1. Introduction
English-learning children sometimes make errors in which they overgeneralise verbs into ungrammatical sentence structures. For example, a child may use an intransitive-only verb, such as cry, in a transitive sentence, producing an ungrammatical utterance like *You just cried me (Bowerman, Reference Bowerman1981). These errors are called overgeneralisation errors because, while it is often appropriate to generalise a verb from one grammatical construction (e.g., intransitive – The ball rolled) to another (e.g., transitive – I rolled the ball), this process is possible with only certain verbs (so-called ‘alternating verbs’).
How children learn to restrict their generalisations to a subset of verbs is a key question in language acquisition because the answer throws light on the mechanisms by which children learn argument structure. For example, the entrenchment mechanism, in which children infer that a verb is ungrammatical in the target structure because it is used in other structures but never in that structure (Braine & Brooks, Reference Braine, Brooks, Tomasello and Merriman1995), predicts that children will make fewer errors with verbs that are heard more frequently as they will have received more evidence. A mechanism in which children use the semantics of a verb to define its argument structure privileges, inferring that a verb is ungrammatical in a structure because its semantics do not overlap with those of the construction (e.g., Pinker, Reference Pinker1989), predicts that verbs’ semantic properties will predict error rates. Finally, the preemption mechanism, in which children infer that a verb is ungrammatical because it is used with structures with similar meanings to that of the target structure, but never in the target structure itself (Goldberg, Reference Goldberg1995), e.g., You made me cry blocking *You cried me, predicts that children will make fewer errors with verbs that are heard more frequently in those competing structures, regardless of its overall frequency.
All three of these mechanisms – entrenchment, verb semantics, and preemption – have been proposed to explain how children retreat from overgeneralisation errors, and all have some evidence to support them (e.g., Theakston, Reference Theakston2004, on entrenchment; Gropen, Pinker, Hollander & Goldberg, Reference Gropen, Pinker, Hollander and Goldberg1991, on semantics; Brooks & Zizak, Reference Brooks and Zizak2002, on preemption). However, it has become increasingly clear that none of these explanations can account for all of the data. For example, neither the entrenchment nor the preemption hypotheses can account for the finding that children show semantically constrained argument structure preferences for completely novel verbs that have been assigned meanings either compatible or incompatible with those structures (e.g., Ambridge, Pine, Rowland, & Young, Reference Ambridge, Pine, Rowland and Young2008; Ambridge, Pine, Rowland, Jones, & Clark, Reference Ambridge, Pine, Rowland, Jones and Clark2009; Ambridge, Pine, Rowland, & Chang, Reference Ambridge, Pine, Rowland and Chang2012; Bidgood, Ambridge, Pine, & Rowland, Reference Bidgood, Ambridge, Pine and Rowland2014). Conversely, the semantic verb class hypothesis cannot explain why all of these studies also show effects of verb frequency (i.e., entrenchment and/or preemption). When taken together, these findings suggest the need for a model of how children retreat from argument-structure overgeneralisation that provides an integrated account of both semantic and statistical effects. They also suggest the need for studies that assess the relative contribution of semantic and statistical factors across a range of verbs and structures, so that the results of these studies can be used to inform the development of such a model.
Recent research has started to address this problem by developing designs that pit semantic and statistical factors against each other. For example, Ambridge, Pine, Rowland, Freudenthal, and Chang (Reference Ambridge, Pine, Rowland, Freudenthal and Chang2014) used a regression design to assess the predictions of the entrenchment, semantic verb class, and preemption hypotheses together against children’s and adults’ judgments of the acceptability of different verbs in prepositional-object (PO) and double-object (DO) datives (e.g., PO: Bart gave a present to Lisa; DO: Bart gave Lisa a present). While some verbs, like give, alternate between the two dative constructions, others are grammatical in only the PO dative (e.g., Bart said something to Lisa; *Bart said Lisa something) or the DO dative (e.g., The book cost Homer $5; *The book cost $5 to Homer).
Ambridge et al. (Reference Ambridge, Pine, Rowland, Freudenthal and Chang2014) asked participants to rate the acceptability of sentences of both types, containing PO-only, DO-only, or alternating verbs. Adults completed written questionnaires, whereas children (aged 5–6 and 9–10 years) heard sentences, for a smaller set of verbs, each accompanied by an animation, and gave their judgments using a 5-point ‘smiley-face’ scale. These ratings constituted the outcome variable. The predictor variables were corpus-based measures reflecting the relative frequencies of the verb in dative (preemption) and other constructions (entrenchment), and a set of semantic predictors calculated from adults’ ratings of each verb’s semantic properties (based on Pinker, Reference Pinker1989). In general, this study found evidence for effects of verb semantics, entrenchment, and preemption for all ages, though correlations between the entrenchment and preemption predictors meant that they could not be satisfactorily dissociated.
Ambridge, Barak, Wonnacott, Bannard, and Sala (Reference Ambridge, Barak, Wonnacott, Bannard and Sala2018) conducted a reanalysis and meta-analytic synthesis of the data from Ambridge et al. (Reference Ambridge, Pine, Rowland, Freudenthal and Chang2014) and analogous studies of the locative construction (Ambridge, Pine, & Rowland, Reference Ambridge, Pine and Rowland2012), the verbal un- prefixation construction (Ambridge, Reference Ambridge2013; Blything, Ambridge, & Lieven, Reference Blything, Ambridge and Lieven2014), and multiple constructions (Ambridge, Bidgood, Twomey, Pine, Rowland, & Freudenthal, Reference Ambridge, Bidgood, Twomey, Pine, Rowland and Freudenthal2015), all of which used a similar methodology, and the same age groups. This reanalysis was designed to address a number of inconsistencies and shortcomings with regard to the original statistical analyses (e.g., Wurm & Fisicaro, Reference Wurm and Fisicaro2014), and to incorporate measures that more accurately operationalise entrenchment and preemption: measures of verb-bias towards / away from particular constructions, based on the chi-square statistic, rather than simple frequency. This reanalysis found that, in single-predictor models – i.e., when entrenchment and preemption are investigated independently, rather than pitted against one another – effects of all three are ubiquitous for all age groups, within each study. Furthermore, effects of entrenchment and preemption are observed in a meta-analysis when collapsing across studies, even using simultaneous regression models that include both predictors as well as semantic predictors (though the semantic predictors could not be included in the meta-analysis themselves, as they were highly heterogeneous between constructions and studies). Within any one particular study, however, effects of entrenchment and preemption were generally (i.e., except for the un- prefixation studies) too highly correlated to allow them to be dissociated.
Although this reanalysis and meta-analysis constitutes important progress in the long-running debate over the acquisition of restrictions on verb argument structure generalisations, two questions remain. First, do these findings extend to overgeneralisations involving the English intransitive and transitive constructions? This question is an important one, since these constructions sit at the very core of the grammar, and are more frequent by an order of magnitude than those investigated in previous studies of this type (Ambridge et al., Reference Ambridge, Bidgood, Twomey, Pine, Rowland and Freudenthal2015, report subtitle-corpus frequencies of 1.6m and 0.4m for intransitive and transitive constructions, as compared to just 0.2m for the figure-locative, ground-locative, PO-dative, and DO-dative combined). In the present study (Experiment 1) we answer this question by applying the methods refined in Ambridge et al. (Reference Ambridge, Barak, Wonnacott, Bannard and Sala2018) to investigate the entrenchment, preemption, and verb-semantics hypotheses with regard to the English intransitive and transitive constructions. Here, errors occur when intransitive-only verbs (e.g., sing, sweat, cry) are used in the transitive construction (e.g., *I’m singing him [pulling string to activate a cow-shaped music box]; *It always sweats me [doesn’t want to wear hot sweater]; *You just cried me [i.e., made me cry]; Bowerman, Reference Bowerman1981) or vice versa (*I better put it down there so it won’t lose [i.e., won’t get lost]; Lord, Reference Lord1979). Since previous studies have focused on constructions that are fairly limited in terms of their variability, including the number of verbs that can be used in those constructions (dative/locative), replication of this method with the much more variable and productive transitive/intransitive constructions is valuable.
The second question is whether the same results hold for production. This remains unaddressed by previous studies that combine entrenchment, preemption, and verb semantics in a single design, all of which rely solely on grammaticality-judgment methodologies. This is an important question, since, ultimately, what we are trying to explain is how children learn to stop making errors in production. Nevertheless, it is very difficult to investigate semantic and statistical factors in spontaneous data since these errors are both fairly rare and potentially subject to experimenter bias (diary studies may be more likely to record only the most salient errors, for example). A secondary goal of the present study is therefore to begin to develop a production methodology that can be used to investigate verb argument structure overgeneralisation errors (Experiment 2); if we are able to elicit errors, we will be able to use the frequency of these errors with different verbs to test the predictions of the entrenchment, semantics, and preemption accounts in production. Our starting point is a production priming methodology that has been frequently used to investigate children’s knowledge of syntax (see Branigan & Pickering, Reference Branigan and Pickering2017, for a review), and recently adapted to investigate morphological overgeneralisation errors (Blything et al., Reference Blything, Ambridge and Lieven2014). However, this method has not, to our knowledge, previously been used to induce verb argument structure overgeneralisation errors. Our approach, then, is to validate these production data by comparing them to comparable data observed using a judgment methodology (Experiment 1) that has been refined and verified across 16 previous studies.
2. General methods: creating the predictor variables
Before describing the individual experiments, we first outline the methods used to create the predictor variables that relate to the entrenchment, preemption, and semantics hypotheses (used across the judgment and production studies).
2.1. verbs
The test items were 40 transitive-only verbs, 40 intransitive-only verbs, and 40 verbs that can alternate between the two structures (based on Pinker, Reference Pinker1989, and Levin, Reference Levin1993). Note that these classifications were used only to select the verbs; none of the analyses use verb type as a categorical predictor, and instead use continuous measures of verb bias. A list of all of the verbs used can be found in Appendix A of the supplementary materials (available at: http://doi.org/10.1017/langcog.2021.8).
2.1.1. Frequency counts for preemption and entrenchment measures
In order to operationalise the predictions of the entrenchment and preemption hypotheses, verb frequency counts were taken from the British National Corpus (BNC, 2007). Although this corpus is not representative of speech to children, it is much larger (100 million words) than available corpora of child-directed speech. In the studies reviewed in Ambridge et al. (Reference Ambridge, Barak, Wonnacott, Bannard and Sala2018), frequency counts from this and other adult corpora were better predictors of children’s performance than counts obtained from corpora of child-directed speech, due to the fact that the latter are considerably smaller (with many stimulus verbs not appearing at all) and hence potentially noisier. Thus, we consider the BNC an appropriate corpus to use here. A custom script (written by the fifth author) was used to obtain counts of overall uses of the relevant lexical item tagged as VERB, and to extract candidate transitives (NP VERB NP), intransitives (NP VERB), passives (NP BE VERBed/en), and periphrastic causatives (NP MAKE NP VERB). Because this process yields a large number of false positives, for each verb a randomly selected sample, from all uses extracted from the corpus for that construction, of 100 transitive, 100 intransitive, 100 passive, and 100 periphrastic causative uses (or if < 100, all uses) was hand-coded (by the first author). This allowed us to estimate the proportion of false positives in the sample as a whole. The full sample was then pro-rated accordingly to yield the final estimate.
Following Stefanowitsch (Reference Stefanowitsch2008; see also Stefanowitsch & Gries, Reference Stefanowitsch and Gries2003, and Gries & Stefanowitsch, Reference Gries and Stefanowitsch2004) and Ambridge et al. (Reference Ambridge, Barak, Wonnacott, Bannard and Sala2018), we then used these corpus counts to create (log transformed) chi-square predictors (note that we use the chi-square values themselves, not the associated p values) that operationalise preemption and entrenchment in terms of each verb’s relative bias towards each target construction and away from (preemption) the closest competing construction and (entrenchment) all constructions (though excluding uses already counted towards preemption). In each case, the sign of the predictor is set to positive if, relative to the other verbs in our verb set, the verb is biased towards the target construction, and to negative if it is biased away from the target construction.
The entrenchment hypothesis (e.g., Braine & Brooks, Reference Braine, Brooks, Tomasello and Merriman1995) posits an inference-from-absence mechanism to explain children’s retreat from overgeneralisation errors: the more a verb is heard in the input, without being heard in the ungrammatical construction, the stronger the inference that that verb–construction pairing must not be possible. Thus, the entrenchment hypothesis predicts that the more a verb has been heard regardless of the construction, the less acceptable it will be in ungrammatical sentences, and the less likely children will be to produce an error with that verb. In most of the previous studies reviewed above, entrenchment has therefore been operationalised simply as overall verb frequency, and calculated only for so-called ‘non-alternating’ verbs (here, intransitive-only or transitive-only verbs). However, as Ambridge et al. (Reference Ambridge, Barak, Wonnacott, Bannard and Sala2018) note, this operationalisation is problematic, because the stipulation ‘without being heard in the ungrammatical construction’ draws an arbitrary line in the sand, ignoring the reality that grammatical acceptability is graded: few verbs are entirely unattested in a particular ‘ungrammatical’ construction, and many verbs that are described as ‘alternating’ between two constructions often show a bias for one over the other that is very similar to that shown by ‘non-alternating’ verbs. Here, we therefore follow Stefanowitsch (Reference Stefanowitsch2008) and Ambridge et al. (Reference Ambridge, Barak, Wonnacott, Bannard and Sala2018) in operationalising entrenchment using the chi-square statistic as a measure of relative bias, as explained in more detail below.
The preemption hypothesis (e.g., Goldberg, Reference Goldberg1995), while related to the entrenchment hypothesis, adds a semantic element: the more a verb is heard in constructions with a roughly equivalent meaning to the ungrammatical construction, the stronger the inference that the ungrammatical verb–construction pairing must not be possible. Thus, the preemption hypothesis predicts that the more a verb is heard in a competing construction with similar meaning, the less acceptable it will be in the relevant target construction, and the less likely children will be to produce this type of error with that verb. To test this account, for intransitive utterances (e.g., *The ball kicked), we followed Brooks and Tomasello (Reference Brooks and Tomasello1999) and designated the passive (e.g., The ball was kicked [by X], including both full and truncated passives) as the competing construction: like the intransitive construction, the passive construction puts the discourse focus on the patient by placing it first in the sentence (e.g., The plate broke; The plate was broken [by Homer]). The truncated passive also allows the sentence to exclude the agent altogether, as in the intransitive. In our corpus data, the majority of passive sentences were truncated (92.15%). Thus, the passive uses of these verbs were very similar to intransitive uses. For transitive utterances (e.g., *The man laughed the girl), again following Brooks and Tomasello (Reference Brooks and Tomasello1999), we designated the periphrastic causative (e.g., The man made the girl laugh) as the competing construction, since this construction expresses a similar meaning to the transitive, and overtly expresses both agent and patient.
As with entrenchment, the majority of previous studies reviewed above operationalised preemption using a raw-frequency measure (i.e., frequency in the grammatical construction of the pair) and ignored so-called alternating verbs. But, again, this draws an arbitrary line in the sand between dispreferred uses that are ‘ungrammatical’, though sometimes attested, and those that are ‘grammatical’, though attested relatively rarely. We therefore follow Stefanowitsch (Reference Stefanowitsch2008) and Ambridge et al. (Reference Ambridge, Barak, Wonnacott, Bannard and Sala2018) in operationalising both preemption and entrenchment using the chi-square statistic as a measure of relative bias.
For preemption, the chi-square statistic reflects the extent to which the bias of a particular verb (e.g., laugh) with respect to two competing constructions (e.g., the transitive vs. the periphrastic causative: e.g.,*The man laughed the girl vs. The man made the girl laugh) is similar to the bias shown by other verbs (here, the other verbs in our stimulus setFootnote 1) with respect to these two constructions. Unlike a version based on raw-frequency, this operationalisation of preemption allows us to calculate this measure for all verbs – ‘alternating’ and ‘non-alternating’ alike – while taking into account the frequency of a particular verb in both the preferred and dispreferred construction of the pair. Unlike a raw frequency measure, it also factors in the base-rate of the two constructions. For example, given that the transitive is, for verbs in general, approximately 800 times more frequent than the periphrastic causative (at least in the present corpus counts), a verb like boil that is ‘only’ 52 times more frequent in the transitive than periphrastic causative in fact shows a relatively strong bias towards the periphrastic causative. Conversely, a verb like destroy, that occurs over 4,000 times in the transitive causative but never the periphrastic causative, shows a strong bias for the former.
For each verb, two preemption predictors were calculated, reflecting each verb’s bias (relative to all other verbs in our verb set) for (a) the transitive versus periphrastic causative construction (e.g., *The man laughed the girl vs. The man made the girl laugh; Someone destroyed the city vs. *Someone made the city destroy), and (b) the intransitive versus passive construction (e.g., *The city destroyed vs. The city was destroyed; The girl laughed vs. *The girl was laughed). Tables 1 and 2 illustrate the calculation of transitive-vs.-periphrastic preemption predictor for laugh, and the intransitive-vs.-passive preemption predictor for destroy. The Pearson chi-squared statistic (without Yates’ correction) was calculated according to the standard formula below.

Because
table 1. Calculation of the transitive-vs.-periphrastic preemption measure for the verb laugh

(31*483-101*477905)2 * (31+101+477905+483)= 61713.26
(31+477905)*(101+483)*(31+101)*(477905+483)
Natural log (1+61713.26) = 11.03
Preemption predictor value = –11.03 (bias away from transitive and towards periphrastic)
table 2. Calculation of the intransitive-vs.-passive preemption measure for the verb destroy

(9*6176-239*667300)2 * (9+239+667300+6176) = 24012.45
(9+667300)*(239+6176)*(9+239)*(667300+6176)
Natural log (1+24012.45) = 10.09
Preemption predictor value = –10.09 (bias away from intransitive and towards passive)
the chi-square test is non-directional, it was necessary (as in Stefanowitsch, Reference Stefanowitsch2008; Ambridge et al., Reference Ambridge, Barak, Wonnacott, Bannard and Sala2018) to set the sign of each predictor to positive (/negative) if, relative to other verbs in the set, the verb in question was biased towards (/ away from) (a) the transitive (vs. periphrastic) construction and (b) the intransitive (vs. passive) construction. As is standard practice for frequency-based measures, the chi-square statistic was natural log (N+1) transformed before its sign was assigned.
The entrenchment predictor was calculated in a similar way, though, for each verb, two different calculations were necessary: (a) entrenchment towards (+) / away from (–) the transitive construction (for use as the entrenchment predictor for trials in which this construction was rated or elicited), and (b) entrenchment towards (+) / away from (–) the intransitive construction (for trials in which this construction was rated or elicited). The major difference is that non-target uses (i.e., the two rightmost cells of the chi-square) were defined not as uses in a specific competing construction (as was the case for the preemption predictor), but rather as all other uses, except those already counted towards the preemption predictor. For example, when calculating (a) entrenchment towards (+) / away from (–) the transitive construction for laugh (see Table 3), we excluded the 101 periphrastic causative uses already counted towards the preemption predictor. Table 4 illustrates (b) entrenchment towards (+) / away from (–) the intransitive construction for laugh.
table 3. Calculation of the transitive-sentence-target entrenchment measure for the verb laugh (+ = bias towards transitive, – = bias away from transitive)

(31*1121630-8115*466905)2 * (31+8115+466905+1121630) = 3296.20
(31+466905)*(8115+1121630)*(31+8115)*(466905+1121630)
Natural log (1+3296.20) = 8.10
Entrenchment predictor value = –8.10 (bias away from transitive and towards non-transitive)
table 4. Calculation of the intransitive-sentence-target entrenchment measure for the verb laugh (+ = bias towards intransitive, – = bias away from intransitive)

(7173*922468-1074*660135) 2 * (7173+1074+660135+922468) = 6903.39
(7173+660135)*(1074+922468)*(7173+1074)*(660135+922468)
Natural log (1+6903.39) = 8.84
Entrenchment predictor value = 8.84 (bias towards intransitive and away from non-intransitive)
This decision was taken in order to ensure parity with the studies analysed in Ambridge et al. (Reference Ambridge, Barak, Wonnacott, Bannard and Sala2018), which is important for meta-analysis, and (as in this previous study) to minimise the correlation between the preemption and entrenchment measures (though in practice they remain highly correlated, presumably because verbs that are (in/)frequent in a given construction tend to be (in/)frequent across the board). However, as a result, this predictor represents a departure from a pure entrenchment predictor (which would require calculating the predictor on the basis of all uses). Rather, it tests a specific prediction of the entrenchment hypothesis: that attested occurrences of a particular verb will contribute to the perceived ungrammaticality of attested uses, even when the two are not in competition for the same message (i.e., even when potentially preempting uses are excluded from the calculation). That said, for the present study, the departure from a pure entrenchment predictor is negligible, since the excluded constructions (periphrastic causative and passive) are extremely infrequent relative to other uses (e.g., transitive, intransitive, single-word fragment).
2.1.2. Semantic ratings
Under the semantics hypothesis, verb semantics determine the permissible constructions for a particular verb, including the transitive and intransitive. The greater the overlap between the semantics of a particular verb and the semantics of the construction itself, the greater the acceptability of the resulting utterance (e.g., Pinker, Reference Pinker1989; Ambridge et al., Reference Ambridge, Barak, Wonnacott, Bannard and Sala2018). Children’s errors therefore reflect, at least in part, a failure to master the semantics of the verb and/or the construction, and disappear as this knowledge is refined. The approach taken by older semantics-based accounts (e.g., Pinker, Reference Pinker1989; Levin, Reference Levin1993) was to identify discrete semantic classes of verbs that are semantically consistent with particular constructions. In line with more recent work (e.g., the studies summarised in Ambridge et al., Reference Ambridge, Barak, Wonnacott, Bannard and Sala2018), we treated construction-consistent verb semantics as a continuum and created an objective measure of verb semantics by conducting a semantic rating task.
This raises the question of how to characterise the semantics of the English intransitive and transitive constructions. A difficulty here is that both of these constructions are highly semantically heterogeneous. However, a general consensus (e.g., Hopper & Thompson, Reference Hopper and Thompson1980; Næss, Reference Næss2007) is that the intransitive construction is prototypically associated with a single participant undergoing an internally caused action (e.g., The girl laughed), while the transitive construction is prototypically associated with an event in which an agent deliberately causes an affected patient to undergo some kind of change (e.g., The girl killed the fly). We operationalised this notion of intransitive- versus transitive-consistent verb semantics by asking participants to rate verbs along Shibatani and Pardeshi’s (Reference Shibatani and Pardeshi2002) causative continuum. Applying this account to English, the main difference between semantically intransitive and semantically transitive verbs is the manner in which the relevant action can be caused:
For semantically intransitive verbs (e.g., laugh), causation entails an event in which “both the causing and the caused event enjoy some degree of autonomy … The caused event … may have its own spatial and temporal profiles distinct from those of the causing event.”
For semantically transitive verbs (e.g., kill), causation “entails a spatiotemporal overlap of the causer’s activity and the caused event, to the extent that the two relevant events are not clearly distinguishable” (Shibatani & Pardeshi, Reference Shibatani and Pardeshi2002, p. 89)
We therefore obtained ratings for verbs on this event-merge measure. It is important to bear in mind that this measure does not capture the full range of intransitive- versus transitive-consistent semantics in English, given the existence of, for example, transitive utterances that suggest but do not entail causation (e.g., The man kicked the ball), and those that do not denote causation at all (e.g., Food costs money). At the same time, given the general agreement regarding the prototypical semantics of these constructions, we anticipated that this event-merge measure would serve as a reasonable approximation of intransitive- and transitive-consistent verb semantics (and, indeed, the present findings bear this out).
2.2. participants
The participants for this semantic rating task were 20 adults aged 18–25, all undergraduate students at the University of Liverpool. They were each paid £10 for their participation. All participants were monolingual speakers of English, and had no known language impairments. They did not take part in the other experiments reported in this paper. The test items were the 120 verbs chosen for use in the main studies (see Appendix A).
2.3. method
Participants rated each verb on this event-merge measure on a visual analogue scale, presented using PsychoPy 2.0 (Pierce, Reference Peirce2009). Participants were told:
You will see 120 videos in which a PERSON/THING carries out/undergoes an ACTION/EVENT/CHANGE. This ACTION/EVENT/CHANGE is caused by another PERSON/THING.
An animation (created using Anime Studio Pro 5.5) was then shown (in each case, one of the same animations used in the subsequent judgment and production studies), accompanied by the following text (at the top of the screen):
Here, A (the CAUSER) causes B (the PERSON/THING) to carry out/undergo an ACTION/EVENT/CHANGE. We are interested in the extent to which A causing the ACTION/EVENT/CHANGE and B undergoing the ACTION/EVENT/CHANGE are separate. Please rate the extent to which …
Displayed below the animation was a single visual analogue scale with the following anchors:
(Left) B’s ACTION/EVENT/CHANGE and A’s causing of it are two separate events, that could happen at different times and/or in different points in space.
(Right) B’s ACTION/EVENT/CHANGE and A’s causing of it merge into a single event that happens at a single time and a single point in space.
Participants clicked on the scale to make their judgments. This procedure was repeated for all 120 trials (presented in random order). The semantic rating for each verb was created simply by taking the mean rating (converted into a 100-point scale) across all participants. Note that, due to the inclusion of animations, this rating task was not a measure of each verb’s global semantics, but rather a measure of the event semantics associated with each verb in a particular scene. This is appropriate, given that (a) the same animations accompanied the sentences during the subsequent judgment and production tasks, and (b) semantic accounts assume that the acceptability of a particular utterance is a function of the understood semantics of the unfolding action/event referred to by the verb, rather than the verb’s global semantics (e.g., Pinker, Reference Pinker1989; Ambridge et al., Reference Ambridge, Pine, Rowland, Jones and Clark2009). Indeed, Ambridge et al. (Reference Ambridge, Pine, Rowland, Jones and Clark2009) showed that manipulating event semantics, while holding the verb constant, significantly affects the rated acceptability of transitivisation errors.
The entrenchment, preemption, and semantic rating measures described above were used as predictor variables in the judgment and production studies described below.
3. Experiment 1: grammaticality judgments with adults and children
3.1. method
3.1.1. Participants
The participants were 96 children aged 5–6 years old (5;3–6;5, M=5;10), 96 children aged 9–10 years old (9;4–10;6, M=9;11), and 24 adults aged 18–25 years old. Four times as many children as adults were required (in each age group) as each child completed only ¼ of the total number of adult trials. The children were recruited from primary schools in the North West of England. The adults were all undergraduate students at the University of Liverpool, and received course credit for their participation. All participants were monolingual speakers of English, and had no known language impairments.
3.1.2. Test items
For each of the 120 verbs, transitive and intransitive sentences were created as follows:
Marge/Bart/Lisa/Homer [VERBed] the [object/person/animal] [modifying phrase]
e.g., Lisa dropped the ball to the floor
The [object/person/animal] [VERBed] [modifying phrase]
e.g., The ball dropped to the floor
The arguments of the intransitive and transitive sentence for each verb were always matched in this way. The transitive and intransitive sentences for each verb were recorded by the final author, a native speaker of British English. Identical animations (the same as those used for the semantic rating task) were used for the transitive and intransitive versions of each sentence. The use of animations aimed to ensure that the veracity of the sentences would not be in doubt, and therefore that participants’ judgments would be more likely to be based on the grammaticality of the sentences only, something that we have previously found to be important when testing young children (e.g., Ambridge et al., Reference Ambridge, Pine, Rowland and Young2008).
3.1.3. Procedure
Test sentences and their accompanying animations were presented to participants using VLC Media Player. Grammaticality judgments were given on a 5-point smiley-face judgment scale (see, e.g., Ambridge et al., Reference Ambridge, Pine, Rowland and Young2008), shown in Figure 1.

Fig. 1 The smiley face scale used by adult and child participants to rate sentences for grammatical acceptability. Reprinted from Cognition, 106(1), Ambridge, B., Pine, J. M., Rowland, C. F. & Young, C. R. (Reference Ambridge, Pine, Rowland and Young2008) The effect of verb semantic class and verb frequency (entrenchment) on children’s and adults’ graded judgements of argument-structure overgeneralisation errors, 87–129, Copyright (2008), with permission from Elsevier.
Adults watched the full set of animations, in a pseudo-random order such that no two sentences containing the same verb were presented consecutively, in small groups of up to 10 participants. Adults marked their responses (individually) on an answer sheet containing one smiley-face scale for each sentence. Due to constraints on attention span, children were tested individually on one quarter of the sentences each (60 in total), split over two days. Each child was tested on transitive and intransitive versions of sentences containing 10 each of transitive-only, intransitive-only, and alternating verbs. Sentences were again presented in a pseudo-random order. Children gave their responses by placing a green or red counter (indicating broadly grammatical or broadly ungrammatical, respectively) onto a single, larger smiley-face scale. They were instructed to choose the green counter if the sentence ‘sounded good’ and the red one if it ‘sounded silly’. They then placed the counter on the scale to indicate how ‘good’ or ‘silly’ it sounded. The experimenter noted down responses by hand.
3.1.4. Statistical analysis
Following Ambridge et al. (Reference Ambridge, Barak, Wonnacott, Bannard and Sala2018), we conducted three sets of analyses with dependent variables of (a) ratings for transitive sentences, (b) ratings for intransitive sentences, and (c) by-participant difference scores reflecting preference for intransitive over transitive uses (i.e., b minus a). Results were analysed in RStudio (version 1.3.959; R version 4.0.2; R Core Team, 2020). Mixed effects regression models were conducted using the lme4 package (version 1.1-23; Bates, Maechler, Bolker, & Walker, Reference Bates, Maechler, Bolker and Walker2015). The predictor variables were the continuous preemption, entrenchment, and verb-semantic measures, scaled into SD units and centred at zero, to allow for comparison with the previous datasets reanalysed in Ambridge et al. (Reference Ambridge, Barak, Wonnacott, Bannard and Sala2018). In terms of model structure, we follow Barr, Levy, Scheepers, and Tily (Reference Barr, Levy, Scheepers and Tily2013) in employing random intercepts for participant and item, and by-participant random slopes for the predictor variables, following a stepwise removal procedure in the event of convergence failure. We first report separate analyses by age group, and subsequently investigate developmental trends with a final set of models that include age group and its interactions with each of the predictor variables.
Technically, linear models require the dependent variable to be interval or ratio scaled, rather than ordinal scaled as in the present case. Nevertheless, the use of linear models with Likert-scale data is common because the intention – although we have no way of knowing whether this in fact the case – is that participants will indeed treat the rating scale as, in effect, interval scaled. In order to check that the present rating-scale data did not depart too far from this assumption, we used the R package ggResidpanel (version 0.3; Goode & Rey, Reference Goode and Rey2019) to create normal QQ plots, histograms, and scale-location plots to verify that the residuals for each model were indeed approximately normally distributed and did not show excessive homoscedasticity. These plots are not shown here, but can be recreated using the code available at <https://osf.io/xw934/>.
A high degree of collinearity between the preemption and entrenchment predictors was observed: r=0.71, r=0.74, and r=0.86 for the datasets containing ratings of transitive sentences, intransitive sentences, and difference scores, respectively. As in Ambridge et al. (Reference Ambridge, Pine, Rowland and Young2008), we address this collinearity in two ways. First, in addition to simultaneous mixed effects regression models, we also report single-predictor models. Second, we do not report final models (since the coefficients of individual predictors in simultaneous models are misleading under collinearity), but only the outcome of likelihood ratio tests comparing a full model to a model with the predictor of interest removed (Barr et al., Reference Barr, Levy, Scheepers and Tily2013), implemented using the drop1 command of the lme4 package. Since this test is non-directional, the direction of each effect is inferred from the single-predictor model (a changed direction between single-predictor and simultaneous models is not interpretable in the event of collinearity).
Together, these precautions ensure that the present analysis constitutes the best test of preemption and entrenchment that can be conducted with natural language data (in which the two measures are inevitably highly correlated). Nevertheless, given the very high correlation between the two, and the likelihood of residual confounding (see Westfall & Yarkoni, Reference Westfall and Yarkoni2016), any apparent effect of preemption beyond entrenchment, or vice versa, should be interpreted with extreme caution. Ultimately, to address the theoretical question of whether entrenchment, preemption, or both are used in children’s retreat from overgeneralisation errors will probably require artificial-language-learning studies in which the two mechanisms are systematically de-confounded (a project that we are currently undertaking; see also Boyd & Goldberg, Reference Boyd and Goldberg2011; Perek & Goldberg, Reference Perek and Goldberg2017).
All data and code are available at the following publicly accessible repository: <https://osf.io/xw934/>.
3.2. results and discussion
The relationship between the continuous preemption, entrenchment and verb semantic measures and ratings for transitive and intransitive sentences are plotted in Figures 2–4, and for difference scores in Figures 5–7.

Fig. 2a Relationship between preemption and judgments of transitive sentences (raw scores).

Fig. 2b Relationship between preemption and judgments of intransitive sentences (raw scores).

Fig. 3a Relationship between entrenchment and judgments of transitive sentences (raw scores).

Fig. 3b Relationship between entrenchment and judgments of intransitive sentences (raw scores).

Fig. 4a Relationship between verb semantics and judgments of transitive sentences (raw scores).
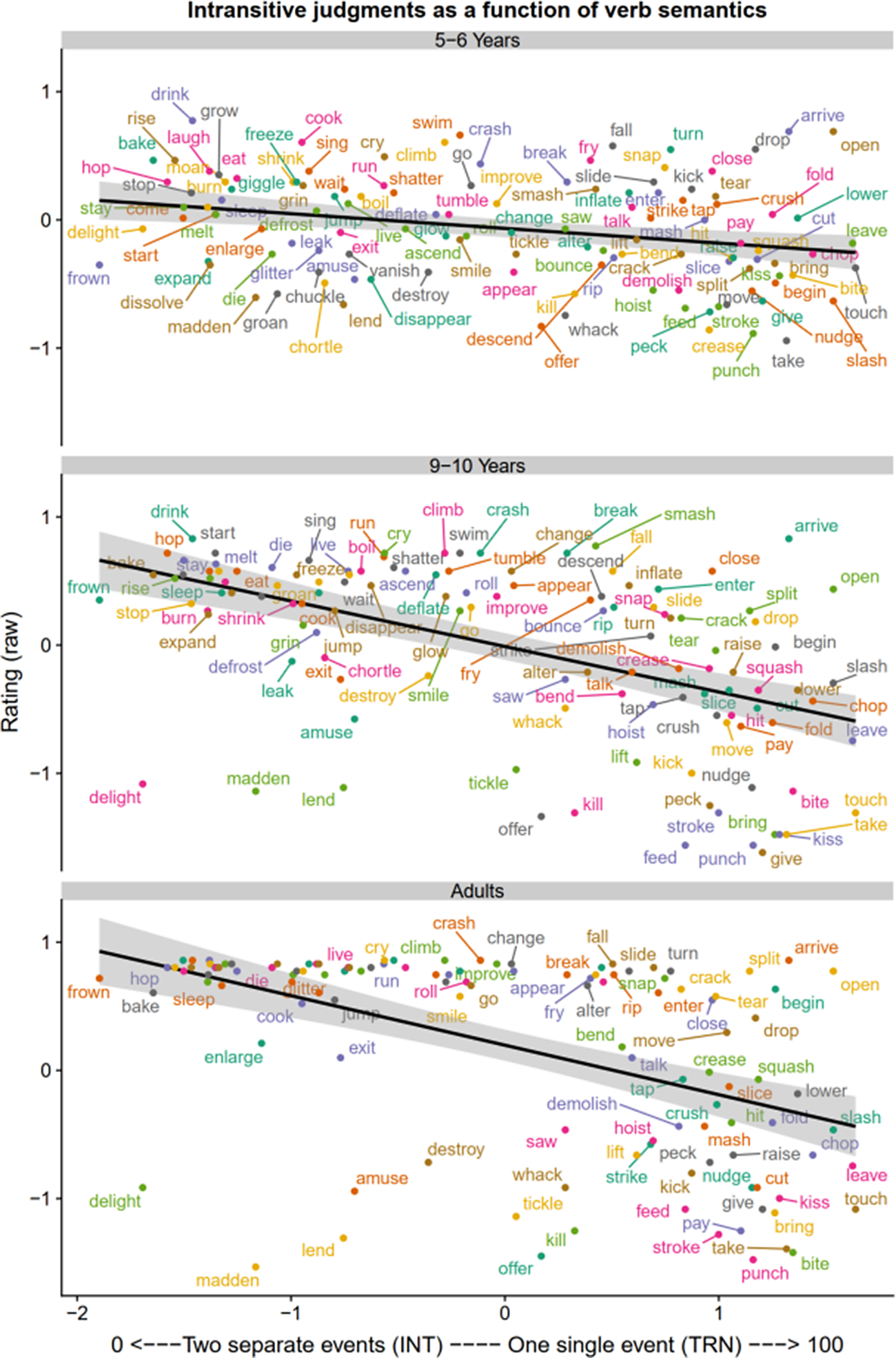
Fig. 4b Relationship between verb semantics and judgments of intransitive sentences (raw scores).

Fig. 5 Relationship between preemption and transitive-minus-intransitive difference scores.

Fig. 6 Relationship between entrenchment and transitive-minus-intransitive difference scores.

Fig. 7. Relationship between verb semantics and transitive-minus-intransitive difference scores.
For the single-predictor models (see Appendix B of the supplementary materials), all with maximal random effects structure, all three predictors were significant, with large chi-square values, for all ages (5–6, 9–10, adults) and all sentence types (transitives, intransitives, difference scores). This echoes the pattern reported by Ambridge et al. (Reference Ambridge, Barak, Wonnacott, Bannard and Sala2018) for the locative, dative, and various constructions.
For the simultaneous models (see Appendix B), it was not possible to use fully maximal random effects structure, but, following Ambridge et al. (Reference Ambridge, Barak, Wonnacott, Bannard and Sala2018), we achieved near-maximal converging models that were identical in structure for all age groups simply by disallowing correlations between random effects (using the double bar lme4 syntax), for example:
Age5TRN = lmer(Rating ∼ (1+PRE_FOR_TRN+ENT_FOR_TRN+Semantics || Participant) + (1|Verb) + PRE_FOR_TRN + ENT_FOR_TRN + Semantics, data=subset(TRN, AgeGroup=="Age5"), REML=F)
drop1(Age5TRN, test = "Chisq")
Compared with the single-predictor models, the ubiquitous effect of verb semantics remained across all simultaneous models, but effects of preemption and entrenchment played off against one another, such that, at most, one or the other – never both – was significant in a particular model. However, it is important to emphasise that, given the high degree of collinearity between these predictors, one should not attempt to interpret the pattern of significant and non-significant preemption and entrenchment effects across models and age groups.
Finally, we conducted a developmental analysis to investigate whether, as suggested by Figures 2–7, the observed effects of preemption, entrenchment, and semantics increase with age. Because simultaneous models are difficult to interpret given collinearity, this was done for single-predictor models only. The model comparison method (e.g., Barr et al., Reference Barr, Levy, Scheepers and Tily2013) was used to determine whether adding the interaction of the relevant predictor with age improved coverage of the relevant single predictor model. This was found to be the case for preemption, entrenchment, and semantics (see rightmost columns of the table in Appendix B). However, caution is required here, because it is impossible to tell whether this developmental pattern reflects genuine increased effects of preemption, entrenchment, and verb semantics with age (as would be predicted by these theories), or simply improved performance on the judgment task itself.
In summary, for all ages, for judgments of transitives, intransitives, and difference scores, the data show effects of semantics and statistics (preemption or entrenchment, but we cannot say which, due to collinearity). This exactly mirrors the pattern observed by Ambridge et al. (Reference Ambridge, Barak, Wonnacott, Bannard and Sala2018) for the locative, dative, and various constructions.
3.3. updated meta-analysis
Given that the present judgment study used the same age groups, methods, and operationalisations of preemption and entrenchment as the studies summarised in the meta-analytic synthesis reported in Ambridge et al. (Reference Ambridge, Barak, Wonnacott, Bannard and Sala2018), we updated this synthesis to reflect these new findings (semantic predictors are not included, since these are highly heterogeneous between studies). Updating the meta-analysis also allowed us to consider differences between constructions and age groups with regard to the size of the observed effects of preemption and entrenchment, which we did by including these factors as potential moderators in the meta-regression models.
The meta-analysis is available at <https://osf.io/r9azw/>. In summary, the results show that the previously observed meta-analytic effects of both preemption and entrenchment hold with the introduction of the present findings. Analysis of true heterogeneity suggests that the effect of preemption (but not entrenchment) varies by construction, with the transitive and intransitive showing middling effect sizes (smaller than for the locative constructions, but larger than for the dative constructions). There was also some evidence to suggest that both preemption and entrenchment effects increase with age, as would be predicted, given that these are developmental processes. However, this finding is also consistent with the possibility that older participants are simply better able to complete the judgment task.
4. Experiment 2: production-priming in five- to six-year-old children
In Experiment 2, we used a priming methodology to attempt to elicit verb argument structure overgeneralisation errors from five- to six-year-old children. Because this method has not, to our knowledge, been previously used to induce such errors, it requires validation. This will be accomplished by comparing the findings to those observed in Experiment 1, which used a paradigm (grammaticality judgments) that is well established in this domain.
4.1. method
4.1.1. Participants
The participants were 64 children aged 5–6 years old (5;2–6;4, M=5;8) recruited from primary schools in the North West of England. All were monolingual speakers of English and had no known language impairments. None of these children had participated in Experiment 1.
4.1.2. Materials
Test items were the same as in Experiment 1, with the addition of a single alternating verb (float, produced by the experimenter only), added for the purposes of the bingo game described below. The 120 verbs used in Experiment 1 (40 each of transitive-only, intransitive-only, and alternating verbs, according to their Pinker/Levin classifications) were split into four sets, each containing 20 alternating verbs and 10 each of the transitive-only and intransitive-only verbs. Alternating verbs were therefore used twice as many times in total as fixed-transitivity verbs, since they were used in both priming conditions. Each child received a single verb set for their target verbs. The experimenter used 20 each of the remaining non-alternating verbs for the prime sentences.
4.1.3. Procedure
The aim of this experiment was to encourage children to produce both intransitivisation errors with transitive-only verbs (e.g., *The ball kicked; cf. Homer kicked the ball) and transitivisation errors with intransitive-only verbs (e.g., *Homer swam the fish; cf. The fish swam). In order to do so, we used a production-priming methodology in which an experimenter produced (a) grammatical intransitive sentences to encourage the child to produce intransitivisation errors with transitive-only verbs and, on a separate day, (b) grammatical transitive sentences to encourage the child to produce transitivisation errors with intransitive-only verbs. In order to encourage use of the target verb, a second experimenter gave both the child and the first experimenter ‘clue words’ to help them describe the animation. Examples of trials in each prime condition are given below, with the target error we were attempting to elicit. Note that all animations included both an agent and a patient, in order to ensure that, in all cases, descriptions using both intransitive and transitive sentence structures were, in principle, possible.
-
(a) Intransitive prime condition (transitive-only target verbs)
-
Experimenter 2 (clue words): lightbulb, glow [animation: Bart turns on a lightbulb]
-
Experimenter 1: The lightbulb glowed
-
Experimenter 2 (clue words): ball, hit [animation: Homer hits a ball]
-
Child: *The ball hit
-
(b) Transitive prime condition (intransitive-only target verbs)
-
Experimenter 2 (clue words): bring, letter [animation: Lisa brings letter]
-
Experimenter 1: Lisa brought the letter
-
Experimenter 2 (clue words): laugh, girl [animation: Bart makes girl laugh girl]
-
Child: *Bart laughed the girl
As these examples show, no content words were shared between the prime and target sentence, ensuring that children could not describe their own animation simply by repeating part of the experimenter’s prime sentence.
Each child participated on two occasions, on separate days. In each session, children took turns with an experimenter to describe a series of animations. These animations were presented using Processing <www.processing.org>. Both experimenter and child were given ‘clue words’ by a second experimenter to encourage them to use the intended verb. The clue words consisted of the verb followed by the direct object, when transitive sentences were being primed, or the subject followed by the verb, when intransitive sentences were being primed. The second experimenter noted down children’s responses, although all sessions were also audio-recorded using Audacity in order to check responses later if there was any doubt about what the child had said.
Half of the children received transitive primes on the first day and intransitive primes on the second, and vice versa for the other children. The first three pairs of animations were training trials containing only transitive-only or intransitive-only verbs for both experimenter and child, whichever the child was to be primed with on that day. These verbs were not in the child’s verb set, nor were they used as primes by the experimenter in that child’s test trials. Twenty test trials then followed, with the experimenter continuing to use transitive-only or intransitive-only verbs, depending on prime condition. The experimenter produced only grammatical sentences. In contrast, half of the target verbs given to the children were alternating verbs (and would therefore be grammatical whether the child produced a transitive or an intransitive sentence) and half were transitive-only or intransitive-only, whichever was the opposite of the prime condition. For these trials, if the child produced a sentence using the same construction as that with which they had been primed, an overgeneralisation error would result.
In order to motivate the children to produce the sentences, a bingo game was used (as in Rowland, Chang, Ambridge, Pine, & Lieven, Reference Rowland, Chang, Ambridge, Pine and Lieven2012). Each time Experimenter 1 or the child produced a sentence, Experimenter 2 (who could not see the computer screen) looked for a matching bingo card. In fact, Experimenter 2 had all of the bingo cards and whether or not the card was given to Experimenter 1 / the child was predetermined: the games were fixed so that the child always won both games on the first day, lost the first game on the second day (in order to maintain tension), and then won the final game. This manipulation required an extra trial for Experimenter 1 only, on Day 2, always with the (alternating) verb float. Each bingo game lasted for ten trials, in order to keep the child’s attention and motivation.
4.1.4. Statistical analysis
Children’s responses were coded for sentence type: transitive (active), intransitive, passive (full or truncated), periphrastic causative, other use of the verb, excluded (target verb not included / no response). As we are investigating overgeneralisations, the errors of interest were intransitive uses of transitive-only verbs and transitive uses of intransitive-only verbs. Sentences were included in the analysis only if the child used the target verb in his/her response, with error rate calculated as a proportion of errors from the total number of responses that included the target verb. Replacement of NPs with pronouns or generic terms was allowed (e.g., the dad hit the ball for Homer hit the ball; it fell for the cup fell), as were changes in tense/aspect (e.g., Homer hit / hits / was hitting the ball), morphological overgeneralisations (e.g., The ball hitted), and additional modifying phrases (e.g., He kicked the ball in the goal).
The binary dependent variable for this experiment was the child’s response: overgeneralisation error (1) or other use of the target verb (0), with all responses in which the child did not use the target verb excluded from the analysis. As the dependent variable was binary, results were analysed using the glmer function of the lme4 package (version 1.1-23; Bates et al., Reference Bates, Maechler, Bolker and Walker2015), with family=binomial. Predictor variables were the same as in Experiment 1.
As with Experiment 1, all data and code are available at the following publicly accessible repository: <https://osf.io/xw934/>.
4.2. results
The mean number of sentences of each type produced by each child is shown in Figure 8, out of a possible maximum of 10. As can be seen in Figure 8, children produced a large proportion of sentences containing errors, in both directions. The fact that intransitivisation and transitivisation errors were observed at similar rates is encouraging in suggesting that the former are genuine intransitivisation errors, and do not merely reflect the child repeating the clue words and adding a determiner (e.g., Experimenter 2: “ball, hit”, Child: “The ball hit”). Such a strategy would not yield transitivisation errors (e.g., Experimenter 2: “wait, boy”, Child: “Lisa waited the boy”), yet such errors occurred at a similar rate to intransitivisation errors. Production priming therefore seems to be a powerful tool for eliciting overgeneralisation errors.

Fig. 8 Response types for all verbs, split by type. Total number of trials per condition is 640 (64 children × 10 responses), although totals do not reach this maximum due to the exclusion of trials where the child did not produce the target verb
However, given that the rate of these errors recorded in natural speech is so low, perhaps our implementation of the methodology has produced an unrealistically high number of errors. High error rates are, nevertheless, in line with findings from previous studies in which priming has been used when eliciting forms prone to overgeneralisation errors, showing just how sensitive young children can be to priming effects. For example, Ramscar and Dye (Reference Ramscar and Dye2011) showed that children could be primed to produce similarly high rates of errors (65%) with compound noun plurals (e.g., *red mice eater, cf. red mouse eater) simply by being asked to produce the irregular plural noun (e.g., mice) beforehand. Ramscar and Dye note that no such errors have been recorded in children’s spontaneous speech data. Similarly, Ramscar and Yarlett (Reference Ramscar and Yarlett2007) showed that, when asked to produce singular-then-plural noun forms in pairs (e.g., mouse–mice, foot–feet, tooth–teeth), children produced overgeneralization errors (e.g., *mouses, *foots, *tooths) at more than double the rate of correct (irregular) plurals (e.g., mice, feet, teeth). The question of ‘unrealistically high’ error rates is one to which we return in the Discussion (4.3). For now, the important point is simply that, as will be shown below, the rate of errors in the current study varied considerably between verbs, and we are therefore still able to test our hypotheses using these data (albeit bearing in mind this potential caveat).
Figures 9–10 plot the rate of transitivisation errors (for intransitive-only verbs) and intransitivisation errors (for transitive-only verbs). All predictors appear to pattern in the expected direction: for semantics, transitivisation errors increase, and intransitivisation errors decrease, as verbs increase on the semantic event-merge measure. This is expected, since a high score on the event-merge measure is associated with transitive, rather than intransitive semantics (e.g., The girl killed the fly, for which, prototypically, the killing and being-killed merge into a single event in time and space). For preemption and entrenchment, rates of transitivisation errors (e.g., *The man laughed the girl) are lowest when the relevant verb is of high frequency in periphrastic (preemption) and other (entrenchment) constructions relative to the transitive construction, as indicated by negative values. Rates of intransitivisation errors (e.g., *The city destroyed) are lowest when the relevant verb is of high frequency in passive (preemption) and other (entrenchment) constructions relative to the intransitive construction, again as indicated by negative values.

Fig. 9 Relationship between verb semantics/preemption/entrenchment and transitivisation errors for intransitive-only verbs.

Fig. 10 Relationship between verb semantics/preemption/entrenchment and intransitivisation errors for transitive-only verbs.
However, with a single exception, these apparent effects were not confirmed by the statistical models (see Appendix B). For the single-predictor models, all with maximal random effects structure (except that correlations were disallowed for the transitive-only verbs dataset), only preemption was significant, and only for transitivisation errors of intransitive-only verbs (e.g., *The man laughed the girl). Preemption was no longer significant in the multiple-predictor models, both with random intercepts only, though this is unsurprising given its collinearity with the entrenchment predictor.
4.3 discussion (Experiment 2: production)
The production priming method used here was successful in eliciting large numbers of overgeneralisation errors with both transitive-only and intransitive-only verbs. This allowed us to test the predictions of the entrenchment, semantics, and preemption hypotheses on production data, something which has not been possible with spontaneous production data given the sparsity of these errors in naturalistic speech. However, the artificially high error rate has potentially caused a number of unintended consequences.
Firstly, the pattern of findings observed for production, with only preemption a significant predictor of error rates, and only for the production of transitive sentences, contrasts with the pattern observed for judgments where all three predictors were significant (at least in single-predictor models). One possibility is that there is no relationship between production and comprehension of overgeneralisation errors; that the factors important in the production of these errors are not related to the factors involved in their comprehension. However, this seems a radical and highly unlikely conclusion to draw. More likely, then, is the possibility that the method itself has elicited a pattern of responses that obscures the effects of entrenchment, semantics, and preemption in children’s avoidance of overgeneralisation errors in production. For example, the binary outcome measure used in the production task (1 = overgeneralisation error, 0 = other use of the verb) is a far less sensitive measure than the continuous measure used in the judgment task (5-point scale). Increasing the number of participants in the production study, then, might have allowed the effects of the semantic and statistical measures to have been better observed.
Another tentative possibility is that we observed only effects of preemption because this is in fact the most prevalent mechanism for avoiding the production of overgeneralisation errors in children of this age. Given the complexity of linguistic systems, there is no reason to expect that the three mechanisms under investigation should develop in parallel. Indeed, in a paired-associate-learning task, Ramscar, Hendrix, Love, and Baayen (Reference Ramscar, Hendrix, Love and Baayen2013) showed that while simple co-occurrence effects were visible at ages 20–30, effects of background rates and blocking, which map on to entrenchment and preemption, respectively, were found only after the age of 30 years (see also Ramscar & Dye, Reference Ramscar and Dye2011, and Ramscar, Dye, & McCauley, Reference Ramscar, Dye and McCauley2013).
In terms of semantics, a potential problem with the production study is that discrete verb classes were used to divide the verbs into several sets, with predetermined transitive-only or intransitive-only verbs in each; transitive-only verbs were primed with only intransitive-only verbs and vice versa. This was done in order to provide the optimum conditions in which to prime error production. However, it also means that we are unable to combine rates of production of each sentence type for verbs of each type (since the transitive-only and intransitive-only verbs were not tested in the same conditions). If verb semantics are used to distinguish between verbs which can and cannot be used in each construction, then our method has, in fact, prevented the semantics mechanism from being demonstrated in production. It seems quite likely that verb semantics could, in principle, explain the different behaviour of different verbs in production if the study were set up so that we could directly compare verbs of different types (i.e., without removing some of the most salient semantic distinctions between the verbs in advance).
A final, more general problem that affects the present study is the large variation in error rates between children, as illustrated in Figures 11–12 (see Appendix C of the supplementary materials), which show the mean rates of transitivisation and intransitivisation errors for each child. Particularly problematic is the fact that 23 and 20 children displayed 100% rates of transitivisation and intransitivisation error, respectively, with 5 and 6 children, respectively, producing no such errors. This suggests that the main determinant of whether or not an overgeneralisation error occurs on a given trial is the identity of the child completing that trial, rather than the identity of the verb, which of course makes any underlying effect of preemption, entrenchment, or semantics difficult to observe. As can clearly be seen from Figures 13–14 (Appendix C), which show the mean rates of transitivisation and intransitivisation errors for each verb, the high degree of by-participant variance makes the estimate of the error rate for each verb unreliable: for most verbs, the Bayesian Highest Density Interval (similar to a confidence interval) spans around 0.5 points on the 0–1 scale (for example, if a verb has a mean error rate of 0.5, the Bayesian Highest Density Interval ranges from around 0.25 to around 0.75). Consequently, the Bayesian Highest Density Intervals for the verbs with the highest and lowest error rates overlap, obscuring any underlying effect of preemption, entrenchment, or semantics.
Before moving on to the General Discussion, we return to the question of whether the rates of overgeneralisation error observed in the present study were ‘unrealistically high’ (100% for many children). It is true that such errors are all but absent in naturalistic corpora, and – in the naturalistic context – have been recorded only in dedicated diary studies (e.g., Lord, 1979; Bowerman, Reference Bowerman1981). Nevertheless, since the alternatives to these types of overgeneralisation errors – passives and periphrastic causatives – are also vanishingly rare in children’s spontaneous speech, it is difficult to know what error rate the handful of documented errors reflect. Yet even if children do produce errors at considerably higher rates in production priming studies than in other contexts, this does not necessarily mean that the rate is ‘unrealistically high’; it depends exactly what we are trying to tap into. If the goal of a particular study is to estimate the rate at which children spontaneously produce such errors in naturalistic speech, then the present methods may well yield estimates that are unrealistically high. But if the goal of a particular study is to gauge the state of the child’s developing language system, high error rates observed as a function of priming may well constitute a true reflection. Under a discriminative learning perspective (e.g., Ramscar & Yarlett, Reference Ramscar and Yarlett2007; Ramscar & Dye, Reference Ramscar and Dye2011; Ramscar, Dye, & McCauley, Reference Ramscar, Dye and McCauley2013; Ramscar, Dye, & Klein, Reference Ramscar, Dye and Klein2013; Ramscar, Hendrix, Loce, & Baayern, Reference Ramscar, Hendrix, Love and Baayen2013), the language system of young children is unstable because learning is far from asymptotic and hence fast. It is therefore highly susceptible to the influence of priming effects. Indeed, the prediction of larger priming effects for younger children is one that is shared by all error-based-learning accounts, and supported by other work with children and adults (e.g., Rowland et al., Reference Rowland, Chang, Ambridge, Pine and Lieven2012).
5. General discussion
In this study we adopted a multi-method approach to the question of how children avoid argument structure overgeneralisation errors. In Experiment 1, we investigated how statistical and semantic constraints influence the way in which children (aged 5–6 and 9–10 years) and adults judge the grammatical acceptability of 120 verbs in transitive and intransitive sentences. In Experiment 2, we successfully used a priming methodology to elicit overgeneralisation errors from five- to six-year-old children, to investigate whether the same constraints appear to be operational in production. For judgments, a clear picture emerged: for all ages, for judgments of transitives, intransitives, and difference scores, the data show effects of semantics and statistics: preemption and/or entrenchment (we cannot say which, due to collinearity), mirroring the pattern observed in a recent meta-analysis of similar studies of other constructions. For production, the picture was less clear, with an effect of preemption observed for transitivisation errors with intransitive-only verbs (e.g., *The man laughed the girl), but no other effects, across both these errors and intransitivisation errors with transitive-only verbs (e.g., *The city destroyed). We concluded that, although it is possible to elicit overgeneralisation errors from five- to six-year-old children, the most likely explanation is that effects of verb semantics and preemption/entrenchment are real, but obscured by particular features of our production task; in particular, (a) the binary nature of the dependent measure, (b) the splitting of verbs into two semantic types, and (c) a high degree of variability between children (particularly the fact that many displayed a 100% error rate). We hope that future production studies will refine this method and overcome at least some of these shortcomings.
In the meantime, if we proceed on the basis of the present judgment findings, and the meta-analytic synthesis to which they contribute, the current best evidence suggests that effects of preemption, entrenchment, and semantics are real, and furthermore, observed across most of the major argument structure constructions for which children make errors, at least in English. This raises the question of what kind of account can explain all three effects.
When considering this question in the light of the previous version of the judgment-data meta-analytic synthesis, Ambridge et al. (Reference Ambridge, Barak, Wonnacott, Bannard and Sala2018) discussed three accounts. First, Ambridge and colleagues’ FIT account (Ambridge & Lieven, Reference Ambridge and Lieven2011; Ambridge, Pine & Rowland, Reference Ambridge, Pine and Rowland2012) posits that verb-in-construction frequency (explaining both entrenchment and preemption effects) combines with construction relevance and the semantic ‘fit’ of the verb in the construction when selecting the best construction to convey the message. Constructions that are heard more frequently with a verb and have a better fit with that verb will be activated more strongly and will therefore be more likely to be chosen. Ambridge and Blything’s (Reference Ambridge and Blything2016) connectionist instantiation of the FIT account was able to simulate both the overall overgeneralisation-then-retreat pattern, and the by-verb pattern of participants’ judgments, observed for the DO-dative construction (e.g., Bart gave Lisa a present).
Second, Goldberg’s (Reference Goldberg2019) CENSE-ME account is similar in many ways to the FIT account, but places more emphasis on competition between constructions (preemption, rather than entrenchment) and on error-driven learning. This account has also been instantiated as a computational model, which uses a Bayesian clustering algorithm to group together verbs with similar semantic and distributional properties (Barak, Goldberg, & Stevenson, Reference Barak, Goldberg and Stevenson2016). Importantly, this model outperformed Ambridge and Blything’s (Reference Ambridge and Blything2016) model of the dative, in that it could explain not only the pattern of judgments observed for the DO-dative, but also the PO-dative (e.g., Bart gave a present to Lisa) and difference scores.
Third, Ambridge et al. (Reference Ambridge, Barak, Wonnacott, Bannard and Sala2018) concluded that the most promising approach lies with a third type of account: discriminative learning (e.g., Ramscar, Dye, & McCauley, Reference Ramscar, Dye and McCauley2013), which has its origins in the animal learning literature (e.g., Rescorla & Wagner, Reference Rescorla and Wagner1972). The advantages of this approach are that (1) it yields effects of preemption, entrenchment, and semantics from a single learning mechanism, (2) it is straightforwardly formalised using a simple learning algorithm, and (3) it already enjoys support in many domains of language acquisition, including word learning (e.g., Ramscar, Dye, & Klein, Reference Ramscar, Dye and Klein2013) and morphosyntax (e.g., Arnon & Ramscar, Reference Arnon and Ramscar2012). Ambridge et al. (Reference Ambridge, Barak, Wonnacott, Bannard and Sala2018, p. 51), summarise the general approach as follows:
The key feature of discriminative-learning models is that learning is a process by which prediction error is used to discriminate uninformative versus informative cues. Thus, such models weight cue strength from both cue-outcome pairings that are observed, and cue-outcome pairings that are predicted, but not observed. For example, suppose that a rat learns to associate a tone (cue) with a shock (outcome), and so freezes in anticipation of a shock whenever the tone is heard. In an otherwise-identical setup with additional tones that are not followed by a shock, learning is attenuated. Indeed, the likelihood of the rat freezing in response to the tone decreases in proportion to the background rate of tones that are not followed by a shock (Rescorla, Reference Rescorla1968).
In principle, then, this account could be applied to the domain of (in)transitivisation errors as follows. The learning situation can be formalised such that children learn the predictive value of real-world semantic cues for particular linguistic outcomes (e.g., CAUSE + BREAK ➔ “X broke Y”; CAUSE + ROLL ➔ “X rolled Y”). Having learned this relationship, children produce errors such as *The man laughed the girl, because the cue of CAUSE is highly predictive of the transitive “X VERBed Y” structure. Errors cease as children learn a more fine-grained discrimination: in fact, it is the combination of the semantic cues CAUSE and, crucially, SINGLE-EVENT (as per the present event-merge measure) that is most predictive of the transitive “X VERBed Y” structure (e.g., CAUSE + BREAK + SINGLE-EVENT ➔ “X broke Y”; CAUSE + ROLL + SINGLE-EVENT ➔ “X rolled Y”). The rival combination of semantic cues CAUSE + SEPARATE-EVENTS is instead highly predictive of the periphrastic causative construction with make (e.g., CAUSE + BREAK + SEPARATE-EVENTS ➔ “X made Y break”; CAUSE + ROLL + SEPARATE-EVENTS ➔ “X made Y roll”). As children learn this discrimination, they will learn to say not *The man laughed the girl, but The man made the girl laugh (i.e., CAUSE + LAUGH + SEPARATE-EVENTS ➔ “X made Y laugh”).
This formalisation corresponds closely to the notion of preemption, but it does so in a way that yields effects of verb semantics and entrenchment for free. Any verb whose semantics are such that it is more likely to appear in a SEPARATE-EVENTS than SINGLE-EVENT scenario (e.g., dance, sing, run) will automatically be generalised into the periphrastic causative, rather than the transitive, as the fine-grained discrimination set out above is learned. Entrenchment effects arise as a function of the fact that (for example) LAUGH + CAUSE events will often occur in the absence of the transitive X VERBed Y construction (e.g., if the speaker chooses simply to say The girl laughed), since learning takes place whenever a predicted outcome (LAUGH + CAUSE ➔ “X VERBed Y”) fails to occur. On this view, entrenchment effects are observed because the overall frequency of laugh in non-causative utterances (e.g., The girl laughed) is a proxy for the frequency of laughing events; in principle, it is the latter that is relevant.
In conclusion, whether or not any of three types of account that we have set out here is along the right lines, the present study has contributed to a growing body of evidence which suggests that any successful account of the retreat from argument structure overgeneralisation errors will need to explain effects of preemption, entrenchment, and verb semantics that are now well established, at least in judgment studies. Future studies should aim to refine the production priming method that we have used here, in order to better investigate the relationship between judgments and production with regard to this debate, which lies at the very heart of theorising about child language acquisition.
Supplementary Materials
To view supplementary material for this article, please visit http://dx.doi.org/10.1017/langcog.2021.8.