No CrossRef data available.
Article contents
Who Gets Flagged? An Experiment on Censorship and Bias in Social Media Reporting
Published online by Cambridge University Press: 05 January 2023
Abstract
An abstract is not available for this content so a preview has been provided. Please use the Get access link above for information on how to access this content.
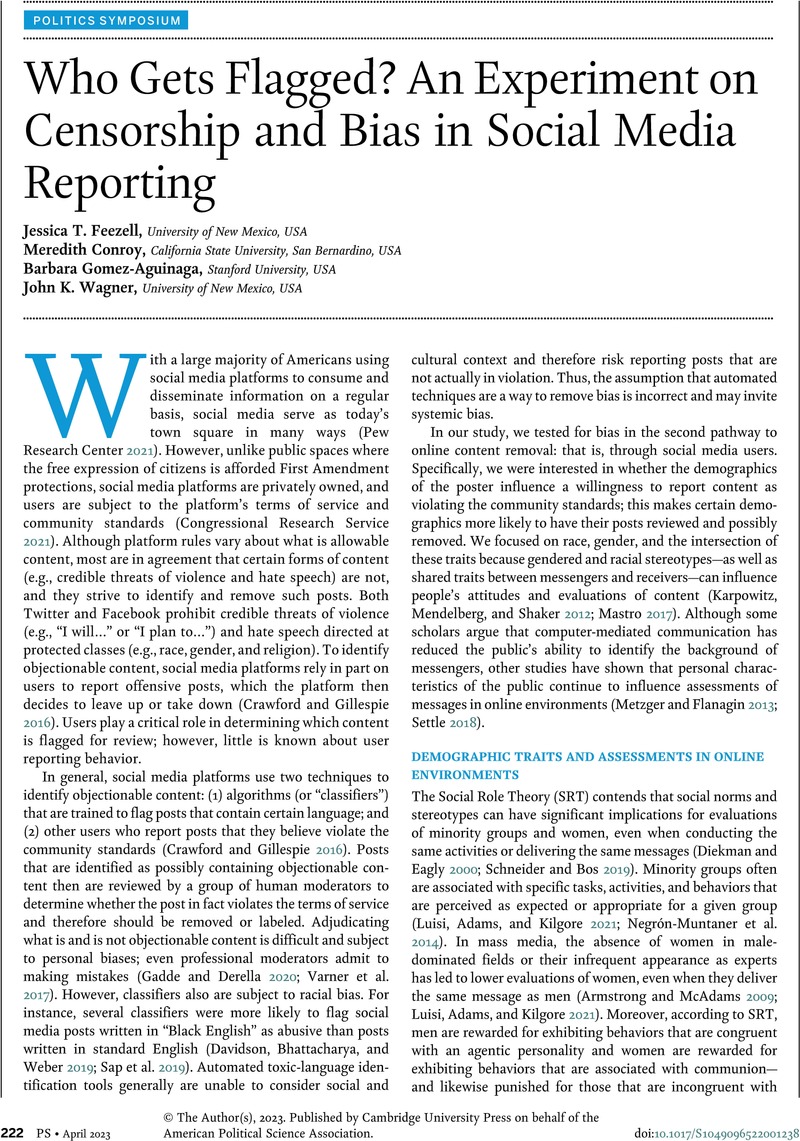
- Type
- Freedom of Expression in an Age of Social Media, Misinformation, and Political Polarization
- Information
- Copyright
- © The Author(s), 2023. Published by Cambridge University Press on behalf of the American Political Science Association
References
REFERENCES
Andsager, Julie L., and Mastin, Teresa. 2003. “Racial and Regional Differences in Readers’ Evaluations of the Credibility of Political Columnists by Race and Sex.” Journalism & Mass Communication Quarterly 80 (1): 57–72.CrossRefGoogle Scholar
Armstrong, Cory L., and McAdams, Melinda J.. 2009. “Blogs of Information: How Gender Cues and Individual Motivations Influence the Perceptions of Credibility.” Journal of Computer-Mediated Communication 14 (3): 435–56.CrossRefGoogle Scholar
Congressional Research Service. 2021. Social Media: Misinformation and Content Moderation Issues for Congress. Washington, DC: Congressional Research Service.Google Scholar
Crawford, Kate, and Gillespie, Tarleton. 2016. “What Is a Flag For? Social Media Reporting Tools and the Vocabulary of Complaint.” New Media & Society 18 (3): 410–28.CrossRefGoogle Scholar
Davidson, Thomas, Bhattacharya, Debasmita, and Weber, Ingmar. 2019. “Racial Bias in Hate Speech and Abusive Language Detection Datasets.” Florence, Italy: Proceedings of the Third Workshop on Abusive Language Online: 25–35.CrossRefGoogle Scholar
Diekman, Amanda B., and Eagly, Alice H.. 2000. “Stereotypes as Dynamic Constructs: Women and Men of the Past, Present, and Future.” Personality and Social Psychology Bulletin 26 (10): 1171–88.CrossRefGoogle Scholar
Feezell, Jessica T., Conroy, Meredith, Gomez-Aguinaga, Barbara, and Wagner, John K.. 2022. “Replication Data for ‘Who Gets Flagged? An Experiment on Censorship and Bias in Social Media Reporting.’” PS: Political Science & Politics DOI: 10.7910/DVN/WM6AOP.CrossRefGoogle Scholar
Gadde, Vijaya, and Derella, Matt. 2020. “An Update on Our Continuity Strategy During COVID-19.” https://blog.twitter.com/en_us/topics/company/2020/An-update-on-our-continuity-strategy-during-COVID-19.Google Scholar
Karpowitz, Christopher F., Mendelberg, Tali, and Shaker, Lee. 2012. “Gender Inequality in Deliberative Participation.” American Political Science Review 106 (3): 533–47.CrossRefGoogle Scholar
Koenig, Annie M., and Eagly, Alice L.. 2014. “Evidence for the Social Role Theory of Stereotype Content: Observations of Groups’ Roles Shape Stereotypes.” Journal of Personality and Social Psychology 107 (3): 371–92.CrossRefGoogle ScholarPubMed
Lee, Jayeon, and Lim, Young-Shin. 2014. “Who Says What About Whom: Young Voters’ Impression Formation of Political Candidates on Social Networking Sites.” Mass Communication & Society 17 (4): 553–72.CrossRefGoogle Scholar
Luisi, Tim, Adams, Kelly L., and Kilgore, LaShawnda. 2021. “Roughing the Caster! Sexism and Perceived Female Sports Broadcasters Credibility.” Atlantic Journal of Communication 29 (4): 262–74.CrossRefGoogle Scholar
Marmat, Geeta. 2022. “Online Brand Communication and Building Brand Trust: Social Information Processing Theory Perspective.” Global Knowledge, Memory, and Communication 71 (6/7): 584–604.CrossRefGoogle Scholar
Mastro, Dana. 2017. “Race and Ethnicity in US Media Content and Effects.” Oxford Research Encyclopedia of Communication. https://doi.org/10.1093/acrefore/9780190228613.013.122.CrossRefGoogle Scholar
Metzger, Miriam J., and Flanagin, Andrew J.. 2013. “Credibility and Trust of Information in Online Environments: The Use of Cognitive Heuristics.” Journal of Pragmatics 59 (B): 210–20.CrossRefGoogle Scholar
Negrón-Muntaner, Frances, Abbas, Chelsea, Figueroa, Luis, and Robson, Samuel. 2014. The Latino Media Gap: A Report on the State of Latinos in U.S. Media. www.scribd.com/document/230135450/Latino-Media-Gap-Report-by-Frances-Negron-Muntaner-with-Chelsea-Abbas-Luis-Figueroa-and-Samuel-Robson.Google Scholar
Pew Research Center. 2021. “Social Media Fact Sheet.” Washington, DC: Pew Research Center. www.pewresearch.org/internet/fact-sheet/social-media.Google Scholar
Sap, Maarten, Card, Dallas, Gabriel, Saadia, Choi, Yejin, and Smith, Noah A.. 2019. “The Risk of Racial Bias in Hate Speech Detection.” Florence, Italy: Proceedings of the 57th Annual Meeting of the Association for Computational Linguistics: 1668–78.CrossRefGoogle Scholar
Schneider, Anne, and Ingram, Helen. 1993. “Social Construction of Target Populations: Implications for Politics and Policy.” American Political Science Review 87 (2): 334–47.CrossRefGoogle Scholar
Schneider, Monica C., and Bos, Angela L.. 2019. “The Application of Social Role Theory to the Study of Gender in Politics.” Political Psychology 40 (S1): 173–213.CrossRefGoogle Scholar
Settle, Jaime E. 2018. Frenemies: How Social Media Polarizes America. Cambridge: Cambridge University Press.CrossRefGoogle Scholar
Todorov, Alexander, and Porter, Jenny M.. 2014. “Misleading First Impressions: Different for Different Facial Images of the Same Person.” Psychological Science 25 (7): 1404–17.CrossRefGoogle ScholarPubMed
Varner, Madeleine, Tobin, Ariana, Angwin, Julia, and Larson, Jeff. 2017. “What Does Facebook Consider Hate Speech?” New York: ProPublica. https://projects.propublica.org/graphics/facebook-hate.Google Scholar
Wojcik, Stefan, and Hughes, Adam. 2019. “Sizing up Twitter Users.” Washington, DC: Pew Research Center. www.pewresearch.org/internet/2019/04/24/sizing-up-twitter-users.Google Scholar