The normalized scores $s^{*}_1, s^{*}_2,\ldots, s^{*}_n$ are exchangeable random variables for the fixed
$n$, i.e., n-exchangeable or finite exchangeable. Their distribution depends on
$n$, and their correlation is a function of
$n$. Therefore, if they are a segment of the infinite sequence
$s^{*}_1, s^{*}_2,\dots$, then they are not exchangeable, i.e., not infinite exchangeable and also not stationary. Accordingly, Berman's theorem [Reference Berman2] (Theorem 2.1 in the article) and Theorems 4.5.2. and 5.3.4. from [Reference Leadbetter, Lindgren and Rootzén5], which hold for stationary sequences, cannot be used in the proofs of Results 2.1. and 2.2. However, from Theorem 1 presented and proved below, for
$P(X_{ij}=1/2)=p\in [1/3, 1)$ or
$p=0$, Results 2.1. and 2.2. follows, and therefore, so do the follow-up Corollaries 2.1. and 2.2.
Let ${I_{j}^{(n)}=I(s_{j}^{*}\gt x_n(t))}$, where we choose
$x_n(t)=a_n t+b_n$, in which
$a_n$ and
$b_n$ are as defined in equation (1) in the article:

Set ${S_n= I_{1}^{(n)}+ I_{2}^{(n)}+\cdots + I_{n}^{(n)}}$.
We prove the following result.
Theorem 1. For $p=0$ or
${p \in [{1}/{3}, 1)}$ and a fixed value of
$k$,
$\lim _{n\rightarrow \infty }P(S_n=k)=e^{-\lambda (t)}({\lambda (t)^k}/{k!})$,
$\lambda (t)= e^{-t}$.
For $p=0$ or
${p \in [{1}/{3}, 1)}$, Results 2.1. and 2.2. follow from Theorem 1, since
${P(s^{*}_{(n-j)}\leq x_n)=P(S_n\leq j)}$, and therefore

Remark 1. It remains an open problem if Theorem 1 holds also for ${p \in (0, {1}/{3})}$.
Proof. (Theorem 1) The result follows from Assertions presented below. Set

Assertion 1.

where ${d_{{\rm TV}}({L}(W_n), {\rm Poi}(\lambda _n))}$ is the total variation distance between distributions of
$W_n$ and Poisson distribution with mean
$\lambda _n$.
Assertion 2.

where ${c_n\sim k_n}$ means
${\lim _{n\rightarrow \infty } c_n/k_n=1}$.
Assertion 3.

Assertion 4.

In our case, since $s_1^{*},\ldots,s_{n}^{*}$ are identically distributed,
${\sum _{i=1}^{n}(\pi _{i}^{(n)})^2=n}$
${P(s^{*}_{1}\gt x_n)P(s^{*}_{1}\gt x_n),}$ and
$\sum _{i\neq j}{\rm Cov}(I_{i}^{(n)}, I_{j}^{(n)})=n(n-1)[P(s_{1}^{*}\gt x_n(t),$
$s_{2}^{*}\gt x_n(t))-P(s_{1}^{*}\gt x_n(t))P(s_{2}^{*}\gt x_n(t))]$. Hence, from (A2) and (A3) it follows that
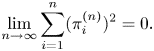
and from (A3) and (A4) it follows that
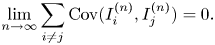
Then, from (F1) and (F2) it follows that ${\lim _{n\rightarrow \infty } d_{{\rm TV}}({L}(W_n), {\rm Poi}(\lambda _n))=0}$, and this completes the proof of Theorem 1.
Proof. (Assertion 1). If $p=P(X_{ij})=0$ then
$X_{ij}$ has Bernoulli distribution which is log-concave; or if
$p\geq 1/3$ then
$2X_{ij}$ has log-concave distribution (i.e., for integers
$u\geq 1$,
$(p(u))^2\geq p(u-1)p(u+1)$). Proposition 1 and Corollary 2 [Reference Ross6] hold in our model with
$p=0$ or
$p\in [1/3, 1)$, and therefore
${\sum _{i=1, i\neq j}^{n}I_{i}^{(n)}|I_{j}^{(n)}=1}$ is stochastically smaller than
${\sum _{i=1, i\neq j}^{n}I_{i}^{(n)}}$. Therefore, from the Corollary 2.C.2 [Reference Barbour, Holst and Janson1] we obtain (A1).
Proof. (Assertion 2). Follows from [Reference Feller4, pp. 552–553, Thms. 2 or 3].
Proof. (Assertion 3). Follows from Assertion 2 combined with [Reference Cramér3] result on p. 374 of his book.
Proof. (Assertion 4). Recall that ${s_1=X_{12}+X_{13}+\cdots +X_{1n}}$ and
${ s_2=X_{21}+X_{23}+\cdots +X_{2n}}$. Hence, condition on the event
${X_{12}=k, k\in \{0, 1/2, 1\}}$,
$s_1$ and
$s_2$ are independent. Let
${s_{1{'}}=X_{13}+\cdots +X_{1n}, s_{2{'}}=X_{23}+\cdots +X_{2n}}$ and denote by
${s_{1{'}}^{*}, s_{2{'}}^{*}}$ the corresponding normalized scores (zero expectation and unit variance). We have,
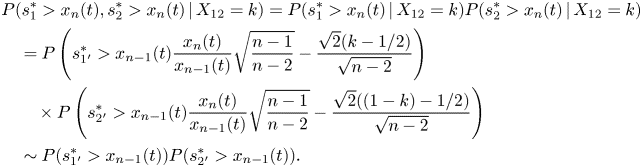
Combining (F3) with the formula of total probability we obtain

and combining it with Assertion 3 we obtain (A4).
Acknowledgement
I am grateful to Pavel Chigansky for pointing out my mistake.