INTRODUCTION
Electronic health records (EHRs) offer a treasure trove of patient information, including diagnoses, symptoms, treatments, and outcomes. Some data serve as biomarkers of prognosis or response to treatments (Feliu et al., Reference Feliu, Jimenez-Gordo and Madero2011; Gagnon et al., Reference Gagnon, Agulnik and Gioulbasanis2013). It is possible that nonbiological EHR data could serve as infomarkers for such other contexts as care practices, but the idea of infomarkers has not been reported on previously. The purpose of our study was to identify infomarkers that appear within nursing care plan data for hospitalized patients prior to death that indicate care goals consistent with palliative care. Identification of such infomarkers is important as a first step to understanding how the context of care practices affect patient outcomes.
We define infomarkers as nonbiological data cues of the human state that are extracted from EHRs using a variety of techniques, such as statistical or data-mining techniques. Infomarkers can be employed to project the possibility of a patient having or transitioning into a state associated with that infomarker. Although there are many ways the infomarker term can be operationalized, in our context the term is focused on EHR data patterns that serve as a cue for identifying a transition in goals of care from nonpalliative (disease- or illness-oriented or health-restorative care) to palliative care (as mainly comfort-oriented care). For example, infomarkers indicative of a shift or need for a shift to palliative care goals among hospitalized patients could be used clinically to generate an EHR alert to initiate appropriate clinical services and inform appropriate use of diagnostic procedures and therapies (Smith et al., Reference Smith, Coyne and Cassel2003). Such efforts could reduce the $2.8 trillion in healthcare costs (Center for Medicare and Medicaid Services, 2014), of which end-of-life (EoL) care now takes a disproportionate share of Medicare expenditures (Zhang et al., Reference Zhang, Wright, Huskamp and Nilsson2009) and of veterans care (Yu, Reference Yu2003). Another benefit of infomarkers is that data miners could use them to identify palliative care patients from big-data repositories for comparative effectiveness and health-services research. A novel idea is the possibility that infomarkers within EHRs could provide a way to extract data for research that could determine additional benefits. Much like biomarkers demonstrate value for personalizing care, EHR data might contain infomarkers that could guide care and be important for health-services and big-data science researchers.
An important but often overlooked component of EHRs is the nursing-care data. When nurses document care-plan data in an EHR every shift in standardized and interoperable format, the data can be analyzed to find any existing infomarkers. Using a dataset with such data, the HANDS (Hands-On Automatic Nursing Data System) database, our specific aim was to identify changes in care plans that could be considered infomarkers of the transition from nonpalliative care goals to those consistent with palliative care. We hypothesized that patients with infomarkers would have better pain outcomes, reflective of the palliative care focus.
METHODS
Design
Our study was a known-group descriptive and comparative analysis of patients who died during a hospital admission as identified from an existing database with deidentified data. Our university's institutional review board determined that the study did not involve human subjects.
The data were derived from a longitudinal study of nine diverse medical–surgical units from four hospitals in the Midwest over a two-year period. A continuous patient stay in a single hospital unit defined a care episode, consisting of the care plans that nurses documented and input into the HANDS EHR system on every shift. Nursing diagnoses were coded with North American Nursing Diagnosis Association–International labels (NANDA–Is) (NANDA, 2003). As part of their clinical routines, nurses updated diagnoses and interventions throughout the course of hospitalization as needed and rated outcomes each shift. At the end of a care episode, nurses recorded the patient discharge disposition. Prior analysis of the dataset demonstrated that after 6–8 hours of training for each of the 787 nurses, there was a high level of compliance (78 to 92%), with updating of the care plan each and every shift and moderately strong validity and reliability in their use of the NANDA–I labels (Keenan et al., Reference Keenan, Yakel and Yao2012).
Sample
Nurses in nine medical surgical units entered a total of 42,403 episodes linked to 34,926 unique patients. In the current study, we examined patient care during the episodes ending in death; therefore, we focused on a subset of 901 patients with a discharge disposition of “expired.” These patients' ages ranged from 20 to 105 years (mean = 74.5 ± 14.6). Gender and race were not required fields.
Procedures
The procedures are described in detail elsewhere, where the investigators showed the reliability and validity of nurses' real-practice-world use of the terms in the care plans (Keenan et al., Reference Keenan, Yakel and Yao2012). Briefly, all nurses received 6–8 hours of extensive training, and then, as part of their routine nursing practice, they recorded into the HANDS EHR system a series of care plans that reflected the care they gave to patients each shift and the evolution of the patients' conditions from unit admission until death. Each care plan documented the patient care during a shift, including all NANDA–I labels (NANDA–Is), and each plan built on the previous one by the shift nurse adjusting (adding or removing) NANDA–Is as needed. Therefore, the care plans were dynamic, representing nursing care focus from shift to shift over the 12 or 24 months that the 9 units from the 4 hospitals participated in the original study.
Analysis
For the 901 patients who died, we examined the 9,196 care plans that were submitted each shift to the HANDS database by 567 of the 787 nurses. On average, there were 3.7 ± 2.2 NANDA–Is on these care plans. We first identified all NANDA–Is that were either added or removed from care plans during the hospitalization episode and examined their characteristics. We next categorized the NANDA–Is as either consistent with palliative care goals or not (nonpalliative care) based on their official NANDA–I definitions. We used the R statistical program for this analysis. Using ANOVA and Tukey's post-hoc tests, we compared groups of patients categorized by patterns of infomarkers for differences by age, length of stay, care-plan elements, and pain outcomes. We set the statistical significance level at p < 0.05.
RESULTS
Identification of Infomarkers
NANDA–I Changes
We identified 515 shifts post-admission where nurses added NANDA–Is. Among these, 102 shifts contained both NANDA–I additions and one or multiple NANDA–I removals during the same shift. We examined these 102 shifts first since they likely represented more radical changes in care than shifts with only NANDA–I additions. Of these, 85 (83%) had either Death Anxiety or Anticipatory Grieving added to the care plan. In addition, we found that the following NANDA–Is were added to six other care plans at the same time as nonpalliative care NANDA–Is were removed: Readiness for Enhanced Family Coping (n = 2), Ineffective Coping (n = 2), or Acute Pain (n = 2) (see Table 1). In summary, 91 of 102 shifts indicated a transition to care goals consistent with palliative care. The remaining 11 reflected adjustments in care not related to a palliative transition.
Table 1. Identified Infomarkers Indicating nursing care consistent with palliative care goals
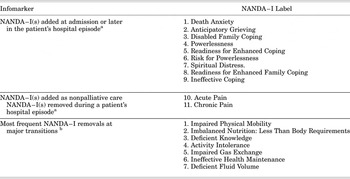
a Continuous patient stay in a single hospital unit defined a care episode.
b Seven most frequent.
We next examined the other shifts where only NANDA–I additions occurred. We identified (in addition to Death Anxiety, Anticipatory Grieving, Ineffective Coping, and Readiness for Enhanced Family Coping) five other NANDA–Is that could indicate care consistent with palliative care goals: Disabled Family Coping, Powerlessness, Readiness for Enhanced Coping, Risk for Powerlessness, and Spiritual Distress (Table 1). We did not consider the addition of either Acute Pain or Chronic Pain in the absence of simultaneous removal of nonpalliative NANDA–Is as an indicator of care consistent with palliative care goals, since the mere addition of Acute or Chronic Pain to the care plan does not necessarily indicate a transition to palliative care goals, even near death. Among the 413 shifts that had only NANDA–I additions, we found 116 where one or more of the previously noted nine infomarkers were added.
Major vs. Minor Transitions During a Care Episode
It appears that the 91 shifts with both NANDA–I additions and removals represent potentially major transitions in care, shifting from nonpalliative to palliative care, while the 116 shifts with only additions represent minor transitions, attempting to incorporate care consistent with palliative care goals into the existing care regime. We examined the distribution of these transition points among patients' care episodes (Figure 1). No episode contained more than one major transition point. Similarly, a vast majority of the episodes with minor transitions contained only one transition point, but we found six episodes in which a minor transition cooccurred either with a major transition or another minor transition. In four episodes there were both a major and a minor transition. In one episode, a single infomarker addition was followed by the addition of two infomarkers on the care plan on the next shift.

Fig. 1. Outline of the care plan (NANDA–I only) for a couple of representative care episodes. The first sample episode contained a major transition, where a marker (labeled in purple) was added and multiple NANDA–Is (labeled in green) were removed. The transition block is labeled in purple. The second sample episode contained a minor transition, where a marker was added but no NANDA–I removed.
In total, we identified 200 patients receiving care consistent with transitions to palliative care goals before they died. Of these, 91 patients had a major transition (4 of whom also had minor transitions), whereas the other 109 only had minor transition(s). The remaining 701 patients did not have a transition point consistent with palliative care goals, but 194 of those patients had one or more of the infomarkers on the care plan at admission, an indication that a patient was recognized as needing care consistent with palliative care goals upon admission. The remaining 507 episodes did not have any of the infomarkers, which constituted 56% of the patients who died. In summary, we found four categories of patients based on infomarker analysis: those with (1) no infomarkers on any of the care plans, (2) infomarkers added on the admission care plan, (3) infomarkers added on a post-admission care plan (minor transitions), and (4) infomarkers added and nonpalliative care NANDA–Is removed on a post-admission care plan (major transition) (Table 2).
Table 2. Care plan comparison of 901 patients classified using infomarkers for those who died during hospitalization

a Continuous patient stay in a single hospital unit defined a care episode.
b Excludes pain NANDA–Is.
c Pain ratings could range from 1 (worst possible rating) to 5 (best possible rating, reflecting what a healthy person of the same age, sex, and cognitive ability would score).
Comparison of Patient Characteristics by the Four Categories
We next compared patients in the four categories (Table 2) and found a significant difference in patients' average age (p < 0.001). Post-hoc tests showed that patients with infomarkers either at admission or with a major transition were on average significantly older than patients without any infomarker on their care plans (p < 0.001). Patients whose care plans only had minor transitions were also younger than patients with infomarkers at admission (p = 0.03) and showed a trend of being younger than patients with a major transition (p = 0.08).
The length of stay for patients in these four categories was significantly different from each other (p < 0.001). Post-hoc tests revealed that patients with infomarkers of major or minor transitions had significantly longer stays than both the patients with infomarkers on admission and those with no infomarker at all (p < 0.001). Those patients with infomarkers on admission had shorter stays than those without an infomarker (p = 0.02). Examination of the time at which the transitions occurred for patients whose care plan had a major or minor transition showed that there was no significant difference in the timing of major and minor transition points (p = 0.54). Further, on average, the transition occurred on day 5 of hospitalization, whereas patients with no infomarkers on average died by day 4.
Comparison of the NANDA–Is for these patients also revealed interesting information. Although there was very little difference in the presence of Acute or Chronic Pain NANDA–Is at admission among these four categories (p = 0.93), there was a clear difference in the number of NANDA–Is focused on nonpalliative care and not related to pain or care consistent with palliative care goals at admission (p < 0.001). The patients whose plans of care contained infomarkers at admission had by far the fewest (p < 0.001) nonpalliative care–oriented NANDA–Is, while the difference between the other three categories was not significant.
Examination of NANDA–Is added after admission showed that patients with major or minor transitions had pain NANDA–Is added much more frequently than patients with an infomarker at admission (p < 0.001) or with no infomarkers (p < 0.005). Patients whose care plan had infomarkers from the start had many fewer additions of nonpalliative care–oriented NANDA–Is after admission than the other patients (p < 0.001), but the difference between the other three categories was not statistically significant.
We found that patients whose care plan had a major transition had more NANDA–I removals than patients in the other three categories (p < 0.001). Patients with minor transitions had significantly more NANDA–I removals than the remaining two categories (p < 0.001). The average number of removals per patient (0.48) for patients with minor transitions, however, was more similar to those of patients with infomarkers upon admission (0.05) and patients without an infomarker (0.11) than to patients with a major transition (3.14). Finally, at the time of a patient death, the number of active non-pain or palliative-related NANDA–Is on the care plan for patients with a major transition was much lower than patients with no infomarkers or with only minor transitions (p < 0.001) and not significantly different from patients with an infomarker upon admission (p = 0.42).
The last two rows of Table 2 show the average pain ratings for patients in the four categories. There was no significant difference in initial ratings (p = 0.36), but there was a substantial difference in pain ratings at the time of death (p = 0.006). Post-hoc testing showed that patients with a major transition from nonpalliative care to care consistent with palliative care goals had a significantly better pain outcome than patients with no infomarkers (p = 0.009). Comparing the initial and final pain ratings in each category, we can see clear improvement for patients with a major transition to care consistent with palliative care goals, but no improvement for patients without an infomarker.
Palliative Transitions
We examined the four categories of patients and the timing of changes in care plans (Table 3). For the majority of pain NANDA–Is, post-admission additions occurred at the transition points. For the NANDA–Is not related to pain or infomarkers, on the other hand, almost all were placed on the care plans preceding transition points, not afterward. Table 3 also shows that there were very few NANDA–I removals outside the transition points.
Table 3. Timing of NANDA–I changes: Averages for patients with major or minor transitions

DISCUSSION
We are the first to identify EHR infomarkers that suggest care consistent with palliative care goals for hospitalized EoL patients. In a known sample of 901 patients who had died, 43.7% had infomarkers on their care plans that were consistent with palliative care goals. Specifically, nine NANDA–I labels that were added to care plans and two NANDA–Is added along with simultaneous removal of other NANDA–Is represent either a major or minor transition point shifting to care consistent with palliative care goals before death. Infomarkers indicative of care consistent with a palliative care focus also were present on the admission care plan for some patients. Comparison of NANDA–Is at admission as well as the type and timing of NANDA–I post-admission changes between four categories of patients provides compelling evidence that these infomarkers indeed indicate care consistent with palliative care goals. Older patients were significantly more likely to have an infomarker at admission or a major transition than younger ones. Younger patients were more likely to have minor transitions than infomarkers at admission. Patients with longer lengths of stay were significantly more likely to have major or minor transitions than those with no transitions or infomarkers at admission. Finally, pain outcomes were significantly better for patients with major transitions than those without infomarkers indicative of care consistent with a palliative care focus.
Patient care at the end of life is often challenging due to the uncertainties related to when nonpalliative disease- or illness-oriented– or health-restorative–focused care should transition to palliative comfort–focused care (Goodman et al., Reference Goodman, Esty and Fisher2011; Mack et al., Reference Mack, Weeks and Wright2010). Consequently, many efforts are expended on futile and costly care at the end of life (Goodman et al., Reference Goodman, Esty and Fisher2011; Mack et al., Reference Mack, Weeks and Wright2010), with interventions and treatments that fall short of addressing pain and suffering for the patient and family prior to death (Cruz et al., Reference Cruz, Camalionte and Caruso2014). In many instances, patients suffer through an undignified death as a result of the futile life-prolonging intensive care (Goodman et al., Reference Goodman, Esty and Fisher2011; Mack et al., Reference Mack, Weeks and Wright2010). Therefore, our finding that pain outcomes were significantly better for patients with major transitions than those without infomarkers supports the validity of our findings. The presence of a major transition as indicated by the addition of infomarkers and the removal of nonpalliative NANDA–Is signifies that the care team is moving away from disease- or illness-oriented or health-restorative–focused care to an emphasis on comfort care for the dying patient.
Perhaps most exciting about our discovery of the infomarkers in the HANDS EHRs and their use to demonstrate transitions in EoL care from an acute to a palliative focus is the potential importance for big-data science using nursing-care data. We have only scratched the surface in the analysis of shift-to-shift changes in care plans, but the identified infomarkers support the relevance of this type of analysis of practice-based data. If confirmed with further research, infomarkers provide a potentially cost-efficient way of identifying patients for palliative care studies using EHR data. Our findings may precipitate further research using big data from EHRs to drive enhanced care practices for the dying and help better direct government spending for EoL care.
Even though our finding about infomarkers is novel and important, there are some limitations. First, it is not clear what the medical care plan was for the 901 patients we studied. Because of the nature of our database, we are not able to determine if the addition, removal, or retention of a patient's nursing diagnosis on the care plans was made independent of or reactive to changes in the medical care goals. It is also not clear from the available data who was first to identify that the patient was at the end of the life—the nurses or another health professional.
In summary, we identified infomarkers which indicate that care consistent with palliative care goals is the dominant goal of nursing care. Using these infomarkers, four categories emerged, patients: (1) with palliative care implemented from admission, (2) with a major transition shifting from nonpalliative care to care consistent with palliative care goals, (3) with minor transitions incorporating care consistent with palliative care goals along with nonpalliative care, and (4) without such palliative care indicators. Even in a small sample, there is evidence suggesting that focusing on care consistent with palliative care goals and removing unnecessary nonpalliative care near the patient's death might lead to better patient outcomes. Electronic health records contain pertinent infomarkers that, if confirmed in future studies, could be employed for timely referral to palliative care for improved focus on comfort for end-of-life patients. These infomarkers could also be used to identify palliative care subjects from data repositories for big-data, comparative-effectiveness, and health-services research.
ACKNOWLEDGMENTS
This research was made possible by Grant Number 1R01 NR012949 from the National Institutes of Health, National Institute for Nursing Research. Its contents are solely the responsibility of the authors and do not necessarily represent the official views of the National Institute for Nursing Research. The final peer-reviewed manuscript is subject to the National Institutes of Health Public Access Policy. The authors thank Veronica Angulo for clerical assistance.
AUTHOR DISCLOSURE STATEMENT
The HANDS software that was employed in this study is now owned and distributed by HealthTeam IQ LLC. Dr. Gail Keenan is currently the President and CEO of this company, and she has a current conflict of interest statement of explanation and management plan in place with the University of Illinois at Chicago.