Introduction
Human–wildlife interactions vary in frequency, intensity, and on a continuum from positive to negative (Soulsbury & White, Reference Soulsbury and White2015). Negative interactions can pose significant threats to human welfare and livelihoods, and to ecosystem structure and function (Estes et al., Reference Estes, Terborgh, Brashares, Power, Berger and Bond2012; Kansky et al., Reference Kansky, Kidd and Knight2014). They are often complex, encompassing an array of species and situations, each with unique factors and thus potential solutions (Dickman, Reference Dickman2010). Conflicts resulting from negative human–wildlife interactions are likely to be exacerbated in the future with further growth of the human population and ongoing destruction of habitats for wildlife.
Large carnivores can cause substantial direct and indirect costs to humans and are particularly prevalent in adverse human–wildlife interactions because they often predate livestock and have large home ranges that are likely to overlap with human-dominated areas (Macdonald & Sillero-Zubiri, Reference Macdonald, Sillero-Zubiri, Loveridge, Lynam and Macdonald2002; Inskip & Zimmermann, Reference Inskip and Zimmermann2009). Conserving large carnivores is vital because they are keystone species that act as umbrella and flagship species for wider biodiversity protection (Loveridge et al., Reference Loveridge, Packer, Dutton, Dickson, Hutton and Adams2009; Macdonald & Loveridge, Reference Macdonald and Loveridge2010), and many are deeply intertwined with human cultures (Kellert et al., Reference Kellert, Black, Rush and Bath1996). However, most (77%) of the largest carnivores now have decreasing populations (Ripple et al., Reference Ripple, Estes, Beschta, Wilmers, Ritchie and Hebblewhite2014). For example, although negative interactions between humans and African lions Panthera leo have happened for millennia (Loveridge et al., Reference Loveridge, Searle, Murindagomo and Macdonald2007), the lion population (43%) and range (92%) have declined dramatically in recent decades (Bauer et al., Reference Bauer, Packer, Funston, Henschel and Nowell2015). Habitat destruction and land-use changes, resulting from the cessation of traditional sustainable land-use practices following European colonization of African countries, are primarily responsible for these declines (Clover & Eriksen, Reference Clover and Eriksen2009; Bauer et al., Reference Bauer, Packer, Funston, Henschel and Nowell2015). As a consequence of their now restricted range and diminished population, the future survival of the African lion may depend upon coexistence with farmers in human-dominated landscapes, because the effectiveness of protected areas can be compromised when lions are drawn out of these areas to replace those killed on the borders (Loveridge et al., Reference Loveridge, Searle, Murindagomo and Macdonald2007; Macdonald & Loveridge, Reference Macdonald and Loveridge2010).
Negative human–carnivore interactions will probably escalate in the future as global livestock production is projected to rise from 258 Mt in 2005–2007 to 455 Mt in 2050, with much of this growth occurring in developing countries (Alexandratos & Bruinsma, Reference Alexandratos and Bruinsma2012). This is a complex issue (Dickman, Reference Dickman2010; Madden & McQuinn, Reference Madden and McQuinn2014), with both tangible and intangible costs to human communities and carnivores (Redpath et al., Reference Redpath, Gutiérrez, Wood and Young2015a; Dickman & Hazzah, Reference Dickman, Hazzah and Angelici2016; Kansky et al., Reference Kansky, Kidd and Knight2016). In particular, the economic costs of predation for livestock farmers are significant (Butler, Reference Butler2000; Patterson et al., Reference Patterson, Kasiki, Selempo and Kays2004) and can lead to substantial losses of annual household income (Wang & Macdonald, Reference Wang and Macdonald2006). Consequently, retaliatory killings are common and lead to conflicts between conservationists and livestock farmers (Redpath et al., Reference Redpath, Bhatia and Young2015b).
Examining the factors that affect livestock predation can help us understand patterns and subsequently guide measures to reduce attacks, foster relationships between conservationists and farmers, and work towards coexistence of farmers and predators (Carvalho et al., Reference Carvalho, Zarco-González, Monroy-Vilchis and Morato2015). Livestock predation risk is influenced by multiple variables, including livestock abundance, farming techniques, mitigation measures, presence of trophy hunting, changes in laws and their implementation, proximity to human settlements and protected areas (Yu et al., Reference Yu, Endi, Zhihong and Xiaojie2006; Van Bommel et al., Reference Van Bommel, Bij De Vaate, De Boer and De Iongh2007), natural prey density and environmental conditions (Sunquist & Sunquist, Reference Sunquist, Sunquist and Gittleman1989; Polisar et al., Reference Polisar, Maxit, Scognamillo, Farrell, Sunquist and Eisenberg2003; Azevedo & Murray, Reference Azevedo and Murray2007; Tortato et al., Reference Tortato, Layme, Crawshaw and Izzo2015). Environmental conditions such as temperature, light level and rainfall are relatively easy to monitor and have overarching impacts on livestock predation by carnivores. It is plausible that variations in such factors could trigger changes in predation patterns, and could thus be used as predictors of the likelihood of attacks. We chose livestock predation by lions as a model interaction to test this idea.
Environmental conditions, particularly temperature and rainfall, have significant effects on lion biology and demography (Celesia et al., Reference Celesia, Peterson, Julian and Gnoske2010). Rainfall has been associated with both increases (Patterson et al., Reference Patterson, Kasiki, Selempo and Kays2004; Kuiper et al., Reference Kuiper, Loveridge, Parker, Johnson, Hunt and Stapelkamp2015) and decreases in lion attacks on livestock (Butler, Reference Butler2000; Schiess-Meier et al., Reference Schiess-Meier, Ramsauer, Gabanapelo and König2007), which are typically related to prey abundance. Compared to non-protected landscapes, protected areas usually have higher prey levels that remain relatively constant over time in non-migratory systems (Macdonald & Loveridge, Reference Macdonald and Loveridge2010). This suggests rainfall is less likely to influence rates of livestock predation in regions surrounded by protected areas. Although temperature is thought to have no influence on lion predation of livestock (Patterson et al., Reference Patterson, Kasiki, Selempo and Kays2004), lions are more active during colder periods and more nocturnal in areas with higher mean annual temperatures (Hayward & Slotow, Reference Hayward and Slotow2009); temperature also affects food intake by lions (West & Packer, Reference West and Packer2002). Because hunting for prey increases body temperature in lions, and their biology leaves them vulnerable to overheating, we expected livestock predation levels to reflect their preference for lower external temperatures. Lions prefer periods without moonlight for hunting wild prey (Schaller, Reference Schaller1972), and travel closer to livestock at lower moonlight levels (Oriol-Cotterill et al., Reference Oriol-Cotterill, Macdonald, Valeix, Ekwanga and Frank2015), probably because of the reduced risk of being seen by people and prey.
We investigated how temperature, moon phase and rainfall affected the incidence and severity of lion predation of livestock over a 7-year period in an area with frequent negative human–lion interactions. We hypothesized that (1) livestock predation would increase with decreasing temperature, (2) livestock predation would increase with decreasing moonlight levels, and (3) rainfall would have no significant effect on livestock predation, given that the study area is surrounded by protected areas.
Study area
The study area (Fig. 1) covers c. 4,500 km2 around Pandamatenga village in north-east Botswana, in the Chobe district near the border with Zimbabwe and close to Hwange National Park. Pandamatenga's human population increased from 1,369 in 2007 (African Development Bank, 2008) to c. 1,943 in 2011 (Central Statistics Office of Botswana, 2015). It is one of Botswana's least arid areas, with a mean annual rainfall of 600 mm, almost all of which occurs during October–April, with a peak during December–February (African Development Bank, 2008).

Fig. 1 The study site around Pandamatenga village in northeast Botswana, showing the area from which the analysed incident reports on livestock predation by lions Panthera leo originated.
Pandamatenga is situated between several protected areas that support c. 5 lion prides comprising at least 52 individuals. Farms are small subsistence operations and all livestock are kept in protective enclosures (kraals) at night and released for grazing during the day; the robustness of the kraals, presence of any other mitigation methods and grazing regimes differ between farmers.
Methods
Incident reports
Botswana provides a state-funded compensation scheme in which farmers file a report when they lose livestock to lions (Hemson et al., Reference Hemson, Maclennan, Mills, Johnson and Macdonald2009). The Department of Wildlife and National Parks provided us with reports on incidents that took place during January 2010–April 2016. We believe these reports provide comprehensive coverage of lion–livestock incidents during this period because farmers must file a report to claim compensation for attacks, and farmers in Pandamatenga lack the financial capacity to leave these unreported. The data included reports on 395 incidents, each with information on date, location, source, livestock owner, the species and number of livestock involved, and whether the incident took place inside or outside a kraal.
Data preparation
Three response variables were explored: incident occurrence (a measure of attack likelihood), the number of livestock involved in incidents (a measure of attack severity), and the total cost of incidents (an alternative measure of attack severity, reflecting farmers’ likely perception of the events). All three response variables were expressed on a daily, monthly, and annual scale. We used a daily scale to investigate the effect of temperature and moon phase on response variables. Daily data covered each day during 1 January 2010–30 April 2016, and included incidents, non-incidents, and the associated response and environmental variables; we removed values of zero for the analysis of livestock attacked per incident and associated cost. We used a monthly scale to investigate the immediate and delayed effect of temperature and rainfall on monthly totals of response variables during January 2010–April 2016. We used an annual scale to examine large-scale patterns in response variables during 2010–2015; data from 2016 were removed from the annual analysis because they were only available until April that year.
Monetary cost in Botswana Pula (BWP) was assigned to each incident based on current compensation scheme values; as of 2013 these were set in BWP as 450 for a goat, 1,000 for a calf, 1,100 for a pig, 3,000 for a heifer, cow, bullock, or oxen, and 5,500 for a bull. Costs were assigned to the incidents irrespective of whether an animal was killed or injured, because information on the severity of the injuries or any livestock fatalities was not included in the reports. Five reported incidents concerned lions being translocated from farmers’ lands; these were removed because they did not involve attacks on livestock. Incidents involving dogs (n = 3) and chickens (n = 1) were included as incidents, but not in the number of livestock attacked or the cost of incidents as neither are covered under the compensation scheme: dogs are not livestock, and the single incident involving 23 chickens could have exerted undue leverage on the results.
We accessed monthly rainfall data (mm) from the meteorological office in Pandamatenga for July 2013–March 2016. Daily moon phase data during 1 January 2010–30 April 2016 were downloaded from USNO (2016). According to results from Packer et al. (Reference Packer, Swanson, Ikanda and Kushnir2011), three moon phases were defined: the full moon and subsequent 9 days (phase 1), the 10 days prior to the full moon (phase 2), and the intermediate days when the moon is least visible (phase 3). Moonlight levels are lowest during phase 3 and highest during phases 1 and 2; however, during phase 1 the moon does not rise until after sunset and thus leaves a period of darkness, whereas during phase 2 the moon is above the horizon at sunset and there is no such interlude. We obtained data on temperature for Pandamatenga during 1 January 2010–30 April 2016 from NOAA (2016). This included daily values for minimum and maximum temperature, and monthly values for extreme daily maximum and minimum, monthly mean maximum and minimum, and monthly mean temperatures. We used linear interpolation to fill in 416 minimum and 300 maximum temperature values that were missing from the daily data (of a total of 1,990 values), with average data gaps being 1.8 and 1.3 days, respectively; linear interpolation was deemed to be more accurate than polynomial, based on plots of the data. Monthly temperature and rainfall data were shifted to match the response variables 1 and 2 months ahead, to test for any delayed response; extreme daily temperatures were not used in these models because it is unlikely that an extreme temperature on a single day would affect the incidents in the following months.
Data analysis
We used R 3.X for all data analysis (R Development Core Team, 2015). To test our hypotheses and investigate the effect of environmental variables on response variables, we used generalized linear models and assumed the following error distributions: binomial for the occurrence of an incident, Poisson for the number of incidents, livestock attacked per month and livestock attacked per incident, and Gaussian for cost (log) per incident and month. We used generalized linear models for daily data to assess the effect of temperature and moon phase on response variables, on monthly data to assess the effect of temperature and rainfall, and on shifted monthly data to assess delayed effects of temperature and rainfall. To control for interdependence between variables, we excluded variables correlated by r > 0.85. When our models assuming Poisson error distributions showed a residual deviance higher than residual degrees of freedom, we compensated for this overdispersion by assuming quasi-Poisson errors. We followed a step-by-step procedure to find the model with the best fit based on Akaike's information criterion (AIC) and the residual deviance relative to degrees of freedom.
Because we split moon phase into three categories, we used a general linear hypothesis test to assess differences amongst them. We calculated the predicted daily probability of an attack on the back-transformed response scale, for a sequence of temperatures at each moon phase, and plotted responses with 95% confidence intervals; we extended the minimum temperature range to −5–30 °C (from −1.11–21.56 °C), and maximum temperature range to 15–50 °C (from 20.56–42.22 °C) to investigate how potential future changes in extreme temperatures might affect livestock predation.
We used a Kruskal–Wallis H test to assess differences in the number of livestock involved and cost of incidents between the years based on a per incident basis and on monthly summaries. Seasons were defined as dry (May–October) and wet (November–April). We compared monthly sums of attacks inside and outside kraals and between seasons for all response variables using a Welch two-sample t test and visualized them with box plots.
Results
Over the 7 years of the study there were 395 lion attacks involving 589 livestock at a total cost of BWP 1,429,850 (GBP 106,833; Supplementary Table 1).
Temperature and moon phase
We could not use minimum and maximum temperature in the same generalized linear model because of intercorrelation, and minimum temperature produced the best-fit model for each response variable. Our best-fit generalized linear models (Table 1) showed that the incidence of attacks on livestock and the number of livestock attacked per event both increased with decreasing minimum temperature (Fig. 2). We also found this pattern for maximum temperature, although the effect was weaker (Supplementary Fig. 1). Based on the coefficient for minimum temperature, holding moon phases at a fixed value, there was a 2.52% increase in the odds of an incident with every 1 °C decrease. Incidents were more likely to occur in moon phases 3 (around new moon) and 1 (post-full moon) compared to moon phase 2 (pre-full moon); however, there were no differences between moon phases for either the number of livestock attacked per incident or associated cost. Odds ratios showed that the likelihood of an incident occurring during moon phases 1 and 3 was 1.32 and 1.53 times higher, respectively, than during phase 2 (Supplementary Table 2). No environmental variables were significant predictors of cost. These results support our hypotheses 1 and 2 that livestock predation would increase with decreasing temperature and moonlight.
Table 1 Summary of the best fit generalized linear models examining the effect of moon phase and temperature on incidents of lion Panthera leo predation on livestock and their severity in Pandamatenga. Significant effects are indicated with *.

1 TMin, minimum temperature (°C) on a given day.

Fig. 2 The predicted probability of a lion attack on livestock in Pandamatenga based on the minimum temperature (°C) of a given day under different moon phases: phase 1 is the full moon and subsequent 9 days, phase 2 is the 10 days prior to the full moon, and phase 3 includes the remaining days around the new moon.
Temperature and rainfall
All monthly temperature variables were correlated and we selected only the temperature variable that produced the best fit for each model (Table 2). The monthly number of livestock attacked increased significantly with decreasing extreme minimum temperature (Fig. 3), and marginally with decreasing extreme maximum daily temperature (Supplementary Fig. 2); this result again supports our hypothesis 2 that decreasing temperature would increase livestock predation. Although decreasing monthly mean minimum temperatures increased the number of incidents and associated costs, these effects were not significant. Rainfall was not predictive of any response variable, which supports our hypothesis 3 that rainfall has no effect on livestock predation in locations surrounded by protected areas.
Table 2 Summary of the best-fit generalized linear models examining the effect of monthly rainfall and temperature on lion–livestock incidents and their severity in Pandamatenga. Significant effects are indicated with *.

1 MMeanMin, monthly mean minimum temperature (°C); ExMinTemp, extreme minimum daily temperature (°C).
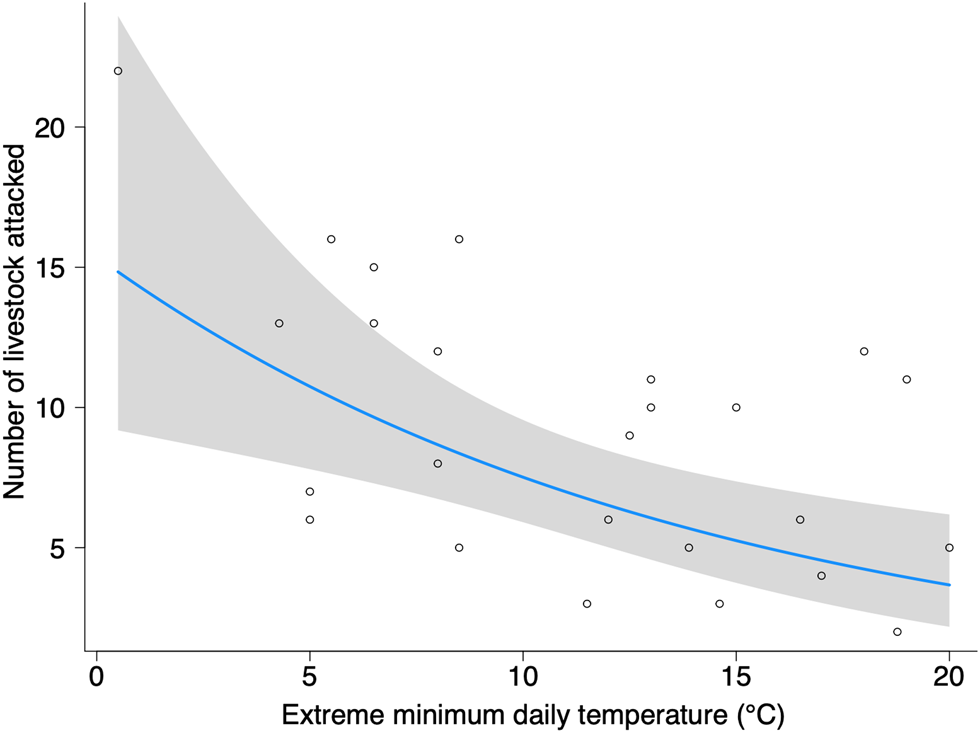
Fig. 3 The effect of extreme minimum daily temperature (°C) on the number of livestock attacked by lions in Pandamatenga per month, showing the fitted generalized linear model and Wald 95% confidence intervals based on standard errors.
Delayed effects of temperature and rainfall
Temperature had no significant 1-month delayed effect on response variables, nor 2-month delayed effect on incidents or cost (Table 3). However, the number of livestock attacked was negatively associated with mean monthly maximum temperature 2 months earlier (Supplementary Fig. 3). Contrary to our hypothesis 3 that rainfall would not influence attacks in the context of nearby protected areas, we found high levels of rainfall decreased the number of livestock attacked and the associated cost in the following month (Fig. 4). Rainfall had no significant 2-month lag effect on response variables.
Table 3 Summary of the best-fit generalized linear models examining the delayed effect of temperature and rainfall on lion–livestock incidents and their severity in Pandamatenga. Significant effects are indicated with *.

1 MeanMaxTemp, mean monthly maximum temperature (°C); MeanMinTemp, mean monthly minimum temperature (°C).

Fig. 4 The effect of the sum of monthly rainfall on (a) the number of predation incidents, (b) the number of livestock attacked, and (c) the log associated cost of attacks in Pandamatenga in the following month, showing the fitted generalized linear model lines and Wald 95% confidence intervals based on standard errors.
Temporal patterns and the effectiveness of kraals
Attacks on livestock tended to be more frequent and severe in the dry season (Supplementary Fig. 4). However, a Kruskal–Wallis H test showed there was no significant difference between months in the number of incidents (χ 2(11) = 11.827, P = 0.377), livestock attacked (χ 2(11) = 12.647, P = 0.317) or cost (χ 2(11) = 7.615, P = 0.747). Similarly, a Kruskal–Wallis H test showed there was no significant difference between years in the number of incidents (χ 2(5) = 8.936, P = 0.112), livestock attacked (χ 2(5) = 10.251, P = 0.068), or cost (χ 2(5) = 10.501, P = 0.062). A Welch two-sample t test showed that significantly more incidents (t = −4.73, df = 119.27, P < 0.00001), involving more livestock (t = −3.149, df = 135.7, P < 0.005), at a higher cost (t = −4.40, df = 126.08, P < 0.0001), occurred outside kraals, based on monthly summaries (Supplementary Fig. 5).
Discussion
Although temperature and moon phase are known to affect lion activity (Hayward & Slotow, Reference Hayward and Slotow2009; Celesia et al., Reference Celesia, Peterson, Julian and Gnoske2010; Packer et al., Reference Packer, Swanson, Ikanda and Kushnir2011; Oriol-Cotterill et al., Reference Oriol-Cotterill, Macdonald, Valeix, Ekwanga and Frank2015), our study is the first to show these variables have a significant effect on the occurrence and severity of lion attacks on livestock.
Effects of temperature
Our hypothesis that livestock predation would increase with decreasing temperature was supported by all models. There have been few previous studies on the effect of temperature on livestock predation by carnivores. Patterson et al. (Reference Patterson, Kasiki, Selempo and Kays2004) found no correlation between temperature and the frequency or severity of lion attacks on livestock. However, their analysis only included temperature data for most days during 1 year whereas we were able to analyse 6 years of data.
Livestock predation is affected by husbandry practices, which vary between individuals and on a spatio-temporal scale (Kgathi et al., Reference Kgathi, Mmopelwa, Mashabe and Mosepele2012). For example, some farmers may leave their cattle out for grazing at night during warmer periods, and others may put less effort into preventing livestock attacks during colder periods to avoid uncomfortable conditions, resulting in more incidents. However, we have no data on individual farmers’ husbandry strategies to assess this.
We can, however, infer from our results that lion physiology and behaviour influence livestock predation. Lions must maintain their core body temperature at 38–39 °C, but have few behavioural mechanisms to facilitate this at high ambient temperatures (Willmer et al., Reference Willmer, Stone and Johnston2006). Given that a successful livestock hunt increases a lion's body temperature (through locomotion, stress and feeding) and a lion's biology leaves it vulnerable to overheating, it is probable that lions favour lower and avoid higher ambient temperatures for livestock predation. Overheating when hunting is a significant danger for lions because they have large amounts of heat-producing muscle tissue and a low surface area to volume ratio, and because locomotion can increase heat production to 10–15 times that of the basal metabolic rate (Pough et al., Reference Pough, Janis and Heiser2012). Overheating is probably a greater problem for males because of their larger body size and the insulating properties of their manes, and males feed significantly less in warmer months, whereas food intake of females remains unchanged at higher temperatures (West & Packer, Reference West and Packer2002). Most lions predating livestock are male, and thus males are probably overrepresented in our data (Macdonald & Loveridge, Reference Macdonald and Loveridge2010). Although hunting livestock may require less physical exertion than hunting wild prey, this could conceivably be outweighed by the fear of human activities in areas with frequent negative human–lion interactions. For example, the body temperature of cheetahs significantly increases after a successful hunt because of the stress induced from remaining vigilant for a dominant predator, rather than the physical exertion of the hunt itself (Hetem et al., Reference Hetem, Mitchell, de Witt, Fick, Meyer, Maloney and Fuller2013). Lions hunting livestock around pastoral lands exhibit a wariness of human activity, altering their behaviour and moving faster than usual (Valeix et al., Reference Valeix, Hemson, Loveridge, Mills and Macdonald2012; Oriol-Cotterill et al., Reference Oriol-Cotterill, Macdonald, Valeix, Ekwanga and Frank2015). It is therefore conceivable that lions will be in an alarmed state after a successful livestock hunt, resulting in higher body temperatures and stress hyperthermia (Meyer et al., Reference Meyer, Fick, Matthee, Mitchell and Fuller2008; Hetem et al., Reference Hetem, Mitchell, de Witt, Fick, Meyer, Maloney and Fuller2013), and thus have a preference for predating livestock during colder periods. Further increasing livestock predation during extremely low temperatures may be a behavioural strategy to avoid critically low body temperatures (Stryker, Reference Stryker2016), as lions require more energy for thermoregulation during these periods, and large meat-based meals increase body temperature significantly (West & Packer, Reference West and Packer2002; Pough et al., Reference Pough, Janis and Heiser2012).
Rather than temperature having a direct effect on predation, it could be that variations in wild prey abundance coincide with seasonal temperature changes, as lion–livestock predation is affected by the relative abundance of wild prey compared to livestock (Hemson, Reference Hemson2003). The mass movement of zebras (Naidoo et al., Reference Naidoo, Chase, Beytell, Du Preez, Landen, Stuart-Hill and Taylor2014), and potentially other ungulates (Harris et al., Reference Harris, Thirgood, Hopcraft, Cromsigt and Berger2009), from Chobe river to Nxai Pan National Park in the wet season may decrease prey availability around Pandamatenga during that time. However, our results show incident number and severity to be generally higher in the dry season, so it is unlikely this migration affects attacks around Pandamatenga. Furthermore, high availability of wild prey has been shown to both increase (Stahl et al., Reference Stahl, Vandel, Herrenschmidt and Migot2001; Treves et al., Reference Treves, Naughton-Treves, Harper, Mladenoff, Rose, Sickley and Wydeven2004) and decrease (Hemson, Reference Hemson2003; Valeix et al., Reference Valeix, Hemson, Loveridge, Mills and Macdonald2012) levels of livestock predation; increases in livestock predation may occur because higher wild prey levels can sustain greater predator populations (Macdonald & Loveridge, Reference Macdonald and Loveridge2010).
Effects of moon phase
Our findings agreed with our hypothesis that livestock predation would increase with decreasing moonlight levels. This is consistent with research indicating that lions travel closer to kraals at lower moonlight levels (Oriol-Cotterill et al., Reference Oriol-Cotterill, Macdonald, Valeix, Ekwanga and Frank2015), and that attack rates of lions on people was 2–4 times lower in the 10 days before the full moon compared to the 10 days after, when the moon rises after sunset, resulting in a period of darkness (Packer et al., Reference Packer, Swanson, Ikanda and Kushnir2011). Lions move more slowly and their movement paths are more tortuous when moonlight levels are high, indicating more caution (Oriol-Cotterill et al., Reference Oriol-Cotterill, Macdonald, Valeix, Ekwanga and Frank2015), and we infer that lions utilize periods of lower moonlight for hunting livestock because it is easier to avoid detection by people and potential prey.
Effects of rainfall
Rainfall had no immediate effect on the number of incidents or their severity, which supports our hypothesis that livestock predation should be unrelated to rainfall in the context of stable wild prey numbers within nearby protected areas. However, attacks were significantly less severe in the month following increased rainfall, which contradicts this hypothesis. Patterson et al. (Reference Patterson, Kasiki, Selempo and Kays2004), in a study carried out in Tsavo National Park, found no delayed relationship between rainfall and predation with lags of 1–6 months but found attacks to be most frequent in the wet season. Pandamatenga's different natural prey densities and landscape could be responsible for this discrepancy between our results and the ones reported from Tsavo National Park. Farmers take their livestock further from kraals when vegetation density and water levels are low (Oriol-Cotterill et al., Reference Oriol-Cotterill, Macdonald, Valeix, Ekwanga and Frank2015), leaving a higher number of livestock more vulnerable to predation. Decreasing levels of rainfall will probably lead to lower vegetation density and water availability in the following month, which could explain the delayed relationship between rainfall and attack severity.
Temporal patterns and the effectiveness of kraals
The fact that there was no significant seasonal trend in the number or severity of attacks suggests that there was relatively high prey availability throughout the year at Pandamatenga, possibly because regions surrounded by protected areas typically have higher ungulate populations (Macdonald & Loveridge, Reference Macdonald and Loveridge2010). We found significantly more incidents occurred outside kraals, which is consistent with other research (Valeix et al., Reference Valeix, Hemson, Loveridge, Mills and Macdonald2012) and suggests kraals are effective to some extent in reducing attacks.
Limitations, and directions for future research
Although we have shown that environmental factors could have significant effects on livestock predation, this only facilitates an understanding of one part of a complex system in which other variables, particularly human factors, are equally important (Oriol-Cotterill et al., Reference Oriol-Cotterill, Macdonald, Valeix, Ekwanga and Frank2015). Future work should encompass a more holistic analysis of the variables affecting predation, including environmental, human, and spatial factors, to compare the effects of these broad categories on livestock predation by multiple large carnivores in different locations. In addition, it would be of value to investigate the effect of temperature and moon phase specifically on livestock predation by other large carnivores in different ecosystems, to establish whether these variables can act as indicators for guiding mitigation more broadly.
We had no information regarding the time of day of attacks, so although most attacks probably occur at night (Macdonald & Loveridge, Reference Macdonald and Loveridge2010), inferences of high temperature avoidance must be taken as general patterns exhibited by the data rather than actual daily preferences shown by lions. Confidence in our results would be strengthened with data on natural prey density and lion predation levels on natural prey, which will have an impact on livestock predation. Additionally, moon brightness varies with weather conditions, and our analysis did not consider cloud coverage, which is probably higher in the wet season. Future work should incorporate these data and involve an analysis of human activities and known attacks on both livestock and wild prey by focusing on activity patterns of individual lions.
Management implications
Environmental variables are easy to monitor and can provide practical guidelines for enhancing mitigation, to help reduce attacks from large carnivores and improve farmer–carnivore coexistence. Considering the demonstrated effect of all environmental variables we investigated, we recommend that livestock farmers increase protective measures for their livestock during colder periods, and particularly when moonlight levels are low. Colder periods typically coincide with the dry season in southern Africa. Furthermore, although the effect of rainfall on livestock predation varies in different areas, measures to prevent predator attacks should be increased in months following lower levels of rainfall in locations surrounded by protected areas. Such measures could include improvements in livestock management, such as keeping cattle within kraals whenever possible and being more vigilant with herding and guarding, using various deterrents (e.g. acoustic, visual or chemical), using specialized guard dogs, or local dogs that act as a warning system, and employing human guardians for livestock (Dickman, Reference Dickman2010). Each area will have different potential for successful mitigations and carnivore conservation based on its ecological, sociological, economic and political profile, and the associated human population pressure (Hemson, Reference Hemson2003; Dickman et al., Reference Dickman, Hinks, Macdonald, Burnham and Macdonald2015). Decreasing predator attacks on livestock could help reduce the persecution of large carnivores and maintain the essential ecosystem services they provide, whilst improving relationships between conservationists and farmers, and facilitating human–carnivore coexistence.
Acknowledgements
JADR thanks Jocelyne Sze Shimin, George Powell and James Foley for their advice on statistical analyses; Joseph Shepherdson, Jim Humphries, James Adams, Jess Williams and Jacky Morrison for reviewing early drafts; and Hil and Jamie Robertson for their support. All authors thank the Department of Wildlife and National Parks for providing the incident reports that made this project possible.
Author contributions
Project conception and study design: JADR, JMR, MR, SGD; writing: JADR; guidance on writing: JMR, SGD; information on local governance, farming practices and lion behaviour: MR, MDB, SGD; initial data collation: MDB; data analysis: JADR, JMR; figures and tables: JADR.
Conflicts of interest
None.
Ethical standards
All authors have abided by the Oryx Code of Conduct for contributors. This project did not involve ethical issues with either human subjects or animals.