Introduction
Subjective cognitive difficulties (SCDs) are common among older adults and involve the self-reported perception of worsening cognitive abilities (Jessen et al., Reference Jessen, Amariglio, Buckley, van der Flier, Han, Molinuevo, Rabin, Rentz, Rodriguez-Gomez, Saykin, Sikkes, Smart, Wolfsgruber and Wagner2020; Rohr et al., Reference Rohr, Pabst, Riedel-Heller, Jessen, Turana, Handajani, Brayne, Matthews, Stephan, Lipton, Katz, Wang, Guerchet, Preux, Mbelesso, Ritchie, Ancelin, Carriere, Guaita, Davin, Vaccaro, Kim, Han, Suh, Shahar, Din, Vanoh, van Boxtel, Kohler, Ganguli, Jacobsen, Snitz, Anstey, Cherbuin, Kumagai, Chen, Narazaki, Ng, Gao, Gwee, Brodaty, Kochan, Trollor, Lobo, Lopez-Anton, Santabarbara, Crawford, Lipnicki and Sachdev2020). The self-reported experience of cognitive changes can reflect individuals’ insight into objective cognitive changes as determined by neuropsychological assessment (Burmester, Leathem, & Merrick, Reference Burmester, Leathem and Merrick2016). Further, SCD has been correlated with neurobiological markers (e.g., hippocampal volume, amyloid burden; (Dauphinot et al., Reference Dauphinot, Bouteloup, Mangin, Vellas, Pasquier, Blanc, Hanon, Gabelle, Annweiler, David, Planche, Godefroy, Rivasseau-Jonveaux, Chupin, Fischer, Chene, Dufouil and Krolak-Salmon2020; Ebenau et al., Reference Ebenau, Timmers, Wesselman, Verberk, Verfaillie, Slot, van Harten, Teunissen, Barkhof, van den Bosch, van Leeuwenstijn, Tomassen, Braber, Visser, Prins, Sikkes, Scheltens, van Berckel and van der Flier2020) and risk factors (e.g., decreased white matter integrity; (Ohlhauser, Parker, Smart, Gawryluk, & Alzheimer’s Disease Neuroimaging, Reference Ohlhauser, Parker, Smart and Gawryluk2019) of neurodegenerative changes, and may be predictive of future cognitive decline (Ebenau et al., Reference Ebenau, Timmers, Wesselman, Verberk, Verfaillie, Slot, van Harten, Teunissen, Barkhof, van den Bosch, van Leeuwenstijn, Tomassen, Braber, Visser, Prins, Sikkes, Scheltens, van Berckel and van der Flier2020; Tsutsumimoto et al., Reference Tsutsumimoto, Makizako, Doi, Hotta, Nakakubo, Makino, Shimada and Suzuki2017).
SCD, however, can also occur with no evidence of these neurobiological changes or worsening clinical state over time, highlighting that there are other underlying factors that can influence SCD (Burmester et al., Reference Burmester, Leathem and Merrick2016). Higher levels of SCD are associated with demographic (race and education; (Rohr et al., Reference Rohr, Pabst, Riedel-Heller, Jessen, Turana, Handajani, Brayne, Matthews, Stephan, Lipton, Katz, Wang, Guerchet, Preux, Mbelesso, Ritchie, Ancelin, Carriere, Guaita, Davin, Vaccaro, Kim, Han, Suh, Shahar, Din, Vanoh, van Boxtel, Kohler, Ganguli, Jacobsen, Snitz, Anstey, Cherbuin, Kumagai, Chen, Narazaki, Ng, Gao, Gwee, Brodaty, Kochan, Trollor, Lobo, Lopez-Anton, Santabarbara, Crawford, Lipnicki and Sachdev2020), health (pain, TBI history; (Roberts et al., Reference Roberts, Zafonte, Speizer, Baggish, Taylor, Nadler and Weisskopf2021; Walton, Kerr, et al., Reference Walton, Kerr, Brett, Chandran, DeFreese, Smith-Ryan, Stoner, Echemendia, McCrea, Meehan and Guskiewicz2021; Westoby et al., Reference Westoby, Mallen and Thomas2009), psychological (depression, anxiety, personality) and situational factors (stress, chronic sleep disturbance, decreased activities and roles; (Ryu et al., Reference Ryu, Lee, Kim and Lee2016; Sabatini et al., Reference Sabatini, Woods, Ukoumunne, Ballard, Collins and Clare2021). Many of the aforementioned factors are highly prevalent and co-morbid in older adults. This can result in elevated SCD in the absence of objective changes and render self-reported cognition as less reliable and accurate.
Higher levels of SCD have been observed among former contact sport athletes (Alosco, M.L., Kasimis, A.B., Stamm, J.M., Chua, A.S., Baugh, C.M., Daneshvar, D.H., Robbins, C.A., Mariani, M., Hayden, J., Conneely, S., Au, R., Torres, A., McClean, M.D., McKee, A.C., Cantu, R.C., Mez, J., Nowinski, C.J., Martin, B.M., Chaisson, C.E., Tripodis, Y., & Stern, R.A. et al., Reference Alosco, Kasimis, Chua, Baugh, Daneshvar, Robbins, Mariani, Hayden, Conneely, Au, Torres, McClean, McKee, Cantu, Mez, Nowinski, Martin, Chaisson, Tripodis and Stern2017; Esopenko et al., Reference Esopenko, Chow, Tartaglia, Bacopulos, Kumar, Binns, Kennedy, Müller and Levine2017). However, other health, mood, and lifestyle factors are also commonly present, often co-occurring, among former contact sport athletes as well (Brett et al., Reference Brett, Walton, Kerr, Nelson, Chandran, Defreese, Echemendia, Guskiewicz, Meehan III and McCrea2021; Esopenko et al., Reference Esopenko, Chow, Tartaglia, Bacopulos, Kumar, Binns, Kennedy, Müller and Levine2017; Iverson et al., Reference Iverson, Van Patten, Terry, Levi and Gardner2021; Kerr et al., Reference Kerr, Marshall, Harding and Guskiewicz2012; Prien et al., Reference Prien, Feddermann-Demont, Verhagen, Twisk and Junge2020; Schwenk et al., Reference Schwenk, Gorenflo, Dopp and Hipple2007). For example, interrelated relationships between pain, depression, and chronic sleep disturbance, have been observed in former elite-level contact sport athletes (Brett et al., Reference Brett, Walton, Kerr, Nelson, Chandran, Defreese, Echemendia, Guskiewicz, Meehan III and McCrea2021; Iverson, Van Patten, et al., Reference Iverson, Van Patten, Terry, Levi and Gardner2021; Schwenk et al., Reference Schwenk, Gorenflo, Dopp and Hipple2007). Given the common comorbidity and dynamic relationships (e.g., increased pain can positively influence sleep disturbance and depression symptoms) of these factors, identifying sources of the greatest contribution to difficulties can be challenging, but doing so may improve the efficacy of targeted treatment strategies. As such, it is critical to gain a greater understanding of the relative influence of these factors on SCD to optimize treatment and prognosis in older adults. Given the notable prevalence and high comorbidities of these factors with SCD among former contact sport athletes and in the general population, it is critical to differentiate which factors are associated with SCD among those with and without documented cognitive impairment (Brett et al., Reference Brett, Walton, Kerr, Nelson, Chandran, Defreese, Echemendia, Guskiewicz, Meehan III and McCrea2021; Esopenko et al., Reference Esopenko, Chow, Tartaglia, Bacopulos, Kumar, Binns, Kennedy, Müller and Levine2017; Iverson, Van Patten, et al., Reference Iverson, Van Patten, Terry, Levi and Gardner2021; Kerr et al., Reference Kerr, Marshall, Harding and Guskiewicz2012; Schwenk et al., Reference Schwenk, Gorenflo, Dopp and Hipple2007).
The first aim of the study was to investigate the relative influence of comorbid contributing factors on SCD through dominance analysis. Through this approach, it can be determined which factors are more likely to contribute to SCD, as compared to other factors, regardless of the combinations and numbers of other SCD-factors considered/present (i.e., complete dominance). In other words, if factor 1 completely dominates factor 2, the number or different combinations of other factors that can increase SCD will not alter the fact that factor 1 influences SCD at a greater level than factor 2. Dominance analysis also allows for examination of which factors exhibt greater average conditional (when the same number of SCD-factors are considered) contribution to SCD across different combinations of models (i.e., general dominance; see below for further description). Secondly, the study aimed to identify these factors’ differential associations with SCD among those with and without documented cognitive impairment (i.e., diagnosis of mild cognitive impairment; MCI), given that comorbid factors can differ among those with and without documented cognitive impairment (Benito-Leon et al., Reference Benito-Leon, Mitchell, Vega and Bermejo-Pareja2010; Markova et al., Reference Markova, Nikolai, Mazancova, Cechova, Sheardova, Georgi, Kopecek, Laczo, Hort and Vyhnalek2019).
Methods
Participants
Inclusion criteria for the study required one-year of professional American football in the National Football League (NFL). Players were recruited through multiple means, including individual team and organizational (e.g., NFL Alumni Association) communications (e.g., newsletter notification, mailed letters) via email and hard copy questionnaires. Overall, 15,025 former NFL players were contacted and 1,784 (11.9%) completed a health and function questionnaire, 989 who were aged ≥ 50-years (Brett et al., Reference Brett, Walton, Kerr, Nelson, Chandran, Defreese, Echemendia, Guskiewicz, Meehan III and McCrea2021; Walton, Kerr, et al., Reference Walton, Kerr, Brett, Chandran, DeFreese, Smith-Ryan, Stoner, Echemendia, McCrea, Meehan and Guskiewicz2021). Given that anosognosia (i.e., decreased insight into cognitive symptoms and lower endorsement) increases with greater disease severity, individuals who indicated a physician-based diagnosis of dementia (n = 82; n=75 also reporting a diagnosis of MCI) were excluded, leaving 907 for analyses (Supplemental Figure 1; (Edmonds et al., Reference Edmonds, Weigand, Thomas, Eppig, Delano-Wood, Galasko, Salmon and Bondi2018). The study was carried out in accordance with the Declaration of Helsinki and approved by an Institutional Review Board at the Medical College of Wisconsin and University of North Carolina at Chapel Hill #19-1065 and participants provided written informed consent prior to any study activities.
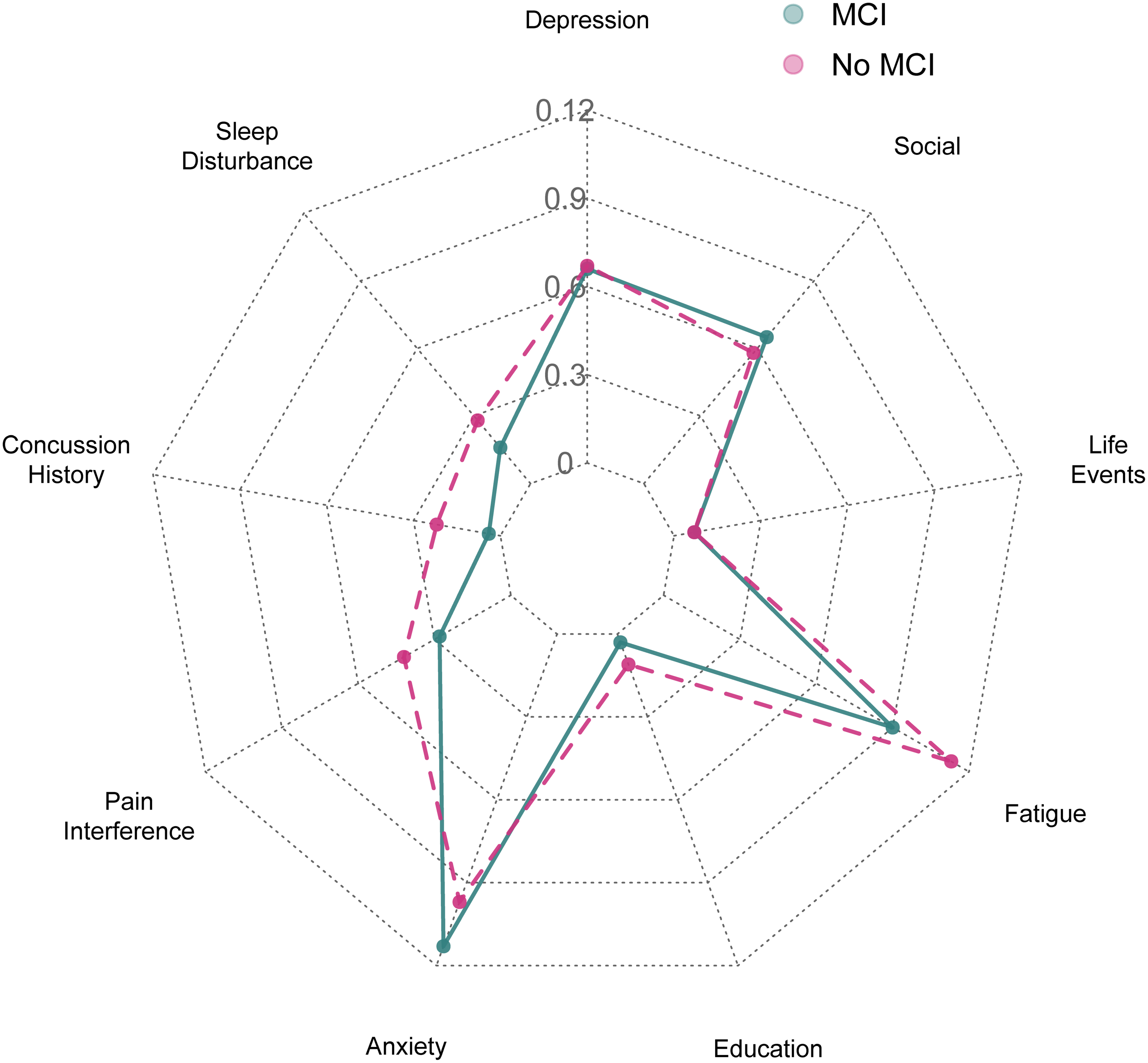
Figure 1. Radar plot of average contributions of SCD-associated factors of explained variance in self-report ratings of general cognition in MCI and non-MCI groups. Numerical scale of figure represents R2 value contribution of average SCD-associated factor. Z-score transformations revealed that the strength of the association (based on average contribution of variance explained via general dominance) between each SCD-associated factor and ratings of general cognition across MCI and non-MCI groups did not statistically differ (Z-statistics <1.96, ps>.05).
The comprehensive health and function questionnaire completed by participants involved demographic information, medical history, concussion history, years of American football participation, and various self-reported measures of health and functioning. The questionnaire was adapted from a previously utilized general health survey in this population and was further modified to include additional previously well-validated inventories of neurobehavioral function, psychosocial function, health-promoting behaviors, and other domains (Guskiewicz et al., Reference Guskiewicz, Marshall, Bailes, McCrea, Cantu, Randolph and Jordan2005; Guskiewicz et al., Reference Guskiewicz, Marshall, Bailes, McCrea, Harding, Matthews, Mihalik and Cantu2007). Modifications to the questionnaire were made based on contributions from neuropsychologists, athletic trainers, epidemiologists, physicians, and other sports medicine professionals. These individuals helped to refine aspects of the previous survey (e.g., health/injury history questions) and selected well-validated instruments based on their area of expertise (e.g., physical activity and diet questionnaires selected by exercise physiologists; see below for instruments utilized in the current study and description of their validation in prior studies). Additionally, former NFL players pilot tested the questionnaire and provided input to improve the questionnaire’s administration and clarity.
Participants reported the number of prior concussions (medically diagnosed and those not medically diagnosed) based on a standard definition provided as part of the questionnaire (McCrea et al., Reference McCrea, Hammeke, Olsen, Leo and Guskiewicz2004). The definition provided included: “A concussion typically occurs from a blow to the head and is followed by a variety of symptoms that may include any of the following: headache, dizziness, loss of balance, blurred vision, ‘seeing stars’, feeling in a fog, or slowed down, memory problems, poor concentration, nausea, or throwing-up. Getting ‘knocked out’ or being unconscious does NOT always occur with a concussion.” Participants reported the presence of MCI (yes/no) by indicating that they been diagnosed by a physician or other healthcare professional.
Comorbid contributing factors to SCD
Factors were selected a priori based on previous investigations included 1) depression symptom severity (Roberts et al., Reference Roberts, Zafonte, Speizer, Baggish, Taylor, Nadler and Weisskopf2021; Schwenk et al., Reference Schwenk, Gorenflo, Dopp and Hipple2007), 2) anxiety symptom severity (Roberts et al., Reference Roberts, Zafonte, Speizer, Baggish, Taylor, Nadler and Weisskopf2021; Sabatini et al., Reference Sabatini, Woods, Ukoumunne, Ballard, Collins and Clare2021), 3) sleep disturbance (Stenfors et al., Reference Stenfors, Marklund, Magnusson Hanson, Theorell and Nilsson2013), 4) pain interference (Schwenk et al., Reference Schwenk, Gorenflo, Dopp and Hipple2007; Westoby et al., Reference Westoby, Mallen and Thomas2009), 5) ability participate in social roles and activities (Ryu et al., Reference Ryu, Lee, Kim and Lee2016), 6) level of current stress-related life events (Nelson et al., Reference Nelson, Gavelin, Boraxbekk, Eskilsson, Josefsson, Slunga Jarvholm and Neely2021), 7) fatigue (Rasouli et al., Reference Rasouli, Gotaas, Stensdotter, Skovlund, Landro, Dastol and Fors2019), 8) concussion history (Roberts et al., Reference Roberts, Zafonte, Speizer, Baggish, Taylor, Nadler and Weisskopf2021), and 9) education (Rohr et al., Reference Rohr, Pabst, Riedel-Heller, Jessen, Turana, Handajani, Brayne, Matthews, Stephan, Lipton, Katz, Wang, Guerchet, Preux, Mbelesso, Ritchie, Ancelin, Carriere, Guaita, Davin, Vaccaro, Kim, Han, Suh, Shahar, Din, Vanoh, van Boxtel, Kohler, Ganguli, Jacobsen, Snitz, Anstey, Cherbuin, Kumagai, Chen, Narazaki, Ng, Gao, Gwee, Brodaty, Kochan, Trollor, Lobo, Lopez-Anton, Santabarbara, Crawford, Lipnicki and Sachdev2020). Current level of stress-related events was measured using the Holmes and Rahe Stress Inventory, which is comprised of 43 items that represent different quantified (i.e., points) stressors that can be summed for a total score. Similar to previous research, self-reported lifetime concussion history was binned into five categories: 0, 1–2, 3–5, 6–9, and 10+ (Walton, Kerr, et al., Reference Walton, Kerr, Brett, Chandran, DeFreese, Smith-Ryan, Stoner, Echemendia, McCrea, Meehan and Guskiewicz2021). Education was characterized as a categorical variable with three levels that included attainment of less than bachelor’s degree, bachelor’s degree, and professional degree (e.g., MS, JD, PhD).
The Patient Reported Outcomes Measurement Information System-29 (PROMIS-29 v2.1) is comprised of multiple PROMIS Short Form inventories corresponding with several of SCD-associated factors above (Rose et al., Reference Rose, Bayliss, Huang, Baseman, Butcher, Garcia and Edelen2018). PROMIS Short Form inventories for each of the included domains were adapted from the original full item measures (initially developed from databanks involving over 15,000 respondents across the United States) using advanced analytical approaches, such as item response theory, in order to yield maximum information with minimal items administered (Cella et al., Reference Cella, Choi, Condon, Schalet, Hays, Rothrock, Yount, Cook, Gershon, Amtmann, DeWalt, Pilkonis, Stone, Weinfurt and Reeve2019). All PROMIS Short-Form inventories included as SCD-associated factors consist of four items per construct. Previous studies have reported on the psychometric properties of the PROMIS Short Form inventories and their aggregation as the PROMIS-29, and established various forms of validity (e.g., content, concurrent, ecological, discriminant validity) in the general population and various clinical populations (e.g, Cella et al., Reference Cella, Choi, Condon, Schalet, Hays, Rothrock, Yount, Cook, Gershon, Amtmann, DeWalt, Pilkonis, Stone, Weinfurt and Reeve2019; Deyo et al., Reference Deyo, Katrina, Buckley, Michaels, Kobus, Eckstrom, Forro and Morris2016; LaVela et al., Reference LaVela, Etingen, Miskevics and Cella2019; Riley et al., Reference Riley, Rothrock, Bruce, Christodolou, Cook, Hahn and Cella2010; Rose et al., Reference Rose, Bayliss, Huang, Baseman, Butcher, Garcia and Edelen2018). See the following examples of psychometric validation studies for each of the PROMIS Short Form inventories included as part of the PROMIS-29 that were SCD-associated factors of interest for the current study; Anxiety (Pilkonis et al., Reference Pilkonis, Choi, Reise, Stover, Riley and Cella2011; Schalet et al., Reference Schalet, Pilkonis, Yu, Dodds, Johnston, Yount, Riley and Cella2016), Depression (Pilkonis et al., Reference Pilkonis, Choi, Reise, Stover, Riley and Cella2011; Schalet et al., Reference Schalet, Pilkonis, Yu, Dodds, Johnston, Yount, Riley and Cella2016; Teresi et al., Reference Teresi, Ocepek-Welikson, Kleinman, Ramirez and Kim2016), Sleep Disturbance (Full et al., Reference Full, Malhotra, Crist, Moran and Kerr2019; Yu et al., Reference Yu, Buysse, Germain, Moul, Stover, Dodds, Johnston and Pilkonis2011), Fatigue (Broderick et al., Reference Broderick, Schneider, Junghaenel, Schwartz and Stone2013; LaVela et al., Reference LaVela, Etingen, Miskevics and Cella2019), Ability to Participate in Social Roles and Activities (Bode, Hahn, DeVellis, Cella, & Patient-Reported Outcomes Measurement Information System Social Domain Working, Reference Bode, Hahn, DeVellis and Cella2010; Terwee et al., Reference Terwee, Crins, Boers, de Vet and Roorda2019), and Pain Interference (Broderick et al., Reference Broderick, Schneider, Junghaenel, Schwartz and Stone2013; Deyo et al., Reference Deyo, Katrina, Buckley, Michaels, Kobus, Eckstrom, Forro and Morris2016). All items from PROMIS-29 Short Form inventories were rated on a 5-point Likert scale and individual participant responses (i.e., raw sum scores) for all measures were converted to t-scores (mean = 50 and standard deviation, SD = 10) based on normative data obtained from the general population. Higher t-scores on the Anxiety, Depression, Sleep Disturbance, Fatigue, and Pain Interference inventories indicated greater dysfunction (i.e., poorer) in these domains. Higher T-scores on the Ability to Participate in Social Roles and Activities domain indicated higher levels (i.e., better) of function.
SCD outcomes
The primary outcome of interest, SCD, was measured using the PROMIS Cognitive Function Short-Form v2.0, which yields a total score based on items reflecting various aspects of cognition such as attention/concentration, memory, processing speed, and executive function (multitasking/mental flexibility; (Lai et al., Reference Lai, Wagner, Jacobsen and Cella2014). Given that neurobehavioral dysfunction has also been reported as a common corollary of cumulative concussion among former NFL players (Montenigro et al., Reference Montenigro, Alosco, Martin, Daneshvar, Mez, Chaisson, Nowinski, Au, McKee, Cantu, McClean, Stern and Tripodis2017), a secondary SCD-associated outcome included the Quality of Life in Neurological Disorders (Neuro-QoL) Emotional and Behavioral Dyscontrol v1.0 (Cella et al., Reference Cella, Lai, Nowinski, Victorson, Peterman, Miller, Bethoux, Heinemann, Rubin, Cavazos, Reder, Sufit, Simuni, Holmes, Siderowf, Wojna, Bode, McKinney, Podrabsky, Wortman, Choi, Gershon, Rothrock and Moy2012). Items from the Neuro-QoL Emotional and Behavioral Dyscontrol reflect various aspects of neurobehavioral dysfunction, such as emotional lability, disinhibition, irritability/impatience, and impulsiveness.
Similar to the other PROMIS measures noted above, prior studies investigating the psychometric properties of the PROMIS Cognitive Function (Iverson et al., Reference Iverson, Marsh, Connors and Terry2021; Thackeray et al., Reference Thackeray, Marcus, Yu, McCracken, Cardell and Hanmer2021) and Emotional and Behavioral Dyscontrol (Gershon et al., Reference Gershon, Lai, Bode, Choi, Moy, Bleck, Miller, Peterman and Cella2012) have established various forms validity (e.g., construct validity) across the general population and multiple clinical populations (e.g., Becker et al., Reference Becker, Stuifbergen, Lee and Kullberg2014; Kozlowski et al., Reference Kozlowski, Cella, Nitsch and Heinemann2016; Nowinski et al., Reference Nowinski, Siderowf, Simuni, Wortman, Moy and Cella2016; Valentine et al., Reference Valentine, Weiss, Jones and Andersen2019; Victorson et al., Reference Victorson, Cavazos, Holmes, Reder, Wojna, Nowinski and Cella2014). PROMIS Cognitive Function Short-Form (four items) and Neuro-QoL Emotional and Behavioral Dyscontrol Short-Form (eight items) items were rated on a 5-point Likert scale and raw sum were converted to T-scores (mean = 50 and standard deviation, SD = 10) based on normative data obtained from the general population. Greater dysfunction in the domain of Emotional and Behavioral Dyscontrol is represented by higher T-scores. Higher T-scores on the Cognitive Function indicated higher levels of function (i.e., better self-rated cognitive function).
Statistical analysis
Statistical analyses were performed in R (version 4.0.5). Associations between missing and observed data were not observed upon inspection of visual plots (i.e., missing at random) and as such, multiple imputation by chained equations (five iterations) was utilized in order to address missingness and maintain cases (Zhang, Reference Zhang2016). Missingness frequency and percentages for variables of interest provided in Supplemental Table 1. Independent sample t-tests compared SCD-associated outcomes across MCI and non-MCI groups and effect sizes (Cohen’s d) were calculated (0.0–0.2=small, 0.3–0.5=medium, ≥0.8=large; (Cohen, Reference Cohen1988). Single-predictor regression analyses were first performed to ensure that a priori selected comorbid contributing factors were significantly associated with SCD scores for the MCI and non-MCI group. A multivariable linear regression model including all factors was then fit for each outcome. Sensitivity analyses involving similar multivariable linear regression models that included participants with a diagnosis of dementia as part of the MCI group were also fit (Supplemental Table 2).
In order to determine the relative influence of each comorbid contributing factor on SCD outcomes, dominance analyses were performed for each outcome, with bootstrap sampling (1000 samples) for estimation of SE and confidence intervals in MCI and non-MCI groups. Dominance analysis was developed as a method to determine the relative importance of each predictor within multivariable regression models by comparing their unique contributions to the variance explained in the dependent variable across all subsets of predictors (Azen & Budescu, Reference Azen and Budescu2003). By examining the increase in variance explained across all possible models, this method allows for comparing the additional contribution of each predictor to equivalent subsets of models (e.g., X1 added to model X2 + X3, X2 added to model X1 + X3, X3 added to X1 + X2). Given the high correlation between comorbid contributing factors (Brett et al., Reference Brett, Walton, Kerr, Nelson, Chandran, Defreese, Echemendia, Guskiewicz, Meehan III and McCrea2021; Esopenko et al., Reference Esopenko, Chow, Tartaglia, Bacopulos, Kumar, Binns, Kennedy, Müller and Levine2017; Iverson, Van Patten, et al., Reference Iverson, Van Patten, Terry, Levi and Gardner2021; Kerr et al., Reference Kerr, Marshall, Harding and Guskiewicz2012; Schwenk et al., Reference Schwenk, Gorenflo, Dopp and Hipple2007), this method is advantageous in determining unique contribution of these factors to levels of subjectively rated cognition, as one factor’s additional contribution to the outcome could drastically differ depending on which other variables are included in the model; as well as the fact that a one-unit increase in one factor can simultaneously influence the outcome directly and indirectly through an increase in another factor (increase in pain can simultaneously increase depression, both acting on the outcome).
Complete dominance of one factor over another is established if it consistently contributes additional variance in the outcome over another predictor across all model subsets (as measured by increase in R 2 ). If complete dominance is not observed, two lower levels of dominance, conditional and general, can be established. Conditional dominance can be established if the average additional contribution of explained variance of one factor over another is consistently recorded for models within each size (i.e., every combination of models with the same number of predictors). General dominance of a factor involves a greater average of conditional contributions across all model sizes over another. If complete dominance is achieved, conditional and general dominance is implied. Comparison of the average contribution of variance accounted for (i.e., based on general dominance of the analysis) by each SCD-associated factor for the two outcomes of interests between the MCI and non-MCI groups was performed based on a Z-statistic distribution, derived from Fisher’s r to z transformation (i.e., is the comorbid contributing factor more strongly related to SCD in MCI vs. the non-MCI group). Statistical significance for all analyses was evaluated at the 0.05 level.
Results
The final sample included 907 former NFL players with a mean age of 64.7 ± 8.9 years (Table 1). A diagnosis of MCI was reported by 18.2% (n = 165) of the sample. Those with MCI endorsed greater general cognitive difficulties ([t(905)=14.03, p<.001, d = 1.31; mean difference=10.4]) and emotional-behavioral dyscontrol (t[905]=−9.12, p<.001, d = 0.78; mean difference=−7.5). Single-predictor analyses of the nine individual comorbid contributing factors confirmed significant associations between these factors and SCD outcomes for the non-MCI group (Table 2). Within the MCI group, univariate analyses indicated most SCD factors were significantly associated with SCD outcomes, except for education and concussion history (significantly associated with emotional and behavioral dyscontrol, but not with general cognitive function). Associations between years of football participation and SCD-outcomes were not observed in the MCI (general cognition, B=−.01[0.11], p=.903; emotional and behavioral dyscontrol, B = −0.16[0.16], p=.303) or non-MCI groups (general cognition, B = 0.02[0.07], p=.754; emotional and behavioral dyscontrol, B = −0.01[0.07], p=.938); as such, they were not included in the dominance analyses.
Table 1 Participant information and characteristics
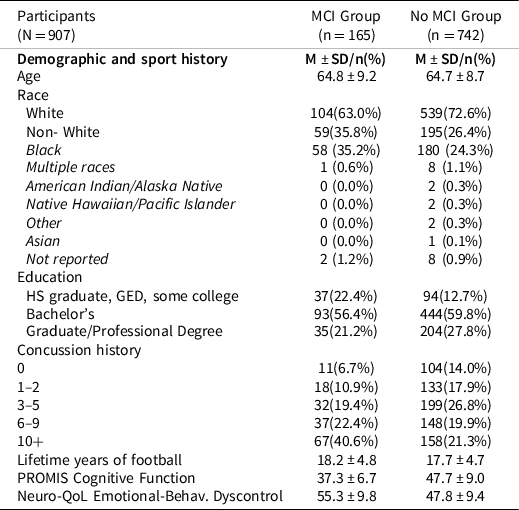
Table 2. Single-predictor analyses of individual comorbid contributing factors and SCD outcomes

MCI=mild cognitive impairment; B=unstandardized beta coefficient; SE=standard error; R2=coefficient of determination; social=ability to participate in social roles and activities; education 1 unit increase= increase in education level from below bachelor’s degree, bachelor’s degree, and professional degree; concussion history 1 unit increase=increase in concussion history group from 0, 1–2, 3–5, 6–9, and 10+; Depression, Sleep Disturbance, Pain Interference, Anxiety, and Ability to Participate in Social Roles and activities 1 unit increase = 1 T-score point change. Stress-Related Events =Social Readjustment Rating Scale (SRRS), 1 unit=1 point increase on the SRRS; Higher T-scores on the Anxiety, Depression, Sleep Disturbance, and Pain Interference indicate greater (i.e., poorer) dysfunction in that area. Higher T-scores on the Ability to Participate in Social Roles and Activities inventory indicated higher levels (i.e., better) of function on these domains.
There were no statistically significant associations between race (White and non-White participants) and emotional and behavioral dyscontrol in the MCI (B = 3.03[1.55], p=.053) and non-MCI groups (B = 0.26[0.77], p=.739). There was no statistically significant association between race and general cognition in the MCI group (B = −1.68[1.10], p=.128). Within the non-MCI group, subjective ratings of general cognition were significantly lower in non-White participants (Mean t-score=46.14) as compared to White participants (Mean t-score=48.27; B = −2.13[0.74], p=.004). Further examination of specific racial identity groups for the non-MCI participants revealed a high degree of heterogeneity in subjective ratings of general cognition across individual groups within the non-White category (e.g., Asian mean t-score=37.9; Multiple Races mean t-score=42.79; Black mean t-score=46.45), indicating that aggregation of all groups into a single category would not be appropriate. Given the high degree of heterogeneity across individual groups, low statistical power for individual group comparison, and the desire to avoid systematically excluding smaller individual racial identity groups in the sample, race was not included in the subsequent dominance analyses.
Comorbid contributing factors of general cognition
General SCD in MCI
Only anxiety (B = −0.26[0.08], p=.002) and fatigue (B = −0.16[0.07], p=.022) remained significantly associated with subjectively rated general cognition in the multivariable model that included all nine SCD-associated factors within the MCI group (Table 3). The bootstrap dominance analysis of subjectively rated cognition revealed that complete dominance was established for anxiety over the other six comorbid contributing factors, but not social roles and activities or fatigue (Supplementary Table 3; summary of all dominance analyses for each outcome across MCI and non-MCI groups provided in Table 4). Conditional dominance was established for fatigue over five comorbid contributing factors (sleep disturbance, concussion history, pain, education, stress-related life events). Additionally, conditional dominance for ability to participate in social roles and activities was established over education within bootstrap results. Various combinations of the remaining SCD-associated factors were observed as establishing general dominance over others (Supplementary Table 3; strength of variable effect listed in order for all supplementary tables), with depression and pain being the most robust factors (i.e., general dominance over the remaining variables).
Table 3. Multivariable models of all comorbid contributing factors with general cognition and emotional and behavioral dyscontrol

MCI=mild cognitive impairment; B=unstandardized beta coefficient; SE=standard error; β=standardized coefficient; social=Ability to Participate in Social Roles and Activities; education 1 unit increase= increase in education level from below bachelor’s degree, bachelor’s degree, and professional degree; concussion history 1 unit increase=increase in concussion history group from 0, 1–2, 3–5, 6–9, and 10+; Depression, Sleep Disturbance, Pain Interference, Anxiety, and Ability to Participate in Social Roles and activities 1 unit increase = 1 T-score point change. Stress-Related Events =Social Readjustment Rating Scale (SRRS), 1 unit=1 point increase on the SRRS; Higher T-scores on the Anxiety, Depression, Sleep Disturbance, and Pain Interference indicate greater (i.e., poorer) dysfunction in that area. Higher T-scores on the Ability to Participate in Social Roles and Activities inventory indicated higher levels (i.e., better) of function on these domains; MCI multivariable with Cognition as the dependent variable, R2=.390; MCI multivariable with emotional and behavioral dyscontrol as the dependent variable, R2=.430; Non-MCI multivariable with Cognition as the dependent variable, R2=.445; Non-MCI multivariable with emotional and behavioral dyscontrol as the dependent variable, R2=.409.
Table 4. Summary of bootstrap dominance analysis results of comorbid contributing factors with ratings of general cognition and emotional and behavioral dyscontrol in MCI and non-MCI groups

A=dominance at that level for general cognition in MCI group (factori over factorj); B=dominance at that level for general cognition in the non-MCI group (factori over factorj); C=dominance at that level for emotional and behavioral dyscontrol in MCI group (factori over factorj); D=dominance at that level for emotional and behavioral dyscontrol in the non-MCI group (factori over factorj); *Factorj over factori for “D”
General SCD in non-MCI
A multivariable model showed that anxiety (B=−.25[0.05], p<.001), fatigue (B=−.27[0.04], p<.001), concussion history (unstandardized beta[standard error]; B=−.66[0.19], p<.001), and education (B = 1.21[0.40], p=.003) were significantly associated with greater general SCD among former players without a diagnosis of MCI (Table 3). Bootstrap dominance analysis indicated complete dominance of anxiety over depression, sleep disturbance, concussion history, education and stress-related life events, as well as conditional dominance over pain and ability to participate in social roles and activities. Complete or conditional dominance was established for fatigue over all comorbid contributing factors except for anxiety. Complete and conditional dominance over stress-related life events was established for concussion history and ability to participate in social roles and activities, respectively. Aside from the most robust factors (anxiety and fatigue), the other comorbid contributing factors exhibited various patterns of general dominance (Supplementary Table 4; Table 4).
Group differences in comorbid contributing factors of general SCD
Based on the general dominance level (i.e., average contribution to model R 2 across all possible model sizes), anxiety and ability to participate in social roles and activities contributed more to general SCD in the MCI group than non-MCI group (Table 5; Figure 1). Conversely, fatigue, pain interference, sleep disturbance, concussion history and education were more likely to be associated with general SCD in non-MCI participants. The largest differences in average contribution to SCD of general cognition across the non-MCI and MCI groups included fatigue (R2 diff = −.023) and concussion history (R2 diff = −.018), where these factors had a greater influence on SCD in the non-MCI group. The average contributions of variance explained by each comorbid contributing factor for ratings of general cognition across MCI and non-MCI groups did not significantly differ (Z-statistic <1.96, ps>.05).
Table 5. Bootstrap estimates of average contribution of all comorbid contributing factors to variance explained in players’ ratings of general cognition and emotional and behavioral dyscontrol based on dominance analysis

MCI=mild cognitive impairment; B=unstandardized beta coefficient; SE=standard error; β=standardized coefficient; social=Ability to Participate in Social Roles and Activities; Stress-Related Events =Social Readjustment Rating Scale (SRRS), 1 unit=1 point increase on the SRRS; average contribution=average contribution of unique variance explained by the factor in the outcome across all combinations of models (i.e., R2). Z-statistics and corresponding p-values reflect comparison of the strength of association between the average contribution of each SCD-associated factor and the outcomes of interests between the MCI and non-MCI groups based on Fisher’s r to z transformations.
Comorbid contributing factors of emotional and behavioral dyscontrol
Emotional and behavioral dyscontrol in MCI
A multivariable model indicated that only anxiety (B = 0.51[0.11], p<.001) and education (higher emotional and behavioral dyscontrol among higher education; B = 2.84[0.93], p = .003) remained significantly associated with ratings of emotional and behavioral dyscontrol. The corresponding bootstrap dominance analysis indicated complete dominance of anxiety over all other eight comorbid contributing factors. Depression exhibited conditional dominance over stress-related life-events. The remaining comorbid contributing factors exhibited various patterns of general dominance, with average unique contributions across the same-level models provided in Supplementary Table 5.
Emotional and behavioral dyscontrol in non-MCI
Depression (B = 0.14[0.06], p = .019), anxiety (B = 0.24[0.06], p<.001), fatigue (unstandardized beta[standard error]; B = 0.20[0.04], p<.001), and ability to participate in social roles and activities (B = −0.11[0.05], p = .027) remained significantly associated with ratings of emotional and behavioral dyscontrol in a multivariable model. There was complete dominance of fatigue over sleep disturbance, concussion history, pain, education, stress-related events, and ability to participate in social roles and activities. Complete dominance was established for anxiety over the same variables with the exception of pain and ability to participate in social roles and activities, in which conditional dominance was observed. Conditional dominance was also observed for depression and ability to participate in social roles and activities over sleep disturbance. Depression exhibited general dominance over ability to participate in social roles and activities, and both exhibited general dominance over the remaining comorbid contributing factors (Supplementary Table 6).
Group differences in comorbid contributing factors of emotional and behavioral dyscontrol
Anxiety and education explained greater endorsement of emotional and behavioral dyscontrol in the MCI group than non-MCI group across equal sets of models (Table 5; Figure 2). Conversely, fatigue, depression, pain interference, and sleep disturbance were more likely to contribute explained variance in endorsement of emotional and behavioral dyscontrol in non-MCI participants. Z-score transformations revealed that the average contributions of variance explained by each SCD-associated factor for ratings of emotional and behavioral dyscontrol across MCI and non-MCI groups did not significantly differ (Z-statistics <1.96, ps>.05).

Figure 2. Radar plot of average contributions of SCD-associated factors of explained variance in self-report ratings of emotional and behavioral dyscontrol in MCI and non-MCI groups (i.e., general dominance). Numerical scale of figure represents R2 value contribution of average SCD-associated factor. Z-score transformations revealed that the strength of the association (based on average contribution of variance explained via general dominance) between each SCD-associated factor and ratings of emotional and behavioral dyscontrol across MCI and non-MCI groups did not statistically differ (Z-statistics <1.96, ps>.05).
Discussion
A number of factors that are particularly prevalent in older former contact sport athletes are associated with elevated SCD. Any combination these factors is highly comorbid at clinical presentation, with or without objective evidence of cognitive impairment. Anxiety and fatigue are the most robust factors associated with SCD in former professional football players, though fatigue was a more robust predictor of SCD regardless of other factors in former players without a diagnosis of MCI. Depression was more robustly associated with endorsement of emotional and behavioral dyscontrol over other factors, as compared to general cognition. Patterns of the relative strength of comorbid contributing factors between those with and without MCI, did not significantly differ. As such, the presence of comorbid contributing factors can similarly influence subjectively reported cognitive complaints in those with and without documented cognitive impairment, and self-reported cognitive deficits may be less reliable in detecting objective cognitive decline in the presence of these factors.
Comorbid contributing factors in MCI and non-MCI
Within both the MCI and non-MCI groups, anxiety was the strongest and most robust factor associated with SCD reflecting general cognition, regardless of which number or combination of variables were considered. Prior studies have reported a positive association between anxiety symptoms and greater SCD among those with and without objectively documented cognitive impairment in the general population and former NFL players (Jenkins et al., Reference Jenkins, Tree, Thornton and Tales2019; Yates et al., Reference Yates, Clare, Woods and Mrc2017). Although there has been some debate as to whether SCD purely represents a manifestation of anxiety among older adults or reflects concern about true cognitive changes experienced by the individual, anxiety and SCD have also been observed as independent risk factors for future cognitive decline (i.e., onset of MCI or dementia; (Liew, Reference Liew2020). Furthermore, the co-occurrence of both anxiety and SCD together confers twice the risk of developing MCI or dementia than either risk factor in isolation (Liew, Reference Liew2020). In combination with results from the current study, in which the association between anxiety and subjective ratings of cognition were comparable across MCI and non-MCI groups, this suggests that anxiety can likely represent general concern about potential cognitive changes in the absence objective changes. Additionally, anxiety could also potentially reflect a reaction to experienced cognitive decline.
Fatigue was also observed as one of the strongest correlates of SCD in both the MCI and non-MCI groups. However, fatigue was a notably more robust factor associated with SCD (general cognition and emotional and behavioral dyscontrol) among those without, as compared to those with MCI. Specifically, the number and combination of factors present were more likely to change the association between fatigue and SCD-outcomes among individuals with MCI. Associations between fatigue and SCD, but not objective impairment, have primarily been investigated in conditions outside of neurodegenerative disease/dementia, such as multiple sclerosis and stroke (Kinsinger et al., Reference Kinsinger, Lattie and Mohr2010; van Rijsbergen et al., Reference van Rijsbergen, Mark, Kop, de Kort and Sitskoorn2019). Studies investigating fatigue in non-clinical populations have reported positive associations between fatigue and SCD among those with and without objective cognitive deficits (Nelson et al., Reference Nelson, Gavelin, Boraxbekk, Eskilsson, Josefsson, Slunga Jarvholm and Neely2021; Rasouli et al., Reference Rasouli, Gotaas, Stensdotter, Skovlund, Landro, Dastol and Fors2019). Taken together, the presence of fatigue among those reporting SCD likely represents a complex bidirectional relationship, similar to that of SCD and anxiety, and does not differentiate those with and without objective cognitive impairment in former professional football players.
The current study demonstrated that the relative influence of comorbid contributing factors on SCD-outcomes were broadly equivalent across MCI and non-MCI groups. A slight variation was observed between the two groups, where the influence of depression on general cognition ratings was greater than fatigue in the non-MCI group. Conversely, the influence of fatigue on emotional and behavioral dyscontrol ratings was greater than anxiety in the MCI group, compared to the non-MCI group. However, factors associated with increased reporting of SCD were not well differentiated across MCI and non-MCI groups overall (i.e., effect sizes did not significantly differ across groups).
Education was significantly associated with SCD-outcomes in the non-MCI group univariate models and remained significantly associated with ratings of general cognition in the multivariable models for the non-MCI group. Within the dominance analyses, education did not exhibit dominance at any level (complete, conditional, or general) over other SCD-associated factors in the MCI or non-MCI groups, and there were no differences in education on SCD-associated outcomes at the general dominance level for either group. Taken together, these results suggests that education may exhibit a unique influence (variance accounted for) on SCD-outcomes within those without a diagnosis of MCI; however, it’s relative importance is lower in relation to other SCD-associated factors, particularly when different combinations of SCD-associated factors are considered.
Treatment and clinical management considerations
Given that the influence of comorbid contributing factors on subjectively rated cognitive difficulties were not well differentiated across those with and without documented cognitive impairment in the current study, self-reported cognitive deficits may be less reliable in detecting objective cognitive changes in the presence of these factors. This finding suggests that a comprehensive assessment involving a clinical interview and objective performanced-based neuropsychological testing is optimal when evaluating individuals who present clinically with one or a combination of these comorbid contributing factors. Additionally, current results indicate a need to screen for the presence of several comorbid contributing variables, such as anxiety and fatigue, when individuals present clinically with SCD, regardless of whether objective impairment is recorded.
The clinical implication of this finding is particularly pertinent when attempting to assess neurobehavioral changes in older former contact sport athletes. Recently developed diagnostic criteria for traumatic encephalopathy syndrome (TES), the purported clinical correlate of chronic traumatic encephalopathy (CTE), requires objective cognitive impairment be confirmed via neuropsychological assessment (Katz et al., Reference Katz, Bernick, Dodick, Mez, Mariani, Adler, Alosco, Balcer, Banks, Barr, Brody, Cantu, Dams-O’Connor, Geda, Jordan, McAllister, Peskind, Petersen, Wethe, Zafonte, Foley, Babcock, Koroshetz, Tripodis, McKee, Shenton, Cummings, Reiman and Stern2021). Results from the current study and the fact that comorbid contributing factors are notably prevelant among former contact sport athletes (Brett et al., Reference Brett, Walton, Kerr, Nelson, Chandran, Defreese, Echemendia, Guskiewicz, Meehan III and McCrea2021; Esopenko et al., Reference Esopenko, Chow, Tartaglia, Bacopulos, Kumar, Binns, Kennedy, Müller and Levine2017; Iverson, Van Patten, et al., Reference Iverson, Van Patten, Terry, Levi and Gardner2021; Kerr et al., Reference Kerr, Marshall, Harding and Guskiewicz2012; Schwenk et al., Reference Schwenk, Gorenflo, Dopp and Hipple2007), reinforces the asseration that comprehensive evaluation involving objective performance-based testing is necessary to assess neurobehavioral changes within the population.
The clinical implications of the current study also underscore the need for multifaceted intervention. Treatment for patients who present to clinic with SCD would likely be most effective if elements to address SCD and comorbid contributing factors were incorporated. For example, results from the current study suggest that cognitive-behavior therapy for improving anxiety symptoms, if present, and concurrent cognitive rehabilitation to assist with compensating for potential cognitive changes would likely be most efficacious in treatment of SCD. The relative rank of comorbid contributing factors identified in the current study suggests a hierarchy for which comorbid contributing factors to prioritize of multiple are present, though each patient should be treated according to their own specific needs.
Limitations
The most notable limitation of the current study involves the self-report nature of medical history, including MCI diagnosis. Although the item regarding the diagnosis of MCI specifically inquired about “physician diagnosed MCI,” inter-individual variability for establishing the diagnosis can occur across providers and the diagnosis was not able to be corroborated with objective neuropsychological assessment within this survey-based study. Additionally, the duration of the diagnosis could not be verified. Given the fact that elevated psychological distress (i.e., depression and anxiety) and SCD in cognitively intact individuals is a notable risk factor for future decline, it is possible that select participants in the non-MCI group may be experiencing objective cognitive impairment and are either in the intermediary stage of conversion to MCI or have not yet been formally tested/diagnosed. Relatedly, given the correlational and cross-sectional nature of the current study, the course of associations observed cannot be established and future longitudinal studies are needed to determine the causal direction of SCD-associated factors and SCD. Additionally, a select “disconnected” subgroup of former NFL players not involved with any of the multiple organizations or agencies through which study solicitation occurred (paper versions sent via traditional mail, email notifications and links to online version) could, in theory, have not been reached. It is important to note, however, that prior a study involving this cohort has shown that the prevalence of MCI within the current sample is greater than the general population overall, and at each decade of life, decreasing the likelihood of bias in which only healthy individuals completed the questionnaire (Walton, Brett, et al., Reference Walton, Brett, Chandran, DeFreese, Mannix, Echemendia, Meehan, McCrea, Guskiewicz and Kerr2021). Finally, the current sample was not statistically powered to effectively examine the influence of race across multiple individual racial identity groups. Given that differences in subjective cognitive ratings were observed across various racial identity groups in the current study and have been reported elsewhere (Ganbat & Wu, Reference Ganbat and Wu2021; Jang et al., Reference Jang, Haley, Choi and Franco2022; Lee et al., Reference Lee, Nam, Yi, Bhimla, Nelson and Ma2021), future work should look to aggregate datasets in order to fully examine the effect of race on SCD ratings within former contact sport athletes, particular in relation to other SCD-associated factors.
Conclusion
The current study indicates that anxiety and fatigue are the most robust factors associated with SCD in former NFL football players when considering various combinations of other SCD-related factors, regardless of the whether documented cognitive impairment is present. Self-reported cognitive deficits may be less reliable in detecting objective cognitive impairment in the presence of these factors and optimal treatment of SCD likely includes a multifaceted approach to directly address cognitive symptoms/weaknesses and indirectly through the influential factors. Further research may seek to identify novel factors that differentiate SCD among those with and without objective impairment and/or investigate the associations of these factors with advanced biomarkers of pathological aging.
Supplementary material
To view supplementary material for this article, please visit https://doi.org/10.1017/S135561772200056X
Acknowledgments
We are grateful for the participation of the athletes, without whom this research would not be possible. The authors would also like to thank Alexa Wild (Department of Neurosurgery at the Medical College of Wisconsin), Candice Goerger (Center for the Study of Retired Athletes at the University of North Carolina at Chapel Hill), Caprice Hunt (Center for the Study of Retired Athletes at the University of North Carolina at Chapel Hill), and Gregory Kobelski (Department of Orthopedics at Boston Children’s Hospital) for study coordination and management; We would also like to thank Daniel Huber for his assistance with data quality and management (Department of Neurosurgery at the Medical College of Wisconsin).
Funding Statement
NFL-LONG was funded by the National Football League (NFL) and this study was supported by the National Institutes of Health National Institute on Aging (BLB- K23AG073528-01).
Conflicts of Interest
Dr. Brett reports grants from the National Institute on Aging and National Institute of Neurological Disorders and Stroke. Dr. Kerr reports grants from National Institutes of Health; grants from Centers for Disease Control and Prevention; and grants from National Football League. Dr. Chandran discloses funding from the National Collegiate Athletic Association and an honorarium received from the Sports Neuropsychological Society. Dr Mannix reports grants from U.S. Department of Defense; grants from NFL Foundation; and grants from National Institute of Neurological Disorders and Stroke. Dr. Echemendia is a paid consultant for the National Hockey League and co-chair of the National Hockey League/National Hockey League Players Association Concussion Subcommittee, Major League Soccer and Princeton University Athletic Medicine, and provides testimony in matters related to mTBI. Dr Guskiewicz reports compensation from National Collegiate Athletic Association for other services and grants from Boston Children’s Hospital (sub-award from the National Football League). Dr. McCrea acknowledges researching funding from the National Institutes of Health, U.S. Department of Defense, Centers for Disease Control and Prevention, National Collegiate Association and National Football League (via subaward from Boston Children’s Hospital). Dr. Meehan receives royalties from ABC-Clio publishing, Springer International, and Wolters Kluwer. His research is funded by philanthropic support from the National Hockey League Alumni Association through the Corey C. Griffin Pro-Am Tournament and a grant from the National Football League.