tic generation and volitional control (Ganos, Roessner, & Münchau, Reference Ganos, Roessner and Münchau2013). Its benefic effects on these areas may, in turn, spread to other regions functionally connected to the CSTC loop, and improve other cognitive functions impaired in TD.
Advantages of multivariate approaches
The results of our study demonstrated that multivariate approaches can be successfully used to predict adult TD based on abnormal patterns of rs-FC. Recent studies have confirmed the advantage of multivariate approaches, in particular SVM, in investigating patterns of differential functional connectivity between children and adults with TD (Nielsen et al., Reference Nielsen, Gratton, Church, Dosenbach, Black, Petersen and Greene2020), or between children with TD and age-matched HCs (Greene et al., Reference Greene, Church, Dosenbach, Nielsen, Adeyemo, Nardos and Schlaggar2016). Yet, these studies have applied a feature reduction, and restricted the analysis to a smaller number of connections (Greene et al., Reference Greene, Church, Dosenbach, Nielsen, Adeyemo, Nardos and Schlaggar2016). Indeed, this methodological choice improves the classification accuracy and computational time, as the complexity of the model is reduced, however, it introduces a priori information on which regions carry the relevant information, often achieved through univariate comparisons (Greene et al., Reference Greene, Church, Dosenbach, Nielsen, Adeyemo, Nardos and Schlaggar2016), and might thus exclude other areas still relevant for the understanding of TD. Moreover, the interpretability of the results obtained after feature selection has been recently questioned (Nielsen et al., Reference Nielsen, Barch, Petersen, Schlaggar and Greene2019). We opted for no feature selection, as we were interested in the connectivity at the whole-brain scale, and our classifier showed similar performance to other studies in TD (Greene et al., Reference Greene, Church, Dosenbach, Nielsen, Adeyemo, Nardos and Schlaggar2016; Nielsen et al., Reference Nielsen, Gratton, Church, Dosenbach, Black, Petersen and Greene2020).
Conclusions
Overall, our results showed the potential of multivariate classification methods for clinical use, to help the diagnostic process and/or to evaluate the effects of treatments. Also, the results of this study hold a promise to identify an imaging-based biomarker of TD and to monitor treatments. Future research on a larger sample will allow for accurate models in relationship with co-morbidities of TD, and will move the field closer toward imaging-based biomarkers to guide clinical decisions (Wolfers, Buitelaar, Beckmann, Franke, & Marquand, Reference Wolfers, Buitelaar, Beckmann, Franke and Marquand2015).
Supplementary material
The supplementary material for this article can be found at https://doi.org/10.1017/S0033291721004232
Acknowledgements
The authors acknowledge the Swiss National Science Foundation (SNSF), the Paris Brain Institute and the University Hospitals Pitié-Salpêtrière for the support. They also thank all the participants of our study.
Financial support
This work was supported by the Swiss National Science Foundation (GAZ, grant P400PM_183958), the Fondation pour la Recherche Médicale (FRM), the French association for Tourette disorder (AFSGT) and the National Research Agency (YW, grant ANR-18-CE37-0008-01).
Conflict of interest
None.
Ethical standards
The authors assert that all procedures contributing to this work comply with the ethical standards of the relevant national and institutional committees on human experimentation and with the Helsinki Declaration of 1975, as revised in 2008.