1. Introduction
An important measure of quality assurance in contemporary methodological thinking is replication (Marsden et al., Reference Marsden, Morgan-Short, Thompson and Abugaber2018a, Reference Marsden, Morgan-Short, Trofimovich and Ellis2018b; Morgan-Short et al., Reference Morgan-Short, Marsden, Heil, Issa II, Leow, Mikhaylova, Mikołajczak, Moreno, Slabakova and Szudarski2018; Porte & McManus, Reference Porte and McManus2019). Replication reigns over most openness initiatives (e.g., open data, open materials, preregistration, registered reports, badges) in that, if replicability across the social sciences was perceived as satisfactory, support for these initiatives would have lost vigor. Voices in support of reproducibility and replicability initiatives have grown in urgency over the years, as a lack of replicability continues to plague many domains of the social sciences. Because of the centrality of replication in contemporary methodological thinking, a great deal of attention has been paid to various aspects of replication, such as its definition and types, interpretation of replication results, and incentives for replication research.
As a sign of the growing recognition of the role of replication in second language development (SLD) research, scholars have proposed somewhat different replication taxonomies and associated terminology. These relate primarily to the comparability of designs across studies. According to one definitional framework, a replication attempt may be direct, partial, or conceptual (Marsden et al., Reference Marsden, Morgan-Short, Thompson and Abugaber2018a). A direct replication involves no intention to change any variables in the initial study,Footnote 1 while minor deviations that will inevitably occur are to be reported as fully as possible. In a partial replication, researchers intentionally change one significant element with the aim of testing the generalizability of a theory and its boundaries. This change could be the type of instrument used, the background of the participants involved, or the outcome variable of interest. Finally, a conceptual replication introduces more than one significant change to the initial study design.
Another prominent approach to classifying replication research is offered by Porte and McManus (Reference Porte and McManus2019). According to this view, a replication attempt may be close, approximate or conceptual. In a close replication, one major variable is deliberately modified; in an approximate replication, two variables are modified. Within this view, there is no room for exact or direct replication because a ‘replicated study can never really be the same, be it repeated by the same researcher in the same context, or others’ (Porte & McManus, Reference Porte and McManus2019, p. 72). Another feature of this approach is its emphasis on study sequence. As this approach is explicitly concerned with systematic programs of research, a close replication is seen as the first step that can then be followed by an approximate replication.
Considering the multidimensionality of SLD, it would seem that one reason why SLD is not currently experiencing a replication crisis is that the field has yet to look systematically at the replicability of its findings or attempt replications at scale (Marsden et al., Reference Marsden, Morgan-Short, Thompson and Abugaber2018a). Underlying this broader lack of engagement with replication, no doubt, are persistent issues of whether replication is valued by researchers (across all career stages), seen as adequately original to merit consideration by scholarly outlets in the field, and otherwise incentivized by institutions and knowledge sharing structures. Despite growing acknowledgement that replication studies are ‘feasible, necessary, and publishable’ (Porte & Richards, Reference Porte and Richards2012, p. 285), in the SLD literature little attention has been paid to replication in the context of complex dynamic systems theory (CDST; de Bot et al., Reference de Bot, Lowie and Verspoor2007; Hiver & Al-Hoorie, Reference Hiver and Al-Hoorie2020b; Larsen-Freeman & Cameron, Reference Larsen-Freeman and Cameron2008). This may be due, in part, to the unfortunate (mis)perception that CDST is essentially a rejection of all causality, quantification, and generalization (e.g., Dewaele, Reference Dewaele, Wright, Harvey and Simpson2019).Footnote 2 Similar arguments have been made about specific sub-disciplines in the field (e.g., Markee, Reference Markee2017; Matsuda, Reference Matsuda2012). Thus, it is important to reflect on how replication fits into the philosophy and practice of CDST research.
In this paper, we add our voice to recent calls to reaffirm a common ‘commitment to sound empirical work’ (e.g., Markee, Reference Markee2017, p. 381) by expanding the ontology and epistemology of replication research. Our overall argument is that centering the discourse on ‘replication’ narrows the scope of fruitful research into open systems, making replication in the conventional, broad sense hardly tenable. We therefore recommend a broader perspective centered around what we call ‘substantiation’ research and explain the conditions that must be met before a replication might be meaningfully attempted. Finally, we survey three avenues relevant to replication in the context of CDST research.
2. A CDST view on replication
2.1 Philosophical implications for replication
CDST is primarily interested in studying complex systems. Open, complex systems present unique challenges to replication researchers. First, while replication may serve a useful verification purpose, the ethos of most conceptualizations of replication tends to lack a systems perspective. CDST studies take a relational-developmental view of the human and social world (Overton & Lerner, Reference Overton and Lerner2014) and examine complex interactions among multiple factors over time. Second, variability is at the heart of CDST research. Replication sometimes sees variability as a problem to be minimized with more precise instrumentation, measurement, and data analysis. CDST research views both inter-individual and intra-individual variability as an indispensable source of information (Molenaar & Campbell, Reference Molenaar and Campbell2009; see also Arocha, Reference Arocha2021; van Geert & van Dijk, Reference van Geert and van Dijk2021), and indeed independent of the scale of observation (Verspoor & de Bot, Reference Verspoor and de Bot2021): Variability both allows for flexible and adaptive behavior and is usually needed for development to occur.
Furthermore, replication is sometimes used as a means to investigate the extent to which findings of ‘what works’ are robust across samples and settings. Even partial and conceptual replications that we have briefly described above seemingly treat context as mere backdrop as they investigate and falsify empirical claims. Consequently, and contrary to CDST research, context is a mere peripheral element of replication. Such an approach to scientific inquiry stems from hypothetico-deductive reasoning that tends to eliminate spatio-temporal context from explanation for purposes of parsimony. For CDST research, though, contextualization is a core principle of knowing (Morin, Reference Morin2008), and CDST research views empirical findings as contingent and contextually-dependent. ‘What works’ is bounded by the history of the system in context, shaped by inside and outside forces, and emerges in context over time.
Moreover, the most common view of replication is where the focus of the study is at the level of the group. That is, the focus of research aggregating data and drawing inferences about the state of affairs in a population—for example, mean patterns or the structure of differences in a population (Molenaar, Reference Molenaar2013)—is squarely on the group level. An implicit assumption of such group-based analyses, in most instances, is that results that hold in a population of more or less homogenous individuals also correspond to the individuals who comprise that population. This ecological fallacy has been challenged by CDST research (e.g., Larsen-Freeman, Reference Larsen-Freeman2006; Lowie & Verspoor, Reference Lowie and Verspoor2019) showing that ‘the individual and the local are intelligible in themselves’ (Horn, Reference Horn2008, p. 138). CDST has therefore been called a science of the individual (see Molenaar, Reference Molenaar2004; Rose et al., Reference Rose, Rouhani and Fischer2013). Whereas a pragmatic approach to CDST research can adopt either individual- or group-level analyses (Hiver & Al-Hoorie, Reference Hiver and Al-Hoorie2020b), equivalence in CDST research is never assumed as results obtained at the group level may not automatically transfer to the level of the individual, and vice versa.
Finally, replication is premised on an assumption that change in the value of an effect is proportionate to changes in causal elements. The simplest expression of this is through a linear equation—as in the general linear model—showing that if value(s) of the causal elements change by any given amount, a proportionate change in the value of the dependent element may be predicted (Byrne & Callaghan, Reference Byrne and Callaghan2014). There is little room for emergence or the indeterminacy of outcomes that result from CDST's soft-assembly in these causal accounts. In contrast, CDST research is interested in non-linear systems and processes, in which the size of the outcome may not be directly related or proportional to the size or direction of the input. Instead, such complex regularities and dynamic trajectories can be understood as the emergent result of interaction among agents.
Two widely popularized philosophy of science views seem to have diverted attention from accounting for the complexity of social phenomena. It has been proposed that theories start as a conjecture that needs to be falsified (Popper, Reference Popper2014), and that science operates through successive scientific revolutions as theories no longer satisfactorily account for findings (Kuhn, Reference Kuhn2012). In what seems to be an implicit acknowledgement of the elusiveness of these philosophies, however, the actual practice of social science researchers has challenged notions of falsification, paradigms, and scientific revolutions (Sanbonmatsu et al., Reference Sanbonmatsu, Posavac, Behrends, Moore and Uchino2015). When an object theory is still immature, adopting the Popperian hypothetico-deductive model makes hypothesis tests weak, their interpretation elusive, and confident conclusions from them untenable—a situation some describe as ‘hypothetico-deductivism gone awry’ (Scheel et al., Reference Scheel, T, Isager and Lakens2021, p. 745).Footnote 3 Attempting to falsify the inherently vague findings obtained within theoretically still immature topical areas can be of little value, as this may result in prematurely rejecting a partially valid theory (Loehle, Reference Loehle1987; Scheel et al., Reference Scheel, T, Isager and Lakens2021), a process Lakatos (Reference Lakatos1978) called dogmatic falsificationism.
Progress, discoveries, and breakthroughs are less often the result of bold armchair conjecturing, and arise more frequently due to technological and methodological innovations (Greenwald, Reference Greenwald2012), leading to tools and instruments that open up realms previously inaccessible. Following Occam's razor, propositions resulting from such armchair conjecturing tend to be commonsensical and unappreciative of the (already acknowledged) underlying real-life complexity—to the extent that practitioners (Al-Hoorie et al., Reference Al-Hoorie, Hiver, Kim and De Costa2021), or even laypeople (Hoogeveen et al., Reference Hoogeveen, Sarafoglou and Wagenmakers2020), can readily anticipate them. Further, theoretical advances are not the exclusive domain of the paradigm shifts and revolutions described by Kuhn (Reference Kuhn2012). Current theory and understanding of a field no doubt builds incrementally on past understanding (Larsen-Freeman, Reference Larsen-Freeman, Ortega and Han2017), and any romanticized breaks with the past may represent little more than unrealistic ‘cartoon caricatures’ (Sanbonmatsu & Johnston, Reference Sanbonmatsu and Johnston2019, p. 680). The idealistic and stereotypical views proposed by Popper and Kuhn—which Kuhn for his part distanced himself from in later years of his career (Sanbonmatsu & Johnston, Reference Sanbonmatsu and Johnston2019)—might have impeded theory development through genuinely productive exploratory research practices, which in some circles have become ‘second-class citizens’ (Klahr & Simon, Reference Klahr and Simon1999, p. 526) of methodological designs. Emphasis on falsification and anticipation of revolutions might have dampened interest in exploration of complex phenomena, development of novel instruments that could facilitate construct formation and elaboration (Loehle, Reference Loehle1987; Scheel et al., Reference Scheel, T, Isager and Lakens2021), and consequently enhancement of theoretical maturity.
2.2 Practical implications for replication
In contrast to the highly controlled conditions of experimental research conducted under lab settings, open complex systems involve a multitude of factors that are beyond the control or even the knowledge of the researcher. The development of these systems is also unpredictable due to sensitivity to initial conditions, even in cases that follow deterministic structures (Lorenz, Reference Lorenz1963). The systems approach stands in contrast to claims that there is a degree of correspondence between experimental results, their description (often using statistical formalisms), and reality (Byrne & Callaghan, Reference Byrne and Callaghan2014). Thus, the contribution of CDST research and its systems perspective to the field suggests that more established conceptual frameworks upon which conventional replication research is built, have so far proven ‘inadequate to the task of integrating new empirical advances’ (Overton, Reference Overton2013, p. 94). The driving question is whether theories of language use and language development, as with other theories in the social sciences, have reached a level of theoretical maturity that allows accurate prediction and thus replication (Scheel et al., Reference Scheel, T, Isager and Lakens2021).
As Sanbonmatsu and Johnston (Reference Sanbonmatsu and Johnston2019; see also Broers, Reference Broers2021) point out, theories of complex topics in the social sciences hardly ever offer precise point predictions of treatment effects, group differences, or relations among constructs. Instead, these theories typically predict the sign of a certain relationship, as in anticipating that learners with certain characteristics tend to develop ‘faster,’ ‘better’ or ‘further’ than others, without specifying exact, theoretically-driven quantitative point estimates such as a mean group difference of 2.17 or an effect size of 4.35. Nor do these theories provide clear replication intervals (Stanley & Spence, Reference Stanley and Spence2014), outside of which the theory is falsified; besides unknown moderators, researchers readily acknowledge that results can potentially vary—even in sign—due to factors as mundane as time of the day, mood of the learner, and a multitude of unknown and unknowable contingencies. Indeed, ‘Anything that is complex, upon closer examination becomes more complex’ (Hansen, Reference Hansen2011, p. 119). The most these theories may be able to offer is qualitative trends rather than quantitative estimates—even when an impressive array of advanced statistical analyses is employed (Sanbonmatsu & Johnston, Reference Sanbonmatsu and Johnston2019). Indeed, it is no secret that effect sizes are not independent from the designs (Vacha-Haase & Thompson, Reference Vacha-Haase and Thompson2004, p. 478) or the designers (Plonsky & Gass, Reference Plonsky and Gass2011, p. 353) that created them (see also Broers, Reference Broers2021, for further critique of effect sizes). This raises the question of the extent to which it makes sense to speak of replication in the conventional sense in relation to complex SLD-related topics.
Consider, for example, the case of real-world interventions. In replication of field experiments, there is a constant tension between adherence to and adaptation of experimental protocols (Bauman et al., Reference Bauman, Stein and Ireys1991; Hansen, Reference Hansen2011; von Thiele Schwarz et al., Reference von Thiele Schwarz, Förberg, Sundell and Hasson2018). When replicating an intervention (e.g., to improve learning or teaching) in a new context (e.g., another country—or even another school), ensuring intervention fidelity becomes a fuzzy judgement call, making the replication by definition conceptual due to the various contextual affordances related to culture, history, student population, faculty training, laws and regulations, to name only a few. Even replicating a (successful) intervention in the same context sometime in the future can lead to different, or even contradictory (Hansen, Reference Hansen2011), outcomes owing to generational characteristics, emerging technologies, evolving social, political and economic circumstances, and a myriad of other factors. Considering that most theories, due to their immaturity, are silent about the effect of numerous moderators and contingencies in the context where they were originally devised, let alone in new or future contexts, this again raises the question of the extent to which it makes sense to speak of conventional notions of replication in relation to complex systems.
Beyond structural contextual differences, mere happenstance can also prevent an intervention from proceeding as intended. As Kaplan et al. (Reference Kaplan, Cromley, Perez, Dai, Mara and Balsai2020) argued, the actual implementation of educational interventions is bound to encounter fortuitous circumstances that are part and parcel of everyday reality. In reflecting on the ten randomized controlled educational experiments they conducted over four years, Kaplan and colleagues recounted some of the unanticipated events they encountered, such as students cramming rather than drawing from the treatment (cognitive and motivational support in their case) at one institution, and administrators rescheduling the course and said treatment due to snow closures at another institution. Eliminating these ‘nuisance’ factors from consideration for the sake of so-called theoretical purity simply means shifting the burden of dealing with these real-life issues from researchers to the end consumers. At the same time, the more research attempts to approximate the complexities of real life, the more unwieldy theories inevitably become, illustrating the perpetual trade-off between generality and precision in social science theorizing (Sanbonmatsu & Johnston, Reference Sanbonmatsu and Johnston2019). By the time broad-strokes pedagogical implications reach the practitioner, research findings become so irrelevant and in need of substantial localization, making most generalities closer to pseudo-applications (Al-Hoorie et al., Reference Al-Hoorie, Hiver, Kim and De Costa2021).
2.3 The substantiation framework
Our discussion so far has raised a number of issues related to feasibility and meaningfulness of replication when it comes to open, complex systems. Addressing some of these conceptual ambiguities involves three dimensions: result interpretability, theoretical maturity, and terminological precision. The first dimension entails a major shift in attitude: Replication is to be (re)defined in relation to interpretability of results rather than in relation to methodological comparability to the replication study (Nosek & Errington, Reference Nosek and Errington2020). From this perspective, the new definition of a replication shifts focus from the operational characteristics of the replication study (i.e., a continuum of how close it is to the initial study at the methodological level: direct, partial, close, approximate, etc.) to the interpretability of possible outcomes. More specifically, the definition of a replication now becomes ‘a study for which any outcome would be considered diagnostic evidence about a claim from prior research’ (Nosek & Errington, Reference Nosek and Errington2020, p. 1, emphasis added). Accordingly, in order for a study to qualify as a replication both positive and negative resultsFootnote 4 must hold evidentiary value; findings consistent with the initial claim must lend support to it, while findings inconsistent with it must also decrease confidence in it and not be dismissed as a design artifact.
The second dimension is theoretical maturity (e.g., Loehle, Reference Loehle1987; Nosek & Errington, Reference Nosek and Errington2020; Valentine et al., Reference Valentine, Biglan, Boruch, Castro, Collins, Flay, Kellam, Mościcki and Schinke2011). A mature theory provides a clear account of the phenomenon of interest, including the necessary and sufficient conditions to reproduce certain outcomes (Open Science Collaboration, 2015). When it comes to direct replication, even though the replication study may not exactly mirror the initial study, theoretical clarity would justify describing the replication attempt as direct when dissimilar aspects are theoretically posited as being irrelevant prior to reproducing a specific result. In other words, according to the theory under investigation, two studies would be considered the same given that ‘the theoretically relevant conditions’ (Stroebe & Strack, Reference Stroebe and Strack2014, p. 62)—that is, the conditions under which a theory applies and that are necessary to arrive at an outcome of interest—are satisfied. From this point of view, it would be fair to assume that a condition is irrelevant if it is not explicitly stated otherwise in the initial study (Simons et al., Reference Simons, Shoda and Lindsay2017; see also later). Both positive and negative results from direct replications can therefore provide evidentiary value when a theory is mature.
When it comes to conceptual replication, particularly when the results are negative, this type is considered ‘in a weaker position’ (Marsden et al., Reference Marsden, Morgan-Short, Thompson and Abugaber2018a, p. 366) and consequently it is ‘a more high-risk undertaking’ (Porte & McManus, Reference Porte and McManus2019, p. 94) than other types of replications. Failed conceptual replications may be less likely to be published (Crandall & Sherman, Reference Crandall and Sherman2016) and may generate less circulation even in informal channels (Pashler & Harris, Reference Pashler and Harris2012). This is due to the chronic ambiguity about whether the result inconsistency is due to a flawed theory or an inappropriate operationalization on the part of the conceptual replication. However, a replication study based on mature theorizing would be able to provide evidentiary value whether the results are positive or negative. In fact, a conceptual replication under theoretical maturity may provide stronger, not weaker, evidence because what matters is not merely reproducing a certain outcome, but verifying the postulated processes underlying it (Stroebe & Strack, Reference Stroebe and Strack2014). As a result, theoretical maturity allows both positive and negative results, from both direct and conceptual replications, to make valuable contributions to the scientific community and, thus, to merit publication.
Finally, accounting for interpretability of results and the maturity of theories requires more precise terminology when considering a study a replication. Whereas a common terminology has helped provide a taxonomy of purposes and design characteristics of replication studies, current nomenclature centers around replication, making it an umbrella term covering too wide a range of research activities. We see this lack of terminological precision as counterproductive. As explained above, the redefinition of replication requires that both positive and negative results be informative and operate under a mature theory. Conceptual replication is, technically, an extension of the theory underpinning the initial results, testing it under different conditions (see, e.g., Marsden et al., Reference Marsden, Morgan-Short, Thompson and Abugaber2018a; Porte, Reference Porte and Porte2012). It may therefore be misleading to describe a study testing a different condition as replication when in fact it is an extension, a follow-up, or a generalizability test (see Marsden et al., Reference Marsden, Morgan-Short, Thompson and Abugaber2018a; Nosek & Errington, Reference Nosek and Errington2020; Porte & McManus, Reference Porte and McManus2019; Zwaan et al., Reference Zwaan, Etz, Lucas and Donnellan2018). This makes the label conceptual replication ‘a practical oxymoron’ (Freese & Peterson, Reference Freese and Peterson2018, p. 302), and therefore we recommend using the term extension instead (see Table 1). Whereas positive results from an extension study would provide evidence in support of extending the theory to these new conditions, negative results may suggest setting a narrower boundary for the explanatory power of the theory or reinterpreting the initial results in light of an alternative explanation. From this perspective, partial, close, and approximate replications become special cases of extension research. Negative results from these designs would be described as failed extensions, not failed replications.
Table 1. From a narrow focus on replication to a broader focus on substantiation
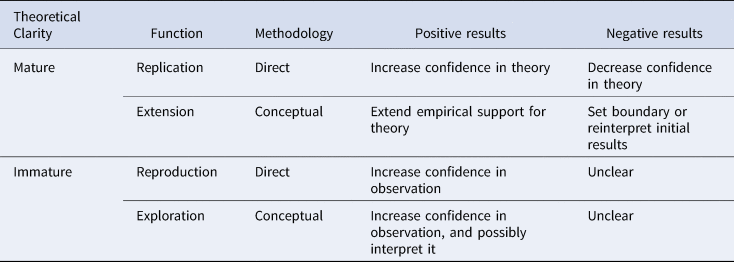
Similarly, speaking of replication under conditions of immature theorizing can be counterproductive. An immature theory does not elaborate on the necessary conditions to produce a particular outcome or the boundaries beyond which the theory no longer holds explanatory power. Findings are therefore merely observations that are not fully understood yet. If theoretical immaturity characterizes the domain under study, researchers may attempt to reproduce an observation under the conditions employed in the initial study. Positive results would increase confidence that the finding is not a fluke, but negative results cannot unambiguously indicate that that observation is a false positive due to the possibility of unknown moderators. On the other hand, testing an ill-defined or atheoretical observation using a different methodology does not constitute an extension—as in the case of a more mature theory—because there are no clear theoretical expectations to test to start with. It would be better conceptualized as an exploration of that observation: positive results increase confidence in it (and possibly help interpret it), but negative results remain unclear.
In short, the meta-scientific discourse currently revolves around replication as the umbrella term that can bias a researcher's thinking and potentially divert attention away from a range of different and legitimate options researchers have. As there does not seem to be an alternative term to replication in current meta-scientific discourse, we recommend substantiation as an organizing framework that covers different functions (replication, extension, reproduction, and exploration) and methodological options (direct and conceptual). This substantiation framework is presented in Table 1.
3. Directions for CDST replication
Any well-articulated attempt to draw a replication map for CDST researchers should, however, acknowledge two related ideas: first, that CDST research must move beyond description and, second, that a major way of doing so is for CDST research to generalize beyond the unique instance. Early CDST research began by describing and analogizing, and it is clear from reviews (Hiver et al., Reference Hiver, Al-Hoorie and Evans2021a, Reference Hiver, Al-Hoorie and Larsen-Freeman2021b) that CDST research has provided particularly strong evidence that many second language (L2) phenomena are relational and non-mechanistic in their development. Beyond describing complex systems and modeling patterns of dynamic change in context, important work remains to be done to help SLD scholars understand whether and how to intervene in and influence the complex dynamic realities of the phenomena under investigation. Intentionally generating positive change that is complex, situated, iterative, and time-scaled in nature may be the next frontier in CDST research (see, e.g., Steenbeek & van Geert, Reference Steenbeek and van Geert2015; van Geert & Steenbeek, Reference van Geert and Steenbeek2014). As applied social scientists, ‘we are not just describers, we are makers and the most important mode through which we make is in application’ (Byrne & Callaghan, Reference Byrne and Callaghan2014, p. 12). Consequently, CDST research must redouble its commitment to yielding knowledge that is of practical use in applied settings and that has potential for social engagement (Levine, Reference Levine2020).
At a rather broad level, conventional replication can be seen as a quest for generalizability. CDST has a unique stance with regards to generalizing (i.e., it is not the same as universalizing), and some have cautioned that assuming an understanding of human and social phenomena only when there is a high degree of predictability and generalizability relies too heavily on deterministic principles (Allen & Boulton, Reference Allen, Boulton, Allen, Maguire and McKelvey2011). Conventional notions of generality may be too restrictive for CDST. However, even researchers operating within assumptions of context-dependence, the importance of initial conditions, interconnectedness, soft-assembly, and emergence should be able to make claims beyond the unique instance. As Byrne (Reference Byrne, Byrne and Ragin2009) proposed, ‘the central project of any science’ is to go beyond the purely idiographic and still elucidate causes that ‘extend beyond the unique specific instance’ (p. 1). Whereas replicability should not be conflated with generalizing beyond the unique instance (cf. Table 1), it may be that certain forms of substantiation can assist in this project of developing a broader understanding of phenomena while also paying ‘careful attention to the limitations of our knowledge claims in time and space’ (Byrne, Reference Byrne, Byrne and Ragin2009, p. 9) in order to prevent premature closure (Larsen-Freeman, Reference Larsen-Freeman and Kramsch2002). With this in mind, the remaining part of this paper presents three approaches to dealing with substantiation and replication in complex systems.
3.1 From representing to an intervening mindset
As mentioned earlier, an important factor behind the complexity of the social sciences is context (Hiver & Al-Hoorie, Reference Hiver and Al-Hoorie2020b; Larsen-Freeman & Cameron, Reference Larsen-Freeman and Cameron2008), as social science phenomena are not invariant across context (Sanbonmatsu & Johnston, Reference Sanbonmatsu and Johnston2019). Results can vary from one condition to another, and the answer is always ‘it depends’. In theory, one way to account for such variability quantitatively is through interactions (as in multiple regression), which permit examining the effect in relation to different factors or treatment conditions. In practice, however, these factors are too numerous and potentially countless.
Once we attend to interactions, we enter a hall of mirrors that extends to infinity. However far we carry our analysis—to third order or fifth order or any other—untested interactions of a still higher order can be envisioned. (Cronbach, Reference Cronbach1975, p. 119)
As explained earlier, this situation becomes even more complex when temporal variation is considered, since factors influencing language use and development change over time as language use and development unfold.
One ontology adopted by most language researchers—including many CDST scholars—is realism (or objectivism), which assumes that a reality exists out there and that the researcher's task is to try and understand it (Hiver & Al-Hoorie, Reference Hiver and Al-Hoorie2020b). Following this philosophy, the researcher's ultimate aim has generally been to propose theories that represent reality as closely as possible. The better researchers come to understand (complex) underlying causal relations, the more accurate their expectations should be. This representational philosophy of science (Freese & Peterson, Reference Freese and Peterson2017; Hacking, Reference Hacking1983; Pickering, Reference Pickering1995) devalues exploratory and pre-theoretical observation and experimentation, consequently impeding the maturation of theories (Scheel et al., Reference Scheel, T, Isager and Lakens2021). An alternative philosophy lets go of the prerequisite to understand reality, its existence notwithstanding, and instead focuses on intervention. Intervening, dealing with, and predicting (especially continually recalibrated short-term predictions, such as what takes place in design-based or single-case research) does not presuppose a full or explicit understanding of the phenomenon or a comprehensive theory of it (Gigerenzer, Reference Gigerenzer2008).
Breaking away from what might be called a theory fetish has been clearly demonstrated in machine learning (Larsen-Freeman, Reference Larsen-Freeman2019). In machine learning, particularly deep learning, huge amounts of data are fed into computer algorithms that generate models too complex to understand but that nonetheless make more accurate predictions than many models derived using conventional theorizing (Yarkoni & Westfall, Reference Yarkoni and Westfall2017). Through the use of big data, these models offer more accurate predictions because they can be as complex as needed to approximate real life, whereas human-generated theoretical models are required to start simple (or simplistic) in line with Occam's razor. The logic behind the machine learning approach flips explanation and prediction. Instead of trying to first come up with an explanation that accounts for the various mediating and moderating mechanisms involved and then leads to prediction, machine-aided algorithms first provide predictions that researchers can later use as input to enhance their understanding of the phenomenon of interest. Indeed, many questions language learning researchers are interested in are inherently predictive, such as finding out who might be successful, who might struggle, who might need additional support, and how long one will need to acquire a particular language feature—all of which are context-dependent.
In one demonstration of the effectiveness of this approach, Kosinski et al. (Reference Kosinski, Stillwell and Graepel2013) analyzed the digital footprint, represented in Facebook Likes, of over 58,000 individuals. Analysis of the contents of their Likes led to successful, if surprising, prediction of various personal attributes such as gender, age, intelligence, ethnicity, sexual orientation, personality traits, as well as religious and political views. The shift to big data requires a change of mindset, putting aside the urge to uncover underlying causal relations, at least temporarily. It also requires developing techniques for continuous data collection to be able to make successive, short-term predictions to accommodate and do justice to developmental variability in language learning.Footnote 5
3.2 From theory to a mini-theory mindset
Sometimes language researchers do wish to understand the causal dynamics of their phenomena. Nevertheless, in recognition of the complexity of language learning, authors are usually expected to devote some space to highlighting the ‘limitations’ of their inquiry. However, limitations sections are often written in a perfunctory manner, highlighting methodological (rather than theoretical) limitations. For example, one popular default is to state that the sample studied or the instrument used is a limitation of the study, but this does not explain the theoretical expectations when this study is replicated on a different sample or with a different instrument. These exclusive methodological limitations ignore the extent to which the theory may come under question when the results fail to replicate. An unfortunate side effect of this ambiguity is ‘the possible cop-out’ (de Ruiter, Reference de Ruiter2018, p. 17) as authors explain away findings that do not replicate. It is all too easy to retrospectively blame a slight design alteration or simply an unknown moderator for results inconsistent with those from the initial study. In a worst-case scenario, initial authors and replicators could keep running in circles trying to interpret non-replications.
One solution to address this inferential looseness is for initial study authors to explicitly specify, in a separate section, the conditions under which the findings should and should not replicate. This additional section has been referred to as constraints on generality (Simons et al., Reference Simons, Shoda and Lindsay2017) and boundary conditions (Busse et al., Reference Busse, Kach and Wagner2017), an exercise that might help address the boundary problem in CDST (Larsen-Freeman, Reference Larsen-Freeman, Ortega and Han2017). Not only does this additional section help future research assess claims less equivocally and attempt to replicate findings (and, more generally, substantiate theories) systematically, but it also forces initial study authors to think more carefully about the replicability of their findings. In other words, this section constitutes a commitment on the part of initial study authors about what would count as evidence or counterevidence of the theory underlying the study—not simply methodological limitations of their particular study. A constraints-on-generality statement may be thought of as ‘a preregistered commentary on future replication studies’ (Simons et al., Reference Simons, Shoda and Lindsay2017, p. 1124), while conditions not explicitly excluded are automatically assumed to be covered by the scope of the initial study conclusions, consequently minimizing disagreements about the interpretation of future non-replications. Without these constraints, readers are left to assume the broadest generalization of a finding, when in fact it might be limited by certain study characteristics such as the type of participants, materials and stimuli, procedures followed, or contextual and historical specificity. An upshot of explicitly stating where the theory is expected to apply and not apply, and where it is silent or agnostic, is that it also provides a rationale for journals to be more willing to consider follow-up replication, extension and, more generally, substantiation research. (See Appendix for an example of a constraints-on-generality statement.)
This way of setting quite narrow bounds (Wallot & Kelty-Stephen, Reference Wallot and Kelty-Stephen2018) and highly circumscribed situations (Sanbonmatsu & Johnston, Reference Sanbonmatsu and Johnston2019) would suggest that it is more appropriate to use the appellation mini-theory instead of theory. Whereas in CDST research everything counts and everything is connected, if applied uncritically, other popular labels such as ‘system’ and ‘model’ (the latter typically being one mathematical manifestation of a theory, as in structural equation modeling; see Larsson et al., Reference Larsson, Plonsky and Hancock2020) may encourage a mindset of universality rather than specificity and locality. A mini-theory with explicit constraints would also compel researchers to generate clear hypotheses for future testing, a feature notably missing from certain subdisciplines in the field (Hiver & Al-Hoorie, Reference Hiver and Al-Hoorie2020a). Explicit mini-theory constraints would additionally oblige terminological precision. The absence of such precise terminology may otherwise lead to a worrisome proliferation of terms with substantial overlap and redundancy (Al-Hoorie, Reference Al-Hoorie2018; MacIntyre, Reference MacIntyre, Al-Hoorie and Szabó2022), all of which are left up to each reader to form their own conception of its meaning and boundaries. Setting bounds and constraints helps form and refine constructs (Busse et al., Reference Busse, Kach and Wagner2017) and avoids conceptual stretching (Scheel et al., Reference Scheel, T, Isager and Lakens2021), a precondition to prediction, replication, expansion, and substantiation. Without clear hypotheses and precise terminology, research remains exploratory aiming to substantiate an as yet immature theory—and should not be represented otherwise.
3.3 From individual findings to a cumulative mindset
In the not-too-distant past, findings reported in a published article were viewed as scientific facts that had withstood the test of peer review. Most scholars now realize that a finding can ‘vibrate’ up and down (Ioannidis, Reference Ioannidis2012)—with effect sizes sometimes ranging between 0.1 and 0.8—depending on the analytical options used. At times, this vibration is due to questionable analytical options selected to produce the best-looking outcome, and at other times simply due to commitment to an arbitrary set of preregistered protocols. Rigorous large-scale randomized controlled trials exhibit a similar level of heterogeneity of effects. For example, a recent meta-analysis of 141 rigorous educational experiments involving over one million students reported a negligible mean effect size (d = 0.06) but with an uninformative range from –0.16 all the way to 0.74 (Lortie-Forgues & Inglis, Reference Lortie-Forgues and Inglis2019). Registered replication reports, where studies are even more tightly controlled and correspond closely to predefined protocols, have similarly shown a pattern of effect variability that is effectively comparable to sampling variability of individual data points within a single study (McShane et al., Reference McShane, Tackett, Böckenholt and Gelman2019; Tackett & McShane, Reference Tackett and McShane2018).
Variability is expected to occur from a CDST perspective. Beyond this inherent variability, such extreme variability is to be expected simply as a result of sampling error and measurement error. For instance, simulation research by Stanley and Spence (Reference Stanley and Spence2014) reached the surprising conclusion that, with a sample size of 80 and a reliability of .90, the replication interval of a (true) correlation coefficient of .30 ranges from .10 to .45. With a reliability of .70, the replication interval ranges from .00 to .50. In other words, assuming that the ‘true’ effect size is .30, a replication study reporting .00 does not constitute counterevidence or a failed replication, but rather a perfectly reasonable outcome. Variability becomes even more extreme with smaller samples, with lower reliability, and with smaller underlying effect sizes. Again, the distribution of results from individual studies is not unlike that of data points within one study. These findings make us wonder about the interpretive value of individual studies, whether initial or replicated, especially when reliability is at the so-called acceptable level of .70 (see also Al-Hoorie & Hiver, Reference Al-Hoorie, Hiver, Al-Hoorie and Szabó2022).
Some approaches have been proposed to address this inferential indeterminacy. Some of these approaches rely on manipulation of variables across a range rather than just two levels (Scheel et al., Reference Scheel, T, Isager and Lakens2021) and on small-N within-study replications (Hiver & Al-Hoorie, Reference Hiver and Al-Hoorie2020b, Chap 16; Smith & Little, Reference Smith and Little2018). These approaches are particularly conducive to CDST research and involve built-in replication that draws from more intensive individual-level data collection (i.e., the individual as unit of analysis across time). Thus, a study involving five participants is equivalent to one initial study and four replications as the individual not the study is the replication unit (see Lowie, Reference Lowie, Ortega and Han2017). Clearly, inferences from such designs have higher power and more validity, a fact that has made disciplines adopting small-N paradigms more immune to perceptions of replication crises (Little & Smith, Reference Little and Smith2018). Furthermore, in complex topics, it would be very unusual to find an effect that is uniform across all participants. In contrast to the null hypothesis significance testing approach, which gives a yes–no answer (significant or non-significant), the ‘self-replicating’ feature of small-N designs provides a cumulatively richer picture by pointing out the pattern of replicability as well as the extent of its replicability with each participant. This level of transparency offers researchers greater input to formulate more complex models that explain observed phenomena.
Admittedly, though, not all fields can switch to data-intensive approaches. A popular alternative is adopting a meta-analytic mindset (e.g., Norris & Ortega, Reference Norris and Ortega2000) that helps avoid priming readers into ‘a competitive, score-keeping mentality (e.g., 2 failures vs. 1 success)’ (Brandt et al., Reference Brandt, IJzerman, Dijksterhuis, Farach, Geller, Giner-Sorolla, Grange, Perugini, Spies and van‘t Veer2014, p. 222). Nonetheless, traditional meta-analytic procedures are not without limitations. One limitation of a standard meta-analytic design is that it relies on secondary summary statistics (e.g., effect sizes) rather than the raw data directly. Recent advances in meta-analysis of raw data, coming from the emerging field of integrative data analysis, may help circumvent this limitation. Some of the advantages of meta-analyzing pooled raw data include the ease of studying extended developmental phenomena, rare phenomena and underrepresented subgroups that can be lost in summary statistics, and heterotypic continuity (i.e., changing manifestations of the same construct through time) (Bainter & Curran, Reference Bainter and Curran2015; Curran & Hussong, Reference Curran and Hussong2009; Hussong et al., Reference Hussong, Curran and Bauer2013).
A second limitation of standard meta-analytic designs is investigating heterogeneity, if found, through artificially separate, piecemeal moderator analyses. One approach to address this limitation is meta-regression, which models several (study-level) covariates simultaneously as well as estimating nonlinear relationships between covariates and effect sizes (Borenstein et al., Reference Borenstein, Hedges, Higgins and Rothstein2009). An additional approach is three-level meta-analysis (Pastor & Lazowski, Reference Pastor and Lazowski2018), which researchers can use to handle data dependences stemming from, for example, research by the same teams as well as data from the same participants, from participants with similar cultural backgrounds or contexts, from multiple time points or conditions, or from similar constructs (Cheung, Reference Cheung2015). Yet another approach is moderated nonlinear factor analysis models (Bauer & Hussong, Reference Bauer and Hussong2009), a confirmatory factor analytic model that permits the additional estimation of nonlinear relationships between latent factors and indicators measured with a mix of continuous, categorical, binary, and count items, in addition to allowing model parameters (e.g., item intercepts, factor loadings, mean and variance) to vary as a function of moderators. With insights from these advanced analytical tools, we wonder whether it makes sense to ask in CDST research about ‘the’ effect size of a phenomenon when different effect sizes should be expected to emerge almost by default in different contexts and under different contingencies.Footnote 6
Adoption of a meta-analytic mindset has several implications. First, individual studies—particularly those not drawing from intensive small-N designs—are seen merely as individual data points. Just as it is meaningless to try and interpret a single data point within an individual study and compare it with the next data point, it is equally meaningless to interpret the results of an initial study and compare it with one replication (Stanley & Spence, Reference Stanley and Spence2014). In other words, this mindset will ‘transform scientific experts from the producers of finished science to data farmers, producing grist for a meta-analytic mill’ (Freese & Peterson, Reference Freese and Peterson2018, p. 290) curating a continually accumulating set of findings. Not all data points are equal in quality, and since effect sizes from one-shot small-scale studies are too variable and noisy (Stanley & Spence, Reference Stanley and Spence2014), some commentators have argued that ‘there is a serious debate to be had about whether it is scientifically useful to conduct small-sample research at all’ (Yarkoni & Westfall, Reference Yarkoni and Westfall2017, p. 1110).
We would add that serious debate should also be had about whether it makes sense to require each individual study (which is merely a data point) to have a full-length discussion section. Especially with one-shot small-scale studies, asking authors to discuss results at length seems hardly different from asking them to discuss the implications of a single data point. This ‘McDonaldized’ journal article format could be seen as a waste of authors’ time and journal space—particularly as article length, number of references, and the age of these references have been empirically shown to increase in ‘softer’ sciences (Fanelli & Glänzel, Reference Fanelli and Glänzel2013). In contrast, an aspect that decreases in softer sciences is number of coauthors. Collaboration among more authors on one project facilitates drawing from different areas of expertise (Duff, Reference Duff2019; The Douglas Fir Group, 2016), sampling from multiple sites (Morgan-Short et al., Reference Morgan-Short, Marsden, Heil, Issa II, Leow, Mikhaylova, Mikołajczak, Moreno, Slabakova and Szudarski2018; Vitta & Al-Hoorie, Reference Vitta and Al-Hoorie2021), minimizing publication bias and enhancing inference quality, particularly when preregistered (Simons et al., Reference Simons, Holcombe and Spellman2014), and offering contextual, social, and cultural insights that are hard to reach otherwise (Hiver et al., Reference Hiver, Al-Hoorie and Larsen-Freeman2021b; Pettigrew, Reference Pettigrew2018).
4. Conclusion
Having explored such a range of ideas in our quest to chart a place for replication in CDST research, we agree that it would be productive for CDST to join the field's ongoing conversation about replication research. As Alexander and Moors (Reference Alexander and Moors2018) pointed out, many solutions to the replication crisis are decades old, and decades from now we will likely still be discussing reproducibility problems. Lack of exact, quantitative prediction is not a temporary state but an inherent feature of social science theories, reflecting the inherent complexity of the human and social world (Larsen-Freeman & Cameron, Reference Larsen-Freeman and Cameron2008). Instead of a narrow focus on replication per se, a more general attention to substantiation will help SLD theories mature, permit better replication designs, and improve reproducibility in the field.
Appendix
Simons et al. (Reference Simons, Shoda and Lindsay2017) provide a concrete example of a constraints-on-generality statement based on their research, which focuses on the willingness of participants to offer support to individuals expressing distress. Here is how they phrase their constraints-on-generality statement:
The stimuli consisted of a large number of video clips in which a large number of different undergraduates sampled from the subject pool at the University of Washington each expressed mild distress in their own way. Thus, we expect the results to generalize to situations in which participants view similar video clips, as long as manipulation checks indicate the clips depict a variety of ways in which people express mild distress. Unpublished studies from our laboratory resulted in similar results despite variations in the testing context (e.g., different research assistants). Consequently, we do not expect such variations to matter. We believe the results will be reproducible with students from similar subject pools serving as participants. However, we do not have evidence that the findings will occur outside of laboratory settings. The distress expressed in the video clips was triggered by a specific laboratory induction, and we lack evidence showing that the results will generalize to expressions of distress in response to other situations. We have no reason to believe that the results depend on other characteristics of the participants, materials, or context. (Simons et al., Reference Simons, Shoda and Lindsay2017, p. 1127)
Ali H. Al-Hoorie works at the Jubail English Language and Preparatory Year Institute, Royal Commission for Jubail and Yanbu, Saudi Arabia. He completed his Ph.D. in Applied Linguistics at the University of Nottingham under the supervision of Professors Zoltán Dörnyei and Norbert Schmitt. His research interests include motivation theory, research methodology, and complexity. His books include Research methods for complexity in applied linguistics (Multilingual Matters, 2020, with P. Hiver) and Student engagement in the language classroom (Multilingual Matters, 2021, coedited with P. Hiver and S. Mercer), and Contemporary language motivation theory: 60 years since Gardner and Lambert (1959) (Multilingual Matters, 2020, coedited with P. MacIntyre). The latter book is the winner of the Jake Harwood Outstanding Book Award.
Phil Hiver is an Assistant Professor in the School of Teacher Education at Florida State University. His published research focuses on the psychology of language learning and teaching and its interface with instructed language development and language pedagogy. He has also written on innovation and precision in research methods and the contribution of complex dynamic systems theory (CDST) to applied linguistics research. He is co-author of Research methods for complexity theory in applied linguistics (Multilingual Matters, 2020, with A. Al-Hoorie), and co-editor of the Routledge handbook of second language acquisition and individual differences (Routledge, 2022, with S. Li and M. Papi).
Diane Larsen-Freeman is Professor Emerita of Education and Linguistics, Research Scientist Emerita, and former Director of the English Language Institute at the University of Michigan. She is also Professor Emerita at the SIT Graduate Institute in Vermont and a Visiting Faculty Member at the University of Pennsylvania. Her recent books are Complex systems and applied linguistics (Oxford University Press, 2008, with L. Cameron), winner of the MLA's Kenneth Mildenberger Book Prize, the third edition of Techniques and principles (Oxford University Press, 2011, with M. Anderson), the third edition of The grammar book, form, meaning, and use for English language teachers (Heinle Cengage, 2015, with M. Celce- Murcia), and Second language development: Ever expanding (2018). Dr. Larsen-Freeman edited the journal Language Learning for five years, and later served as Chair of its Board of Directors.
Wander Lowie holds a Ph.D. in Applied Linguistics from the University of Groningen and is Chair of Applied Linguistics at this university. He is also a research associate of the University of the Free State in South Africa and is Associate Editor of Modern Language Journal. He was one of the co-organizers of the AILA2021 World Conference. His main research interest lies in the application of Complex Dynamic Systems Theory to second language development (learning and teaching), and is also interested in L2 phonology (especially prosody). He has published more than 70 articles and book chapters and (co-)authored six books in the field of Applied Linguistics.