1. Introduction
Argument mining (AM) is an emerging area in computational linguistics that aims to explain how argumentative discourse units (e.g. sentences, clauses) function in the discourse and relate to each other, forming an argument as a whole (Lippi and Torroni Reference Lippi and Torroni2016). Argumentative structure is particularly useful for computational models of argument and reasoning engines. AM has broad applications in various areas, such as in the legal domain (Ashley Reference Ashley1990; Yamada, Teufel, and Tokunaga Reference Yamada, Teufel and Tokunaga2019), in news (Al-Khatib et al. Reference Al-Khatib, Wachsmuth, Kiesel, Hagen and Stein2016), and in education (Stab and Gurevych Reference Stab and Gurevych2014; Wachsmuth, Al-Khatib, and Stein Reference Wachsmuth, Al-Khatib and Stein2016; Cullen et al. Reference Cullen, Fan, van der Brugge and Elga2018).
It is common in AM to use well-written texts by proficient authors, as do Ashley (Reference Ashley1990), Peldszus and Stede (Reference Peldszus and Stede2016) and Al-Khatib et al. (Reference Al-Khatib, Wachsmuth, Kiesel, Hagen and Stein2016) among others. However, there are more non-native speakers than native speakers of English in the world (Fujiwara Reference Fujiwara2018), yet there is no specific prior study in AM focusing on non-native texts. It is well known that texts written by non-native speakers suffer from many textual problems, especially in education area, where language learners are still learning how to write effectively. It has been observed that student texts often require improvement at the discourse level, where persuasiveness and content organization are concerned (Bamberg Reference Bamberg1983; Silva Reference Silva1993; Garing Reference Garing2014; Zhang and Litman Reference Zhang and Litman2015; Carlile et al. Reference Carlile, Gurrapadi, Ke and Ng2018). Texts written by non-native speakers are also less coherent and less lexically rich, and exhibit less natural lexical choices and collocations (Kaplan Reference Kaplan1966; Johns Reference Johns1986; Silva Reference Silva1993; Rabinovich et al. Reference Rabinovich, Nisioi, Ordan and Wintner2016).
In this article, we are interested in the application of AM for non-native speakers of intermediate-level language proficiency. Particularly, we annotate the argumentative structure in English-as-a-foreign-language (EFL) essays written by college students in various Asian countries. The following example shows an argumentative essay written by a Chinese student in response to the promptFootnote a “Smoking should be banned at all the restaurants in the country” (ICNALE (Ishikawa Reference Ishikawa2013, Reference Ishikawa2018) essay “W_CHN_SMK0_275_B2_0_EDIT”; we refer to this essay as “high-quality example”):
$_\mathrm{(S1)}$ It is universally recognized that smoking does much damage to human health and that second-hand smoking causes even more serious effects to the people around smokers.
$_\mathrm{(S2)}$According to the statistics shown in newspapers, about five percent of deaths are related to second-hand smoking.
$_\mathrm{(S3)}$Due to the terrible effects of public smoking, I hold the opinion that smoking should be banned in any public restaurants across the country.
$_\mathrm{(S4)}$By doing so, one of the most important favorable effects is that diseases related to smoking, such as lung cancer, can be cut down significantly.
$_\mathrm{(S5)}$The ban contributes a lot to the creation of a healthy dining environment for people who frequently eat outside, which takes up a large proportion of the whole population.
$_\mathrm{(S6)}$Second, prohibiting smoking in some public areas contributes greatly to the development of social culture and ideology.
$_\mathrm{(S7)}$Like drunken driving, which poses threats to citizens’ safety, smoking in public does harm to others’ health.
$_\mathrm{(S8)}$Such behavior is against our goal of establishing a harmonious society.
$_\mathrm{(S9)}$In addition, the forceful act of a complete ban raises the awareness of the harm of smoking among the general public.
$_\mathrm{(S10)}$More and more smokers will gradually get rid of this bad habit for the interest of their own health.
$_\mathrm{(S11)}$To conclude, it is high time for us to take strong measures to put an end to this smoking era.
$_\mathrm{(S12)}$A complete change to the legal system regarding the smoking issue is necessary for the final settlement of this social problem.
Successful argumentative essays such as this example typically introduce the discussion topic (here, S1–S2), state their stance on the topic (S3), support their stance by presenting reasons from various perspectives (S4–S10), and then provide a conclusion (S11) (Silva Reference Silva1993; Bacha Reference Bacha2010). The author of the above example was at upper-intermediate to advanced proficiency and had a TOEFL iBT Score of 98. However, not all EFL students posses the skill to write at this level.
Consider the following essay, which was written in response to the prompt “It is important for college students to have a part-time job”, by an Indonesian student with lower-intermediate to intermediate proficiency (ICNALE essay “W_IDN_PTJ0_050_A2_0_EDIT”; we refer to this essay as “intermediate-quality example”):
$_\mathrm{(S1)}$The costs students incur on campus are not small; every month can cost up to a million for meals, transportation, books, and cigarettes for smokers.
$_\mathrm{(S2)}$The income of a parent who is an entrepreneur can sometimes cover this amount, but other parents need more than one income.
$_\mathrm{(S3)}$Every student wants to cover the cost when they live far away from their parents.
$_\mathrm{(S4)}$Some students who have many necessary payments on campus need to look for money by themselves, so they usually work at a cafe, do car washing, work as a newspaper agent, or work at an Internet rental shop.
$_\mathrm{(S5)}$But sometimes, they have problems dividing their time, and they sometimes ignore their assignments from college.
$_\mathrm{(S6)}$But, they feel proud they can complete part of their costs of college without asking their parents.
$_\mathrm{(S7)}$If all students do this, surely all parents would feel proud but they must not complete all of the necessary things.
$_\mathrm{(S8)}$Thus, if sometimes the parents’ income is not enough to pay the campus costs, we have to get money by ourselves to cover everything from books to the boarding house without asking our parents.
$_\mathrm{(S9)}$In my opinion, a part-time job helps students support their financial problems and I agree that students should work part time.
In this study, we work on essays of intermediate quality, such as this second example; this essay differs from the high-quality example above in at least two respects. First, the intermediate-quality example does not adhere to the typical English argumentation development strategy. For instance, the discussion topic is not introduced, and the stance (underlined) is given at the end of the essay, rather than at the beginning. This contrasts with a more straightforward structure in the high-quality example, which presented the stance right at the beginning. Second, the intermediate-quality example presents the argument only from a single viewpoint (arguing in favor of part-time jobs for financial reasons), whereas the high-quality example considers another as well (arguing in favor of banning smoking for health and cultural reasons). We can observe that due to the poorer structure of essays written by intermediate-level writers, they are likely to pose more challenges to any automatic treatment.
Our long-term goal is to help EFL students improve their essays to the native level, and we see creating an annotated EFL corpus as the first step towards building an automatic AM system for better EFL education. The traditional use of an annotated corpus is to train a supervised machine learning system, but in the EFL context, such a corpus in and of itself can already support the theoretical and practical teaching of how to argue. Kaplan (Reference Kaplan1966) introduced a teaching strategy based on contrastive rhetoric, where the idea is to show EFL students the differences between the structures of their writings and native (and thus presumably “good”) writings. Our corpus can be used for theoretical studies in contrastive rhetoric, and it can also be used practically in the classroom today. This should prove particularly effective if combined with visualization of the structure (Cullen et al. Reference Cullen, Fan, van der Brugge and Elga2018; Matsumura and Sakamoto Reference Matsumura and Sakamoto2021).
The main contributions of this article are twofold:
• We introduce an annotation scheme for argumentative discourse structure of EFL essays and an associated corpus called ICNALE-AS.Footnote b This corpus contains 434 annotated essays written by Asian learners and is publicly available.Footnote c Inter-annotator and intra-annotator agreement studies were conducted that showed a reasonable level of agreement considering the difficulty of the task. To the best of our knowledge, this is the first corpus of EFL texts annotated with argument structure. The use of EFL texts differentiates our study from most existing AM studies which employ well-structured coherent texts written by proficient authors.
-
• We present several structure-based metrics for the calculation and better interpretation of inter-annotator agreement for argument structure analysis, including a meta-evaluation via crowd-sourcing, which quantifies the reliability of these proposed metrics in comparison to existing ones.
The rest of this article is organized as follows. Section 2 gives an overview of related work. Our annotation scheme is then introduced in Section 3. Section 4 explains the shortcomings of traditional inter-annotator agreement metrics in the context of discourse structure analysis. We propose and meta-evaluate several structure-based inter-annotator agreement metrics. Section 5 describes the corpus resulting from our annotation effort. Finally, Section 6 concludes this article.
2. Related work
This section gives an overview of related work, including argumentative discourse structure analysis, the connection between argumentative structure and text quality, the role of such structural analysis in teaching, and a description of existing corpora annotated with argumentative structure.
2.1 Discourse structure and argument mining
Discourse theories aim to explain how individual discourse units (e.g. sentences or clauses) relate to each other and what roles they play in the overall discourse (Grosz and Sidner Reference Grosz and Sidner1986; Mann and Thompson Reference Mann and Thompson1988; Wolf and Gibson Reference Wolf and Gibson2005; Prasad et al. Reference Prasad, Dinesh, Lee, Miltsakaki, Robaldo, Joshi and Webber2008). The automatic recognition of discourse structure is attractive as it benefits various downstream tasks, for example, text assessment (Feng et al. Reference Feng, Lin and Hirst2014; Wachsmuth et al. Reference Wachsmuth, Al-Khatib and Stein2016), text generation (Hovy Reference Hovy1991; Yanase et al. Reference Yanase, Miyoshi, Yanai, Sato, Iwayama, Niwa, Reisert and Inui2015; Al-Khatib et al. Reference Al-Khatib, Wachsmuth, Hagen and Stein2017), and summarization (Teufel and Moens Reference Teufel and Moens2002).
Different types of discourse structure have been proposed over the years (Webber, Egg, and Kordoni Reference Webber, Egg and Kordoni2012). Rhetorical Structure Theory (RST) modeled the relations between adjacent discourse units, which form a tree (Mann and Thompson Reference Mann and Thompson1988). The Penn Discourse Treebank project (Prasad et al. Reference Prasad, Dinesh, Lee, Miltsakaki, Robaldo, Joshi and Webber2008) analyzed local discourse relations and the discourse markers that signal the relations. Wolf and Gibson (Reference Wolf and Gibson2005) observed that texts often contain various kinds of crossed dependencies between sentences as well as nodes with multiple parents. As a result, they modeled text as a graph. In contrast, Hearst (Reference Hearst1997) segmented text into a linear sequence of thematically coherent topics.
While the theories mentioned above are designed to be general across genres, discourse structure analysis is also often tailored to the target text genre and the research goal. Since we are trying to analyze argumentative essays written by EFL students, we approach the discourse structure analysis from the argumentation perspective.
Traditionally, the annotation of argumentative discourse structure consists of two main steps (Lippi and Torroni Reference Lippi and Torroni2016). The first is argumentative component identification, which determines the boundaries of discourse units. The units are then differentiated into argumentative and non-argumentative components. Argumentative components (ACs) function to persuade readers, while non-argumentative components (non-ACs) do not (Habernal, Eckle-Kohler, and Gurevych Reference Habernal, Eckle-Kohler and Gurevych2014). Non-ACs are often excluded from further processing because they do not contribute to the argumentative structure. ACs can be further classified according to their roles in argumentation, for example, claim and premise (Peldszus and Stede Reference Peldszus and Stede2013; Habernal et al. Reference Habernal, Eckle-Kohler and Gurevych2014). These roles can be extended according to the application context. For example, Stab and Gurevych (Reference Stab and Gurevych2014) used major claim, claim, and premise for persuasive essays, whereas Al-Khatib et al. (Reference Al-Khatib, Wachsmuth, Kiesel, Hagen and Stein2016), working on news articles, differentiated between common ground, assumption, testimony, statistics, anecdote, and other.
The second step is argumentative discourse structure prediction. This step establishes labeled links from source to target ACs to form the text structure, which can be a tree (Stab and Gurevych Reference Stab and Gurevych2014) or a graph (Sonntag and Stede Reference Sonntag and Stede2014; Kirschner, Eckle-Kohler, and Gurevych Reference Kirschner, Eckle-Kohler and Gurevych2015). Typically, all ACs must be connected to the structure, while all non-ACs remain unconnected. Links (also called edges) can be directed (Stab and Gurevych Reference Stab and Gurevych2014) or undirected (Kirschner et al. Reference Kirschner, Eckle-Kohler and Gurevych2015). The links are then labeled according to the relationship between the source and target ACs, for example, using the labels support and attack (Stab and Gurevych Reference Stab and Gurevych2014). Similar to the variations in AC labels, previous studies in AM have also tailored relation labels to specific research goals and needs. For example, Kirschner et al. (Reference Kirschner, Eckle-Kohler and Gurevych2015) proposed the detail relation that roughly corresponds to the elaboration and background relations in RST (Mann and Thompson Reference Mann and Thompson1988). Skeppstedt, Peldszus, and Stede (Reference Skeppstedt, Peldszus and Stede2018) observed another frequent relation, namely restatement, which applies in those cases when an important part of the argument, such as a major claim, is repeated and summarized in strategically important places, such as at the end of the essay.
2.2 Argumentative structure and text quality
Writing coherent argumentative texts requires reasoning and effective framing of our opinions. A coherent argumentative text has to contain the desired argumentative elements; ideas should be clearly stated, connected to each other, and supported by reasons. The ideas should also be logically developed in a particular sequencing, such as by time or importance, and accompanied by appropriate discourse markers. Only then can the writing ultimately communicate the desired ideas as a whole (Silva Reference Silva1993; Reed and Wells Reference Reed and Wells2007; Bacha Reference Bacha2010; Blair Reference Blair2012; Peldszus and Stede Reference Peldszus and Stede2013; Wachsmuth et al. Reference Wachsmuth, Naderi, Hou, Bilu, Prabhakaran, Thijm, Hirst and Stein2017).
The idea that there is a close connection between argumentative structure (and discourse structure in general) and text quality has been applied in text assessment studies. Persing, Davis, and Ng (Reference Persing, Davis and Ng2010) provided an automatic organization score based on the patterns of rhetorical-category transitions between sentences. Wachsmuth et al. (Reference Wachsmuth, Al-Khatib and Stein2016) also used a similar strategy when scoring various aspects of argumentation. Discourse structure also correlates with text coherence, and various coherence models have been developed that rely on this interaction. For example, Lin, Ng, and Kan (Reference Lin, Ng and Kan2011) and Feng et al. (Reference Feng, Lin and Hirst2014) measured text coherence based on discourse relation transition bigrams.
It has been argued that discourse structure forms a plan to order sentences (Hovy Reference Hovy1991). Hence, many natural language generation studies attempted to produce coherent and persuasive texts by following certain discourse patterns. Yanase et al. (Reference Yanase, Miyoshi, Yanai, Sato, Iwayama, Niwa, Reisert and Inui2015), for instance, ordered sentences in debate texts using a “claim-support” structure. In the claim–support structure, the first sentence describes an opinion, which is followed by support sentences expressing reasons for the opinion. On the other hand, Al-Khatib et al. (Reference Al-Khatib, Wachsmuth, Hagen and Stein2017), working on news editorial texts, assumed that a persuasive argument can be built based on fixed argumentation strategies; they identified several such argumentation strategies in the form of common patterns of N-grams over component types. In another NLG approach, El Baff et al. (Reference El Baff, Wachsmuth, Al Khatib, Stede and Stein2019) pooled text pieces from many different texts and then generated text as a slot-filling process. Their system proceeded by selecting one discourse unit after the other from the pool if it satisfied the rhetorical function needed in the template. In the final output, only a small proportion of all available sentences were used.
2.3 The role of argumentative structure analysis in teaching
Many existing studies have attempted to correct spelling and grammatical errors (e.g. Hirst and Budanitsky Reference Hirst and Budanitsky2005; Han, Chodorow, and Leacock Reference Han, Chodorow and Leacock2006; Yuan and Briscoe Reference Yuan and Briscoe2016; Fujiwara Reference Fujiwara2018), but studies at the discourse and argumentation level are still limited. Teaching students how to argue effectively can be difficult, particularly if the medium of expression is not their first language (Silva Reference Silva1993; Bacha Reference Bacha2010). Cullen et al. (Reference Cullen, Fan, van der Brugge and Elga2018) showed how teaching to argue can be supported by annotating the implicit argumentative structure. They performed a controlled study where one group of students were taught to annotate argumentative structure in a visual manner, whereas the control group was taught traditionally, that is, through written or verbal explanation. When measuring the improvement of both groups in a logical reasoning test before and after the teaching sessions, they found a larger increase in the visually-taught group than in the control group, suggesting that learning to annotate arguments led to improvements in students’ analytical-reasoning skills.
The analysis of argumentative structures enables writers to check completeness (are all necessary parts there?) and coherence (do relations among parts make sense?) (Bobek and Tversky Reference Bobek and Tversky2016). Such analysis also facilitate discussions between students and instructors about text structure because students can share their interpretations through the annotated structure. This allows instructors to quickly identify gaps in students’ understanding of the learning material and then provide relevant feedback to the students (Cullen et al. Reference Cullen, Fan, van der Brugge and Elga2018). For example, instructors may check whether an argument is balanced and contains the necessary material (Matsumura and Sakamoto Reference Matsumura and Sakamoto2021) or, if not, encourage a student to find new relevant material and to incorporate it into the essay. We are more interested in a situation where the necessary material has already been provided by the student, but it is possibly in a sub-optimal order. Rather than organizing a text from scratch, we are therefore interested in reorganization of sentences in the text, an aspects which EFL students often struggle with.
Studies in contrastive rhetoric investigate how students’ first language might influence their writings in the second language. Many studies found that non-native speakers tend to structure and organize their texts differently from native speakers (Kaplan Reference Kaplan1966; Johns Reference Johns1986; Silva Reference Silva1993; Connor Reference Connor2002). If EFL students use the customs, reasoning patterns and rhetorical strategies of their first language when writing in the second language, there is a danger that the different organization of ideas can violate the cultural expectations of native speakers (Kaplan Reference Kaplan1966). For example, in writings by Asian students, it is sometimes observed that reasons for a claim are presented before the claim, which is not common in Anglo-Saxon cultures (Silva Reference Silva1993). This can result in a situation where writings of Asian students may appear less coherent in the eyes of native readers. The instructional approaches for argumentation strategies also vary among cultures. For example, Liu (Reference Liu2005) found that American instructional approaches encourage the consideration of opposing ideas, while the Chinese approaches describe the importance of analogies, and epistemological and dialogical emphases. Therefore, studies argued that EFL students need specific instructions to account for cultural differences in L1 and L2 (Kaplan Reference Kaplan1966; Silva Reference Silva1993; Connor Reference Connor2002; Bacha Reference Bacha2010). Argumentative structure analysis helps EFL students to understand and bridge the cultural gaps between writing strategies in their native languages and English, but no AM study before us has provided support for this specific task.
2.4 Existing corpora annotated with argumentative structure
There exist corpora covering various aspects of argumentation analysis, for instance, argument strength (Persing and Ng Reference Persing and Ng2015), type of reasoning (Reed et al. Reference Reed, Palau, Rowe and Moens2008), and argumentative relations (Kirschner et al. Reference Kirschner, Eckle-Kohler and Gurevych2015). Considering our target domain, the most relevant corpora for the current work are the microtext corpus by Peldszus and Stede (Reference Peldszus and Stede2016) and the persuasive essay corpus Footnote d by Stab and Gurevych (Reference Stab and Gurevych2014, Reference Stab and Gurevych2017).
The microtext corpus is a collection of 112 short texts that were written in response to various prompts. The texts contain roughly five ACs per text with no non-ACs present. Each text is centered around a single major claim, while other ACs act as proponent (defending the major claim) or opponent (questioning the major claim). All components form a single tree structure, whereby the links can be of three types: support, rebuttal, and undercut. The texts in the original study were written in German and then translated into English, but in a follow-up study (Skeppstedt et al. Reference Skeppstedt, Peldszus and Stede2018), crowd workers were employed to write in English. Efforts were made to create argumentation of the highest possible quality; texts with possible lower-quality argumentation were removed.
With their average length of 18 sentences, the 402 texts in the persuasive essay corpus are longer than those in the microtext corpus. They contain both ACs and non-ACs, on average, 15 ACs and 3 non-ACs. The texts, which are written in English, were randomly collected from essayforum.com, an online forum where students can receive feedback on their writing. ACs are subdivided into major claim, claim, and premise, with link types support and attack, forming a tree in which the major claim acts as the root (level-0). Supporting or attacking claims, which are marked as such, then follow in level-1, which in turn is followed by premises at an even deeper level ($\geq2$). This means that the discourse function is doubly marked in this scheme: by the level of an AC in the hierarchy and by an explicit labeling of ACs.
Neither of these corpora is appropriate for our task. The authors of the microtext corpus were assumed to be fully competent in writing argumentative texts or the texts were filtered so that only high-quality texts remain. Additionally, the persuasive essay corpus is problematic for our research purpose because it does not distinguish between native and non-native speakers and gives no information about the (assumed or observed) quality of the essays. In our study, we specifically target intermediate-level non-native speakers. To this end, we strategically sample our target essays from an Asian EFL essay corpus, namely ICNALE, on the basis of ratings by professional ICNALE assessors.
3. Discourse model for EFL essay
We now turn our attention to the annotation scheme we developed for the ICNALE-AS corpus.
3.1 Target domain
Our target texts are sourced from ICNALEFootnote e (Ishikawa Reference Ishikawa2013, Reference Ishikawa2018), a corpus of 5600 argumentative essays written in English by Asian college students. The vast majority of these are written by non-native English speakers, although 7.1% of the essays are written by Singaporeans, for whom English is typically the first language. ICNALE essays contain 200–300 words and are written in response to two prompts: (1) “It is important for college students to have a part-time job” and (2) “Smoking should be completely banned at all the restaurants in the country.” Note that the students are asked to write their essays in a stand-alone fashion, that is, under the assumption that the prompt is not deemed as part of the essay and therefore not read together with it.
Following Skeppstedt et al. (Reference Skeppstedt, Peldszus and Stede2018), an important aspect of our work is that we treat a student’s argumentation skills as separate from their lexical and grammatical skills. There is a subset of 640 essays in ICNALE that have been corrected in terms of grammatical and “mechanical”Footnote f aspects, and which we can take as the starting point for this study.
From this subset, we exclude low-quality essays, those with extremely poor structure or so little content that they are hard to interpret. We use the preexisting scoring system in the 640-subset to this end. Essays are scored with respect to five aspects, namely content, organization, vocabulary, language use, and mechanics; the five scores are then combined into a total score in the range of [0,100].Footnote g We manually investigated the quality of randomly sampled essays to check the total score at which the quality drops to a point where it is hard to understand what the students want to convey. We identified that point as a score of 40 points, affecting 4.1% of all essays. Essays scoring below this point would require a major rewrite before they could be analyzed.
At the other end of the spectrum, we also exclude essays that are of very high quality. The annotation of such already well-written essays would be of limited use towards our long-term goal of improving the writing of EFL students who have not yet reached this level. We found that essays scoring 80 points or more (15.2% of the total) are already well written and coherent. Of course, it might be possible to improve their quality and persuasiveness even further, but we believe they are comparable with essays written by advanced or proficient writers. The remaining 517 essays scoring between 40 and 80 points (80.8% of the total) should therefore be what we consider intermediate-quality essays. We had to manually discard a further 63 essays for the reason that they contained a personal episode related to the prompt instead of a generalized argument or they lacked a clear argumentative backbone for some other reason. While the 454 surviving texts are sometimes still far from perfect, they are quite clear in almost all cases in terms of what the author wanted to say. These essays also contain a plan for an argument that is at least roughly acceptable, as well as the right material for the plan.
The average length of the texts in our corpus is 13.9 sentences. We used 20 essays for a pilot study not reported here,Footnote h which left us with 434 essays; these constitute the pool of essays we use in this article (hereafter referred to as “ICNALE essays” or “ICNALE corpus”).
3.2 Annotation of argumentative structure
Following common practice in AM (cf. Section 2), our annotation consists of two steps. The first is argumentative component identification, where we identify sentences as ACs and non-ACs. The second step is argumentative structure prediction, where we identify relations between ACs. These relations then form a hierarchical structure. For other genres, such as scientific papers (Kirschner et al. Reference Kirschner, Eckle-Kohler and Gurevych2015) and user comments (Park and Cardie Reference Park and Cardie2018), annotation schemes are sometimes based on graphs rather than trees. For our argumentative essays, however, we observed that a simple tree structure suffices in the overwhelming number of cases and that it most naturally expresses the predominant relation where a single higher-level statement is recursively attacked or supported by one or more lower-level statements (Carlile et al. Reference Carlile, Gurrapadi, Ke and Ng2018).
In a departure from existing work, where the textual units of analysis are represented at the clause level, the units (ACs and non-ACs) in our scheme are always full sentences. Textual units smaller than sentences but bigger than words, such as clauses, are hard to define in a logical and linguistically clear manner suitable for annotation. Despite many attempts in the literature (e.g. Fries Reference Fries and Coulthard1994; Leffa and Cunha Reference Leffa and Cunha1998; Huddleston and Pullum Reference Huddleston and Pullum2002), there is still no easily applicable annotation instruction for capturing meaningful argumentation units at the sub-sentential level. In practice, annotation studies often use an idiosyncratic definition of which textual units constitute an argumentative component (Lippi and Torroni Reference Lippi and Torroni2016), resulting in a lack of interoperability between annotation schemes. While we acknowledge that our use of sentences in this article is a theoretical simplification, it is well-motivated from the computational perspective. In fact, existing works in AM also operate at the sentence level, for example, Teufel, Carletta, and Moens (Reference Teufel, Carletta and Moens1999), Carstens and Toni (Reference Carstens and Toni2015), Kirschner et al. (Reference Kirschner, Eckle-Kohler and Gurevych2015), Wachsmuth et al. (Reference Wachsmuth, Al-Khatib and Stein2016). When defining units, we certainly cannot go beyond the sentence level toward larger units. Students may have added paragraph breaks, but these are not recorded in the ICNALE corpus. In any case, paragraphs would certainly be too large as atomic units given that the ICNALE essays only have an average length of 13.9 sentences.
In our scheme, as in that by Stab and Gurevych (Reference Stab and Gurevych2017), the major claim is topologically distinguished as the root of the tree structure, which is recognizable as the only node with incoming but no outgoing links. In contrast to their scheme, however, we do not additionally label ACs as major claim, claim, and premise. We decide not to do so to avoid conflicts that might arise in long argumentation chains, particularly between claims and premises. A premise at level X can easily itself become the claim for a lower-level premise at level $X+1$, making the AC act as both claim and premise at the same time. With a finite number of labels, this means that none of the fixed labels is applicable. We note that such ambiguous cases do happen in Stab and Gurevych’s persuasive essay corpus; these cases were resolved according to topology, a treatment that is consistent with our decision not to label ACs in the first place. We feel that omitting AC labels makes our annotation scheme not only more economical but also intrinsically consistent.
3.2.1 Non-argumentative material
In this study, we mark discourse units as ACs and non-ACs. Traditionally, non-ACs refer to units that do not function argumentatively. In another departure from existing work, we use a more fine-grained model of non-ACs, as follows:
(a) Disconnected sentences. We exclude isolated sentences, that is, those that do not function argumentatively and thus are not connected to the logical argument. Such sentences might convey an opinion about the prompt statement, for example, “this is a good question to discuss.”, or a personal episode regarding the prompt.
-
(b) Meta-information. We exclude sentences which make statements about other sentences without any new semantic content because such sentences contribute nothing substantial toward the argument. An example is “I will explain my reasons.”
-
(c) Redundant material. We also exclude repetitions of low-level argumentative material such as facts. For instance, “a barista has to interact with lots of people.” might be repeated as “baristas have much contact with customers.” In our scheme, one of these sentences (most often the second one) would be marked as non-AC.
3.2.2 Directed relation labels
We use three directed relation labels: support (sup), detail (det), and attack (att). In our scheme, these relations are defined as going from child node (here also called source sentence) to parent node (target sentence).
Support is a commonly used relation label in AM. Here, the source sentence asserts the reasons why readers of an essay should believe the content of the target sentence. This is done by providing argumentative material in support of the target, such as supporting evidence, and this material should be new to the argument. Attack is another commonly used relation label, denoting a source sentence that argues for the opposite opinion of the target sentence.
The detail label is less common, but there is precedent for it in the work of Kirschner et al. (Reference Kirschner, Eckle-Kohler and Gurevych2015). In our scheme, it is applied if the source sentence does not provide any new argumentative material. This typically happens in two cases: (1) when the source sentence presents additional detail, that is, further explanation, examples, description or elaboration of the target sentence or (2) when the source sentence introduces the topic of the discussion in a neutral way by providing general background. Thus, it is the presence or absence of new argumentative material that differentiates the labels detail and support. There is an interesting distinction between detail and support when it comes to the ordering of sentences. The canonical ordering in a support relation places the target sentence before the source sentence (Kaplan Reference Kaplan1966; Silva Reference Silva1993; Bacha Reference Bacha2010; Yanase et al. Reference Yanase, Miyoshi, Yanai, Sato, Iwayama, Niwa, Reisert and Inui2015). Things are a little more nuanced with detail. When a source sentence in the detail relation appears before its target, we tend to regard it as background information, while we tend to regard it as a further elaboration if it appears after the target sentence.
3.2.3 Restatement
We noticed that in many cases, the major claim is restated in the conclusion section of an essay, summing up the entire argument. Skeppstedt et al. (Reference Skeppstedt, Peldszus and Stede2018) also noticed this and coined the name restatement to model this phenomenon. In our scheme, the Restatement relation holds between two sentences if the second one repeats high-level argument material that has been previously described by the first, without adding a new idea into the discourse. Restatements repeat key argumentative material at a high level in the argument (claims or main claims, not premises or mere facts), and they do so at strategic points in the linear text. This can reinforce the persuasiveness of the overall argument.
Here, we distinguish redundant material (Section 3.2.1) from restatements, which we consider ACs although they do contain repeated information—the difference is that in the case of a restatement, we can assume the repetition is intentional and aimed at affecting the flow of argumentation.
Unlike support, attack, and detail, the restatement relation (which we express by the symbol “ =”) is an equivalence relation and therefore non-directional. Source and target sentences convey the same meaning; they are not in a hierarchical relationship. As a result, we treat the two sentences as an equivalence class with respect to all outgoing and incoming relations they participate in.
In argumentative structure annotation, implicit relations can arise which follow semantically from other annotations even though those relations are not explicitly stated. Restatements introduce one particular kind of such implicit relations. In order to correctly interpret the argument, it can be necessary to also consider the implicit relations.
Figure 1 shows such a situation involving implicit links, where different annotations are compared under restatement closure. Annotation A recognizes a support link between nodes 1 and 2 and an attack link between nodes 3 and 4, whereas Annotation B recognizes the support link between nodes 1 and 4 and the attack link between nodes 3 and 2. The annotations A and B do not share a single one of these explicit links, yet they are identical if we consider implicit restatement-based links. If nodes $\{2,4\}$ are considered as restatement cluster, then both annotations agree that an attack link connects node 3 to restatement cluster
$\{2,4\}$ and a support link connects node 1 to the restatement cluster
$\{2,4\}$, despite the fact that they mark this differently.

Figure 1. Closure over restatement relation. Solid links are explicit, dashed lines implicit. (a) Annotation A. (b) Annotation B.
This new interpretation of the semantics of restatement as an equivalence class is a conscious decision on our part, which necessitates the computation of implicit links by some additional machinery. In argumentation, other implicit links are also theoretically possible,Footnote i but we do not consider them here.
3.3 Annotation procedure and example
Annotators start by dividing the text into its introduction, body, and conclusion sections in their minds,Footnote j and then dividing the body section recursively into sub-arguments. During this process, they also need to identify the major claim.
The idea of sub-arguments is based on the observation that it is common for groups of sentences about the same sub-topic to operate as a unit in argumentation, forming a recursive structure. We instruct our annotators to start the annotation process by marking relations within a sub-argument; later, they analyze how the sub-argument as a whole interacts with the rest of the text. The connection between the sub-argument and the rest of the argument is annotated by choosing a representative sentence standing in for the group.
We now illustrate how our annotation scheme works using a fictional argumentative essay with the prompt “Smoking should be completely banned at all the restaurants in the country.”
$_\mathrm{(S1)}$Government has been trying to introduce laws to ban smoking in restaurants.
$_\mathrm{(S2)}$ I have watched the news.
$_\mathrm{(S3)}$I agree that smoking should be banned at all restaurants.
$_\mathrm{(S4)}$In restaurants, customers enjoy eating and talking.
$_\mathrm{(S5)}$Thus, if somebody smokes in the restaurant, other people may not be able to enjoy their meal.
$_\mathrm{(S6)}$However, if we ban smoking in restaurants, they might lose some customers.
$_\mathrm{(S7)}$But I firmly support banning smoking in restaurants since we need to prioritize health.
$_\mathrm{(S8)}$In conclusion, I encourage banning smoking in all restaurants.
This essay can be divided into several parts. S1–S3 together form the introduction section of the essay. S1 provides a background for the discussion topic, and S3 serves as the major claim of the essay. S2, which describes a personal episode that does not have an argumentative function, is identified as a non-AC, and thus excluded from the argumentative structure.
S4–S5 discuss the topic of enjoyment of eating and talking, with S4 providing the introduction of this idea, and S5 giving an opinion on the topic. Sentence S6 then presents an argument about the number of customers; it supports the opposite opinion of S3. S7 repeats some high-level information that has already been stated before as well as introduce a new health-related argument. Because we cannot assign two relations for S7 as a source sentence, we have to make a choice. Our rule is to always give preference to the new argument; here, this is the material about health. Hence, S7 is marked as attacking S6 (and not as restatement). Finally, S8 concludes the whole argument, by restating the major claim, which this time we can mark as a restatement (expressed by “=”). Figure 2 illustrates the argumentative structure of the essay and shows how it relates to the typical essay development plan.
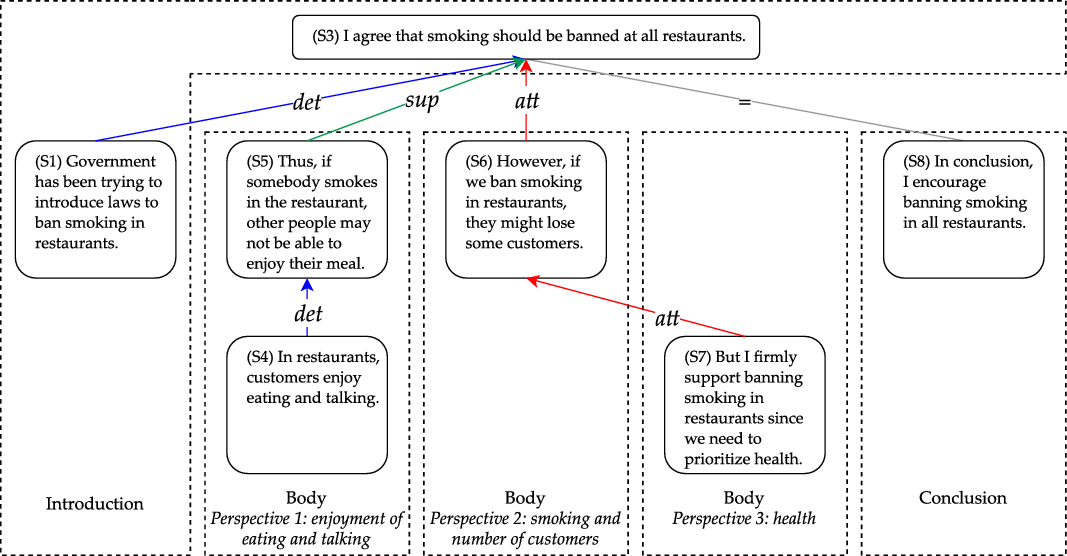
Figure 2. Argumentative discourse structure annotation of example text from page 19.
4. Structure-based agreement metrics
In Section 5, we will perform an agreement study with the newly defined scheme. However, we first need to turn our attention to the question of which agreement metrics would be appropriate for structural annotation scheme such as ours. In addition to the conventional metrics (Section 4.1), we develop new metrics specifically for the study at hand (Section 4.2), and later describe the evaluation of these newly-developed metrics (Section 4.3).
4.1 Conventional agreement metrics
If different annotators produce consistently similar results when working independently, then we can infer that they have internalized a similar understanding of the annotation guidelines, and we can expect them to perform consistently in all similar conditions, in particular with new unseen text. Inter-annotator agreement metrics exist for several types of annotation. Our task here is a categorical classification, where a fixed set of mutually exclusive categories are used and where we assume that the categories are equally distinct from one another (Artstein and Poesio Reference Artstein and Poesio2008). The simplest of these is plain observed agreement (“agreement ratio”). Chance-corrected agreement measures such as Cohen’s $\kappa$ have also been proven to be particularly useful in computational linguistics (Carletta Reference Carletta1996).
In the context of this study, there are three aspects of agreement which can be expressed in terms of categorical classification:
• Argumentative component identification. Each sentence is categorized as either “AC” or “non-AC”.
-
• Existence of links between sentences (“sentence linking”). A binary label (“linked” vs. “not linked”) is assigned to all non-identical sentence pairs in the text (Kirschner et al. Reference Kirschner, Eckle-Kohler and Gurevych2015).
-
• Relation labeling. For all sentence pairs that have been confirmed as being connected by annotators, we measure whether annotators agree on the relation label that holds between them.
We report the agreement of argumentative structure annotation on these three aspects, using agreement ratio and Cohen’s $\kappa$ (Cohen Reference Cohen1960). We also report the agreement ratio for the entire structure (“entire agreement ratio”) to show how errors propagate. The entire agreement ratio measures whether annotators made the same decisions on all aspects of structural annotation for each sentence (as source): the same component category (AC vs. non-AC), the same target sentence and the same relation label. It is analogous to multi-label accuracy.
4.2 Structure-based Inter-annotator agreement metrics
Conventional agreement metrics treat annotated items as independent of each other. However, in argumentative structure and other types of discourse annotation, there are some problems with this assumption. In the sentence linking task, annotation decisions are often structurally dependent on each other; if there is a link from sentence X to sentence Y, other links from sentence X are no longer possible as far as we assume a tree structure. The $\kappa$ metric does not recognize such dependencies and counts non-linked sentence pairs as correct cases, possibly overestimating the true value.
The second problem concerns implicit links. As we have argued in Section 3.2.3, we have to consider implicit links as the result of the semantics of the restatement label. Conventional metrics are not suitable for closure structures because they cannot distinguish between explicit and implicit links; they treat implicit links as if they are explicit. If implicit links in annotation A do not appear in annotation B, they will be treated as mismatches, and conventional metrics will assign a penalty to the score. Therefore, there might be a large difference in agreement scores between a situation in which only explicit links are used and one in which both explicit and implicit links are used, which is undesirable. We also think that the fairest treatment of implicit links is to reward in situations where an implicit link is correct without punishing in situations where the link is incorrect. We will now explain this asymmetry.
Let us consider this point using the two annotations A and B in Figure 3. In Figure 3(a), Annotation A marked an explicit link from nodes 3 to 4, which can be expanded by an implicit link from nodes 3 to 2, cf. Figure 3(c). The fact that the annotators agree that node 3 attacks the restatement cluster $\{2,4\}$ should be rewarded somehow in our opinion.

Figure 3. Example of restatement closures. Solid links are explicit, dashed lines implicit. (a) Annotation A. (b) Annotation B. (c) Closure of A. (d) Closure of B.
Things get more complicated when one annotator links a node into the equivalence cluster when the other annotator links it to a node outside of it. This is illustrated with the links exiting from node 1; A links it to 2 and thus inside the equivalence cluster, whereas B links it to 3 and thus outside the equivalence cluster. It is clear that B should be punished for missing the explicit link 1$\rightarrow$2, which is present in Annotation A. The question is, should B additionally be punished for the lack of the implicit link 1
$\rightarrow$4, which only arose because node 2 happens to be inside the equivalence cluster? We consider this unfair given that from Annotation B’s viewpoint, node 1 is not connected to the equivalence cluster. Without a link to the equivalence cluster, B could not possibly have considered the hypothetical implicit link 1
$\rightarrow$4. Thus, we believe an ideal agreement metric should assign a special treatment to implicit links: (1) to reward implicit links if they match but (2) not to punish when implicit links do not match.
To allow a more holistic view of structural annotation while alleviating the implicit link problem, we propose a new document-level agreement metric based on the notion of recall, that is the degree to which each annotation recalls the other annotation in terms of structure. The total number of units for recall calculation normally differs between annotators; this is so because in the earlier AC versus non-AC classification step, annotators might have classified different sets of sentences as non-ACs. Consequently, we have to average across the two annotations’ recall values and accept that the metric can be defined only for pairs of annotations. We call our new metric mean agreement in recall (MAR). It comes in three variants, which differ in how the units are defined: as links ($\textbf{MAR}^\textbf{link}$), as paths (
$\textbf{MAR}^\textbf{path}$) or as descendant sets (
$\textbf{MAR}^\textbf{dSet}$). The special treatment for implicit links described above is only applicable to
$\mathrm{MAR}^\mathrm{link}$, not to the other variants.
When computing structure-based agreement metrics, we need to operationalize undirected links as directed links; if there is a restatement link between two nodes A and B, we represent this as $A\rightarrow B$ and
$B\rightarrow A$.Footnote k We will now describe the metrics in turn.
4.2.1 Link-based MAR
There are two variants of $\mathrm{MAR}^\mathrm{link}$: (1) considering only explicit links and (2) also considering implicit links. The implicit version (2) rewards implicit links when they appear in another structure but does not punish them when they do not, as described above.
Given two structures A and B with respective sets of explicit links $E_A$ and
$E_B$,
$\mathrm{MAR}^\mathrm{link}$ measures the average recall of links between the two structures as computed in Equation (1). For this metric, relation labels are disregarded. For example,
$\mathrm{MAR}^\mathrm{link}$ between annotation A and B in Figure 3 is
$0.50$.Footnote l

For the closure structures, we modify the formula such that it measures the agreement without giving penalties to implicit links. Given two structures $\mathrm{closure}(A)$ and
$\mathrm{closure}(B)$ with respective sets of link (explicit+implicit)
$EC_A$ and
$EC_B$,
$\mathrm{MAR}^\mathrm{link}$ for closure is calculated as in Equation (2), as the recall of the closure structure w.r.t another explicit structure.

For example, $\mathrm{MAR}^\mathrm{link}$ between
$\mathrm{closure}(A)$ and
$\mathrm{closure}(B)$ in Figure 3 is
$0.75$.Footnote m
4.2.2 Path-based MAR
The second variant is $\mathrm{MAR}^\mathrm{path}$, measuring the agreement on paths. A path is defined as a sequence of nodes in the argument tree with one or more consecutive edges. For example, the set of path P of annotation A in Figure 3 is
$\{ (4,2,1), (4,2), (2,1), (2,4,3), (2,4), (4,3)\}$.
$\mathrm{MAR}^\mathrm{path}$ between two sets
$P_A$ and
$P_B$ are calculated as in Equation (3). For example,
$\mathrm{MAR}^\mathrm{path}$ between annotation A and B in Figure 3 is
$0.31$.

When we also consider the implicit links, a path in the closure structure results as a mixture of explicit and implicit links. Unlike $\mathrm{MAR}^\mathrm{link}$, we treat implicit links the same as explicit links in
$\mathrm{MAR}^\mathrm{path}$.
$\mathrm{MAR}^\mathrm{path}$ between
$\mathrm{closure}(A)$ and
$\mathrm{closure}(B)$ in Figure 3 is
$0.57$.
4.2.3 Descendant set-based MAR
The third variant is $\mathrm{MAR}^\mathrm{dSet}$, which measures the agreement based on the existence of the same descendant sets (dSet) in two structures. In contrast with the other two measures,
$\mathrm{MAR}^\mathrm{dSet}$ performs its calculations using bigger and more interdependent units. We define the descendant set of node X as the set consisting of the node X itself and its descendants. Figure 4 shows an example of the descendant set matching between two annotations. The descendant set in brackets is given below the node ID (which is the sentence position). For example, the descendant set of node 2 of annotation A in Figure 4 (left) is
$\{2,3,4,5\}$.

Figure 4. Example of descendant set matching between annotation A (left) and B (right). Exact-matching scores in red (to the left of each node); partial-matching scores in green to the right. Gray nodes represent non-AC.
We have hypothesized that groups of sentences in an essay operate as one sub-argument. $\mathrm{MAR}^\mathrm{dSet}$ can be seen as a measure of the degree of agreement on such sub-arguments. If two annotations have a high
$\mathrm{MAR}^\mathrm{dSet}$, they group many of the same set of sentences together.
There are two types of matching: exact and partial. Under exact matching, a binary score is calculated and two annotations are required to have identical descendant set in order to score a value of 1. For example, the exact matching score for the descendant set rooted in node 2 between annotation A and B in Figure 4 is 0. Partial matching, in contrast, returns continuous scores based on the recall of the descendant set of one annotation, calculated with respect to the other annotation. Non-argumentative nodes are counted as a match if they are deemed non-argumentative in both annotations.
In this metric, a structure is represented by the descendant set matching scores of its nodes. We define a function f that maps a structure to a vector consisting of descendant set matching scores. For annotation A in Figure 4, $f(A)=[0,0,1,1,0]$ when using exact-matching, and
$f(A)=[\frac{4}{4}, \frac{3}{3}, \frac{1}{1}, \frac{1}{1}, 0]$ when using partial-matching.
$\mathrm{MAR}^\mathrm{dSet}$ is computed as in Equation (4), where
$\sum{}{}$ denotes the summation of vector elements and
$|N|$ corresponds to the number of nodes in the structure. It measures the average of average recall.

$\mathrm{MAR}^\mathrm{dSet}$ scores between annotation A and B in Figure 4 are 0.40Footnote n and 0.76Footnote o for exact and partial matching, respectively.
Here, we report all three MAR variants because together these structure-based metrics provide us with analytical tools which can measure the agreement on argument paths and descendant sets. For comparison with the literature, we will also report the graph-based metric proposed by Kirschner et al. (Reference Kirschner, Eckle-Kohler and Gurevych2015), which is somewhat similar to ours. It measures the extent to which a structure A is included in structure B. The inclusion score $I_A$ is shown in Equation (5), where
$E_A$ represents the set of links in A; (x,y) denotes two nodes connected by a link; and
$\mathrm{SP}_B(x,y)$ is the shortest path between nodes x and y in B.

The same concept is applicable to measure $I_B$. This metric measures whether two linked nodes in annotation A also directly or indirectly exist in annotation B. Similar to
$\mathrm{MAR}^\mathrm{path}$, we consider implicit links as if they are explicit when computing Kirschner’s metric for closure structures, because a path is a mixture of explicit and implicit links. There are two ways to combine inclusion scores
$I_A$ and
$I_B$: by averaging or calculating the F1-score between them. For example, the graph-based agreement scores between two structures in Figure 4 are 0.88 (avg.) and 0.86 (F1).
4.3 Meta-evaluation of structure-based agreement metrics
If one introduces a new metric, one should evaluate it against human intuition; such an undertaking, as an evaluation of an evaluation metric, is referred to as a “meta-evaluation.” We use the crowd-sourcing platform Amazon Mechanical Turk (AMT) for the meta-evaluation, and elicit similarity judgments about pairs of human annotations. In this crowd-sourcing task, workers are asked to judge two different options and to tell us which option represents the higher similarity. One option compares two argumentative structures for an essay X annotated by two different annotators A and B. The other option compares two structures for a different essay Y, again annotated by A and B. Given these two pairs of two structures (a pair for an essay), workers judge which pair is more similar according to their intuition concerning the composition of the hierarchical structures. They evaluated based on three aspects: placement of nodes in the hierarchical structure, grouping of nodes forming sub-trees and links between nodes.
In Figure 5, which illustrates our AMT task, numbered nodes represent sentences and arrows represent argumentative relations between sentences. The structures shown to our workers contain only node IDs and directed links. We replaced undirected links with directed links in order to simplify the task for the crowd workers. We also show the structures without any text or relation labels. This is because the interpretation of the relation labels would require expertise in discourse analysis, which is not available in the crowd-sourcing paradigm. Workers therefore also cannot judge whether implicit links should hold or not, and so our evaluation uses scores which are calculated on explicitly annotated links only.
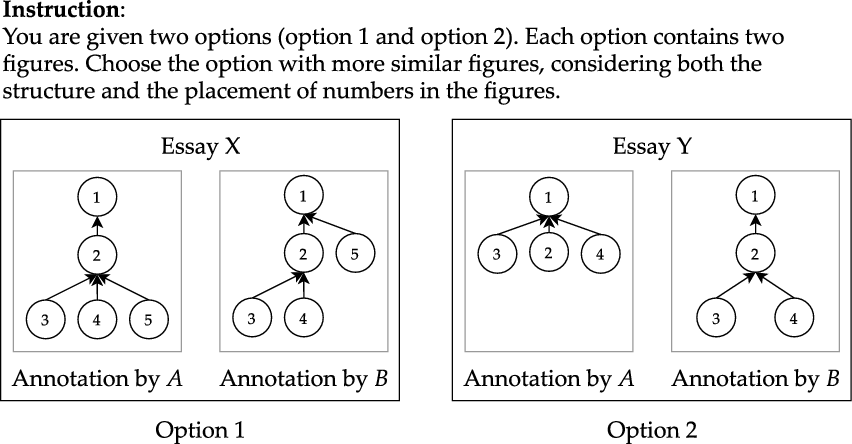
Figure 5. Illustration of an “AMT task.”
In a crowd-sourcing experiment, it is difficult to evaluate whether workers provide their responses earnestly. We employ AMT workers who have above 95% approval rating and record 30 votes in total for each question item. We consider responses made too quick or too slow as noises or spams, and to filter them, we remove responses corresponding to the 5% fastest and slowest, leaving us with the 90% of the responses in the middle.
For each AMT task, we count the votes given by crowd workers for Option 1 and Option 2 as $V_1$ and
$V_2$, respectively. In parallel, we calculate the agreement scores
$M_1$ and
$M_2$, for each option, under each of the metrics M tested here. We compare agreement ratio and four versions of ours, namely
$\mathrm{MAR}^\mathrm{link}$,
$\mathrm{MAR}^\mathrm{path}$,
$\mathrm{MAR}^\mathrm{dSet}$ (exact-match), and
$\mathrm{MAR}^\mathrm{dSet}$ (partial-match), and Kirschner’s metric. We are the first to provide a meta-evaluation of Kirschner’s metric since the original publication did not provide one.Footnote p
There are four aspects of evaluation we measure. First, we use accuracy to measure whether the metrics’ prediction agrees with the majority voting result. When the voting is tied, meaning that the workers have no preference between the two pairs, we also check whether the metric assigns the same score for both pairs. For the second aspect of evaluation, we use weighted accuracy (W.Acc.) to simulate the fuzzy nature of human judgment. When a metric assigns a higher score, for example, $M_1 > M_2$, it gains a normalized voting score
${V1}/{(V1+V2)}$. One can interpret this as the probability of the metric being aligned with the workers’ preference. Third, we calculate the minimum squared error (MSE) between automatically assigned scores and normalized voting differences, that is, between
$(M_1 - M_2)$ and
$\left[ \left(V_1-V_2\right) / \left(V_1+V_2\right)\right]$. This measures whether the metrics can estimate the exact numerical difference of votes. Lastly, we calculate the linear correlation between the differences in metric scores and normalized voting differences.
We use argumentative structures from 20 randomly chosen ICNALE essays, annotated by two annotators each. Random selection was stratified according to score, country, and prompt. The texts contain 13.3 sentences on average.Footnote q If each essay’s structures are compared to each other essay’s structures, $\binom{20}{2}$=190 possible “AMT tasks” result. Given the 30 responses per task, there were a total of 5700 responses. Five-thousand and one-hundred thirty responses remained after we applied the time cutoff described above.
Table 1 shows the results of the meta-evaluation. Kirschner’s metric and $\mathrm{MAR}^\mathrm{link}$ achieve the same performance in terms of accuracy. Kirschner’s metric achieves the highest performance in W.Acc. (0.64), while our proposed metric,
$\mathrm{MAR}^\mathrm{link}$, achieves the best performance in terms of MSE (0.11) and Pearson’s correlation (0.71). For W.Acc, the numerical difference between Kirschner’s metric (F1) and
$\mathrm{MAR}^\mathrm{link}$ is 0.01. The difference between MSE of Kirschner’s metric (F1) and
$\mathrm{MAR}^\mathrm{link}$ is 0.01. Although
$\mathrm{MAR}^\mathrm{link}$ has a slightly higher correlation value to human judgment compared to Kirschner’s metric, the difference is only 0.04. We also note that the agreement ratio performs the worst under all evaluation aspects, with low correlation to human judgments.
Table 1. Evaluation result of structure-based inter-annotator agreement metrics
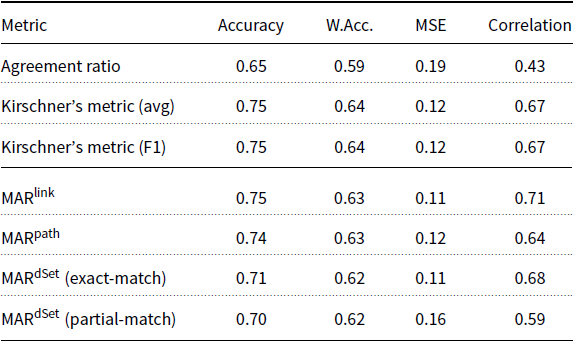
$\mathrm{MAR}^\mathrm{link}$ and Kirschner’s metric are roughly in the same ballpark when it comes to capturing human intuitions, but we still prefer
$\mathrm{MAR}^\mathrm{link}$ because it is able to treat implicit and explicit links differently (although we were not able to test this property in the current experiment). This mechanism is unique among all metrics, and it should be useful for many purposes.
We have performed a preliminary meta-evaluation of our novel structure-based metrics and Kirschner’s metric that shows good results as far as the basic interpretability of these metric goes; correlation with human judgments is moderate to good. We are now in a position where we can analyze structural agreement using these new metrics, as we will do in the rest of this paper.
5. Corpus annotation
This section describes our agreement study and the annotation of the ICNALE-AS corpus. We report intra- and inter-annotator agreement scores to show that our scheme is stable and reproducible. A scheme is stable if independent annotations by the same person result in high agreement, and reproducible if independent annotations by different people result in high agreement.
5.1 Intra- and inter-annotator agreement study
We use the same 20 randomly sampled ICNALE essays as in the meta-evaluation reported in Section 4.3. They contain a total of 266 sentences, with 3496 possible pairs of sentences to be linked. Annotation was performed with the help of the annotation tool TIARA (Putra et al. Reference Putra, Teufel, Matsumura and Tokunaga2020).Footnote r
We report our agreement scores under closure because in our opinion this corresponds most closely to the truth. We also report the scores calculated on explicit links only to allow a comparison with previous argumentation schemes. However, in our opinion, the use of non-closure metrics is not advisable in situations like ours where equivalence classes are defined, which negatively affects the metrics’ interpretability.
To measure annotation stability, we employ a paid annotator (annotator A), a PhD student in English Education with special expertise in text assessment and discourse analysis and years of experience as an EFL teacher. Although not a native speaker of English, annotator A is very familiar with reading, assessing and improving EFL texts in the course of their daily operations. It is generally accepted that it is not necessary to use English native speakers for experiments in argumentation or discourse studies because the associated tasks require cognition rather than syntactic ability.
We prepared guidelines of ten pages describing the semantics of each category, which were available to the annotator during annotation, and asked the annotator to annotate 20 essays twice from scratch over the course of a month of interim period. We assume that a month would be long enough for the annotator to have forgotten their original annotation.
The result of the intra-annotation study, shown in Table 2, demonstrates that the annotation is stable.Footnote s Annotator A has an almost perfect agreement to themselves, including producing almost exactly the same structures (both explicit and implicit). The confusion matrix in Table 3 between the first and second versions of annotations by annotator A shows that the only difficulty faced by annotator A lay in distinguishing between the detail and support labels in a few cases.
We next performed an inter-annotator agreement study between annotator A and the first author of this paper (annotator B) using the same texts as in the intra-annotator study. We compare the first annotation of annotator A with annotator B’s annotations.
Table 4 shows the inter-annotator agreement scores. The agreement scores on argumentative component identification were measured at $\kappa=0.66$ (
$N=266$,
$n=2$,
$k=2$).Footnote t There were only 10 (
$\sim$4%) and 5 (
$\sim$2%) sentences marked as non-argumentative sentences by annotator A and B, respectively. Cohen’s
$\kappa$ was measured at 0.53 (0.50 on closures;
$N=3496$,
$n=2$,
$k=2$) for linking, and 0.61 (
$N=133$,
$n=4$,
$k=2$) for relation labeling. Table 5 shows that the most frequently confused labels are again detail and support.
Table 2. Intra-annotator agreement of annotator A
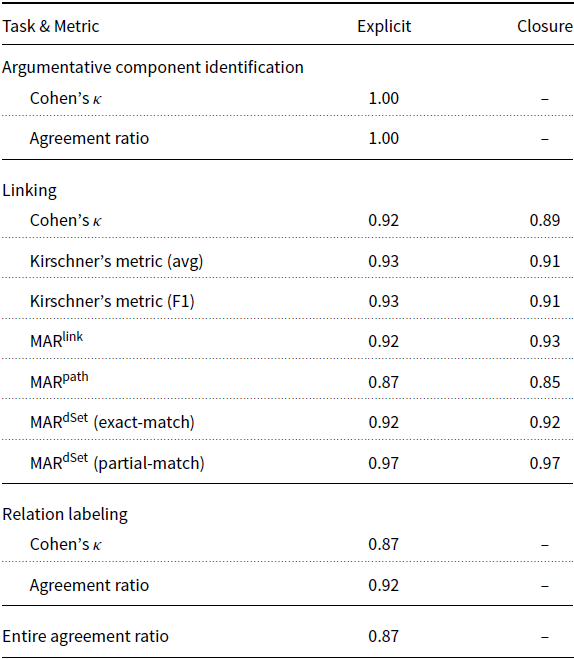
Table 3. Confusion matrix of annotator A in intra-annotator agreement study
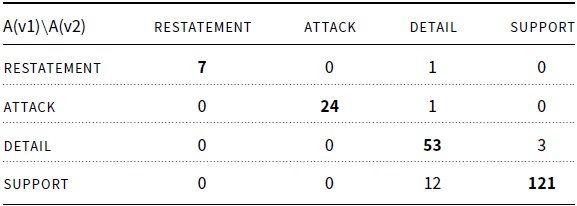
Table 4. Inter-annotator agreement results
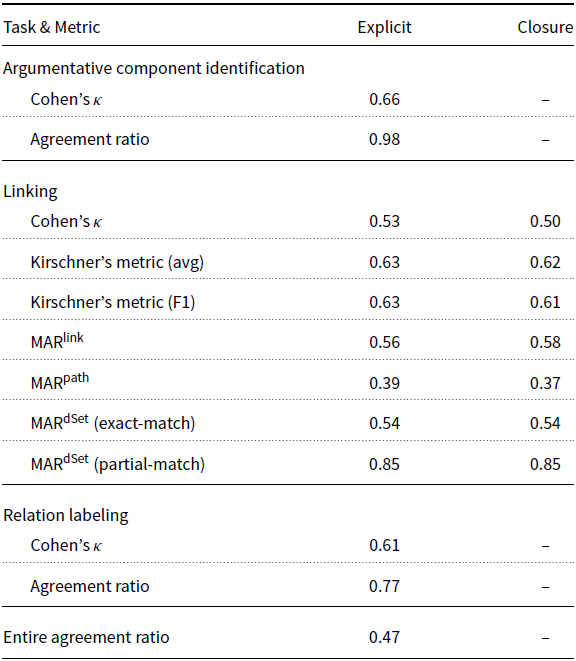
Table 5. Confusion matrix between annotator A and B in the inter-annotator agreement study
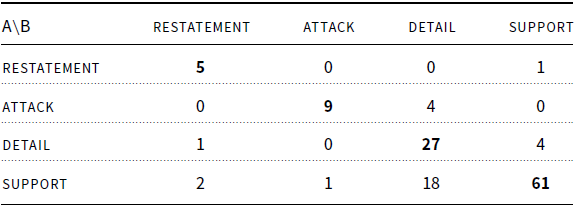
We manually inspected the cases concerned in the confusion between these labels. One of the likely reasons we found concerns a difficulty in judging whether or not some argumentative material is new (if it is new, the correct label is support; if it is not, detail is correct). Another reason concerns the use of examples, as these can be seen as either elaboration (detail) or actual supporting evidence (support). Consider the following excerpt (ICNALE essay W_JPN_PTJ0_005_B2_0_EDIT).
$_\mathrm{(S5)}$ If they have a part-time job they can learn a lot.
$_\mathrm{(S6)}$ For example, responsibility, hospitability, communication skills, how to solve problems, and so on.
S6 can be seen as supporting S5 by bringing to light new evidence or as elaborating on what can be “learned”, which would make it a detail. One way to mitigate the confusion is to explicitly assign all exemplifications as detail in future guidelines. This would acknowledge that in most cases, examples are used to provide additional detail to the target sentences.
5.2 Description of resulting corpus and qualitative analysis
Production annotation was then performed by annotator A on the remaining 414 essays out of 434 ICNALE essays at our disposal (excluding the 20 already used for meta-evaluation and agreement studies).
Our final corpus, ICNALE-AS, consists of 434 essays: 414 production essays + 20 essays from the inter-annotator study. It is the annotations by annotator A that are used throughout, and there are two reasons for this. First, because annotator A is a discourse analyst and EFL teacher, we consider them the expert in the subject area. Second, by employing an external expert annotator, we expect to avoid our own bias and ensure the consistency of the annotation.
The corpus consists of 6021 sentences in total, containing 5799 (96.3%) ACs and 222 (3.7%) non-ACs (cf. Table 6). The argumentative discourse structures have an average depth of 4.3 (root at depth 0). Support is the most commonly used relationship (3029 instances–56.5%), followed by detail (1585–29.5%), attack (437–8.1%) and restatement (314–5.9%). This distribution is unsurprising given that students are often explicitly taught to write supporting reasons for their arguments. The number of restatement relations is lower than the number of essays, which means that some student arguments do not contain any conclusion statements anywhere.
Table 6. Statistics of the final corpus. Sentences and tokens are automatically segmented using nltk (Bird, Klein, and Loper Reference Bird, Klein and Loper2009)
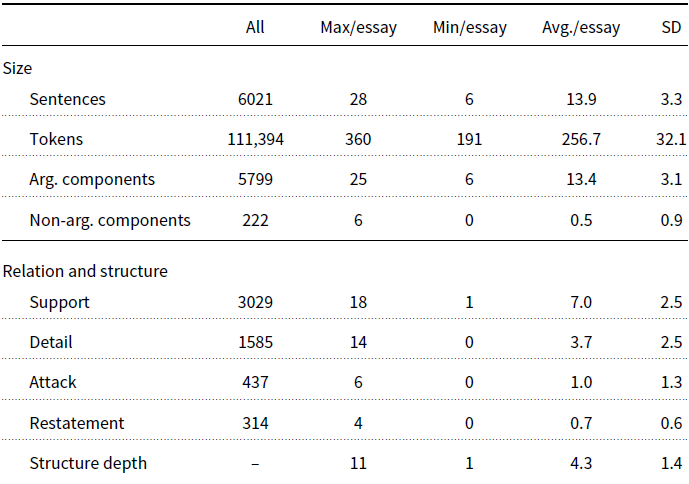
We next look at how far related sentences are separated from each other. In the ICNALE-AS corpus, adjacent links predominate ($56.5$%). Short-distance links (
$2\leq$separation
$\leq 4$) make up
$23.7$% of the total. On the other hand, long-distance links (
$5\leq$separation
$\leq 26$) make up
$19.8$%.
Overall, in 78.5% of directed relations, the source sentence succeeds the target sentence in textual order (or in other words, the link was “backward”). The EFL students predominantly tend to use the “claim–support” structure, in which an opinion is stated first and then its evidence is presented afterward. Again, this is expected, as argumentative writing in English is often taught in this way (Bacha Reference Bacha2010). Table 7 shows the ratio of backward and forward links for each directed relation type. For support and attack, the backward direction is strongly preferred over the forward direction. The detail label stands out because the preference between forward and backward direction is not as strong as the other labels.
Table 7. Distribution of relation direction
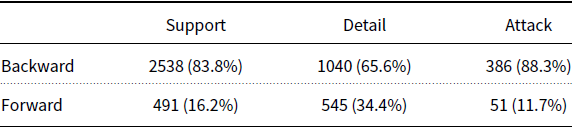

Figure 6. An excerpt of annotation for essay ‘W_PAK_SMK0_022_B1_1_EDIT’. (a) Original essay. (b) A potential improvement for (a).
Our annotation allows for potential argument-related problems to be flagged. Because we can point out the exact problematic sentences or relations, this information can then be used during teaching. For instance, we found 31 essays (7.1%) which contain more forward than backward relations. This contradicts the standard writing preference. These essays tend to present evidence and supporting material at the beginning of the text, followed by the major claim later. We consider this an example of a potential problem. Other cases exist in which a considerable amount of background information is presented before the start of the argument proper, another potential argument-related problem.
Figure 6(a) shows an annotation example. In this figure, sentence S16 has been identified by our annotator as the clearest statement of the major claim; it therefore became the root of the structure. Prescriptive writing guidance for argumentation (Silva Reference Silva1993; Bacha Reference Bacha2010) would advise putting such a sentence early in the text.Footnote u However, the EFL student placed it at the end of the essay. This potential problem could be used as an example in a teaching session.
Another indicator of a problem is crossing links in the structure. Because argumentative relations typically hold between sentences stating similar ideas, crossing links might indicate coherence breaks in texts. Ideally, if all sentences constituting a sub-argument are presented together, few or no crossing links should occur. For example, the topic of both sentences S9 and S14 in Figure 6(a) is nicotine, but the discussion on this topic is interrupted by several sentences discussing different topics. If sentences S9 and S14 were placed close to each other, we can expect an improvement in the textual coherence of the essay.
Figure 6(b) shows one possible improvement of the essay by rearranging sentences in a way that would be consistent with the discussion above—sentence S16 has been moved to the beginning of the essay,Footnote v and sentences S9 and S14 are now adjacent. The improved text is more consistent with the argumentative-development-strategy in prescriptive writing guidelines; it first introduces a topic, then states its stance on that topic, supports its stance by presenting detailed reasons, and finally concludes the essay at the end (Silva Reference Silva1993; Bacha Reference Bacha2010).
However, an essay is not guaranteed to be problem-free, even if the major claim is placed at the beginning and there are no crossing links. The essay in Figure 7(a) is one such case—S1 is its major claim, and S16 restates it, acting as the conclusion at the end. There are no crossing links. However, sentence S17, which supports the major claim, appears after S16. According to prescriptive guidance, reasons supporting the major claim should be placed before the concluding statement. Therefore, S17 should be placed somewhere between sentences S1 and S16, as shown in Figure 7(b).
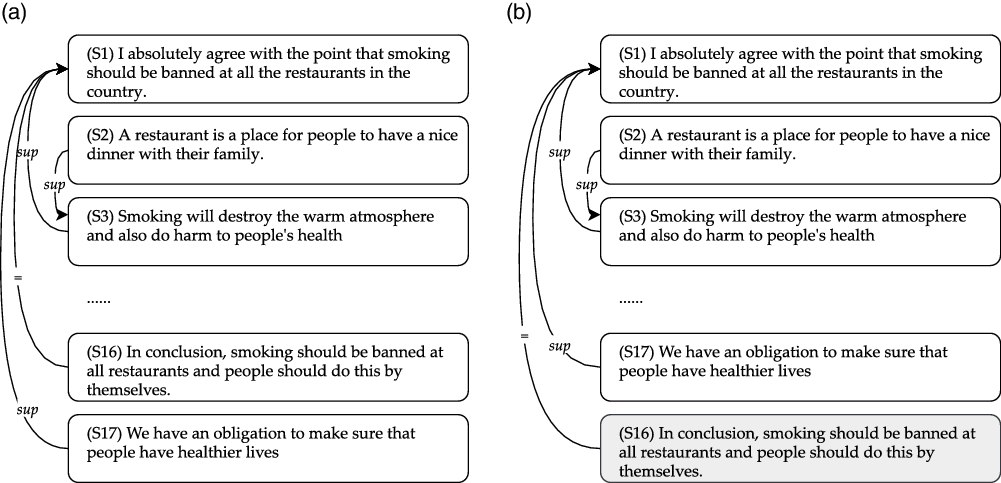
Figure 7. An excerpt of annotation for essay “W_CHN_SMK0_045_A2_0_EDIT”. (a) Original essay. (b) A potential improvement for (a).
6. Conclusion
In this article, we presented ICNALE-AS, a corpus of 434 argumentative essays written by Asian EFL students annotated with argumentative structures. This corpus is unique among other corpora concerned with argumentative text, for example, the microtext (Peldszus and Stede Reference Peldszus and Stede2016) and the persuasive essay corpora (Stab and Gurevych Reference Stab and Gurevych2014; Reference Stab and Gurevych2017), in that it contains the argumentative structures of intermediate-quality texts. We employed four relation types in our scheme, namely support, detail, attack, and restatement. We proposed to encode the semantics of restatement as an equivalence class.
Inter-annotator agreement analysis showed that the proposed annotation scheme is stable, with near-perfect intra-annotator agreement and reasonable inter-annotator agreement. Results for the three tasks we propose are as follows: Cohen’s $\kappa=0.66$ (
$N=266$,
$n=2$,
$k=2$) for argumentative component identification, Cohen’s
$\kappa=0.53$ (
$N=3496$,
$n=2$,
$k=2$) for linking argumentative components, and Cohen’s
$\kappa=0.61$ (
$N=133$,
$n=4$,
$k=2$) for four-way argumentative relation labeling.
This article also proposed a novel structure-based inter-annotator agreement metric, mean agreement in recall (MAR), which provides a more holistic approach to the evaluation of structural agreements. It comes in three variants, which offer different insights depending on which unit of analysis is of interest (link, path, or descendant). A large-scale meta-evaluation using 5130 similarity judgments showed that the simplest variant, $\mathrm{MAR}^\mathrm{link}$, was on par with the structural model proposed by Kirschner et al. (Reference Kirschner, Eckle-Kohler and Gurevych2015) in achieving high correlation with human judgment. However, it was not possible to test all aspects of our metrics within the crowd-sourcing paradigm. We are particularly curious whether our intuitions concerning the implicit links following from the equivalence class property of restatement are borne out in practice. Another meta-evaluation could provide this assessment in the future, but it would require judges with expertise in discourse analysis.
Our qualitative analysis revealed that the argumentative structure annotation can provide us with objective means of improving essay by indicating both potential problems and better sentence rearrangements that can lead to a more coherent text. In our future work, we would like to push this one step further by devising an algorithm for sentence rearrangement to improve the essay quality. The outcome of such a system could help EFL students by showing them how to improve their writings at the discourse level. We plan to provide an additional annotation layer for sentence rearrangement onto the ICNALE-AS corpus. A parallel corpus of original and more-coherent improved texts would enable the empirical analysis of the connections between discourse structure, sentence arrangement and coherence.
The research in this article can thus be seen as one further step away from the more conventional research focused on improving spelling and grammatical errors toward research in improving text at the discourse level.
Acknowledgments
This work was party supported by Tokyo Tech World Research Hub Initiative (WRHI), JSPS KAKENHI grant number 20J13239, and Support Centre for Advanced Telecommunication Technology Research. We are grateful to Professor Yasuyo Sawaki, Kana Matsumura, Dola Tellols Asensi, Haruna Ogawa, and Michael Frey for providing us with feedback for the annotation guidelines and annotation tool TIARA. We also would like to thank anonymous reviewers for their valuable comments.