- DLW
-
doubly labelled water
- EE
-
energy expenditure
- HSE
-
Health Survey for England
- MET
-
metabolic equivalent
- MVPA
-
moderate or vigorous physical activity
- NDNS
-
National Diet and Nutrition Survey
- PAEE
-
physical activity energy expenditure
Regular physical activity is important to promote physical and mental health and well-being( 1 , 2 ). Physical activity is associated with reductions in all-cause mortality( Reference Bull, Armstrong, Dixon and Ezzati 3 , 4 ) obesity, diabetes( Reference Kesaniemi, Danforth and Jensen 5 ), hypertension, CVD( Reference Bull, Armstrong, Dixon and Ezzati 3 ) certain cancers( Reference Bull, Armstrong, Dixon and Ezzati 3 , 4 ), osteoporosis( Reference Wolman 6 ) and depression( Reference North, McCullagh and Tran 7 ). Insufficient physical activity costs about £8·2×109 ($12·7×109, €9·7×109) annually in England (2002 values)( 8 ). In children, physical activity is important for maintenance of a desirable weight for height, for well-being and to inculcate lifelong healthy habits. It also has more immediate health benefits, improving motor development and psychosocial health and reducing adiposity in pre-school children( Reference Timmons, LeBlanc and Carson 9 ). In school-aged children, periodic physical activity increases physical health and attention and improves behaviour, attitudes and educational achievement( Reference Burkhalter and Hillman 10 ), and mental function in adolescents( Reference Ardoy, Fernández-Rodríguez and Jiménez-Pavón 11 ). Physical activity is important for optimal bone and muscle development( Reference Timmons, LeBlanc and Carson 9 , Reference Ardoy, Fernández-Rodríguez and Jiménez-Pavón 11 ); activity sufficient to improve aerobic fitness improves cardiometabolic profiles( Reference Timmons, LeBlanc and Carson 9 , Reference Andersen, Riddoch and Kriemler 12 , Reference Tompkins, Moran and Preedom 13 ).
Assessment of physical activity in health surveys is undertaken mostly to monitor adherence to recommendations or identify population subgroups to target with interventions. However, in dietary surveys, physical activity data are usually collected to enable energy expenditure (EE) to be estimated, not monitor physical activity levels per se.
Accurate assessment of physical activity is difficult, particularly in children, because frequency, duration and intensity each need to be assessed for a potentially large range of activities. These may be structured exercise or sports; informal activity within daily life, such as active travel (walking or cycling); leisure; occupational activity (e.g. newspaper delivery); and informal play, which accounts for most physical activity in pre-school children and a substantial but diminishing proportion in older children. Additionally, physical activity may be regular or may vary considerably from week to week, including the weather, seasonal sports and whether school term or holiday.
Participant (and parental) burden is an important constraint on measuring physical activity, as dietary surveys generally require considerable effort by participants, which can cause issues both for research ethics and of depressed response rates. Some measures that are feasible in a laboratory-based study involving small numbers of children, such as indirect calorimetry, are impractical in larger population surveys. Direct observation of participants by trained researchers is intrusive and labour intensive (so expensive and unrealistic) but also inaccurate, although it can provide context.
Physical activity can be assessed in two main ways: by self-report (or parental proxy reports for children aged <10 years) or objectively through using equipment that measures movement and/or physiological responses to physical exertion. The advantages and problems of the many subjective and objective methods of assessing physical activity for surveillance and epidemiological purposes have been reviewed comprehensively( Reference Warren, Ekelund and Besson 14 ). Earlier reviews considered assessment of physical activity in children( Reference Trost 15 ) and in pre-school children( Reference Oliver, Schonfield and Kolt 16 ). The present paper is aimed at people commissioning or conducting dietary surveys in children (defined broadly to indicate non-adults) who are not experts in measuring physical activity. It summarises issues that may occur, and provides some examples from the authors’ experience from the Health Survey for England (HSE)( Reference Mindell, Biddulph and Hirani 17 , Reference Craig, Mindell and Hirani 18 ) and the UK National Diet and Nutrition Survey (NDNS)( Reference Scholes, Mindell, Bates, Page and Swan 19 ) of unexpected practical issues that can arise, and how these may be overcome.
Subjective assessment of physical activity
Self-report recall questionnaires
Questionnaires, asking about past activity over a predefined time frame, may be self-completed or administered by an interviewer. Children younger than 10 years do not have the capacity to report their activities accurately( Reference Baranowski, Dworkin and Cieslik 20 ). Parental proxy answers may be no better at accurate reporting: they cannot report school-based activity, and informal play is particularly difficult to assess. In 2006, we undertook a desk-based review of all physical activity questionnaires used in diet and nutrition studies. We concluded that physical activity in children under 11 years could not be measured in sufficient detail by the use of questionnaires to estimate EE. A 2010 review found that no questionnaires for children were both reliable and valid, with worse results in younger children than adolescents( Reference Chinapaw, Mokkink and van Poppel 21 ).
Short questionnaires often enquire about the frequency and average duration of categories of activity (usually light, moderate and vigorous) over a pre-specified time frame, giving examples of typical activities within each group, based on the metabolic equivalent (MET; 1 MET=the rate of energy expenditure while sitting quietly) intensity coding scheme developed by Ainsworth and colleagues( Reference Ainsworth, Haskell and Herrmann 22 ). Despite the advantage of less respondent burden, they provide insufficient detail to estimate EE. Detailed questionnaires ask which activities the survey participant have done in a given time frame, and for ‘each activity’ asks about the frequency and average duration, and questions to ascertain the intensity. For example, whether the activity makes the respondent breathless, hot or sweaty (or not) would categorise cycling as vigorous or moderate, respectively( Reference Ainsworth, Haskell and Herrmann 22 ).
Questionnaires are relatively quick, inexpensive, and easy to administer but have many important limitations( Reference Bailey, Olson and Pepper 23 ). A major problem is recall bias. Questionnaires asking about habitual activity( Reference Corder, Ekelund and Steele 24 ) risks responses reporting ‘most active weeks’ instead of ‘usual’ activity or activity in the specified time period, most commonly for children the previous 7 d( Reference Craig, Mindell and Hirani 18 ). Shorter time periods give greater recall accuracy but determine irregular activities less accurately. In some individuals, light activity can be an important component of physical activity energy expenditure (PAEE)( Reference Westerterp and Plasqui 25 ) but light activity is extremely difficult to define and capture using self-report or proxy methods. In children, physical activity is sporadic in nature, being characterised by short bursts: during informal play up to 96 % of activity may occur in bouts <10 s( Reference Corder, Ekelund and Steele 24 ). Social desirability bias may play a part among adolescents( Reference Jago, Baranowski and Baranowski 26 ).
Physical activity of children aged 2–15 years was first assessed in the HSE in 1997 (by parental proxy interview for children aged 2–11 years and self-report for those aged 13–15 years). Differing instruments were used subsequently; a new questionnaire was developed de novo for HSE 2008( Reference Craig, Mindell and Hirani 18 ). For the 2012 HSE, additional questions were added, to assess adherence to national recommendations for muscle strengthening exercises as well as sufficient weekly duration moderate or vigorous physical activity (MVPA)( Reference Scholes, Mindell, Craig and Mindell 27 ). In older children, self-report questionnaires overestimated activity levels compared with accelerometry( Reference Craig, Mindell and Hirani 18 ); in younger children, parental proxy questionnaires substantially underestimated MVPA (Fig. 1)( Reference Craig, Mindell and Hirani 18 ).
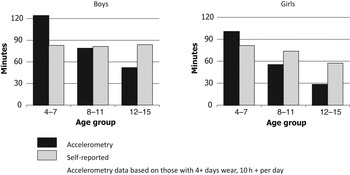
Fig. 1. Comparison of accelerometry data with reported average daily moderate or vigorous physical activity time in children.
For the rolling programme UK NDNS, a new questionnaire for teenagers was developed to attempt to estimate PAEE accurately at the individual level, by including detailed questions on all forms of activity. It performed well at the population level in categorising participants into activity levels but was not accurate at an individual level when compared with doubly labelled water (DLW; an objective measure of EE used in dietary surveys)( Reference Scholes, Mindell, Bates, Page and Swan 19 ).
For questionnaires there is a trade-off between completeness of the information collected, balancing how comprehensive the questionnaire is with participant burden and the risks of missing data items and/or lack of co-operation on further stages of the dietary survey. In practice, questionnaires have been the most frequently used method for recording physical activity, although there is a trend towards moving to more objective methods for children in population surveys( Reference Trost 15 , Reference Oliver, Schonfield and Kolt 16 ).
Assessing energy expenditure from physical activity questionnaires
Population-level assessment of EE may be required to compare changes over time with changes in energy intake and obesity prevalence, or to compare population subgroups. Accurate assessment of individuals’ EE is generally used in dietary surveys for comparison with recorded intake, to assess the accuracy of reported diet. Where EE assessment is not sufficiently accurate, underreporting can be assessed by grouping participants into EE categories for comparison of reported intake.
Questionnaires that cover all physical activity domains contribute significantly to EE and in sufficient detail to record frequency, duration and intensity of activity, are very long but fail to provide accurate EE measures at the individual level because such precise recording of activity intensity and duration across all levels of intensity is not feasible. EE assessment using questionnaires is still a common technique to assess free living EE, although seldom valid due to inadequate coverage of all activity domains and inaccurate assignment of MET values (EE) to reported activities, particularly in children. In NDNS, the population means derived from the questionnaire and from the DLW data were close but participants could not be assigned into meaningful EE categories because of the large variance in the results( Reference Mindell, Stamatakis and Brage 28 ).
Validation may be hampered by use of different time periods( Reference Neilson, Robson and Friedenreich 29 ). If the DLW collection period does not overlap fully with the other measurements (other physical activity data, dietary intake), results will contain additional noise among those participants whose activity pattern is unstable with considerable between-day or between-week variation( Reference Scholes, Mindell, Bates, Page and Swan 19 , Reference Walsh, Hunter and Sirikul 30 ).
Activity diary or log
Use-of-time tools have moderate validity and reliability for assessing young people's physical activity and EE( Reference Foley, Maddison and Olds 31 ). An activity log requires the individual, parent or other responsible adult to record at regular time intervals what activity or activities have been undertaken in that time period. As with questionnaires, young children cannot do this and parents are often not present. Despite the theoretical advantage of better completion, as minimal recall is required, there is a trade-off concerning the time interval selected: the shorter the duration, the more accurately physical activity can be recorded but more frequent recordings add considerably to participant burden and therefore reduce completeness and/or accuracy( Reference Corder, Ekelund and Steele 24 ).
A diary requires the participant to record specific groups of activities. However, diaries usually collect information on MVPA only as a proxy for EE, which is not ideal as light intensity activities, such as standing and non-brisk walking, may contribute substantially to overall PAEE. Activity diaries, used in the first UK NDNS of children( Reference Gregory, Lowe and Bates 32 ), give valid data in adolescents for estimating physical activity category and total EE, assessed by DLW measurement( Reference Bratteby, Sandhagen and Fan 33 ) but add to the participant burden. Electronic diaries have been validated against accelerometry in children( Reference Floro, Dunton and Delfino 34 ) but are relatively costly and are not commonly used in dietary surveys in children.
Objective measures of physical activity
The gold-standard for measuring EE in dietary surveys is through the use of DLW( Reference Bluck 35 ). Survey participants are asked to drink a measured dose of water labelled with two, non-radioactive (stable), naturally occurring isotopes, 2H and 18O, which are assayed in urine in one pre-dose sample and one sample daily for ten consecutive days. Although the most accurate method in regular use, inaccuracies in EE can occur through incomplete consumption of the dose or inaccurate recording of the urine samples, or occasionally poor laboratory techniques. The method is safe but ensuring the entire dose is consumed and collecting the urine samples is difficult in the youngest children.
Monitoring physical activity can be undertaken by recording movement and/or physiological changes( Reference Haskell, Leon and Caspersen 36 ). Such devices avoid problems of recall of frequency and duration and subjective assessment of the intensity. However, they are not infallible and have both theoretical and practical difficulties in use. Similarly to DLW, there is no contextual information about what the individual was doing at the time, unless a diary or log is also kept, but this adds to the respondent burden, reducing response or compliance rates, and adds subjective errors to the objective measure. In dietary surveys, the context is generally not crucial, provided the total volume of physical activity is monitored reasonably accurately. Another important issue pertinent to dietary survey methods is the difficulty translating measured activity into EE.
Pedometers
Pedometers measure steps taken. They can be a useful motivational tool to encourage activity( Reference Beets, Patton and Edwards 37 , Reference Duncan, Schofield and Duncan 38 ). Their main advantages are their low cost, compared with other devices, and the simplicity of the data produced: the number of steps taken. They are therefore useful for large population surveys( Reference Eston, Rowlands and Ingledew 39 ), and were used in the Australian National Survey of Children's Diet and Nutrition( 40 ). However, they cannot capture other forms of activity, including cycling and swimming, nor increased EE from walking on an incline or carrying a load. They have been validated in children( Reference McNamara, Hudson and Taylor 41 ), including adolescents( Reference Jago, Watson and Baranowski 42 ), but are not recommended for pre-school children( Reference Oliver, Schofield and Kolt 43 ).
Dietary surveys need research-grade pedometers that have adequate performance criteria( Reference Tudor-Locke, Sisson and Lee 44 ). They also need to be calibrated, although this is simple, as step size depends on age and speed( Reference Trost 15 ). While some authors have found pedometer data can estimate children's EE reasonably accurately( Reference Corder, Ekelund and Steele 24 , Reference Eston, Rowlands and Ingledew 39 ), most recommend that the data be analysed only as the number of steps taken( Reference Corder, Brage and Ekelund 45 ).
Accelerometers
Accelerometers, introduced in the early 1980s( Reference Matthews 46 ), have been used to assess physical activity and to validate self-reported physical activity. They provide objective, standardised data without recall bias and other subjectivity problems but participants’ co-operation is required to wear the monitor during waking hours( Reference Roth and Mindell 47 ) and, if required, to record activities when not worn.
Accelerometers can measure directly the frequency, duration and intensity of most aspects of physical activity. The greater precision of the objective data yields equivalent statistical power with lower sample sizes. Accelerometer counts increase almost proportionally with ambulatory speed( Reference Matthews 46 ), so are excellent for recording time being inactive and time spent on dynamic activity such as walking or running up to a certain speed. However, they are less good at recording static activity, often important for overall EE estimation. Non-wear time can also be an important issue. In relation to estimating EE, the main issue is that the equations for conversion from accelerometers to EE perform poorly in non-controlled free-living situations( Reference Corder, Ekelund and Steele 24 ).
Accelerometers can measure movement in one, two or three dimensions (uni-, bi- or tri-axial measurements). Uni-axial accelerometers produce data that are the simplest to interpret but are limited by the lack of detection of movement in other directions; for example, rowing and cycling are poorly detected. Uni-axial monitors may also not detect inclines; walking more slowly up a 5 % hill may use more energy than walking faster on the level. No devices detect load-carrying, also underestimating EE while carrying a heavy school bag, for example. Most accelerometers are not water-proof, and must therefore be removed for swimming. Some ethics committees may also impose restrictions, e.g. to instruct participants to remove the device when participating in contact sports, including martial arts.
After the accelerometers have been worn, the devices need to be returned or collected, so that data can be downloaded from the devices and processed. Data processing of accelerometer data includes differentiating between periods of non-wear and low counts indicating inactivity (sedentary behaviour); decisions about minimum wear time for inclusion in analysis (minimum number of hours worn per day (‘sufficient wear’) and minimum number of days of sufficient wear)( Reference Roth and Mindell 47 ); which thresholds to use to categorise activity as sedentary, light, moderate or vigorous( Reference Ainsworth, Haskell and Herrmann 22 ); and which equations to use to estimate MET or EE, if that is intended. Data processing has become much more sophisticated over time. For example, probability modelling is replacing a dichotomous decision of whether or not a device was deemed to be worn at any particular moment, and therefore whether that reading should be included.
Accelerometry has been used for population surveillance. The US National Health and Nutrition Examination Survey was the first general population study to use accelerometry in children and adults in a population-wide survey, in 2003/04( 48 ). They used accelerometers with over 1000 participants, aged 6 years and over, demonstrating the feasibility of objective measurement in large population studies. The first surveys to issue accelerometers ‘in the field’ occurred in England( Reference Craig, Mindell and Hirani 18 , Reference Scholes, Mindell, Bates, Page and Swan 19 , Reference Mindell, Stamatakis and Brage 28 , Reference Roth and Mindell 47 , 48 ). Both the HSE and NDNS used the Actigraph model GT1M, a lightweight, digital, accelerometer, smaller than a matchbox. Movements outside normal human motion are filtered out electronically( Reference Rothney, Apker and Song 49 ). The filtered acceleration signal is digitised and summed over a specified time interval to produce a number of ‘counts’ for that time period, which is stored and the summing restarts. We used a 1 min period, to maximise data storage, but shorter periods give more detailed assessment of short bursts of activity, characteristic of children. In the NDNS, a subsample of children provided DLW estimates over 10 d, concurrent with accelerometer wear( Reference Mindell, Stamatakis and Brage 28 ).
The choice of Actigraph was based on cost, ease of use and compliance, reliability, adequacy for physical activity assessment( Reference Trost, Way and Okely 50 , Reference Pate, Almeida and McIver 51 ), and a wide comparison base. Participants in the HSE and NDNS were asked to wear the accelerometer on their waist, positioned above the right hip, during waking hours for seven consecutive days.
Practicalities of using accelerometers
Accelerometers need to be charged and initialised (to enable fresh data collection) before being given to participants. Initially, this was done centrally by the operations department then sent to interviewers to issue to eligible participants. The instruments were collected by the survey nurse or interviewer at the next planned visit, or an additional visit, or could be posted back using a pre-paid addressed envelope. On receipt by the operations centre, the instrument was logged against the participant identification, the data downloaded, and the device recharged and reinitialised prior to being sent back to the field staff for use with a new participant. However, where there is a subsequent delay before the accelerometers are reissued, it is better for the devices to be charged and initialised by the field staff, to minimise data loss from inadequate charge remaining during the data collection period.
Accelerometry compliance
In the NDNS Comparison Study, of the sixty-four children aged 4–15 years who agreed to participate in the accelerometry sub-study, 95 % wore the accelerometer for ≥500 min daily for at least 4 d. Variations in the time spent wearing an Actigraph by age were not significant( Reference Mindell, Stamatakis and Brage 28 ).
Participants (or their parents) were asked to keep a log of when they put on and removed a device, what they were doing while it was not worn (e.g. if removed because of swimming or contact sports, thus not measuring significant activity), or when they undertook activity not recorded by the device (e.g. rowing or cycling if using a uni-axial accelerometer). Inclusion of log book information required lengthy and often manual processing and affected EE estimation very little. Larger population studies using accelerometers do not ask participants to complete a log, because of the additional errors added by inaccurate record keeping by participants and the need of researchers to make assumption to use those data( Reference Craig, Mindell and Hirani 18 ). The log was therefore dropped from the NDNS in later years.
Assessing energy expenditure from accelerometry data
EE estimates based on assessment of physical activity differ considerably depending on which equations are used to convert physical activity to EE. There are additional inaccuracies from estimating the individual's BMR( Reference Henry 52 ): as with all models, this approximation fits population-level data while being inaccurate for individuals, particularly those with extremes of age, body mass or composition. Additional inaccuracies ensue when there is also measurement error in the assessment of physical activity; EE can be assessed more accurately using objective than self-reported physical activity measures.
There are a number of problems with using accelerometers to assess EE, particularly from free-living data. Calibration is usually performed by walking and running at different speeds on a treadmill. Age and leg length affect the relationship( Reference Stone, Esliger and Tremblay 53 ). Simulation of ‘daily activities’ in the laboratory have produced very different equations for estimating EE( Reference Corder, Ekelund and Steele 24 ) but about 90 % of the data collected lie outside the laboratory range used in deriving the conversion equations( Reference Corder, Ekelund and Steele 24 ); PAEE is zero for complete inactivity, so mathematical methods adjust the equations to ensure this( Reference Brage, Brage and Franks 54 ). Equations used most often for providing estimates of total EE (BMR+PAEE) for children are those by Trost et al. ( Reference Trost, Kerr and Ward 55 ) and by Puyau et al.( Reference Puyau, Adolph and Vohra 56 )
For NDNS, the Actigraph data resulted in a greater underestimate of EE than the questionnaire at the population level but with acceptable variance( Reference Mindell, Stamatakis and Brage 28 ). The Year 4 NDNS report presented counts per minute, to avoid additional errors from assumptions included in converting counts per minute to EE( Reference Scholes, Mindell, Bates, Page and Swan 19 ).
Heart rate monitors
Heart rate monitors are usually worn on a strap around the chest. Measuring changes in heart rate can be a better assessment of physical activity in relation to cardiovascular impact but is not responsive to low-intensity activity and cannot distinguish between different causes of raised heart rates (activity, pain and other emotional stimuli). This is the main issue in free-living studies, where measurement ‘noise’ is more of a problem for heart rate than for motion sensors( Reference Stegle, Fallert and MacKay 57 ). There are also problems of latency, with the heart rate change lagging behind changes in activity, and heart rate elevation persisting after cessation of activity, particularly in less fit individuals. This may conceal intermittent activity, particularly in children.
Combined heart rate and activity monitors
Monitors that detect both movement and heart rate provide more accurate assessment of energy use( Reference Avons, Garthwaite and Davies 58 ). Accelerometry provides accurate data for sedentary and light activity, where heart rate monitors contribute less. Monitoring the heart rate allows the intensity of the activity to be captured more accurately. Such devices can also distinguish well between non-wear and sedentary behaviour, and can help describe the intensity and duration of activity more accurately, although requiring analysis of more complex data. They may provide more accurate assessment of EE( Reference Corder, Ekelund and Steele 24 ), including in children( Reference Eston, Rowlands and Ingledew 39 , Reference Corder, Brage and Wareham 59 ). However, calibration is required for each individual to optimise data interpretation( Reference Brage, Ekelund and Brage 60 ). Current combined sensor technology can be relatively burdensome for the participant (e.g. certain devices require removal of hair from men's chest) and there is a risk that gains in accuracy may be offset by losses in participant adherence. Furthermore, at the time of preparing this report, combined sensors are still very expensive and beyond the reach of most dietary surveys.
Discussion
Expected lack of correlation between questionnaire data and objective measures
One criticism levelled at questionnaire data is the low correlation found with objective measures and, in particular, the much lower activity levels recorded by accelerometers than from questionnaire responses (except in young children where the opposite is found, Fig. 1). However, they measure different things. For example, while individuals report the amount of time spent in a session called ‘playing football’, including time spent organising the game, or moving little while play is elsewhere on the pitch, an accelerometer measures the amount of time during that session that the individual is moderately or vigorously active. For assessment of physical activity against recommendations, questionnaires are valid, given that the heath outcome-based guidance is currently based solely on self-reported activity.
However, for assessing EE, accelerometry is potentially much more accurate, as it can provide information on the amount of time spent in light activity, which contributes substantially to EE because of the much longer accumulated duration in most individuals, and in particular, the great difficulty in recording ‘active play’ and other informal activities among younger children. This greater accuracy needs to be weighed against the greater capital and running costs of the survey, the adherence to wearing the device required of participants and the more complex data processing.
Recent advances and future prospects
Accelerometers have changed substantially over the past few years. In addition to development of tri-axial monitors, newer devices have been validated for wear on the wrist( Reference Ekblom, Nyberg and Bak 61 ), improving acceptability. Cut-points have been validated for MVPA for children( Reference Schaefer, Nace and Browning 62 ) but data from hip-worn accelerometers should not be compared with wrist-worn accelerometers( Reference Routen, Upton and Edwards 63 ), with discrimination between activity intensities better when worn on the hip rather than on the wrist( Reference Phillips, Parfitt and Rowlands 64 ). Newer devices can output raw acceleration signals, offering promise for the future not only for quantification of physical activity but also pattern recognition.
Waterproof devices are now being marketed, so activity while swimming could also be recorded. However, early experience in a survey in Hawaii showed high failure rates: to enable data downloads, the devices cannot be fully sealed and are thus not fully waterproof (C Boushey, personal communication). Devices are being developed that combine motion sensors with other physiological measurements, such as pulse or blood pressure, to increase assessment accuracy for activity and EE.
Computational developments now permit more complex assessment of movement from accelerometry and similar devices. These include non-linear modelling techniques controlled by the investigator, and non-integrated acceleration signals to create an artificial neural network to replace the more limited information from uni-axial accelerometry. A commercially available monitor uses five accelerometers attached to the skin at five locations, with a hip pack( Reference Rothney, Neumann and Beziat 65 ). A further development is high-frequency movement sampling, which can differentiate activities not currently distinguishable by accelerometers in general use.
Conclusions
Questionnaires, although inexpensive and practical, are limited in providing reasonably accurate assessments of PAEE. Objective methods, despite their limitations and cost, offer more promise than subjective methods. Where the aim is to categorise participants into activity groups, questionnaires can be cheaper options for teenagers; however, questionnaires cannot be used for pre-school children and are not recommended for primary school children. Despite the expense combined accelerometry and heart rate data appears the most promising method to estimate PAEE in dietary surveys. As technology progresses fast and costs are continually decreasing, we envisage that such methods will be within the reach of dietary studies in the near future.
The MRC Dietary and Physical Activity Assessment toolkit( 66 ) provides further information about these various approaches, including a matrix to aid selection of the appropriate tool(s) given the question(s) to be answered, the sample size, participants’ age group and other theoretical and practical aspects.
Acknowledgements
The authors are the members of the UCL Physical Activity Research Group (PARG).
Financial support
The Irish Section of the Nutrition Society paid J. M.'s expenses to talk on this topic at their June 2013 Dublin Conference. This research received no other specific grants from any funding agency in the public, commercial or not-for-profit sectors. E. S. is funded by the National Institute for Health Research through a Career Development Fellowship. N. C. is also funded by the National Institute for Health Research through the same source. The views expressed in this article are those of the authors and not the English Department of Health or the National Institute for Health Research. UCL-PARG (led by E. S.) has received conference sponsorship from a manufacturer of accelerometers (Actigraph, Pensacola, Florida, USA).
Conflicts of interest
None.
Authorship
J. M. drafted the initial version and conducted most of the literature review; E. S. provided advice and reviewed three drafts of the manuscript; N. C. revised manuscript drafts and helped with reference searches; all authors contributed to the final text.