Introduction
There have been remarkable developments in fluid and neuroimaging biomarkers that track the progression of Alzheimer’s disease (AD). AD biomarkers can identify pathological changes in amyloid and tau that occur well before symptom onset (Barthélemy et al., Reference Barthélemy, Li, Joseph-Mathurin, Gordon, Hassenstab, Benzinger, Buckles, Fagan, Perrin, Goate, Morris, Karch, Xiong, Allegri, Chrem Mendez, Berman, Ikeuchi, Mori, Shimada and McDade2020; Bateman et al., Reference Bateman, Benzinger, Berry, Clifford, Duggan, Fagan, Fanning, Farlow, Hassenstab, McDade, Mills, Paumier, Quintana, Salloway, Santacruz, Schneider, Wang and Xiong2017; Price et al., Reference Price, McKeel, Buckles, Roe, Xiong, Grundman, Hansen, Petersen, Parisi, Dickson, Smith, Davis, Schmitt, Markesbery, Kaye, Kurlan, Hulette, Kurland and Morris2009; Sperling et al., Reference Sperling, Aisen, Beckett, Bennett, Craft, Fagan, Iwatsubo, Jack, Kaye, Montine, Park, Reiman, Rowe, Siemers, Stern, Yaffe, Carrillo, Thies, Morrison-Bogorad and Phelps2011). Despite these developments, advances in the measurement of cognitive decline – the essence of the disease phenotype – have lagged behind. Secondary prevention trials targeting abnormal biomarker levels in preclinical (presymptomatic) AD are determined to be successful if they stop or slow cognitive decline (Edgar et al., Reference Edgar, Vradenburg and Hassenstab2019; Food and Drug Administration, 2018). Because the declines in cognition that occur in preclinical AD are subtle, capturing declines, slowing of declines, or improvements require reliable cognitive tests that are sensitive to AD pathological processes. However, standard cognitive assessment tools used in AD studies include classic neuropsychological tests that were originally designed to detect overt cognitive impairments or measure facets of intelligence (Sheehan, Reference Sheehan2012; Weintraub et al., Reference Weintraub, Salmon, Mercaldo, Ferris, Graff-Radford, Chui, Cummings, DeCarli, Foster, Galasko, Peskind, Dietrich, Beekly, Kukull and Morris2009; Woodford & George, Reference Woodford and George2007) and often place heavy burden on participants. This poses a critical hurdle for randomized controlled trials (RCTs) examining therapeutics in preclinical and early-stage symptomatic AD populations. Measures with sub-optimal reliability require larger sample sizes to detect cognitive benefits, particularly when the expected effects are subtle (Dodge et al., Reference Dodge, Zhu, Mattek, Austin, Kornfeld and Kaye2015).
Advances in smartphone technology have allowed researchers to embed brief cognitive measures into ecological momentary assessments (EMA). EMA methods investigate psychological states and behaviors as they occur in natural environments (Shiffman et al., Reference Shiffman, Stone and Hufford2008; Sliwinski et al., Reference Sliwinski, Mogle, Hyun, Munoz, Smyth and Lipton2018; Smyth & Stone, Reference Smyth and Stone2003). EMA is defined by several features: (1) data are collected as participants go about their daily lives; (2) assessments are randomly sampled across various occasions to characterize an individual’s average performance on a given variable of interest; and (3) participants perform multiple short assessments to capture behavioral changes over time and across different situations (Sliwinski et al., Reference Sliwinski, Mogle, Hyun, Munoz, Smyth and Lipton2018).
Although traditional laboratory/clinical settings afford precise control over the testing environment, this is not representative of everyday cognitive functioning (Sliwinski et al., Reference Sliwinski, Mogle, Hyun, Munoz, Smyth and Lipton2018). The use of smartphone EMAs in cognitive research can assuage ecological validity concerns as participants perform assessments as they go about their daily lives. Additionally, repeated assessments can improve upon the reliability of conventional measures because they are not collected in just one testing session that may be influenced by variability in participants’ day-to-day stress and mood, amongst other factors (Sliwinski et al., Reference Sliwinski, Mogle, Hyun, Munoz, Smyth and Lipton2018). In individuals with neurodegenerative disorders, cognitive performance can vary with time of day (Wilks et al., Reference Wilks, Aschenbrenner, Gordon, Balota, Fagan, Musiek, Balls-Berry, Benzinger, Cruchaga, Morris and Hassenstab2021), and day-to-day variability can be exaggerated (Matar et al., Reference Matar, Shine, Halliday and Lewis2020), further exacerbating the impact of conventional measures’ low reliability. With EMA, aggregation across repeated measurements ameliorates effects of within-person variability and improves reliability by estimating average functioning (Shiffman et al., Reference Shiffman, Stone and Hufford2008; Sliwinski, Reference Sliwinski2008; Sliwinski et al., Reference Sliwinski, Mogle, Hyun, Munoz, Smyth and Lipton2018). Although ambulatory cognitive testing is not necessarily a replacement of gold standard in-person cognitive testing, smartphone EMAs provide snapshots of cognition that may reveal unique patterns that cannot be captured with conventional testing.
Smartphone-based assessments may offer a more practical and logistically plausible solution for large-scale studies and clinical trials of AD. Allowing individuals to participate in research studies unsupervised, in familiar environments, and using their own devices can increase engagement, reduce experimenter effects (e.g. demand characteristics, “white coat” testing effects), bolster sample size and diversity, and make participation more accessible and inclusive for individuals who may otherwise be unable to come into the laboratory or clinic. Indeed, interest in smartphone studies is growing, and several studies have demonstrated the feasibility and validity of smartphone-based assessments for use in older adults and individuals with preclinical AD (Güsten et al., Reference Güsten, Ziegler, Düzel and Berron2021; Hassenstab et al., Reference Hassenstab, Aschenbrenner, Balota, McDade, Lim, Fagan, Benzinger, Cruchaga, Goate, Morris and Bateman2020; Lancaster et al., Reference Lancaster, Koychev, Blane, Chinner, Chatham, Taylor and Hinds2020; Mackin et al., Reference Mackin, Insel, Truran, Finley, Flenniken, Nosheny, Comacho, Harel, Maruff and Weiner2018; Nicosia et al., Reference Nicosia, Aschenbrenner, Adams, Tahan, Stout, Wilks, Balls-Berry, Morris and Hassenstab2021; Öhman et al., Reference Öhman, Hassenstab, Berron, Schöll and Papp2021; Papp et al., Reference Papp, Samaroo, Chou, Buckley, Schneider, Hsieh, Soberanes, Quiroz, Properzi, Schultz, García-Magariño, Marshall, Burke, Kumar, Snyder, Johnson, Rentz, Sperling and Amariglio2021; Wilks et al., Reference Wilks, Aschenbrenner, Gordon, Balota, Fagan, Musiek, Balls-Berry, Benzinger, Cruchaga, Morris and Hassenstab2021), as well as the potential for high-frequency in-home monitoring to substantially increase the statistical power of therapeutic trials (Dodge et al., Reference Dodge, Zhu, Mattek, Austin, Kornfeld and Kaye2015).
The purpose of the present study was to evaluate the reliability, validity, and feasibility of unsupervised, high-frequency cognitive testing using participants’ personal smartphones. Tasks assessed associate memory, processing speed, and working memory in older adults and individuals with preclinical and early symptomatic AD. If the Ambulatory Research in Cognition smartphone application (ARC) is a reliable, valid, and feasible measure, ARC should: (1) demonstrate high between-subjects and retest reliability; (2) have construct validity (indexed by correlations with correlations with conventional cognitive measures); (3) demonstrate sensitivity to age and AD-related biomarkers; and (4) be well tolerated by older adults regardless of technology familiarity.
Methods
Participants
We recruited participants enrolled in ongoing studies of aging and dementia at the Charles F. and Joanne Knight Alzheimer Disease Research Center (Knight ADRC) at Washington University School of Medicine in St. Louis. ARC was designed to be sensitive to subtle changes in cognition in participants at risk for, or in the earliest stages, of AD, thus enrollment in the ARC study was limited to those with a Clinical Dementia Rating® (CDR®; Morris, Reference Morris1993) of 0 (cognitively normal) or 0.5 (very mild dementia). In-person enrollment began in February of 2020 and was halted in March 2020 due to the SARS-CoV-2 (COVID-19) pandemic. Therefore, beginning April 2020, the majority of participants were enrolled remotely. All participants provided informed consent, and all procedures were approved by the Human Research Protections Office at Washington University in St. Louis and the research was conducted in accordance with the Helsinki Declaration.
Clinical assessment
Clinical status was determined with the CDR which uses a 5-point scale to characterize six domains of cognitive and functional performance (memory, orientation, judgment and problem solving, community affairs, home and hobbies, and personal care) that are applicable to AD and other dementias (Morris, Reference Morris1993). CDR scores are determined through semi-structured interviews with the participant and an informant (i.e., family member or friend). A CDR score of 0 indicates cognitive normality, 0.5 = very mild dementia, 1 = mild dementia, 2 = moderate dementia, and 3 = severe dementia.
Conventional cognitive assessments
Conventional cognitive measures included measures of verbal fluency (Animals, Vegetables, and Verbal Fluency), episodic memory (Wechsler Memory Scale Paired Associates Recall, Free and Cued Selective Reminding Test (FCSRT) Free Recall, Craft Story 21 immediate and delayed recall), language (the Multilingual Naming Test; MINT), processing speed (Number Span Forward, Number Symbol TestFootnote 1 ), and working memory (Number Span Backwards; see Hassenstab et al., Reference Hassenstab, Chasse, Grabow, Benzinger, Fagan, Xiong, Jasielec, Grant and Morris2016 and Weintraub et al., Reference Weintraub, Besser, Dodge, Teylan, Ferris, Goldstein, Kramer, Loewenstein, Marson, Mungas, Salmon, Welsh-Bohmer, Zhou, Shirk, Atri, Kukull, Phelps and Morris2018 for additional information). A global composite similar to the Preclinincal Alzheimer’s Cognitive Composite (PACC; Donohue et al., Reference Donohue, Sperling, Salmon, Rentz, Raman, Thomas, Weiner and Aisen2014; Papp et al., Reference Papp, Rentz, Orlovsky, Sperling and Mormino2017) was created by averaging the standardized scores from FCSRT free recall, Animal naming total score, Craft Story 21 delayed recall, and the total correct score from the Number Symbol test such that higher scores indicated better performance (Weintraub et al., Reference Weintraub, Salmon, Mercaldo, Ferris, Graff-Radford, Chui, Cummings, DeCarli, Foster, Galasko, Peskind, Dietrich, Beekly, Kukull and Morris2009).
Ambulatory research in cognition (ARC) application
The ARC smartphone application is based on principles from EMA and administers brief tests of associative memory, processing speed, and working memory up to 4 times per day over 7 consecutive days. Sampling frequency and duration were chosen based on reliability, validity, and effect size estimates reported in Sliwinski et al. (Reference Sliwinski, Mogle, Hyun, Munoz, Smyth and Lipton2018). ARC is programmed to run on major operating system (OS) versions (currently iOS 12.0+ and Android OS 8.0+) on iOS and Android devices. Participants were encouraged to use their personal smartphones as long as minimum technical requirements were met. Individuals interested in participating who did not own a smartphone or whose smartphone did not meet our criteria were supplied a device (either iOS or Android) for the duration of the study. Device exclusion criteria included software issues, limited phone storage, physical damage, battery problems, or poor responsivity. A trained study coordinator (M.T.) provided participants with detailed instructions regarding the ARC application, and additional guidance on smartphone basics (including device setup and operation) was given to participants who were less familiar with smartphones. Throughout the study, the study coordinator provided extensive support for participants via phone, videoconferencing, email, and text messaging. Participants are reimbursed at a rate of $0.50 per completed assessment session. To incentivize participation consistency, participants receive bonus payments for completing all 4 sessions any given day ($1.00 per occurrence, max of $7.00), completing at least 2 assessments per day for 7 days ($6.00), and completing at least 21 assessments over 7 days ($5.00). The maximum compensation possible for one 7-day assessment visit was $32.00.
ARC assessment notifications were administered pseudorandomly throughout the participant’s self-reported awake hours, with at least 2 hr between each testing session. For example, if a participant reported waking up at 7 am and going to bed at 10 pm, they would receive four test session notifications between 7 am and 10 pm, separated by at least 2 hr (see Figure 1, top). The ARC cognitive tasks, Grids, Prices, and Symbols (see Figure 1, bottom), were administered in a random order during each session.
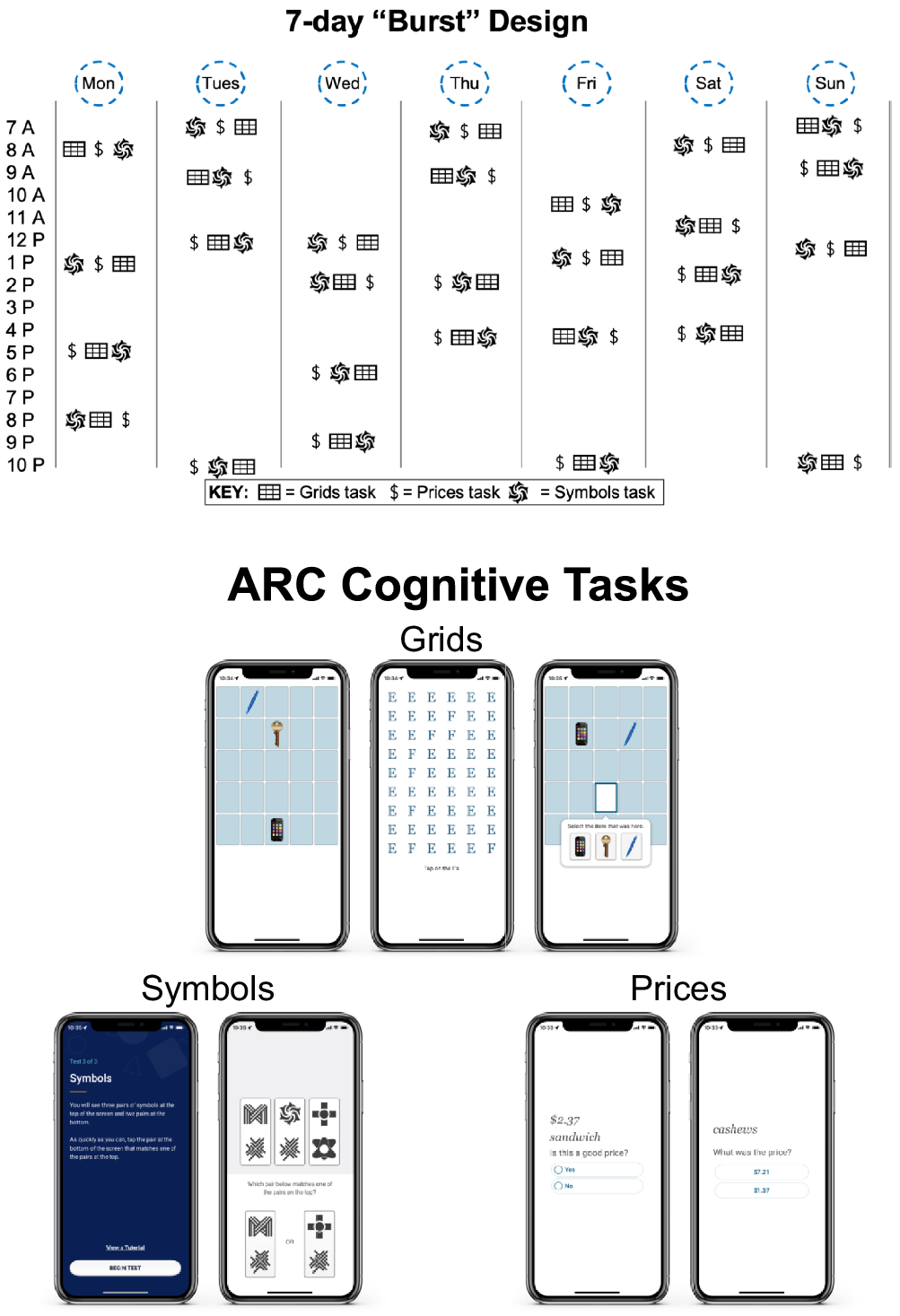
Figure 1. ARC design and cognitive tasks. Note. Top demonstrates if a participant reported waking up at 7 am and going to bed at 10 pm, they would receive four test session notifications between 7 am and 10 pm, separated by at least 2 hr. The ARC cognitive tasks, Grids, Prices, and Symbols are displayed on the bottom.
Grids is a spatial working memory task in which high resolution images of three common objects (key, smartphone, and pen) are displayed on a 5 x 5 grid, and participants are asked to remember the locations of the items. After encoding the locations of each item, participants perform a distractor task (identify Fs in grid of Es) before moving to the retrieval phase. At retrieval, participants are asked to tap the locations where the items were shownFootnote 2 . Participants perform two trials during each test session (lasting approximately 30–40 s) and, across sessions, stimuli are placed at random locations to protect against retest effects. Scores reflect a Euclidean distance estimate, agnostic to item, such that a higher score indicates retrieval placement farther away from the encoded locations (i.e., higher score indicates worse performance; Sliwinski et al., Reference Sliwinski, Mogle, Hyun, Munoz, Smyth and Lipton2018).
Prices is an associate memory task with a learning and recognition phase. In the learning phase, participants are shown 10 item–price pairs for 3 s per pair and asked to remember the items and their corresponding prices. Items were common shopping items (food and household supplies), and the prices were randomly assigned 3-digit prices containing no repeated digits and no more than two sequential digits. In the recognition phase, participants were presented with two prices and asked to choose which was shown with the item during the learning phase. The price choices were separated by at least $3.00 to avoid ceiling and floor effects (Hassenstab et al., Reference Hassenstab, Aschenbrenner, Balota, McDade, Lim, Fagan, Benzinger, Cruchaga, Goate, Morris and Bateman2020). To protect against retest and interference effects, 40 items, chosen without replacement, are never repeated within the same day, and item–price pairs are never re-presented over the 28 sessions. Trials last approximately 60 s and scores reflect the proportion of recognition trial errors such that higher scores indicate worse performance.
Symbols is a processing speed measure based on a task used by Sliwinski et al. (Reference Sliwinski, Mogle, Hyun, Munoz, Smyth and Lipton2018). Participants are shown three randomly assigned pairs of abstract shapes and asked to determine as quickly as possible which of two pairs match one of the three target pairs. To protect against retest effects, item pairs are randomly assigned for each session. Participants complete 12 trials during each session, lasting approximately 20–60 s (duration varied based on participants’ response times (RTs)). Scores reflect RTs on correct trials such that higher scores indicate worse performance. An “ARC composite score” was created in two steps. Z-scores for each task were calculated by subtracting raw scores from the cohort’s mean score and dividing by the cohort’s standard deviation. The Z-scores were then averaged together to form the ARC composite score. Similar to the individual measures, a higher ARC composite score indicated worse performance.
Feasibility and tolerability measures
Technology familiarity was assessed with a novel measure described in Nicosia et al. (Reference Nicosia, Aschenbrenner, Adams, Tahan, Stout, Wilks, Balls-Berry, Morris and Hassenstab2021). Briefly, the assessment combined objective measurements of technology knowledge (technology-related icon recognition) and self-reported ratings of (1) the frequency with which they perform certain smartphone tasks and (2) how difficult it would be for them to perform various technology-related tasks. For the purposes of this study, we report participants’ technology icon recognition, average frequency of smartphone task performance, and average difficulty performing technology-related tasks (for more details see Nicosia et al., Reference Nicosia, Aschenbrenner, Adams, Tahan, Stout, Wilks, Balls-Berry, Morris and Hassenstab2021).
ARC user experience was assessed with a 10-question survey using a 5-point Likert scale to rate aspects of user experience regarding installation, test instructions, frequency of testing, and overall tolerability. Objective measures of feasibility and tolerability included ARC adherence and drop-out rates. Adherence was defined as the number of completed test sessions divided by the total number of assessment sessions (i.e., a participant who completed 21 of 28 sessions would have a 75% adherence rate).
Cerebrospinal fluid collection and processing
Most participants underwent lumbar puncture (LP) to collect cerebrospinal fluid (CSF) following overnight fasting. Participants at the Knight ADRC undergo LP approximately every 3 years; however, CSF collection was postponed in March 2020 due to the pandemic, eliminating the possibility of acquiring more recent samples. Therefore, we limited the use of CSF data to those collected within 5 years of ARC testing (see Table 1; collected on average 2.64 +/- 1.11 years from the first ARC assessment). Twenty to thirty mL of CSF was collected in a 50 mL polypropylene tube via gravity drip using an atraumatic Sprotte 22-gauge spinal needle. CSF was kept on ice and centrifuged at low speed within 2 hr of collection. CSF was then transferred to another 50 mL tube. CSF was aliquoted at 500 µL into polypropylene tubes and stored at −80°C as previously described (Fagan et al., Reference Fagan, Mintun, Mach, Lee, Dence, Shah, LaRossa, Spinner, Klunk, Mathis, DeKosky, Morris and Holtzman2006). Prior to analysis, samples were brought to room temperature per manufacturer instructions. Samples were vortexed and transferred to polystyrene cuvettes for analysis. Concentrations of Aβ40, Aβ42, total tau (tTau), and tau phosphorylated at threonine 181 (pTau) were measured by chemiluminescent enzyme immunoassay using a fully automated platform (LUMIPULSE G1200, Fujirebio, Malvern, PA) according to manufacturer’s specifications. A single lot of reagents were used for all samples.
Table 1. Demographic data

a Mean (SD); n (%).
b Welch two sample t-test; Pearson’s Chi-squared test.
c Gender and race were self-reported.
Neuroimaging
Neuroimaging data were required to be collected within 5 years of ARC (see Table 1; Amyloid positron emission tomography (PET) mean 2.59 +/- 1.04 years, Tau PET mean 2.50 +/- 0.96, and magnetic resonance imaging (MRI) mean 2.55 +/- 1.05 years from the first ARC assessment). Briefly, MRI data were acquired on 3T Siemens scanners and processed using Freesurfer (Fischl et al., Reference Fischl, Van Der Kouwe, Destrieux, Halgren, Ségonne, Salat, Busa, Seidmann, Goldstein, Kennedy, Makris, Rosen and Dale2004) to derive regional volumes and thicknesses. Volumes were adjusted for total intracranial volume (ICV) (see Raz et al., Reference Raz, Lindenberger, Ghisletta, Rodrigue, Kennedy and Acker2008) and a summary thickness composite was calculated (Singh et al., Reference Singh, Chertkow, Lerch, Evans, Dorr and Kabani2006).
Amyloid PET imaging was performed with either florbetapir (18F-AV-45) or Pittsburgh Compound B (PiB). Data were processed with an in-house pipeline using regions of interest derived from FreeSurfer (ttps://github.com/ysu001/PUP; Su et al., Reference Su, D’Angelo, Vlassenko, Zhou, Snyder, Marcus, Blazey, Christensen, Vora, Morris, Mintun and Benzinger2013). A summary standardized uptake value ratios (SUVR) measure was converted to the Centiloid scale (Su et al., 2018, Reference Su, Flores, Wang, Hornbeck, Speidel, Joseph-Mathurin, Gordon, Koeppe, Klunk, Jack, Farlow, Salloway, Snider, Berman, Roberson, Brosch, Jimenez-Velazques, van Dyck and Benzinger2019) in order to combine PiB and florbetapir data. Tau PET imaging with flortaucipir (18F-AV-1451) was summarized using the average SUVRs of the bilateral entorhinal cortex, amygdala, inferior temporal lobe, and lateral occipital cortex (Mishra et al., Reference Mishra, Gordon, Su, Christensen, Friedrichsen, Jackson, Hornbeck, Balota, Morris, Ances and Benzinger2017). SUVRs used a cerebellar cortex reference and were partial volume corrected.
Statistical analyses
Statistical analyses were completed using R (v4.1.0). To characterize the reliability of ARC, descriptive statistics were examined for all ARC and conventional measures. Correlations were used to examine whether ARC captured age-related cognitive declines comparable to conventional cognitive measures. ARC test-retest reliability was assessed based on participants who completed follow-up testing ∼6 months (“visit 2”; on average 6.07 +/- 1.23 months between assessments) and ∼1 year later (“visit 3”; on average 11.84 +/- 0.84 months between assessments). Pearson correlation coefficients with an r of 0.80 to 0.90 were considered “good” reliability (Price et al., Reference Price, Jhangiani and Chiang2015). Intraclass correlations (ICCs), which show how strongly units within the same group resemble each other, were computed to examine test-retest reliability and between-person reliability such that ICCs between 0.75 and 0.90 indicate “good” reliability (Bruton et al., Reference Bruton, Conway and Holgate2000). ARC and conventional cognitive measure correlations were used to examine construct validity. Finally, feasibility and tolerability were assessed by examining: (1) adherence and drop-out rates; (2) correlations between technology familiarity measures and ARC performance; and (3) descriptive statistics from an ARC user experience survey.
Results
Participant characteristics
Of the 316 participants who completed at least one ARC session, 26 were removed due to either low-quality data or unacceptable rates of missing data (>75% missingness) resulting in a sample size of 290 participants (268 CDR 0 s and 22 CDR 0.5 s) ranging from 61 to 97 years of age. As shown in Table 1, all three ARC tasks showed good discrimination between CDR 0 and CDR 0.5 participantsFootnote 3 . Additionally, ARC performance, as indexed by the ARC composite score, did not differ as a function of gender, t(181.46) = 0.63, p = 0.53, or race, t(28.096) = 1.92, p = 0.06, and was modestly associated with education, r = −0.18, p = 0.01.
Descriptive statistics
Table 2 shows the descriptive statistics for the ARC and conventional cognitive measures as well as adherence and drop-out rates. The t-tests comparing ARC task performance of CDR 0 and 0.5 individuals (significant ts 2.12–3.52) were comparable to comparisons with conventional cognitive measures (significant ts 2.17–4.96). CDR 0 s and 0.5 s in this sample did not differ on Number Span Forward, Number Span Backward, or the MINT. Adherence and drop-out rates did not differ as a function of CDR status (ts < 0.38).
Table 2. Descriptive statistics at ARC baseline

Note.
* Indicates p-value < 0.05.
** Indicates p-value < 0.01.
*** Indicates p-value < 0.001.
Between-person reliability
As mentioned above, aggregation of EMA scores across sessions boosts reliability compared to conventional “one-shot” approaches (Shiffman et al., Reference Shiffman, Stone and Hufford2008). Unconditional multilevel mixed models using restricted maximum likelihood were employed for each ARC task to compute between-person reliability scores (Raykov & Marcoulides, Reference Raykov and Marcoulides2006; Sliwinski et al., Reference Sliwinski, Mogle, Hyun, Munoz, Smyth and Lipton2018). The reliabilities of scores aggregated across ARC sessions were quite high: 0.81 for Prices, 0.90 for Grids, and 0.98 for Symbols (see Table 3). These reliabilities are based on 21 (75%) sessions of ARC assessments, which reflects the average number of sessions participants completed.
Table 3. ARC reliabilities for individual tasks

Note. ARC participants received 4 sessions/day for 7 day.
Next, we conducted follow-up analyses to determine how many sessions would be required to obtain reliabilities of aggregated scores that ranged from 0.80 to 0.90. Following Sliwinski et al. (Reference Sliwinski, Mogle, Hyun, Munoz, Smyth and Lipton2018), we fit a series of unconditional multilevel mixed models and calculated reliabilities. These results indicated that 19 sessions (or ∼ 5 days) of Prices, 9 sessions (or ∼ 2 days) of Grids, and 2 sessions (or ∼1 day) of Symbols are required to attain reliabilities greater than 0.80 (see Table 3 and Figure 2).

Figure 2. Between-person reliabilities for ARC tasks. Note. Between-person reliabilities for each ARC cognitive task. Following Sliwinski et al. (Reference Sliwinski, Mogle, Hyun, Munoz, Smyth and Lipton2018), a series of unconditional multilevel mixed models were fit to determine how many sessions would be required to obtain good reliability. Blue line indicates 0.85 reliability threshold.
Test-retest reliability
As of manuscript preparation, a subset of participants also completed testing ∼6 months (N = 185) and ∼1 year (N = 83) after their initial visit. Figure 3 displays test-retest reliability for the 6-month and 1-year follow-ups for the individual tasks and ARC composite score. ARC demonstrated high test-retest reliability for individual ARC tasks as well as the ARC composite score at both follow-ups (all ICCs > 0.85). Considering retest effects (Table 4), there were small but significant improvements from visit 1 to visit 2 on Prices, Symbols, and the ARC Composite, but not on Grids. There were no practice effects evident between visits 2 and 3, suggesting that practice effects diminish after completion of the first testing cycle. A detailed analysis of practice effects will be considered in future studies.

Figure 3. ARC Test-retest reliabilities at 6 month (top) and 1 year (bottom) follow-up.
Table 4. ARC test-retest

a Mean (SD).
b Welch two sample t-test.
Note. Values represent participants mean score for that visit, values in parentheses represent standard deviations. Significant p-values indicate the presence of a practice effect.
Construct validity
As shown in Figure 4 (right), the ARC composite score was correlated with the global composite score created from the conventional measures (r = −0.53; this was also the case in the CDR 0 sample, r = −0.47), indicating good construct validity. Additionally, Figure 4 (left) displays correlations between ARC and conventional cognitive measures (raw scores), and the top row shows the correlations with age. ARC tasks showed similar correlations with age as the conventional cognitive measures and exhibited convergent validity such that measures were correlated within the same domains. Note that correlations between the conventional and ARC measures are negative because higher scores on the ARC tasks indicate worse performance, whereas higher scores on the conventional cognitive measures indicate better performance (except for the Trailmaking Test Parts A & B), thus the negative correlations displayed in Figure 4 (left) are in the hypothesized direction. Specifically, the Prices task was correlated with conventional memory measures (WMS Associates Recall: r = −0.24, FCSRT free recall: r = −0.32, Craft Story immediate recall: r = −0.22, Craft Story delayed recall: r = −0.27), the Grids task was correlated with all of the conventional cognitive measures (r’s = −0.15 to −0.36), and the Symbols task was correlated with all the conventional cognitive measures but particularly the fluency tasks and the Number Symbol test (Category Fluency Animals: −0.36, Category Fluency Vegetables: −0.40, Verbal Fluency: −0.36, Number Symbol test: −0.57).

Figure 4. ARC, conventional, and AD biomarker correlations. Note. Correlations amongst ARC and conventional measures (raw scores) shown on the left (N = 282). Correlations of the ARC composite score (higher = worse) and global composite score (higher = better), and AD-related biomarkers are shown on the right (Ns = 146 for CSF measures, 212 for amyloid PET, 173 for tau PET, 175 for AD ROI cortical thickness, and 290 for hippocampal volume). Significant correlations (p < 0.05) are displayed with colored circles, non-significant correlations are blank. Because in-clinic and ARC measures have opposing directionality, the negative correlations amongst the conventional and ARC measures are in the hypothesized direction.
Criterion validity
Criterion validity of ARC was examined by comparing ARC and global composite score correlations with AD biomarkers. As shown in Figure 4 (right), the ARC composite score was correlated in the predicted directions with all AD biomarkers. All correlations remained significant after controlling for age, rs > 0.20, ps < 0.02, except for the relationships with the neurodegeneration and tauopathy measures, ps > 0.18. We also examined correlations between the ARC composite score and AD biomarkers with only CDR 0 participants. Correlations in the cognitively normal subsample (CDR 0 individuals) were weaker than in the full sample (see Supplemental Materials Figure 1), as expected, but were consistent with the magnitude of values seen in other studies which have explored such relationships (for example, see Papp et al., Reference Papp, Samaroo, Chou, Buckley, Schneider, Hsieh, Soberanes, Quiroz, Properzi, Schultz, García-Magariño, Marshall, Burke, Kumar, Snyder, Johnson, Rentz, Sperling and Amariglio2021 among others). Additionally, the correlations were comparable to, though slightly weaker than, correlations between the global composite score and AD biomarkers. Specifically, Fisher’s Z test indicated that, compared to the global composite score, all correlations with the ARC composite score were not significantly different except for the correlations with CSF pTau:Aβ42 (Z = −1.96, p = 0.049), Hippocampal Volume (Z = −1.99, p = 0.045), and PET Tau (Z = −2.20, p = 0.03), which were only marginally to slightly weaker. There were no significant differences in correlations between AD biomarkers and the two composite scores in the CDR 0 subsample.
Feasibility and tolerability
Of the 290 participants included in the present analyses, a subset (N = 220) completed the technology familiarity survey. Figure 5 displays the correlations among age, adherence, the technology familiarity measures, and ARC performance. Greater technology-related icon recognition was associated with better performance on Grids (r = −0.16) and Symbols (r = −0.14), but not on Prices (r = −0.02). Self-reported frequency performing smartphone tasks was unrelated to ARC performance, but perceived difficulty performing technology tasks was related to worse performance on all ARC measures (r’s 0.17–0.24). Adherence was correlated with performance on all three ARC measures (though only weakly for Prices) and the ARC composite score, such that participants who completed more sessions tended to perform better on ARC.

Figure 5. Age, technology familiarity, and ARC performance correlations. Note. Of the 290 participants included in the present analyses, 220 completed the technology familarity survey (see Nicosia et al., Reference Nicosia, Aschenbrenner, Adams, Tahan, Stout, Wilks, Balls-Berry, Morris and Hassenstab2021) which assessed the frequency with which participants perform smartphone-related tasks, how difficult participants find various technology-related tasks, and how well participants could recognize technology-related icons. Significant correlations (p < 0.05) are displayed with colored circles whereas non-significant relationships are blank.
A subset of participants (N = 228) also completed a user experience survey after their first ARC visitFootnote 4 . As shown in Figure 6, participants reported an overall positive experience with the ARC application, and most reported that they preferred ARC over conventional assessments. Participants reported little difficulty installing the ARC app, were generally unconcerned about privacy, and that completing 2 weeks of ARC testing per year would not be difficult.

Figure 6. ARC user experience survey results. Note. Of the 290 participants included in the present analyses, 228 completed the ARC user experience survey which assessed participants attitudes towards their experience with the ARC application after their first week using it.
Finally, as shown in Tables 1 and 2, adherence rates were quite high at 81% and 79% for CDR 0 and 0.5 participants, respectively. Drop-out rates were low for both groups as well – 4.9% for CDR 0 s and 4.5% for CDR 0.5 s. The high adherence and low drop-out rates suggest that ARC was well tolerated by older adults, even those with very mild dementia.
Discussion
The present study demonstrates that EMA cognitive assessments conducted on individuals’ personal smartphones can be reliable, sensitive to age and AD biomarkers, and are well-tolerated by older adults regardless of technology experience. There were several main findings: first, between-person reliability of the ARC tasks across the 7-day protocol all exceeded 0.85. Second, individual ARC tasks and the ARC composite score showed exceptionally good test-retest reliabilities at 6-month and 1-year follow-ups (ICCs > 0.85). Third, both the individual ARC tasks and the ARC composite score were correlated with conventional measures of the same domain (r’s = −0.22 to −0.57). The composite scores from ARC and conventional measures were also highly correlated (r = −0.53). Fourth, the ARC composite score showed similar validity to the global composite in predicting AD biomarkers. Finally, both cognitively normal older adults and individuals with very mild AD successfully participated in the ARC study remotely, without supervision, and had extremely low drop-out rates. Overall, the results of the present study suggest that high-frequency smartphone-based assessments are promising tools for assessing cognition in clinical studies of aging and neurodegenerative diseases.
Although classic neuropsychological tests, such as episodic memory and executive functioning tests, are regarded as the most sensitive to AD pathology, they were not designed for frequent assessment and can have poor reliability (Calamia et al., Reference Calamia, Markon and Tranel2013). Using measures with suboptimal reliability can impact statistical power and necessitate larger sample sizes or increased measurement frequency. Our results suggest that a high-frequency EMA approach to cognitive assessments may help overcome these challenges. When averaged across sessions, all three ARC tests had excellent between-subject reliability (r’s > 0.85), consistent with Sliwinski et al. (Reference Sliwinski, Mogle, Hyun, Munoz, Smyth and Lipton2018). The results also demonstrated that good between-person reliabilities can be achieved with < 7 days of assessments (averaging across 5 days produced reliabilities > 0.80 for all ARC tasks). The Symbols test achieved excellent reliability in just 3–5 sessions, which is remarkable considering that each session requires ∼30–40 s to complete. Although conventional cognitive measures would also receive a boost in reliability if averaged across repeated assessments, it is impractical and burdensome to assess participants at a frequency sufficient to overcome suboptimal reliability. Using an EMA smartphone protocol, researchers can efficiently obtain repeated measurements to boost reliability.
Test-retest reliability studies in AD samples have indicated “adequate” to “excellent” reliability (e.g., Benedict et al., Reference Benedict, Schretlen, Groninger and Brandt1998; Woods et al., Reference Woods, Delis, Scott, Kramer and Holdnack2006) over intervals ranging from several days to several weeks apart. However, cohort studies are typically conducted annually and yield lower reliability estimates. Specifically, test-retest correlations for delayed memory tests, a cornerstone of AD clinical trials (Bateman et al., Reference Bateman, Benzinger, Berry, Clifford, Duggan, Fagan, Fanning, Farlow, Hassenstab, McDade, Mills, Paumier, Quintana, Salloway, Santacruz, Schneider, Wang and Xiong2017; Donohue et al., Reference Donohue, Sperling, Salmon, Rentz, Raman, Thomas, Weiner and Aisen2014; Langbaum et al., Reference Langbaum, Hendrix, Ayutyanont, Chen, Fleisher, Shah, Barnes, Bennett, Tariot and Reiman2014; Ritchie et al., Reference Ritchie, Ropacki, Albala, Harrison, Kaye, Kramer, Randolph and Ritchie2017), can be particularly unsatisfactory, with reliabilities ranging from 0.50 to 0.75 (Calamia et al., Reference Calamia, Markon and Tranel2013; Dikmen et al., Reference Dikmen, Heaton, Grant and Temkin1999; Lo et al., Reference Lo, Humphreys, Byrne and Pachana2012). The increased reliability demonstrated by high-frequency assessments like ARC could substantially reduce sample sizes needed in AD prevention RCTs (Dodge et al., Reference Dodge, Zhu, Mattek, Austin, Kornfeld and Kaye2015).
ARC demonstrated exceptionally high test-retest reliability for the individual ARC tasks and the ARC composite score at 6-month and 1-year follow-ups (all ICCs > 0.85). The Symbols test demonstrated exceptionally high test-retest reliability exceeding its paper and pencil equivalents (i.e., Wechsler Digit Symbol Substitution test and the Symbol-Digit Modalities test which typically have good test-retest reliabilities; Calamia et al., Reference Calamia, Markon and Tranel2013; Pereira et al., Reference Pereira, Costa and Cerqueira2015). Test-retest reliability for the Prices test was also good but trailed behind the Symbols and Grids tests. Relatedly, a version of the Prices test demonstrated good validity and reliability in a recent EMA study of older adults (Thompson et al., Reference Thompson, Harrington, Roque, Strenger, Correia, Jones, Salloway and Sliwinski2022), but was also rated the most difficult and the least enjoyable of three cognitive tasks, reflecting the challenges of designing repeatable episodic memory measures that are reliable, feasible, and tolerable.
Our results also support the construct and predictive validity of ARC. ARC tasks exhibited convergent validity as evidenced by correlations with conventional cognitive measures (r’s −0.22 to −0.57). Similarly, the ARC composite score was correlated with the global composite score (r = −0.53). Albeit smaller than anticipated, the correlations observed here were comparable, if not stronger, than correlations observed in other digital assessment studies including the Cambridge Neuropsychological Test Automated Battery (CANTAB; rs 0.14 to 0.39; Dorociak et al., Reference Dorociak, Mattek, Lee, Leese, Bouranis, Imtiaz, Bernstein, Kaye and Hughes2021; Gills et al., Reference Gills, Glenn, Madero, Bott and Gray2019; Smith et al., Reference Smith, Need, Cirulli, Chiba-Falek and Attix2013). Additionally, the individual ARC tasks and the ARC composite score showed comparable correlations with age as the conventional measures and global composite score. Given well-known associations between age and cognitive performance, these relationships provide evidence that ARC is a valid measure of cognitive aging.
ARC also demonstrated good predictive validity when assessing sensitivity to AD biomarkers. Worse ARC performance was associated with reduced cortical thickness and hippocampal volume (r’s = −0.18 and −0.19, respectively) and increased levels of amyloid and tau (as indexed by both PET and CSF measures; r’s = 0.11 to 0.29). These relationships were comparable, though smaller in magnitude, to AD biomarker correlations with conventional measures suggesting that ARC captures biomarker burden similarly to conventional measures. Correlations in the cognitively normal subsample (CDR 0 individuals) were on par with other studies which have examined such relationships (Braak & Braak, Reference Braak and Braak1991; Papp et al., Reference Papp, Samaroo, Chou, Buckley, Schneider, Hsieh, Soberanes, Quiroz, Properzi, Schultz, García-Magariño, Marshall, Burke, Kumar, Snyder, Johnson, Rentz, Sperling and Amariglio2021; Snitz et al., Reference Snitz, Tudorascu, Yu, Campbell, Lopresti, Laymon, Minhas, Nadkarni, Aizenstein, Klunk, Weintraub, Gershon and Cohen2020; Van Strien et al., Reference Van Strien, Cappaert and Witter2009).
Evaluation of feasibility and tolerability of a smartphone application for use in older adults is critical, and especially so for applications like ARC that require unsupervised daily interactions. Overall, adherence was excellent at 80.42%, exceeding that seen in many remote studies (Pratap et al., Reference Pratap, Neto, Snyder, Stepnowsky, Elhadad, Grant, Mohebbi, Mooney, Suver, Wilbanks, Mangravite, Heagerty, Areán and Omberg2020) and similar to rates observed in other cognitive EMA studies (Sliwinski et al., Reference Sliwinski, Mogle, Hyun, Munoz, Smyth and Lipton2018). A common concern regarding technology use in older adults is that of technology familiarity. Our results demonstrate that greater technology knowledge was associated with better processing speed and visual working memory task performance, but not memory performance. Interestingly, self-reported frequency of smartphone interactions was not related to ARC performance, but those who reported more difficulty interacting with technology tended to perform worse on all ARC measures. However, when the familiarity assessment results were compared to conventional cognitive measures (see Supplemental Materials Figure 2), similar patterns emerged even on nontechnology-related measures like story recall, number span, confrontation naming, and verbal fluency, suggesting that difficulty with technology may also reflect, to some extent, overall cognitive abilityFootnote 5 . Finally, considering the high adherence rates, and the overall favorable ratings from the user experience survey, it appears that with adequate instruction and support, older adults are capable and motivated participants in smartphone studies of cognition.
Limitations and future considerations
The findings of this study should be considered in light of several limitations which may be addressed in future studies. First, although the benefits of EMA smartphone studies are clear, it can be unclear whether participants are fully engaging with the assigned tasks. To address this, participants are asked at the end of each session whether they were interrupted during the session. In the analyses presented here, sessions where participants reported being interrupted were removed. Similarly, many ambulatory assessments are limited when researchers do not collect additional contextual information. Participants were asked a battery of environmental questions at the end of each session, and future studies will investigate the impact of these factors on participants’ performance. Second, as noted in the Methods section, if an individual did not have a device which met study criteria, they were supplied a device. Since it is possible this could have introduced bias, several follow-up analyses were run to test for differences in age, technology familiarity, and ARC performance/adherence. As shown in Supplementary Materials Table 2, even though individuals who were supplied with a device were slightly older and less familiar with technology, there were no differences in CDR, ARC task performance, adherence, or AD biomarkers. Third, it is important to note that the Prices task lagged behind the Symbols and Grids tasks in terms of participants’ performance and the between-subjects reliability (possibly due to the difficulty and task demands). Nevertheless, the Prices task showed good reliability and was correlated with age and conventional memory measures. Finally, Knight ADRC participants consist of highly educated and primarily White older adults motivated to engage in extensive imaging and fluid biomarker studies. Future work is needed to determine the feasibility of ARC in more diverse populations.
Supplementary material
The supplementary material for this article can be found at https://doi.org/10.1017/S135561772200042X
Author contributions
AA, DAB, MJS, JCM, & JH conceptualized the study and acquired funding. AA, MT, SSS, & HW adminstered the project and supervised data collection. JN, AA, CX, & JH curated the data and conducted statistical analyses. All authors provided critical feedback on manuscript preparation and editing of revisions.
Funding statement
This work was supported by the National Institutes of Health Grants P30AG066444, P01AG03991, and P01AG026276 (PI Morris) and R01AG057840 (PI Hassenstab) and a grant from the BrightFocus Foundation A2018202S (PI Hassenstab). We would also like to thank the Shepard Family Foundation for their financial support.
Conflicts of interest
None.