1. Introduction
Similar to face-to-face bullying, cyberbullying can be captured formally using the Routine Activities Model (RAM) (see Miethe and Meier (Reference Miethe and Meier1994), wherein ‘a likely offender and a suitable target converge in a place in the abscence of a suitable and capable guardian.’). In cyberbullying, the victim, bystanders and the bully are in close proximity to one another via the interaction of their user profiles on online social networking (OSN) platforms. The cyberbullying nomenclature has evolved alongside paradigm shifts in technology, especially with the sporadic bloom of OSN platforms. Patchin and Hinduja (Reference Patchin and Hinduja2006) define cyberbullying as the infliction of intentional and repetitive harm through electronic and digital technologies. The extensive literature on cyberbullying recognizes that cyberbullying does not only involve predators and victims but also bystanders. Studies by Leung, Wong, and Farver (Reference Leung, Wong and Farver2018) and Song and Oh (Reference Song and Oh2018) strongly suggest that there might be a complex variety of bystanders in cyberbullying. These include,
Bystander-enabler, an individual aware of cyberbullying and enables or motivates the perpetrator to carry on with the victimization of people
Bystander-defender, an individual aware of cyberbullying and calling out the perpetrator.
Studies by Bauman (Reference Bauman2015), Slonje, Smith, and Frisén (Reference Slonje, Smith and Frisén2013) and Nadali et al. (Reference Nadali, Murad, Sharef, Mustapha and Shojaee2013) have discussed the varied forms of antisocial online behaviour that help detect cyberbullying. These include,
Flaming – hostile, angry, insulting interactions that frequently are hurtful personal attacks
Harassment – hostile actions based on someone’s gender, age, race, sexual orientation and is considered illegal.
Denigration – demeaning or disrespecting another person using technology
Masquerading – pretending to be another person and sending messages that appear to come from the victim but in reality, using some level of (technical) sophistication, is sent by the predator/bully
Social Exclusion – deliberate and pointed action to make it clear to individuals that they are not part of the group and that their presence is not wanted
Cyber-stalking – an electronic version of stalking (repeatedly threatening or harassing someone)
Since the announcement of the COVID-19 (SARS-CoV-2) virus pandemic by the World Health Organization (WHO) in March 2020 (World Health Organization, 2020), countries all over the world have enforced lockdown (mandatory quarantine) procedures to help prevent the spread of this virus. Due to such restrictions and with schools moving towards online education, the only source of interaction for children with their peers is via OSN platforms. The recent transparency reportsFootnote a shared by OSN platforms do indicate that there has been both a surge in cyberbullying-related incidents and an increase in moderation and intervention actions by AI-assisted human moderation tools (Facebook Transparency Report 2021; Report, Reference Report2021), (YouTube Community Guidelines enforcement). Leveraging the recent advancements in the field of natural language processing (NLP), deep learning (DL), machine learning (ML) and social network analysis (SNA), these AI moderation tools have enabled companies to tackle 44% of teens/pre-teens related cyberbullying incidents (Armitage, Reference Armitage2021). While the transparency reports by OSN companies indicate progress in detecting and moderating cyberbullying-related comments and posts, the AI-based cyberbullying detection algorithms still require human moderation to a great extent. (Klonick, Reference Klonick2019) Recent studies by Rosa et al. (Reference Rosa, Pereira, Ribeiro, Ferreira, Carvalho, Oliveira, Coheur, Paulino, Veiga Simão and Trancoso2019a), Bayari and Bensefia (Reference Bayari and Bensefia2021a), Emmery et al. (Reference Emmery, Verhoeven, Pauw, Jacobs, Hee, Lefever, Desmet, Hoste and Daelemans2019), Nakov et al. (Reference Nakov, Nayak, Dent, Bhatawdekar, Sarwar, Hardalov, Dinkov, Zlatkova, Bouchard and Augenstein2021), Salawu, He, and Lumsden (Reference Salawu, He and Lumsden2020) and Thomas et al. (Reference Thomas, Akhawe, Bailey, Boneh, Bursztein, Consolvo, Dell, Durumeric, Kelley, Kumar, McCoy, Meiklejohn, Ristenpart and Stringhini2021) have reviewed existing literature on the applications of NLP, DL, ML and SNA techniques and state-of-the-art algorithms to tackle cyberbullying. They identified a scarcity of studies and quality annotated datasets, targeted to identify the diverse roles and forms of cyberbullying.
Developing and disseminating publicly available datasets in the domain of pre-adolescent cyberbullying is an extremely challenging task. There is a myriad of ethical concerns and challenges when focusing on pre-adolescent research. These include but are not limited to consent and assent, maintaining privacy, vulnerability, confidentiality, etc. (Asai, Reference Asai2020; Bailey, Patel, and Gurari, Reference Bailey, Patel and Gurari2021). Of the many cyberbullying-related datasets publicly available, there are to our knowledge only two datasets that provide rich and high-quality annotations. The studies by Van Hee et al. (Reference Van Hee, Jacobs, Emmery, Desmet, Lefever, Verhoeven, De Pauw, Daelemans and Hoste2018) and Sprugnoli et al. (Reference Sprugnoli, Menini, Tonelli, Oncini and Piras2018) in the English/Dutch and Italian languages respectively provide fine-grained annotations for (a) different forms of cyberbullying – insult, threat, exclusion, profanity etc. and (b) different roles in cyberbullying – bully, victim, bystander-assistant, bystander-defender. This level of granularity is in line with the description of cyberbullying by Miethe and Meier (Reference Miethe and Meier1994) (i.e., RAM, Leung, Wong, and Farver (Reference Leung, Wong and Farver2018) and Song and Oh (Reference Song and Oh2018)). As the focus of the study is to tackle cyberbullying in pre-adolescents, we searched for relevant in-domain datasets. Sprugnoli et al. (Reference Sprugnoli, Menini, Tonelli, Oncini and Piras2018) overcome the ethical barriers in pre-adolescent research by engaging pre-teens in role-playing activities on the popular instant messaging platform, WhatsAppFootnote b . This is achieved by creating realistic scenarios for discussion focus groups with Italian pre-adolescents and engaging in annotation activities in order to create a synthetic fine-grained pre-adolescent cyberbullying dataset in Italian. Until now, no such datasets exist in the English language, which poses a significant challenge for developing a native data-driven classification tool in this domain. Given the ethical and methodological challenges mentioned earlier, it is clear that engineering a native English cyberbullying-related synthetic dataset is non-trivial. This motivates the exploration of alternative strategies such as leveraging existing quality annotated data in other languages (i.e., Italian or Dutch ) using (machine) translation techniques.
While human translation (HT) ensures quality translation, it is costly and time consuming (Zhou and Bollegala, Reference Zhou and Bollegala2019). However, recent advances by neural machine translation (NMT) (Vaswani et al., Reference Vaswani, Zhao, Fossum and Chiang2013; Luong, Pham, and Manning, Reference Luong, Pham and Manning2015; Lin et al., Reference Lin, Yao, Yang, Liu, Zhang, Luo, Huang and Su2021) have shown impressive results. In addition, NMT survey studies by Wang et al (Reference Wang, Wu, He, Huang and Ward Church2021) and Ranathunga et al. (Reference Ranathunga, Lee, Skenduli, Shekhar, Alam and Kaur2021) suggest that though there is the scope of improvements in NMT systems, development of tools and resources for low-resourced languages (LRLs) (Koehn and Knowles, Reference Koehn and Knowles2017) have greatly improved. In this study, we leverage advances in NMT to automatically translate labelled user-generated content within the cyberbullying domain from Italian into English language – specifically the dataset by Sprugnoli et al. (Reference Sprugnoli, Menini, Tonelli, Oncini and Piras2018).
Our overall goal is to explore whether the application of NMT machine translation to user-generated Italian source content in the pre-adolescent cyberbullying domain can offer a cost effective and ethical means of automatically producing an equivalent dataset in a target language (English) for building a native in-domain classifier of comparable quality to the original source language (Italian) classifier. Specifically, this paper makes the following novel contributions,
A one of a kind high-quality English gold standard dataset within the cyberbullying domain contains fine-grained annotations by manually translating all messages in the Italian WhatsApp dataset (Sprugnoli et al., Reference Sprugnoli, Menini, Tonelli, Oncini and Piras2018). In addition, we map the fine-grained source annotations of this dataset over to the target dataset (English). (See Section 3)
Quality assessment and estimation with respect to our human-translated English dataset. This is done at the human level by (i) assessing the translation quality factoring in both source and target data (ii) estimating the translation quality of the English output. (See Section 5)
By leveraging both the back-translation technique and the encoder-decoder architectureFootnote c of transformers, we train the MT systems to translate the Italian corpus to English. Due to the lack of domain-specific datasets, we leverage out-of-domain user-generated content for training the MT systems. (See Section 4.2)
By conducting a series of binary cyberbullying experiments with ML/DL techniques we ascertain that English output of an NMT system can be leveraged for classifier training, achieving approximately similar performance metrics to a system to human-translated English test data. (See Section 4.3)
We also replicate the experiments by Corazza et al. (Reference Corazza, Menini, Cabrio, Tonelli and Villata2019) to examine the gold standard corpus with the native classifier. (See Section 4.3)
With the help of cyberbullying domain experts and a teen, the translated corpus are examined for any contextual disparities. (See Section 6.1)
The present study is further divided into five sections. Relevant literature that has influenced this study is discussed in Section 2. In Section 3, we examine the dataset developed by Sprugnoli et al. (Reference Sprugnoli, Menini, Tonelli, Oncini and Piras2018). The HT and machine translation (MT) techniques are discussed in detail in Section 4. Finally, we discuss the results of the translation experiments as well as the performance of the original binary classifier by Corazza et al. (Reference Corazza, Menini, Cabrio, Tonelli and Villata2019) after retraining on the translated English output in Section 5.
2. Related work
While text-based interaction remains the dominant form of communication on OSN platforms, the level of connectivity attained by textual communication can potentially lead to the exchange of profanity, non-consensual or bullying messages amongst pre-adolescents (PEW Research Center, 2015). The detection of cyberbullying, online harassment, online abuse or hate speech is often systematized as a classification problem. Studies by Emmery et al. (Reference Emmery, Verhoeven, De Pauw, Jacobs, Van Hee, Lefever, Desmet, Hoste and Daelemans2021), Salawu et al., (Reference Salawu, He and Lumsden2017), Bayari and Bensefia (Reference Bayari and Bensefia2021b), Vidgen and Derczynski (Reference Vidgen and Derczynski2020) and Rosa et al. (Reference Rosa, Pereira, Ribeiro, Ferreira, Carvalho, Oliveira, Coheur, Paulino, Simão and Trancoso2019b) have examined and reviewed both the computational approaches in cyberbullying detection and the existing cyberbullying-related datasets. These studies have categorized almost all cyberbullying research in the amalgamated fields of NLP, ML, DL and SNA in three groups, viz, binary cyberbullying detection research, fine-grained cyberbullying detection research and multilingual cyberbullying detection research.
2.1 Binary cyberbullying detection research
Computational research for the past few decades has been focused on engineering supervised classifiers from a training corpus containing a set of labelled sentences or phrases to classify an unlabelled sentence as bullying and non-bullying. Such a task that aims to predict the distinction between bullying and non-bullying sentences or phrases is called a binary cyberbullying classification task. Studies by Yin et al. (Reference Yin, Xue, Hong, Davison, Kontostathis and Edwards2009) and Reynolds, Kontostathis, and Edwards, (Reference Reynolds, Kontostathis and Edwards2011) trained traditional ML algorithms like Support Vector Machines (SVM) and Decision Tree classifiers on the CAW 2.0 (Content Analysis for Web 2.0) datasetFootnote d to make such binary cyberbullying classifications. Emmery et al. (Reference Emmery, Verhoeven, De Pauw, Jacobs, Van Hee, Lefever, Desmet, Hoste and Daelemans2021) suggest that studies using the CAW 2.0 dataset are generally unsuitable for cyberbullying classification as in addition to only providing harassment labels, the conversations are generally between adults and lack cyberbullying-related labels. Studies by Dinakar, Reichart, and Lieberman (Reference Dinakar, Reichart and Lieberman2011) and Sanchez and Kumar (Reference Sanchez and Kumar2011) accumulated comments from YouTubeFootnote e and TwitterFootnote f respectively to formulate a binary cyberbullying dataset. The study by Bayzick, Kontostathis, and Edwards (Reference Bayzick, Kontostathis and Edwards2011) gathered text-based and user profile-based data from OSN websites MySpace and now outdated Formspring.meFootnote g . Such datasets are the earliest cyberbullying-related datasets that have been annotated by at times by two or more human annotators. Another study by Bretschneider and Peters (Reference Bretschneider and Peters2016) collected text data from discussion forums of two popular online multiplayer games, World of Warcraft (WoW)Footnote h and League of Legends (LoL)Footnote i . This dataset was first annotated by three human annotators for the presence of harassment and later for the two cyberbullying roles – bully and victim. More recent studies by Dadvar and Eckert (Reference Dadvar and Eckert2018), Iwendi et al. (Reference Iwendi, Srivastava, Khan and Maddikunta2020), Gada, Damania, and Sankhe (Reference Gada, Damania and Sankhe2021), Al-Hashedi, Soon, and Goh (Reference Al-Hashedi, Soon and Goh2019), Al-Garadi, Varathan, and Ravana (Reference Al-Garadi, Varathan and Ravana2016), Paul and Saha (Reference Paul and Saha2020), and others have demonstrated accurate and precise binary cyberbullying classifications by leveraging above mentioned datasets and recent DL approaches to NLP such as Recurrent Neural Networks (Medsker and Jain, Reference Medsker and Jain2001), Long-term Short-term Memory (Sundermeyer, Schlüter, and Ney, Reference Sundermeyer, Schlüter and Ney2012), Gated Recurrent Units (GRUs) (Cho et al., Reference Cho, Van Merriënboer, Gulcehre, Bahdanau, Bougares, Schwenk and Bengio2014) and Bidirectional Encoder Representations from Transformers (BERT) (Devlin et al., Reference Devlin, Chang, Lee and Toutanova2018). Though such studies have reported high accuracy and precision in their classification predictions, they are unable to detect the key elements of cyberbullying – as understood from a social science perspective. Despite their many advantages, these studies cannot be relied on their entirety for effective detection of cyberbullying amongst adults and pre-adolescents.
2.2 Fine-grained cyberbullying detection research
One of the common drawbacks of previously discussed research was their focus on binary classification of a single text-based message as bullying or non-bullying. A study by Xu et al. (Reference Xu, Jun, Zhu and Bellmore2012) in the past decade has taken the first steps towards unearthing traces of cyberbullying by finding the multiple forms and roles of bullying. This research has conceptualized the fine-grained approach for cyberbullying detection on OSN platforms. Many recent studies are influenced by the work of Xu et al. (Reference Xu, Jun, Zhu and Bellmore2012) and can devise high-quality cyberbullying-related datasets. A study by Van Hee et al. (Reference Van Hee, Jacobs, Emmery, Desmet, Lefever, Verhoeven, De Pauw, Daelemans and Hoste2018) aims at detecting signals of cyberbullying on OSN platforms by collecting text-based content from ASK.fmFootnote j platform. Their research focus is targeted towards detection of the multiple roles in cyberbullying like bullies, victims and bystanders, and multiple forms of cyberbullying like insults, threats, curses, defamation, sexual talk, etc. For this purpose, Van Hee et al.(Reference Van Hee, Jacobs, Emmery, Desmet, Lefever, Verhoeven, De Pauw, Daelemans and Hoste2018) collected data from ASK.fm, and by extracting text-based features like the n-gram bag-of-words (BoW), subjectivity lexicon features – features that help ascertain the positive and negative word ratios, devised a primitive classifier using SVM to make classification predictions. More recent studies by Hosseinmardi et al. (Reference Hosseinmardi, Mattson, Rafiq, Han, Lv and Mishra2015) and Rafiq et al. (Reference Rafiq, Hosseinmardi, Han, Lv, Mishra and Mattson2015) collected data from widely used OSN platforms like InstagramFootnote k and now obsolete media-sharing platform, VineFootnote l , respectively. Their work is fundamental in distinguishing between cyber aggression and cyberbullying. They suggest that the LIWC (linguistic inquiry and word count) (Pennebaker, Francis, and Booth, 2001) categories such as death, appearance, religion and sexuality are adequate indicators of cyberbullying. Their research is one of the first to leverage both metadata features such as user profile properties, image and video content, and text-based features like bag-of-words for making fine-grained cyberbullying classifications. Despite many recent studies by Chen and Li (Reference Chen and Li2020), Cheng et al. (Reference Cheng, Li, Silva, Hall and Liu2019) and others leveraging such datasets, they are still far off from detecting cyberbullying amongst pre-adolescents.
2.3 Multilingual cyberbullying detection
The earlier discussed dataset by Van Hee et al. (Reference Van Hee, Jacobs, Emmery, Desmet, Lefever, Verhoeven, De Pauw, Daelemans and Hoste2018) is the first multilingual cyberbullying dataset in English and Dutch with high-quality annotations. With a corpus size of 113,698 English sentences or phrases and 78,387 Dutch sentences and phrases, this dataset has very sparse bullying-related annotations. This English and Dutch corpus have only 4.73 % and 6.97% bullying posts, respectively. Despite their pre-eminent efforts to construct an SVM classifier with hyper-parameter optimization, the highly imbalanced and sparse nature of the dataset made it difficult to achieve accurate and precise cyberbullying detection. With over 300 million Arabic speakers worldwide, Haidar, Chamoun, and Serhrouchni (Reference Haidar, Chamoun and Serhrouchni2019) devised a binary Arabic cyberbullying corpus, that is, a corpus in Arabic language with binary labels that only capture the presence or absence of cyberbullying in a sentence or a phrase. Though they gathered Arabic tweets based on popular hashtags (trends) on Twitter, their dataset is also sparse, with only 8.6% bullying-related tweets. In multilingual societies, the usage of code-switching languages is quite common for conveying opinions on OSNs. Code-switching is a natural occurrence when speakers alternate between a variety of languages (Gysels, Reference Gysels1992; Myers-Scotton, Reference Myers-Scotton1993; Poplack and Walker, Reference Poplack and Walker2003). Recent studies by Kumar and Sachdeva (Reference Kumar and Sachdeva2020) and Bohra et al. (Reference Bohra, Vijay, Singh, Akhtar and Shrivastava2018) construct a Twitter-based code-switched cyberbullying corpus with Hindi (regional Indian language) and the English language. Despite their linguistic novelties, these datasets are annotated for the task of binary classification, so tweets are labelled only for the presence or absence of cyberbullying. Of all the datasets that currently exist in the public domain, the dataset developed by Sprugnoli et al. (Reference Sprugnoli, Menini, Tonelli, Oncini and Piras2018) for the European Project CREEPFootnote m is undoubtedly the only relevant pre-adolescent cyberbullying dataset publicly available in the Italian language.
2.4 MT for user-generated content
Some papers investigate translating social media texts in order to map widely available English sentiment labels to a less supported target language and thus be able to perform the sentiment analysis in this language (Balahur and Turchi, Reference Balahur and Turchi2012, Reference Balahur and Turchi2014). Several researchers attempted to build parallel corpora for user-generated content in order to facilitate MT. For example, translation of Twitter micro-blog messages by using a translation-based cross-lingual information retrieval system is applied in Jehl, Hieber, and Riezler (Reference Jehl, Hieber and Riezler2012) on Arabic and English Twitter posts. Ling et al. (Reference Ling, Xiang, Dyer, Black and Trancoso2013) crawled a considerable amount of Chinese-English parallel segments from micro-blogs and released the data publicly. Another publicly available corpus, TweetMT (naki San Vicente et al., Reference San Vicente, Alegria, España-Bonet, Gamallo, Oliveira, Garcia, Toral, Zubiaga and Aranberri2016), consists of Spanish, Basque, Galician, Catalan and Portuguese tweets and has been created by automatic collection and crowd-sourcing approaches. The authors (Banerjee et al., Reference Banerjee, Naskar, Roturier, Way and van Genabith2012) investigated domain adaptation and reduction of out-of-vocabulary words for English-to-German and English-to-French translation of web forum content. Estimation of comprehensibility and fidelity of machine-translated user-generated content from English to French is investigated in Rubino et al. (Reference Rubino, Foster, Kaljahi, Roturier and Hollowood2013), whereas Lohar, Afli, and Way(Reference Lohar, Afli and Way2017) and (Reference Lohar, Afli and Way2018) explore maintaining sentiment polarity in German-to-English MT of Twitter posts. Overall translation performance of NMT systems for user reviews of IMDb movies and Amazon products was explored in Lohar, Popović, and Way (Reference Lohar, Popović and Way2019)and Popović et al. (Reference Popović, Poncelas, Brkić Bakarić and Way2021). As for hate-speech detection, (Ibrahim, Torki, and El-Makky, Reference Ibrahim, Torki and El-Makky2020) used MT in order to balance the distribution of classes in training data. Existing English tweets were machine-translated into Portuguese (shown to be the best option), and then, these translations were translated back into English. In this way, new tweets were created with different words and/or a different structure – the number of instances of rare classes was increased, as well as the diversity of data, without collecting any new data. To the best of our knowledge, MT has not yet been investigated in the context of cross-lingual hate-speech detection.
3. The WhatsApp dataset
Social scientific research studies by DeSmet et al. (Reference DeSmet, Rodelli, Walrave, Soenens, Cardon and De Bourdeaudhuij2018), Lee and Shin (Reference Lee and Shin2017), Van Royen et al. (Reference Van Royen, Poels, Vandebosch and Adam2017), Leung, Wong, and Farver (Reference Leung, Wong and Farver2018), and Song and Oh (Reference Song and Oh2018) suggest that detection, intervention and prevention strategies to tackle cyberbullying need to observe social interactions amongst pre-adolescents at a fine-grained level. Such social scientific research encourages the creation of detailed taxonomy with a vast variety of categories that help in distinguishing between different forms and user roles in cyberbullying. The Italian corpus developed by Sprugnoli et al. (Reference Sprugnoli, Menini, Tonelli, Oncini and Piras2018) via the aforementioned CREEP project is the only dataset for pre-adolescents available in the public domain. Furthermore, this dataset was devised synthetically by engaging Italian pre-teens in role-playing scenarios on an instant messaging social networking platform, WhatsApp. The reader should note that it is very challenging to collect data from this platform due to its end-to-end encryptionFootnote n that makes data inaccessible for extraction (Verheijen & Spooren Reference Verheijen and Spooren2017). Overall, 70 pre-adolescents aged between 12 and 13 years participated in this activity. The participants engaged in discourse on 10 different WhatsApp conversations on four different scenarios. The four different scenarios were labelled as A, B, C and D by the original Italian dataset authors. Each of these scenario-based discourses had different number of sentences, together amounting to 2192 sentences as represented in Table 1.
Table 1. Scenario-wise sentence breakdown.
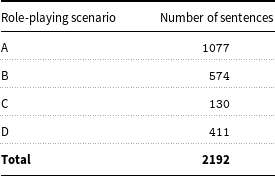
The goal of their study was to analyse the linguistic encoding of cyberbullying in WhatsApp messages. The Italian dataset is the only annotated WhatsApp corpus freely available with fine-grained labels that aid in recognition of both the complex forms of cyberbullying – insult, threat, curse, exclusion, etc, but also the complex roles in the cyberbullying phenomenon – victim, bystander-assistant, bystander-defender, bully. Tables 2 and 3 represent the number of multiple cyberbullying entities and roles annotated by researchers Sprugnoli et al. (Reference Sprugnoli, Menini, Tonelli, Oncini and Piras2018). Due to the unavailability of such rich labelled corpus in the English language catering towards pre-teens, we felt it was important to explore MT as a potential avenue for generating quality training data in other languages (English in this case) as mechanism for rapid cross-lingual language resource engineering in this domain.
Table 2. Count-wise fine-grained entity-based annotations.

Table 3. Count-wise fine-grained role-based annotations.

Another study by researchers Corazza et al. (Reference Corazza, Menini, Cabrio, Tonelli and Villata2019) based on this dataset and other Italian online hate-related datasetsFootnote o consolidated the multiple annotations for the WhatsApp corpus in Tables 2 and 3 to construct binary hate/ non-hate dataset for a cross OSN platform hate classification task. As the label consolidation technique was not available in their work, an e-mail exchange with the authors helped to elucidate this matter (Corazza, Reference Corazza2021). We were informed that a message is considered as hate (binary label), if it does not contain the following labels,
Defence in entity-type
Encouragement_to_the_Harasser in entity-type
Flagged as non-offensive
Victim in role-type
After following this consolidation strategy of merging the multiple labels into binary labels as hate and non-hate, we were left with only 741 sentences labelled as hate. In Section 4, we discuss in detail the replication of the original experiments (Corazza et al., Reference Corazza, Menini, Cabrio, Tonelli and Villata2019) with respect the original source (Italian) dataset as well both the human and machine English translations, respectively.
4. Experimental setup
To explore whether the application of NMT and HT techniques for user-generated Italian source pre-adolescent cyberbullying-related data can aid in devising a one of a kind high-quality English gold data standard, we conducted a series of experiments. These experimental tasks include,
HT
MT
Hate-speech Binary Classifier
4.1 HT
The HT task was conducted in collaboration with professional linguists at TransPerfectFootnote p . TransPerfect is a translation, electronic discoveryFootnote q and language services company based in New York City, USA, with offices in Dublin, Ireland. All professional linguists at TransPerfect meet the requirements of ISO 17100Footnote r – the translation industry-standard. The source Italian language cyberbullying dataset by Sprugnoli et al. (Reference Sprugnoli, Menini, Tonelli, Oncini and Piras2018) comprising 2192 sentences from all four scenarios was translated to the target language – English. The translation process required native speakers of both Italian and English language, to perform granular analysis of the Italian set and relaying the messages in English while capturing the same connotations, expressiveness and intent. The process involved three professional linguists, one translator, one editor and one proofreader. The linguists were all certified and fully briefed on the needs of the experiment and the level of attention and precision required.
To assess the quality of the translation, we relied on both HT quality metrics and error analysis by domain experts. Both metrics and domain analysis are discussed in detail in Sections 5.1 and 5.2, respectively.
4.2 MT
Our systems are based on the Transformer architecture (Vaswani et al., Reference Vaswani, Shazeer, Parmar, Uszkoreit, Jones, Gomez, Kaiser and Polosukhin2017) and built using the Sockeye implementation (Hieber et al., Reference Hieber, Domhan, Denkowski, Vilar, Sokolov, Clifton and Post2018). The systems operate on sub-word units generated by byte-pair encoding (BPE) (Sennrich, Haddow, and Birch, Reference Sennrich, Haddow and Birch2016) with 32000 BPE merge operations both for the source and for the target language texts.
The systems have Transformer architecture with 6 layers for both the encoder and decoder, model size of 512, feed forward size of 2048, and 8 attention heads. For training, we use Adam optimizer (Kingma and Ba, Reference Kingma and Ba2015a), initial learning rate of 0.0002, and batch size of 4096 (sub)words. Validation perplexity is calculated after every 4000 batches (at so-called ‘checkpoints’), and if this perplexity does not improve after 20 checkpoints, the training stops.
To build an MT system for a specific domain, in our knowledge the best way is to take parallel data from the cyberbullying domain for training. The key problem in the cyberbullying domain is as highlighted by studies like Emmery et al. (Reference Emmery, Verhoeven, De Pauw, Jacobs, Van Hee, Lefever, Desmet, Hoste and Daelemans2021), Salawu et al. (Reference Salawu, He and Lumsden2017), Bayari and Bensefia (Reference Bayari and Bensefia2021a), Vidgen and Derczynski (Reference Vidgen and Derczynski2020), and many other studies are the paucity of quality cyberbullying datasets. So, we leverage out-of-domain data (texts which are not from this domain but other domains). In our case, for out-of-domain data, we leverage existing user-generated corpus from news, subtitles, technical manual, etc. One way to do it is to use in-domain monolingual data in the target language, translate them by an MT system and build a ‘synthetic’ in-domain data where the target language part is ‘normal’ and the source language part is a MT. This method is called back-translation, and it is widely used in modern MT systems. The usual way is then to combine this synthetic in-domain data with out-of-domain parallel data.
As the scope of this study is focused on cyberbullying classification and detection amongst pre-adolescents and due to the lack of such distinct datasets, we leveraged other in-domain datasets. So, we used following datasets for the in-domain (cyberbullying) English data,
Instagram cyberbullying corpus by Hosseinmardi et al. (Reference Hosseinmardi, Mattson, Rafiq, Han, Lv and Mishra2015)
Vine cyberbullying corpus by Rafiq et al. (Reference Rafiq, Hosseinmardi, Mattson, Han, Lv and Mishra2016)
ASK.fm cyberbullying English corpus by Van Hee et al. (Reference Van Hee, Jacobs, Emmery, Desmet, Lefever, Verhoeven, De Pauw, Daelemans and Hoste2018)
For out-of-domain data, we used a portion of English-Italian OpenSubtitles publicly available on the OPUS web-siteFootnote s .
We investigated the following two set-ups:
training on in-domain synthetic data
training on this data in combination with natural out-of-domain data
Finally, for the test set, we used the WhatsApp test-set both with and without emojis. Table 4 depicts the number of sentences (segments) for both training and test sets for the MT system.
Table 4. Number of segments (sentences) in training and test data for MT systems.

4.3 Hate-speech binary classifier
In the recent study by Corazza et al. (Reference Corazza, Menini, Cabrio, Tonelli and Villata2019) to evaluate cross-platform hate-speech detection, the authors leveraged the Italian WhatsApp corpus and other freely available datasets from OSN platforms like Twitter and Instagram. To ascertain any contextual disparities between the original Italian corpus and both human and machine translations into English, we replicated the binary hate-speech classifier made available by Corazza et al. (Reference Corazza, Menini, Cabrio, Tonelli and Villata2019). The rationale for adopting this replication strategy is to assess if there is any significant variance between the original source Italian and both HT & MT English translations with respect to the same binary classification task in Corazza et al. (Reference Corazza, Menini, Cabrio, Tonelli and Villata2019). We believe this strategy helps in observing any contextual loss, that is, to observe if the binary classification for an Italian sentence is hate, does the same binary classifier (with language specific embeddings) classify the corresponding HT or MT translated English (test) sentence as hate or non-hate.
Furthermore, we conducted the classification experiments with other native binary classifiers leveraged in existing literature for binary cyberbullying detection. To create a baseline system for assessing model behaviour on the different sourced datasets, we used traditional supervised ML, ensemble learning and DL algorithms. To assess the performance of each of the algorithms, we utilized pre-trained Twitter-based fastTextFootnote
t
word embeddings and generated word embeddings using the native Term Frequency-Inverse Document Frequency (TF-IDF) technique (Yun-tao, Ling, and Yong-cheng, Reference Yun-tao, Ling and Yong-cheng2005). The classification algorithms we employed included ML algorithms like SVMFootnote
u
and Decision TreeFootnote
v
, ensemble learning bagging algorithm Random ForestFootnote
w
and boosting algorithm XGBOOSTFootnote
x
, and DL algorithms like bi-drectional Long-short-Term Memory (Zhou et al., Reference Zhou, Qi, Zheng, Xu, Bao and Xu2016), and Convolutional Neural Networks (Moriya and Shibata Reference Moriya and Shibata2018). Recent studies by Chen and Li (Reference Chen and Li2020) and Cheng et al. (Reference Cheng, Li, Silva, Hall and Liu2019) have also leveraged the latest NLP development by Devlin et al. (Reference Devlin, Chang, Lee and Toutanova2018) BERT for binary cyberbullying detection. Hence, we also leveraged pre-trained BERT
$_{\text{base-uncased}}$
model for this binary classification task.
4.3.1 Dataset split
The original dataset by Sprugnoli et al. (Reference Sprugnoli, Menini, Tonelli, Oncini and Piras2018) was developed by engaging pre-teens in role-playing based on four scenarios and was retrieved from four different WhatsApp group conversations. Every sentence retrieved from a group conversation is contextually and semantically related to sentences from that group conversation. So, for the purpose of HT quality error analysis (further discussed in Section 5.2), we split the 130 sentences from Scenario-C as an additional hold-out test set with supplementary binary labels. We split the remaining corpus data as training, validation, hold-out test set and their sizes are represented in Table 5. In Section 5.2, we discuss in detail the rationale for the domain expert error analysis with additional binary labels.
Table 5. Dataset split size.

4.3.2 Hyper-parameters
Corazza et al. (Reference Corazza, Menini, Cabrio, Tonelli and Villata2019) developed the binary hate classifier on an Italian corpus and leveraged the Italian FastTextFootnote y embeddings with a size of 300. In our experiments with the Italian and English translated corpus, we used both English and Italian FastText embeddings of the same size, respectively. Byrd and Lipton (Reference Byrd and Lipton2019) in their recent study describe that the machine and DL model must make errors somewhere when training on a training set. So, by feeding neural network weights into the model, Corazza et al. altered the relative contribution of mistakes on various training points of the loss function. As the experiments by Corazza et al. were conducted a few years ago, the neural network weights were not available; hence, we reproduced the experiments without any such weights. Corazza et al. also used binary cross-entropy Footnote z as the loss function to train the model. scores. The details hyper-parameter optimization for the replicated GRU-binary classifier are as follows,
Recurrent Layer size: 200
Batch size: 32
Embedding size: 300
Epochs: 5
Lambda (regularization): 0.1
Loss Function: Binary Cross Entropy
Optimizer Function: ADAM
Activation Function: Sigmoid
To leverage the best ML or DL algorithm and to avoid any over-fittingFootnote aa and under-fittingFootnote ab on the training set, we used the grid search (Pedregosa et al., Reference Pedregosa, Varoquaux, Gramfort, Michel, Thirion, Grisel, Blondel, Prettenhofer, Weiss, Dubourg, Vanderplas, Passos, Cournapeau, Brucher, Perrot and Duchesnay2011) algorithm. This algorithm enables an estimator model to capture the accurate relationship between the variables in the data. The algorithm allows the estimator model to loop through a set of pre-defined hyper-parameters to find the optimal hyper-parameters that prompt best classification or prediction scores. In our experiments, we leverage the grid search algorithm to find optimal number of epochs and the batch size.
4.3.3 Pre-processing
Text pre-processing is often referred to as the first step in the NLP pipeline. Some extensively used text pre-processing techniques include lemmatization, stemming, lower-casing, spell correction, tokenization or multi-word grouping In this study, we conduct the following text pre-processing tasks,
Change all text to lower-case
Transcribe emojis in text in English using official plain-text description of emojisFootnote ac
Construct an additional corpus by removing all emojis in the text
4.3.4 BERT Fine-tuning
Leveraging the BERT model by Devlin et al. (Reference Devlin, Chang, Lee and Toutanova2018) with 12 layers (transformer blocks) and trained with 110 M parameters, we fine-tune pre-trained BERT-base-uncased model with different hyper-parameters on the training data. Our experimentsFootnote ad utilized the implementations provided by HuggingFace’s Transformer library (Wolf et al., Reference Wolf, Debut, Sanh, Chaumond, Delangue, Moi, Cistac, Rault, Louf, Funtowicz and Brew2019). We trained the BERT model for both 2 and 4 epochs and fine-tuned each model for both HT and MT datasets individually and set the maximum sequence length between 128 and 256 tokens. We fine-tuned the classification layer for transformer-based models using ReLU and the Adam Weighted optimizer (Kingma and Ba, Reference Kingma and Ba2015b) with a learning rate ranging from 0.1, 0.001, 1e-5, 5e-6.
5. Results
In this section, we examine the results of our experiments with respect to (i) human and (ii) MT of the fine-grained Italian WhatsApp dataset (Sprugnoli et al., Reference Sprugnoli, Menini, Tonelli, Oncini and Piras2018) to English (iii) our domain expert error analysis of the human-translated output and iv) our experiments in training a binary classifier for hate speech in English using the translated dataset.
5.1 HT quality assurance
Transperfect relies on a proprietary hybrid model established on LISA QA and SAE J2450 (Martínez, Reference Martínez2014) quality metrics for the purposes certifying its professional translator. This proprietary technology offers automated checks for numbers, consistency of terms, acronyms, spelling, etc. With respect to the translated dataset (See Section 3), three professional linguists and two quality assurance professionals at Transperfect vetted the quality of the translation output. However, for social media generated texts TransPerfect had to primarily rely on annotations and input from their linguists given the fact that this type of content does not follow the same rules as any other publication material for instance The use of ellipses (e.g., ttyl), modified spelling (yasss vs yes), use of punctuation (a period being viewed as aggressive, using multiple exclamation points for expressiveness, etc), emoticons, etc. This type of digital language is new and requires a specific approach and understanding from both translation and analytical standpoint to correctly decode and interpret the content.
5.1.1 Data availability
The translated corpus is available online at https://gitlab.computing.dcu.ie/vermak3/translated-bullying-whatsapp-corpus
5.2 Domain expert error analysis
As discussed in earlier in Sections 1 and 2, respectively, for a sentence to be labelled as cyberbullying, the sentences need to fit into one or more of the multiple forms of antisocial online behaviour like abusive, offensive, harassing, masquerading For domain expert annotators to adjudicate if the sentence qualifies as cyberbullying, it is important to semantically and contextually understand the sentences. The original dataset by Sprugnoli et al. (Reference Sprugnoli, Menini, Tonelli, Oncini and Piras2018) was developed based on four role-playing scenarios by a group of pre-adolescents on the instant messaging platform, WhatsApp in the Italian language and was translated in the target language, English, by human translators. As earlier discussed in Section 4.3.1, the semantics of each sentence from a group conversation naturally depend on the context of the surrounding discourse; we selected all sentences from Scenario-C to be analysed by Domain experts.
Scenario – C of the original study by Sprugnoli et al. (Reference Sprugnoli, Menini, Tonelli, Oncini and Piras2018) is a case study where:
‘Your classmate is very good at school, and everyone thinks he is an overachiever. He studies a lot and he never goes out. He does not speak much with his classmates, who from time to time tease him for his unsocial life. Things have slightly changed recently: your classmate’s mum convinced teachers to increase the homework for all the students. A heedless teacher revealed the request to the classroom, and now some students are very angry at him’.
We conducted an error analysis of the translated English language from both a computational linguistic and a social scientific perspective. Three annotators reviewed the 130 translated sentences in Scenario-C from Sprugnoli et al. (Reference Sprugnoli, Menini, Tonelli, Oncini and Piras2018) (See Table 1). The annotators included (a) one teenager (target stakeholder) and native speaker of English, (b) two cyberbullying experts of which one is a native Italian speaker and was allowed to leverage the original Italian source language dataset. Following the United Nations Convention on the Rights of the Child (UNCRC)’s Article 12, children (considered as those under the age of 18) have the right to be heard on matters that concern them. (UNCRC, 2021). It is therefore important to include the target stakeholders (teenagers in this context) into the research process. This evaluation involves two types of error analysis:
Original labelling error analysis, which involved the identification and classification of label errors in the manually translated English output. The labels were mapped over from the Italian source and are based on a study by the original authors (Sprugnoli et al., Reference Sprugnoli, Menini, Tonelli, Oncini and Piras2018). The authors consolidated multiple labels into binary labels (hate/non-hate) for conversion into a binary classification task (offensive, non-offensive). We include this error analysis as we are interested in analysing the binary labels from domain experts’ perspective (both social scientists with expertise in cyberbullying).
Domain expert translation error analysis which involves the identification of translation errors in the manually translated English output which affects the original labels. The domain expert native Italian speaker analysed the translations for both (a) translation errors. affecting original source labels and (b) translation errors not affecting the original labels. All translation errors were considered as word choice errors which resulted in ambiguous translations. See Section 6.1 for example errors.
The results for the above can be found in the Table 6, (please note, B.E denotes Bullying (domain) Expert).
Table 6. Translation ambiguity, original labelling and domain expert error analysis.

All three annotators were provided with clear instructions to assess whether the translated sentences are relatively correct or accurate as examples of harassment or non-harassment, based on their knowledge. The sample sentences provided to the annotators may not have met the full criteria of cyberbullying – in that abusive or harassing activity may not be repetitious; hence, they labelled it at minimum as abuse or harassment (offensive). Such types of labels are commonly referred to as binary labels. To examine the inter-annotator reliability, we leveraged Krippendorff’s alpha (Hayes and Krippendorff, Reference Hayes and Krippendorff2007) as discussed in studies by Bermingham and Smeaton (Reference Bermingham and Smeaton2009) and Warrens (Reference Warrens2010) for statistically evaluating the inter-annotator agreement between two or more annotators. Krippendorff’s alpha is a robust probabilistic measure that observes variability between annotators is due to chance and does not require that every annotator annotate every document.
$\alpha$
(Krippendorff’s alpha) for the three annotators is 0.7725, this indicates that there is a significant level of agreement between the annotators.
The authors of the original study annotated the sentences for multiple labels that is, they identified not only the form of cyberbullying (curse, exclusion, insult, etc.) but also the role of cyberbullying (victim, bystander_assistant, bully, etc) for each sentence. In one study by original authors Corazza et al. (Reference Corazza, Menini, Cabrio, Tonelli and Villata2019), they consolidated the multiple labels into binary labels (hate/non-hate). This label consolidation method was not available in their study and as discussed earlier in Section 3, an mail exchange with the authors (Corazza, Reference Corazza2021) helped clarify this matter. We followed the same strategy by Corazza et al. (Reference Corazza, Menini, Cabrio, Tonelli and Villata2019) to consolidate the multiple labels into binary hate/non-hate labels.
To assess each of our annotator’s agreement levels with the original authors’ consolidated binary labels in the study by Corazza et al. (Reference Corazza, Menini, Cabrio, Tonelli and Villata2019), we leveraged the Cohen’s Kappa coefficient (McHugh, Reference McHugh2012) to calculate the annotator reliability level.
Table 7 (please note, B.E denotes Bullying (domain) Expert) represents the inter-annotator reliability agreement of each of our annotators with the original annotations. The results range from 0.57/0.69/0.73 for the three annotators, which indicates that the annotators do have a significant level of agreement with original annotations. This tells us that the semantic context of sentences was not lost during HT, and our annotators interpreted the translated text in similar manner as the original annotators.
Table 7. Cohen’s Kappa score for individual annotators with original annotations.

5.3 MT results
We first evaluated all translation outputs using the following overall automatic MT evaluation metrics: BLEU (Papineni et al., Reference Papineni, Roukos, Ward and Zhu2002) and chrF (Popović, Reference Popović2015). BLEU is a word-level metrics based on precision, whereas chrF is a character-based metric based on F-score, namely both precision and recall. Both metrics are based on n-gram matching, BLEU on word n-grams and chrF on character n-grams. The results can be seen in Table 8.
Table 8. Comparison of Italian-English systems by automatic evaluation scores BLEU and chrF.

As expected, increasing training data by out-of-domain data notably improved the scores. Furthermore, it can be seen in the Table 8 that both MT systems perform better on test set without emoticons.
While the system performance in terms of MT scores can certainly be further improved in future work, the focus of the experiment was to ascertain if machine-translated English output can be leveraged in train a native binary cyberbullying classifier, which is discussed in the Section 5.4
5.4 Hate-speech binary classifier results
The F-measure or F-statistic is a measure to test the accuracy of the estimator model. Calculated as the harmonic mean of precisionFootnote ae and recallFootnote af , it is commonly used to assess a binary classification model’s performance (Sasaki, Reference Sasaki2007). Opitz and Burst (Reference Opitz and Burst2019), and many other statistical studies suggest that ‘macro f1’ statistics is an ideal performance measure while classifying unevenly distributed classes as it gives equal importance to each class. As a result, we rely on ‘macro f1’ statistic to determine the performance of the binary classifiers.
5.4.1 GRU replication results on the Italian corpus
Table 9 represents the F-statistic results reported by both the original study by Corazza et al. (Reference Corazza, Menini, Cabrio, Tonelli and Villata2019) and the replication experiment using the GRU architecture on the raw Italian corpus. Due to the unavailability of weights as discussed in Section 4.3.2, we observed an infinitesimal drop in classification scores. (See Table 9) As discussed in Section 4.3.2, due to the unavailability of the neural network weights used by the original study, we observe a very infinitesimal difference in both F-statistic scores. This difference is insignificant, and therefore, we can state that we were able to reproduce the GRU architecture with similar precision and accuracy.
Table 9. Original and replicated classification results on the Italian corpus.

5.4.2 Binary classification results with original labels for HT and MT corpus
In addition to reproducing the binary hate classifier for the Italian corpus, as discussed in earlier sections, we conducted binary hate classification experiments with other DL and ML models. As seen in Table 10, fine-tuning pre-trained BERT bert-base-uncased model by Devlin et al. (Reference Devlin, Chang, Lee and Toutanova2018) with transcribed Emojis on the human-translated English corpus outperforms all the other ML and DL classifiers and yields the best result with 0.83 macro-average F1 score. This fine-tuned BERT model on the MT English corpus also outperforms other ML and DL models trained on the same corpus with 0.76 macro-average F1 score. Detailed F-statistic performance of all models trained on both HT and MT English corpus and test on the hold-out set can be found in Appendix 1 in supplementary material.
Table 10. Hold-out test set binary classification best results with GRU.

5.4.3 Binary hate classifier with expert annotations
The rationale for domain expert annotations discussed in Section 5.2 aids in engineering additional labels for Scenario-C, and as observed in Section 4.3.1, we test all the ML and DL models on these additional binary labels. Table 11 shows that the fine-tuned pre-trained BERT bert-base-uncased model outperforms all the other ML and DL models with a F1 macro-average score of 0.81 and 0.75 on the human-translated and machine-translated English corpus respectively. Detailed F-statistic performance of all models trained on MT English corpus and test on the Scenario-C set can be found in Appendix 2 in supplementary material.
Table 11. Binary classification best results on Scenario-C additional annotations.

6. Discussions and conclusions
In this section, we discuss the key findings, contributions and suggestion by domain experts. This section is further divided as follows,
Domain Expert Analysis
Summary and Future Work
6.1 Domain expert analysis
6.1.1 Translation errors
As mentioned earlier in Section 5.2, one of our domain expert annotators is a native Italian speaker identified a small number of translation errors. Of the 130 sentences assessed by them, no critical translation errors were found; however, 11 errors were classified as incorrect word choices which results in misinterpreted translations. For example, with resepct to the translation of the source Italian sentence
‘Quasi quasi verrei lì da te e te li farei fare tutti i nostri compiti in più crepa’
to English as:
‘I almost would come to you and I would make you do all our extra homework. Die.’
Our native italian domain expert notes that the Italian expression ‘quasi quasi’ is very idiomatic and hard to translate into English. Although a direct translation for the expression was provided by the human translator, it probably does not sound correct in English and should have been translated as ‘I would be tempted to come to you
$\ldots$
’. Futhermore, another translation error was identified for the English translation of the Italian word ‘infatti’ as ‘in-fact’, the native domain expert suggests the correct translation should have been ‘indeed’.
6.1.2 Labelling disagreement with original annotators
On reviewing the annotations and comments by the domain expert annotators, we found a few sentences of the sample set, where there were disagreements with original annotators. For example, the domain experts disagreed with original labels for the English translation ‘I offend whoever I want’ of the Italian sentence ‘Io offendo chi voglio’. The original annotators did not categorize this sentence for any form of cyberbullying (harassment, offensive, abusive, etc), whereas the domain experts suggest though it is tricky to label this sentence, as the person is boasting of being able to offend whoever they want, it should be identified as a marker for harassment. The original annotators labelled the Italian sentence ‘Con i compiti che ci ha dato la prof mi ci pulisco il c. . O’ as non-related to bullying, the domain experts disagree, as the correct English translation ‘With the homework the teacher gave us I wipe my a. . O’ shows the intent of the message being related to harassment. The domain experts suggest that though both, the original Italian sentence ‘beh per prima cosa (se non vuoi che tuttti ti odiano) vai da tua madre e convincila a ritornare dalle prof (per dirgli che cosí sono troppi i compiti’ and the English translation ‘well first thing (if you do not want that everyone hates you) go to your mother and convince her to go back to the teachers (to tell them that we have too much homework to do)’ is not offensive on its own. However on closer examination of the surrounding context, we can see that the author of the message is trying to push the victim into a certain behaviour which can be viewed as harassment.
6.1.3 Sarcasm not identified by original annotators
Emmery et al. (Reference Emmery, Verhoeven, De Pauw, Jacobs, Van Hee, Lefever, Desmet, Hoste and Daelemans2021) in their study on data scarcity in cyberbullying have identified that human labelling of datasets might face issues of ambiguity and sarcasm, which are difficult to assess when messages are taken out of context. During the annotation review by the two domain experts, such ambiguity due to sarcasm in sentences was identified as the key reason for disagreement between the original annotations and domain expert annotations. For example, the English translation for the Italian sentence ‘Parla lei’ was ‘She is talking’, the native domain expert suggests that though this is tricky, the translation should reflect the contextual sarcastic meaning of the Italian phrase and should be translated as ‘look who’s talking’ or ‘coming from her’, as it is implied that if something ‘comes from her’ has no value. Another Italian sentence, ‘No carino quello il tuo lavoro se non inizi a studiare un p’ translated as ‘That’s your job, dear, if you don’t start studying’ in English by human translators, the Italian word for ‘dear’, ‘carino’ in this sentence is interpreted as being sarcastic and therefore is considered as offensive, as opposed to original annotation identifying it as non-bullying-related. The English translation ‘And think about it, miss beautiful, it’s useless to keep up with him, you too have more homework to do’ for the Italian sentence ‘E pensaci sì signorina bella guarda che è inutile che li stai dietro anche tu ai i compiti in più da fare’ has the phrase ‘miss beautiful’, which according to the domain experts is sarcastic and can be regarded as offensive. Another Italian sentence ‘magari scoprite che vi piace leggere e studiare’, marked as non-bullying-related by original annotators, translated correctly to English ‘Maybe you discover that you like reading and studying.’ by human translators, according to domain experts in context of the scenario sounds sarcastic and can be considered as offensive.
6.2 Limitations
The study involves many intersecting subject areas that is, social science, bullying NLP, DL and MT. However, there are some limitations in the experiment with respect to each subject area. In this section, we will shed light on each of these challenges and our attempts to mitigate them to the best of our ability.
One of the limitations on leveraging translation to generate a new dataset is the ability to capture the meaning behind idiomatic sentences, which are culture-dependent and may or may not be offensive depending on specific cultures. We believe that by working in a multilingual team with expertise in the cyberbullying domain, this challenge has been reduced significantly.
Though the experiment with BERT shows that a contextual DL model can make very similar classifications for both HT and MT translation outputs, the models trained on HT English text perform better than MT English text (F1 is greater by 0.07). Additionally, the BLEU ratings in the study are low, indicating that MT is far from providing the same level of quality as HT.
Another limitation is that the study relies on using ML/DL techniques with text and metadata from a relatively small dataset drawn from a single social media platform.
Also, it can be very difficult for any AI system to capture fully all of the definitional criteria of cyberbullying (Patchin & Hinduja Reference Patchin and Hinduja2015); however, some of the posts in this dataset still exhibit many of these criteria in both the original source and in the translated texts.
6.3 Summary and future work
As many computational studies like Emmery et al. (Reference Emmery, Verhoeven, De Pauw, Jacobs, Van Hee, Lefever, Desmet, Hoste and Daelemans2021), Salawu et al. (Reference Salawu, He and Lumsden2017) and others have addressed the scarce and sparse nature of the publicly available cyberbullying datasets, our both human and machine translations into English of a rare fine-grained dataset in Italian for the pre-adolescent cyberbullying are extremely novel. To validate both the translated English corpus, we not only reproduce the binary hate classifier proposed by Corazza et al. (Reference Corazza, Menini, Cabrio, Tonelli and Villata2019) (original corpus researchers) accurately but we also leverage state-of-the-art Transformers (BERT) as well as other and other ML and DL algorithms for developing validating native English classifier for the cyberbullying domain. The results for the binary offensive/non-offensive classification experiment discussed in Section 5.4 show that the classification of the MT English corpus is at par with the classification of the human-translated English output.
Motivated by this inter-disciplinary research work, the future scope of this study from different perspectives is as follows,
Machine translation perspective: One direction is to try to build MT systems using more data, both in-domain and out-of-domain data. Furthermore, other domain adaptation methods, (Imankulova et al., Reference Imankulova, Dabre, Fujita and Imamura2019 ; Pham, Crego, and Yvon, Reference Pham, Crego and Yvon2021), are possible which can be investigated in future work.
Domain expert perspective: Cyberbullying in pre-adolescent discourse despite recent computational advances is still quite under-researched. Scrutinizing other OSN discourse platforms to create more resources for cyberbullying detection that can aid in creating plausible OSN monitoring and intervention policies can be a significant contribution to this domain.
ML/DL classification perspective: Due to the sparse and scarce nature of the publicly available cyberbullying resources, recent advances in DL have been focused only on the binary classification or detection of cyberbullying. Fine-grained role-based or entity-based cyberbullying detection and classification is however quite under-researched. Through this research, we realize that there is significant progress yet to be made in this discipline of cyberbullying and engineering both bullying-related corpus and an OSN platform-agnostic cyberbullying detection system.
Acknowledgements
We thank the authors Sprugnoli et al. (Reference Sprugnoli, Menini, Tonelli, Oncini and Piras2018) and Corazza et al (Reference Corazza, Menini, Cabrio, Tonelli and Villata2019) for making the code and data repositoryFootnote ag reproducible and freely available.
The research conducted in this publication was funded by the Irish Research Council and Google, Ireland, under grant number EPSPG/2021/161, Facebook/Meta Content Policy Award, Phase 2: Co-designing with children: A rights-based approach to fighting bullying. This research has also received funding from the European Union’s Horizon 2020 research and innovation programme under the Marie Skłodowska-Curie grant agreement No. 801522, by Science Foundation Ireland and co-funded by the European Regional Development Fund through the ADAPT Centre for Digital Content Technology grant number 13/RC/2106_P2.
Competing interests
The authors declare none.
Supplementary materials
To view supplementary material for this article, please visit http://doi.org/10.1017/S1351324922000341.