Introduction
Psychotic-like experiences (PLEs), also referred to as subclinical psychotic symptoms or psychotic experiences, are odd or unreal perceptions, thoughts, or beliefs (Hinterbuchinger & Mossaheb, Reference Hinterbuchinger and Mossaheb2021). It is one of the earliest manifestations of psychotic disorders and can be common among children (Linscott & van Os, Reference Linscott and van Os2013; Schultze-Lutter et al., Reference Schultze-Lutter, Renner, Paruch, Julkowski, Klosterkötter and Ruhrmann2014). PLEs in childhood are associated with elevated rates of schizophrenia diagnoses and suicide attempts in adulthood (Fisher et al., Reference Fisher, Caspi, Poulton, Meier, Houts, Harrington and Moffitt2013; Poulton et al., Reference Poulton, Caspi, Moffitt, Cannon, Murray and Harrington2000). Additionally, persistent and distressing PLE may be more indicative of psychopathology later on in life with greater functional impairment, greater cognitive impairment, and increased mental health service utilization (Karcher et al., Reference Karcher, Loewy, Savill, Avenevoli, Huber, Makowski and Barch2022; Oh et al., Reference Oh, Karcher, Soffer-Dudek, Koyanagi, Besecker and Devylder2024). Given the high prevalence of PLE in early adolescence and its clinical significance to later risk for psychosis and general psychopathology, exploring the risk factors and mechanisms underlying persistent and distressful PLE is crucial to improve psychiatric risk assessments and more effective prevention strategies in children and adolescents.
It has long been known that urban upbringing is a major risk factor for developing psychotic disorders, including schizophrenia (March et al., Reference March, Hatch, Morgan, Kirkbride, Bresnahan, Fearon and Susser2008; Pignon, Szoke, Ku, Melchior, & Schurhoff, Reference Pignon, Szoke, Ku, Melchior and Schurhoff2023). Under the social determinants of health model (SDOH) for psychosis, certain environmental and social conditions (e.g. pollution concentrations, social fragmentation, and socioeconomic deprivation) may impact downstream psychosocial and biological processes and, in turn, lead to psychosis (B. S. Ku, Addington, et al., Reference Ku, Addington, Bearden, Cadenhead, Cannon, Compton, Cornblatt, Druss, Gülöksüz, Mathalon, Perkins, Tsuang, Walker, Woods and Carrión2023; D. M. Anglin et al., Reference Anglin, Ereshefsky, Klaunig, Bridgwater, Niendam, Ellman and van der Ven2021; Karcher, Schiffman, & Barch, Reference Karcher, Schiffman and Barch2021; Walker et al., Reference Walker, Aberizk, Yuan, Bilgrami, Ku and Guest2024). Early exposure to air pollution has been associated with increased odds of psychotic-like experiences in adolescence, potentially mediated by inflammation and excessive oxidative stress (Newbury et al., Reference Newbury, Heron, Kirkbride, Fisher, Bakolis, Boyd and Zammit2024). The Social Disorganization Theory posits that the wider community plays an important role in shaping social interactions, whether through sports or extracurricular activities, which may be crucial for mental health, especially for children (Ku, Compton, Walker, & Druss, Reference Ku, Compton, Walker and Druss2021). For example, youth living in neighborhoods that were more walkable, had less crime, and greater social cohesion participated in more physical activities, particularly team sports (Wang, Narcisse, & McElfish, Reference Wang, Narcisse and McElfish2023; Xiao et al., Reference Xiao, Mann, Chow, Brown, Snowden, Yip and Su2023). And greater involvement with team sports, as opposed to individual sports, was shown to be associated with reduced risk for future psychopathology, including persistent distressing PLE (Hoffmann, Barnes, Tremblay, & Guerrero, Reference Hoffmann, Barnes, Tremblay and Guerrero2022; B. S. Ku, Q. Yuan, et al., Reference Ku, Yuan, Arias-Magnasco, Lin, Walker, Druss and Guloksuz2024). Perhaps structural factors may facilitate youth coming together to play team sports, and certain aspects of these social ties may be relevant to offset the risk of psychosis (B. S. Ku, Q. Yuan, et al., Reference Ku, Yuan, Arias-Magnasco, Lin, Walker, Druss and Guloksuz2024).
Prior literature has largely focused on analyzing each neighborhood characteristic as it relates to psychosis separately (B. S. Ku, Q. Yuan, et al., Reference Ku, Yuan, Haardörfer, Addington, Bearden, Cadenhead, Cannon, Cornblatt, Keshavan, Mathalon, Perkins, Stone, Woods, Druss, Walker and Anglin2024; Deidre M. Anglin et al., Reference Anglin, Espinosa, Addington, Cadenhead, Cannon, Cornblatt and Ku2023; Ku, Addington, et al., Reference Ku, Addington, Bearden, Cadenhead, Cannon, Compton and Druss2021; B. S. Ku, J. Ren, et al., Reference Ku, Ren, Compton, Druss, Guo and Walker2024; O’Donoghue, Roche, & Lane, Reference O’Donoghue, Roche and Lane2016). Existing measurement tools, such as the Area Deprivation Index (ADI) and Child Opportunity Index (COI), have been limited in their ability to capture the multifaceted impacts of various neighborhood characteristics simultaneously while also highlighting individual contributions of each neighborhood characteristic (Benson S. Ku et al., Reference Ku, Aberizk, Feurer, Yuan, Druss, Jeste and Walker2024; Sandel et al., Reference Sandel, Faugno, Mingo, Cannon, Byrd, Garcia and Boynton-Jarrett2016). These indices are constructed from a chosen set of SDOH variables, and little is currently known about the combinations of these environmental and social factors as well as the mechanisms through which they lead to psychosis. Without such information, it may be difficult to devise effective, targeted policies and interventions.
This study aimed to fill this knowledge gap by using innovative methodologies that capture and analyze SDOH’s multidimensional nature and uncover their association with distressing PLE and physical activities. We hypothesized that certain exposure profiles of neighborhood characteristics would be associated with persistent distressing PLE and that team sports would partially explain this association.
Methods
The data were collected from a population-based sample of 9-to-10-year-olds in the Adolescent Brain and Cognitive Development Study (ABCD) 5.0 release, which included visits collected between 1st, September 2016, and 15th, January 2022 (Fan et al., Reference Fan, Marshall, Smolker, Gonzalez, Tapert, Barch and Herting2021). Data from the baseline and three follow-up time points were included.
The ABCD Study is a nationwide longitudinal study of brain, behavioral, and cognitive development in adolescents and involves 22 research sites throughout the United States, with more than 11,868 children recruited at baseline. Within these sites, public, private, and public charter schools within a 50-mile radius of the data-collecting site were randomly selected for recruitment (Garavan et al., Reference Garavan, Bartsch, Conway, Decastro, Goldstein, Heeringa and Zahs2018). Children aged 9–10 years old enrolled in selected primary schools were included for recruitment. Those with diagnoses of schizophrenia, autism spectrum disorder, substance use disorder, or medical or intellectual disability were excluded from the study (ABCD Study Team, 2024). Informed written consent was obtained from their parents and assent from children, and ethical approval was obtained from each site’s institutional review boards. We followed the Strengthening the Reporting of Observational Studies in Epidemiology (STROBE) reporting guidelines.
Participants
This study included 8,145 participants based on the availability of environmental exposures, outcome variables, and sociodemographic covariates. Supplementary efigure 1 shows a flowchart of included and excluded participants. Supplementary eTable 1 compares those included and excluded based on missing data.
Sociodemographic and clinical characteristics
Sociodemographic characteristics, including age, sex, race, ethnicity, parental education, household income, and family history of psychosis, were collected through parent reports and interviews during baseline assessment. Race and ethnicity were aggregated into five categories (non-Hispanic White, non-Hispanic Black, non-Hispanic Asian, non-Hispanic other races, and Hispanic), a schema commonly used in the ABCD study (Saragosa-Harris et al., Reference Saragosa-Harris, Chaku, MacSweeney, Guazzelli Williamson, Scheuplein, Feola and Mills2022). High parental education was defined as having at least one parent or caregiver who obtained a bachelor’s degree or higher. The income-to-needs ratio was calculated by the median value of the income band divided by the federal poverty line for the respective household size (Rakesh, Zalesky, & Whittle, Reference Rakesh, Zalesky and Whittle2022). A value greater or less than one would denote above or below the poverty threshold, respectively. Family history of psychosis in first-degree and second-degree relatives was assessed using the parent-rated Family History Assessment Module Screener (Van Dijk, Murphy, Posner, Talati, & Weissman, Reference Van Dijk, Murphy, Posner, Talati and Weissman2021).
Area-level exposures
Area-level data were derived from participants’ primary home addresses at baseline, which were geocoded to retrieve information at the census tract level. These geospatial location data were then linked to external environmental constructs, which were part of the ABCD 5.0 release (Fan et al., Reference Fan, Marshall, Smolker, Gonzalez, Tapert, Barch and Herting2021). Exposure variables were used to construct five domains, including the Area Deprivation Index (ADI) (Kind et al., Reference Kind, Jencks, Brock, Yu, Bartels, Ehlenbach and Smith2014), Child Opportunity Index 2.0 (COI) (Acevedo-Garcia et al., Reference Acevedo-Garcia, McArdle, Hardy, Crisan, Romano, Norris and Reece2014), Crime (Investigation, 2014), Environmental Quality (Requia et al., Reference Requia, Di, Silvern, Kelly, Koutrakis, Mickley and Schwartz2020), and Social Vulnerability Index (SVI) (Fatemi, Ardalan, Aguirre, Mansouri, & Mohammadfam, Reference Fatemi, Ardalan, Aguirre, Mansouri and Mohammadfam2017) based on their respective sources and prior literature (Fan et al., Reference Fan, Marshall, Smolker, Gonzalez, Tapert, Barch and Herting2021). A comprehensive list of variables included in our study, exposure definition, and years measured are described in Table 1 and Supplementary eTable 2. Distance to major roadways, population density, and percentage of households without a car, were used to classify the exposure profiles as urban, suburban, and rural as done in prior literature (Ostermeijer, Koster, Van Ommeren, & Nielsen, Reference Ostermeijer, Koster, Van Ommeren and Nielsen2022).
Table 1. Description of study sample by exposure profiles.
A. Study sample characteristics. B. Area-level deprivation index. C. Child opportunity index. D. Crime. E. Environmental quality. F. Social vulnerability index. G. Urbanicity features. H. Physical activities. I. Outcome variablea
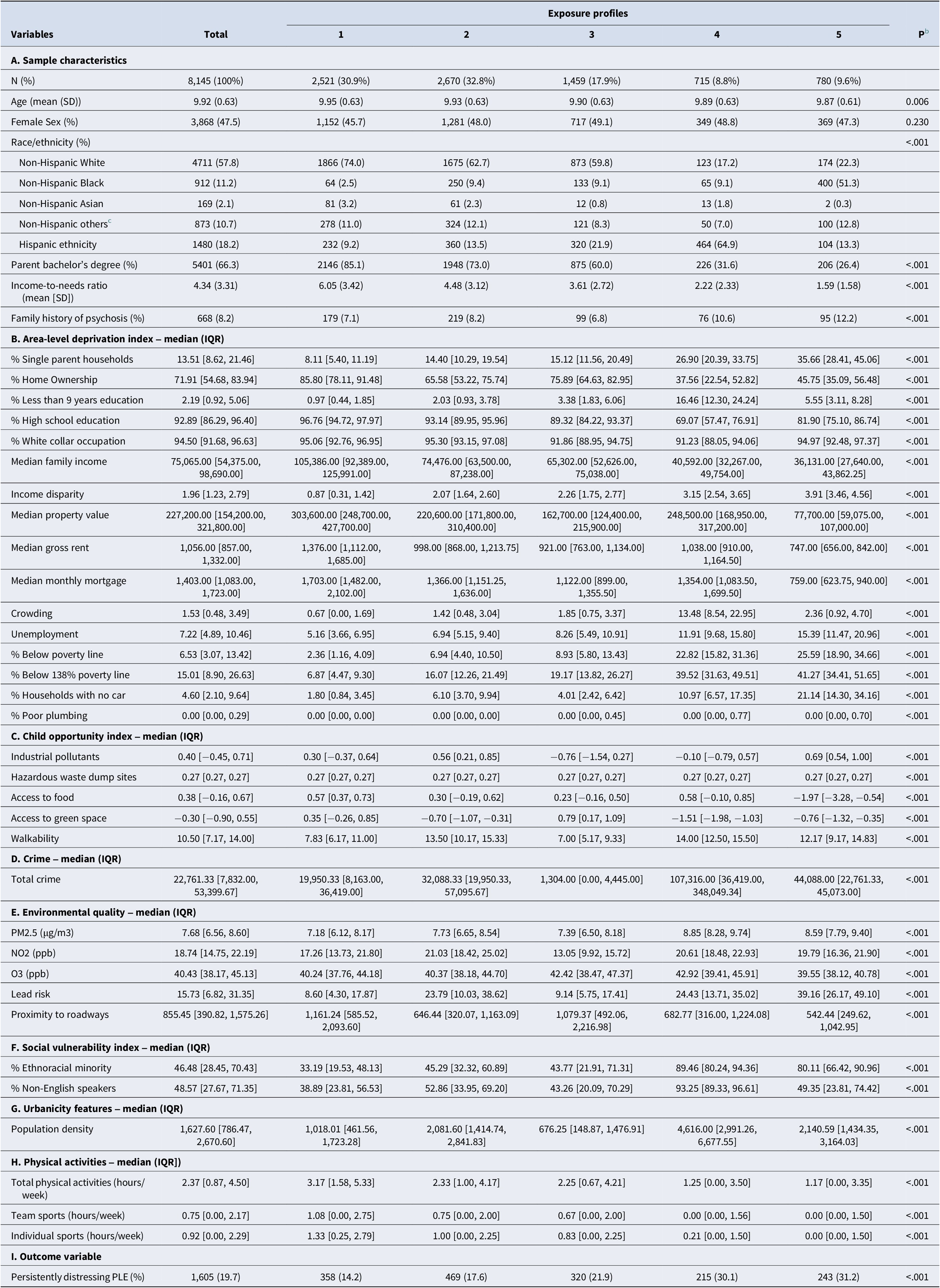
Note: SOM, Self-organizing map; INR, income-to-needs ratio; PLE, psychotic-like experiences.
a Detailed descriptions of the exposure components and years measured were summarized in Supplementary eTable 2 in the Supplementary Materials.
b P values correspond to the Kruskal–Wallis Rank Sum Test comparing medians, Fisher’s Exact test for proportions, and ANOVA comparing between means.
c Others included participants who identified as American Indian, Alaska Native, Native Hawaiian or Other Pacific Islander, Middle Eastern or North African, reported race not included in the list, did not know their race, did not disclose, or reported multiple races.
Persistent distressing psychotic-like experiences (PLE)
Participants completed the Prodromal Questionnaire-Brief Child Version (PQBC) to assess PLE (e.g. hallucinations, unusual thought content, paranoia, and other perceptual or thought disturbances) over the past month (Loewy, Pearson, Vinogradov, Bearden, & Cannon, Reference Loewy, Pearson, Vinogradov, Bearden and Cannon2011) (see Supplementary eMethods in supplement for the 21 questions assessing PLE). Initially, participants were categorized as either having experienced distressing PLE (rating of at least one PLE > = 3 on a five-point distress scale) or not having experienced distress, following criteria based on prior literature on distressing PLE (Karcher et al., Reference Karcher, Loewy, Savill, Avenevoli, Huber, Makowski and Barch2022). Participants with distressing PLEs were grouped into persistent and non-persistent categories, with “persistent” indicating distress at two or more follow-ups out of 4 waves (baseline, 1-year, 2-year, and 3-year). This binary categorization was based on prior literature demonstrating such persistency (as opposed to transiency) was specifically associated with a polygenic risk score for schizophrenia (B. S. Ku, Q. Yuan, et al., Reference Ku, Yuan, Arias-Magnasco, Lin, Walker, Druss and Guloksuz2024), greater cognitive deficits, worse social functioning, and more health service utilization (Karcher et al., Reference Karcher, Loewy, Savill, Avenevoli, Huber, Makowski and Barch2022) as well as a greater risk for future diagnosis of schizophrenia (Dominguez, Wichers, Lieb, Wittchen, & van Os, Reference Dominguez, Wichers, Lieb, Wittchen and van Os2011). Because psychotic symptoms may develop throughout adolescence, we conducted a sensitivity analysis and redefined persistent distressing PLE to incorporate individuals who reported distressing PLE only at the last follow-up.
Physical activities
Physical activities were derived from the Sports and Activities Involvement Questionnaire (SAIQ). Physical activities were further subcategorized into the team and individual sports (Supplementary eTable 3). The study focused on the level of involvement in 23 physical activities, with caregivers reporting (1) the time spent per session in minutes, (2) the number of days per week of participation, and (3) the number of months per year of engagement. The mean participation hours per week for each sport endorsed in the past 12 months were calculated using the formula: average hours per week per sport in the past year = (time spent × days per week × months per year) / 12/60. (Palmer et al., Reference Palmer, Sheth, Marshall, Adise, Baker, Chang and Yurgelun-Todd2021).
Statistical analysis
The self-organizing map (SOM) is an unsupervised machine learning technique for multi-dimensional data reduction and visualization, and identifying profiles of correlated characteristics (e.g. pollution concentrations and socioeconomic deprivation). SOM aims to map complex, multi-dimensional data into a simpler, easy-to-visualize format where multiple exposures are grouped into an exposure profile. SOM was used to cluster 29 area-level exposure profiles in this study. See Table 1 and Supplementary eTable 2 for a complete list of exposure variables. The optimal number of profiles was determined using within-cluster sum of squares, between-cluster sum of square statistics, and visual inspection of the exposure profile star plot (Christensen et al., Reference Christensen, Li, Pearce, Marcus, Lah, Waller and Hüls2022). These methods seek to maximize homogeneity within profiles and heterogeneity between profiles. The reference profile had the highest socioeconomic status and lowest hazardous environmental exposure (e.g. air pollution). Once participants were assigned to an exposure profile, unadjusted and adjusted logistic mixed models were used to estimate the association between exposure profiles, modeled as a categorical exposure, and persistent distressing PLE as well as physical activities (i.e. team and individual sports). The model adjusted for age, sex, family history of psychosis, race and ethnicity, parental education, and income-to-needs ratio, as individual-level fixed effects, and family groups and recruiting sites as random effects.
We then assessed whether team and individual sports would mediate the association between exposure profile and persistent distressing PLE. Mediation analysis was only conducted for exposure profiles that were statistically significantly associated with persistent distressing PLE by modeling each significant exposure profile as a dichotomous exposure variable in the mediation analysis. Detailed definitions and methodology for the mediation analyses were provided in the Supplementary eMethods.
Subsequently, we used another method, weighted quantile sum (WQS) regression, to understand the overall effect of the exposure mixture and how much of the total mixture effect on the outcome is explained by each area-level characteristic within the mixture. WQS is a method that estimates the effect on the outcome of increasing all exposures simultaneously by one quantile, as well as the weighted contribution of each exposure variable to the association between the overall exposure mixture and the outcome while accounting for the complex correlation structure among exposures (Carrico, Gennings, Wheeler, & Factor-Litvak, Reference Carrico, Gennings, Wheeler and Factor-Litvak2015; Renzetti, Gennings, & Calza, Reference Renzetti, Gennings and Calza2023). The detailed methodology of the WQS is summarized in Supplementary eMethods. All SOM analyses were performed using code within the ECM package (https://github.com/johnlpearce/ECM). The other analyses used the following R packages, lme4 (Bates, Mächler, Bolker, & Walker, Reference Bates, Mächler, Bolker and Walker2015), lmerTest (Kuznetsova, Brockhoff, & Christensen, Reference Kuznetsova, Brockhoff and Christensen2017), mediation (Tingley, Yamamoto, Hirose, Keele, & Imai, Reference Tingley, Yamamoto, Hirose, Keele and Imai2014), WQS (Renzetti, Curtin, Allan, Bello, & Gennings, Reference Renzetti, Curtin, Allan, Bello and Gennings2016). All analyses were conducted using R version 4.2.1. Statistical significance was determined using an alpha level of 0.05.
Results
Descriptive statistics
The study included 8,145 participants aged 9 to 10 years at baseline followed until 13 to 14 years, with 3,868 (47.5%) females, 5,566 (68.3%) non-Hispanic White, 956 (11.7%) non-Hispanic Black, 159 (2.0%) non-Hispanic Asian, and 1,480 (18.4%) Hispanic participants. Among the participants, 5,401 (66.3%) had parents with at least a bachelor’s degree and 668 (8.2%) had a family history of psychosis. Across the four years, 1605 (19.7%) participants had persistent distressing PLE (Table 1). Overall, 1157 (14.21%) of participants did not engage in any physical activity, 2983 (36.62%) and 2559 (31.42%) did not report participating in team sports and individual sports respectively. Individual neighborhood-level characteristics were correlated with each other in the expected direction (Supplementary eFigure 2).
Five distinct exposure profiles
Five exposure profiles were identified upon visual inspection. Each participant was assigned to one of the five profiles, and their demographics stratified by the exposure profiles are shown in Table 1. Figure 1a presents a detailed description of each profile.

Figure 1. Associations between exposure profiles and persistent distressing PLE.a
aSlices from the exposure profiles represent median values of a mixture component. Each circle represents an exposure profile. Profile 1: Suburban affluent areas (n = 2521, 30.9%), Profile 2: Suburban areas with high pollution concentrations (n = 2670, 32.8%), Profile 3: Rural areas with low walkability and high ozone (n = 1459, 17.9%), Profile 4: Urban areas with high ADI, crime, and pollution (n = 715, 8.8%), and Profile 5: Urban areas with high ADI and low access to food (n = 780, 9.6%).
bPink gradient slices correspond to Area-level Deprivation Index (ADI) variables, orange gradient slices correspond to Child Opportunity Index (COI) variables, green slices correspond to total crime, blue gradient slices correspond to environmental exposures, and purple gradient slices correspond to ethnoracial minority and non-English speaker concentration. All variables were coded such that higher scores indicate worse outcomes.
cAdjusted logistic regression model results of associations between exposure profiles and persistent distressing PLE, adjusted for age, sex, race and ethnicity, family history of psychosis, parental education, income-to-needs ratio, and included family and sites as random effects.
Profile 1 (n = 2521, 30.9%), the reference profile, was characterized as suburban affluent areas with the lowest socioeconomic deprivation, highest education attainment, and low pollution concentrations. Profile 2 (n = 2670, 32.8%) captured suburban areas with higher pollution concentrations, socioeconomic deprivation, and high crime rates compared to Profile 1. Profile 3 (n = 1459, 17.9%) were rural areas with low walkability, high ozone concentrations, and greater socioeconomic disadvantage but high greenspace and low industrial pollution levels. Profile 4 (n = 715, 8.8%) captured urban areas with high ADI, high crime rates, pollution concentrations, crowding, and minimal green space and homeownership. Profile 5 (n = 780, 9.6%) reflects urban areas with high ADI, low food access, income disparity, and high levels of industrial pollutants, alongside the lowest property values and incomes.
Associations between exposure profiles and persistent distressing PLE
Profile 3, characterized by rural areas with low walkability and high ozone, and Profile 4, characterized by urban areas with high ADI, crime, and pollution, were significantly associated with persistent distressing PLE compared to the reference Profile 1 (Profile 3: adjusted odds ratio (OR): 1.34, 95% CI: 1.09—1.64, p = .006; Profile 4: adjusted OR: 1.40, 95% CI: 1.08—1.81, p = .01) (Table 2, Figure 1b). Sensitivity analyses of (1) an alternative version of persistent distressing PLE and (2) only including participants who lived in their addresses for more than one year showed consistent results (Supplementary eTable 4). Variance inflation factors (VIF) for exposure profiles and individual-level covariates ruled out multicollinearity in our models (Supplementary eTable 5).
Table 2. Associations between exposure profiles and persistent distressing PLEa
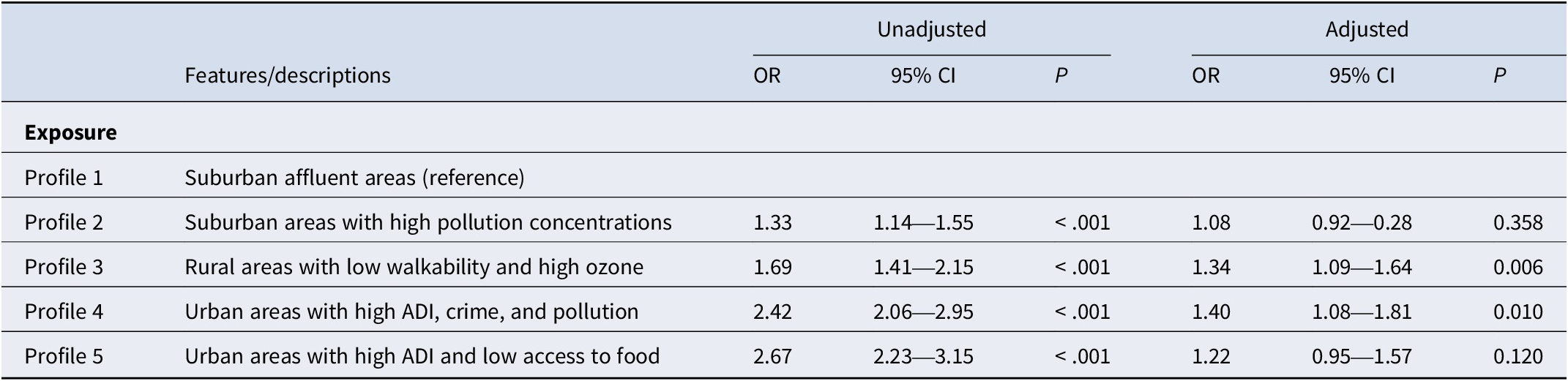
Note: ADI, area-level deprivation; and PLE, psychotic-like experiences.
a All models included recruiting sites and family groups as two random intercepts. The adjusted model adjusted for individual-level covariates: age, sex, race and ethnicity, family history of psychosis, parents with a bachelor’s degree, and income-to-needs ratio.
Mediation of the relationship between Profile 3 and persistent distressing PLE by team sports
Team sports explained 6.14% of the relationship between Profile 3 and persistent distressing PLE (indirect effects adjusted β: 0.002, 95% CI: <.001—.005, p = .013) (Figure 2). However, team sports did not significantly mediate the association between Profile 4 and persistent distressing PLE (Figure 2). Total physical activities or individual sports did not significantly mediate the association between Profile 3 or Profile 4 and persistent distressing PLE (Supplementary eTables 6 and 7).

Figure 2. Mediation of the relationship between Profile 3 and persistent distressing PLE by team sports.a
aMediation analysis was conducted for a subgroup of Profile 3 versus Profile 1 (n = 3980), and Profile 4 versus Profile 1 (n = 3236). The mediation analyses included age, sex, race, family history of psychosis, parents with a bachelor’s degree, and income-to-needs ratio as fixed effects and sites as random effects. The coefficients, confidence intervals, and p values of the indirect effects were ascertained by 5000 bootstraps using the mediate() function in the R mediation package (Tingley et al., Reference Tingley, Yamamoto, Hirose, Keele and Imai2014).
Individual exposure contribution assessed by WQS regression weights
The positively constrained model demonstrated that the overall effect of increasing all exposures simultaneously by one decile corresponded to 12% greater odds for endorsement of persistent distressing PLE (adjusted OR: 1.12, 95% CI: 1.02—1.23, p = .03). The tree plot presented in Figure 3 demonstrates the contribution of each exposure to a higher odds of persistent distressing PLE with greater total crime having the highest mean weight at 0.130, followed by lower walkability at 0.106, greater ethnoracial minority concentration at 0.090, higher ozone at 0.085, and greater industrial pollution levels at 0.077 (Supplementary eTable 8). The negatively constrained model was not significant (adjusted estimate: < .01, 95% CI: −0.10—0.09, p = .95) (Supplementary eTable 9).

Figure 3. Positive Constrained Weighted Quantile Sum (WQS) Regression Weights.a
aThe positively constrained model demonstrated that the overall effect of increasing all exposures simultaneously by one decile corresponded to 12% greater odds for endorsement of persistent distressing PLE (adjusted OR: 1.12, 95% CI: 1.02—1.23, p = .03). This tree plot demonstrates the contribution of each exposure to a higher odds of persistent distressing PLE. Mean weights represent the relative contribution of each mixture component to the overall mixture effect for persistent distressing PLE. A list of full names for mixture components and mean weights are shown in Supplementary eTable 8. Covariates included age, sex, family history of psychosis, race, and ethnicity, parents with at least a bachelor’s degree, and income-to-needs ratio.
Discussion
In this study, we identified five unique exposure profiles and investigated their associations with persistent distressing PLE and physical activities. We found two exposure profiles to be associated with greater odds of developing persistent distressing PLE. Rural areas that were less walkable with high ozone (Profile 3) and urban areas with high socioeconomic disadvantage, crime, and pollution (Profile 4) were both uniquely associated with greater odds for persistent distressing PLE even after adjusting for individual-level covariates. It is likely that a combination of factors, including financial stress, food insecurity, limited opportunities for physical activities, and the high concentration of pollution, might heighten the likelihood of PLE (Newbury et al., Reference Newbury, Heron, Kirkbride, Fisher, Bakolis, Boyd and Zammit2024). Chronic stress has been shown to disrupt the hypothalamic–pituitary–adrenal axis and elevate cortisol levels, which has been associated with neuroanatomical changes linked to psychotic illnesses (Katrina Aberizk et al., Reference Aberizk, Addington, Bearden, Cadenhead, Cannon, Cornblatt and Ku2023; K. Aberizk et al., Reference Aberizk, Collins, Addington, Bearden, Cadenhead, Cornblatt and Walker2022; Ku et al., Reference Ku, Collins, Anglin, Diomino, Addington, Bearden and Walker2023).
Subsequently, our mediation analyses suggested that there may be distinct biopsychosocial pathways through which the environment may play a role in the development of psychosis. For example, the degree of involvement in team sports (but not individual sports) modestly mediated the association between Profile 3 and PLE by more than 6%. However, none of the subcomponents of physical activities mediate such relationships for Profile 4. Although the association between Profile 3 and PLE was mainly driven by other factors rather than team sports, it is possible that involvement in team sports (e.g. pick-up basketball) and perhaps other informal social interactions may be less likely to occur in more rural and less walkable places (Aznar et al., Reference Aznar, Jimenez-Zazo, Romero-Blanco, Gómez, Homs, Wärnberg and Molina-García2024). This lack of social engagement with peers may play a role in the future risk of psychotic experiences (Glover, Todd, & Moyer, Reference Glover, Todd and Moyer2022).
Our findings demonstrating that the association between Profile 4 and PLE was not explained by physical activity suggest that there may be an alternative mechanism through which urban areas characterized by high crime and pollution may be associated with PLE. It is possible that threat from exposure to crime (Bhavsar, Boydell, Murray, & Power, Reference Bhavsar, Boydell, Murray and Power2014), air pollution (Newbury et al., Reference Newbury, Arseneault, Beevers, Kitwiroon, Roberts, Pariante and Fisher2019), and/or financial stress (St-Hilaire, Brunila, & Wachsmuth, Reference St-Hilaire, Brunila and Wachsmuth2024) may play a more important role for this subgroup of youth with these specific combinations of exposures. In fact, recent literature has suggested that deprivation and threat may be two environmental factors that underlie partially distinct biological pathways in the development of psychosis (Thomas et al., Reference Thomas, Rakesh, Whittle, Sheridan, Upthegrove and Cropley2023). Our findings may partially reflect this dimensional model of adversity in psychosis, and it is possible that different environmental factors may impact psychosis through distinct mechanisms.
In addition to the findings using SOM, the WQS regression model also points to similar factors that may drive the development of PLEs. High crime rates and low walkability carried the greatest weight in driving the effects of persistent distressing PLE, which align with high crime rates in Profile 4 and low walkability in Profile 3. Prior research has shown that living in high-crime neighborhoods was associated with subclinical psychotic symptoms, including suspiciousness and paranoia among help-seeking adolescents (Vargas et al., Reference Vargas, Rakhshan Rouhakhtar, Schiffman, Zou, Rydland and Mittal2020), as well as a higher incidence of first-onset schizophrenia (Bhavsar et al., Reference Bhavsar, Boydell, Murray and Power2014). Although walkability has not been widely studied in psychosis, places with less walkability tend to have less access to community services and recreational centers (Wang et al., Reference Wang, Narcisse and McElfish2023). Access to these resources may have downstream effects on exercise and involvement with team sports, which has been shown to be inversely associated with psychopathology and PLE (Hoffmann et al., Reference Hoffmann, Barnes, Tremblay and Guerrero2022).
One of the key strengths of this study is our approach to identifying multidimensional exposure profiles as measured by area-level characteristics, as opposed to traditional single-indexing methods. In addition, we used another exposure mixture method (i.e. WQS) to enhance the rigor of our findings and demonstrated consistent factors that may be driving the association with PLE. Future prospective studies should further investigate the biopsychosocial mechanisms through which neighborhood characteristics, including crime and walkability (and so forth types of crime, park access, street connectivity, and mixed land use) (Leyden, Hogan, D’Arcy, Bunting, & Bierema, Reference Leyden, Hogan, D’Arcy, Bunting and Bierema2024) may drive the development of psychotic disorders.
Limitation
This study has several limitations. First, we excluded several participants due to missing data. Participants excluded were from lower SES households, which may impact the findings’ generalizability. Second, PLEs were self-reported, and physical activities were parent-reported, which may be biased. Third, the outcome of this study is sub-threshold PLEs instead of clinical diagnoses of mental health disorders. Future studies should validate our findings using longitudinal clinical diagnosis data from ABCD follow-up assessments or data from different cohorts. Lastly, this study did not test whether there was a sensitive period in which environmental factors may have a more pronounced effect on long-term psychosis risk. Prospective studies should collect this data and analyze whether environmental factors at various developmental periods may differentially impact psychosis risk.
Conclusion
This study identified five exposure profiles, of which two were associated with persistent distressing psychotic-like experiences among children and adolescents across four years. These two exposure profiles were characterized by (1) rural areas with low walkability with high ozone (Profile 3), and (2) urban areas with high socioeconomic deprivation and high levels of pollution concentrations (Profile 4). Moreover, we found that less involvement with team sports partially mediated the positive association between Profile 3 (but not Profile 4) and greater odds of developing persistent distressing psychotic-like experiences. These findings suggest that there may be distinct mechanisms through which environmental factors may impact psychosis. Future studies should further explore the mechanisms through which living in certain neighborhoods may confer risk for the development of psychosis.
Supplementary material
The supplementary material for this article can be found at http://doi.org/10.1017/S0033291725000224.
Data availability statement
The ABCD study anonymized data are released annually and are publicly available via the NIMH Data Archive (NHA). All data from the Adolescent Brain Cognitive Development (ABCD) Study (https://nda.nih.gov/abcd/request-access) are made available to researchers from universities and other institutions with research inquiries following institutional review board and National Institute of Mental Health Data Archive approval.
Acknowledgments
This work was supported by the National Institute of Mental Health (NIMH K23-MH129684; Dr. Ku), the National Institute on Aging (NIA R01AG079170; Huels/Wingo), the National Institute of Mental Health (NIMH R01 MH129855; Risk), the Emory Constructive Collision Grant (Ku/Risk/Huels), and Emory HERCULES Pilot Award (Ku/Risk/Huels). The content is solely the responsibility of the authors and does not necessarily represent the official views of the National Institutes of Health, the National Institute of Mental Health, or Emory University.
Data used in the preparation of this article were obtained from the Adolescent Brain Cognitive DevelopmentSM (ABCD) Study (https://abcdstudy.org), held in the NIMH Data Archive (NDA). This is a multisite, longitudinal study designed to recruit more than 10 000 children age 9–10 and follow them over 10 years into early adulthood. The ABCD Study® is supported by the National Institutes of Health and additional federal partners under award numbers U01DA041048, U01DA050989, U01DA051016, U01DA041022, U01DA051018, U01DA051037, U01DA050987, U01DA041174, U01DA041106, U01DA041117, U01DA041028, U01DA041134, U01DA050988, U01DA051039, U01DA041156, U01DA041025, U01DA041120, U01DA051038, U01DA041148, U01DA041093, U01DA041089, U24DA041123, U24DA041147. A full list of supporters is available at https://abcdstudy.org/federal-partners.html. A listing of participating sites and a complete listing of the study investigators can be found at https://abcdstudy.org/consortium_members/. ABCD consortium investigators designed and implemented the study and/or provided data but did not necessarily participate in the analysis or writing of this report. This manuscript reflects the views of the authors and may not reflect the opinions or views of the NIH or ABCD consortium investigators. The ABCD data repository grows and changes over time.
Competing interest
The authors declare none.