INTRODUCTION
Researchers conducting in-person and telephone surveys have long found that the ways respondents answer questions can vary depending on the race, gender, or ethnicity of the interviewer (Adida et al., Reference Adida, Ferree, Posner and Robinson2016; Cotter et al., Reference Cotter, Cohen and Coulter1982; Davis, Reference Davis1997; Davis and Silver, Reference Davis and Silver2003; Hatchett and Schuman, Reference Hatchett and Schuman1975; Huddy et al., Reference Huddy, Billig, Bracciodieta, Moynihan and Pugliani1997; Reese et al., Reference Reese, Danielson, Shoemaker, Chang and Hsu1986). This is generally argued to occur for two main reasons. First, the provision of information about the investigator could create demand effects whereby the subjects guess the purpose of the study or the interviewer’s views and change their responses to align with this perceived purpose.Footnote 1 Second, potential subjects may be more or less comfortable answering questions from researchers with a particular identity and subsequently either refuse to participate in studies, decline answering certain questions, or censor the ways in which they answer, all of which could substantively change the results of survey research. Researchers often seek to mitigate these concerns when designing surveys.Footnote 2
In this paper, we build upon prior research and empirically test whether researcher identity affects survey responses in online survey platforms. We do so by varying information about the researcher—conveyed through their name—in both the advertisement for survey participation and the informed consent page. We take this approach for two reasons. First, the inclusion of researcher names at each of these junctures is a common practice. Second, an emerging strain of research throughout the social sciences demonstrates how inferences made from names can affect behavioral outcomes even in the absence of in-person or telephone interactions.Footnote 3 We go on to test how this variation in the researcher name affects the ways in which respondents answer questions in online surveys.
The experiment conducted in this paper contributes to an expanding strain of research exploring the composition and attributes of online survey pools.Footnote 4 Our findings help to interpret the substantive results of prior studies that used online surveys,Footnote 5 and also provide guidelines for researchers as they move forward. In this study, we fail to reject the null hypothesis of no difference in respondents’ behavior when assigned to a putatively black/white or female/male researcher. Our estimates suggest that there could be a substantively small difference between question responses for putatively male and female researchers, but given the high power of the experiment, we are able to bound the substantive size of the effect. We conclude that these differences are likely substantively negligible for most researchers. In general, the results of this paper demonstrate that researchers need not worry that using their own names in either survey advertisements or online consent forms will substantively affect online survey results.
EXPERIMENTAL DESIGN
In the experiment, each respondent was treated with one researcher name intended to cue race and gender, appearing first in the advertisement for the survey and then in the consent form inside the survey. The experiment was conducted on Amazon’s Mechanical Turk (MTurk), where it is common for researchers’ names to appear at both of these points.Footnote 6 To generate the names associated with each of these manipulations, we combined three commonly used lists of racially distinct first and last names.Footnote 7 We crossed the lists of first and last names to produce many possible combinationsFootnote 8 and drew two names for each of the four manipulation categories (black men, black women, white men, and white women). The full list of names used in this experiment is presented in Table 1.
Table 1 Names Used for Each of the Four Investigator Name Manipulations

Investigator name manipulations are based on lists from Bertrand and Mullainathan (Reference Bertrand and Mullainathan2004), Fryer, Jr. and Levitt (Reference Fryer and Levitt2004), and Word et al. (Reference Word, Coleman, Nunziata and Kominski2008).
We then created accounts under the names of our hypothetical researchers (“Ebony Gaines,” “Brett Walsh,” etc.) and recruited subjects through these named accounts. We also included these researcher names on the consent forms for our study. This dual approach is both realistic and methodologically useful. Many Institutional Review Boards require that the researcher include their names on the consent form, and as shown in Table 2, a large number of researchers post studies on platforms such as MTurk under their own names. Given these practices, the substantive nature of treatment is consistent with common practices for researchers using the MTurk survey pool. Moreover, the research design allows us to measure how knowledge about researchers’ identities can shape not only the nature of responses, but the overall response rate.Footnote 9 Posting the survey from named researcher accounts means that potential respondents see the name of the researcher before deciding whether or not to participate, allowing us to capture the selection process that may occur in real studies.
Table 2 The Number of Unique Accounts on MTurk Using Real Names

To calculate these amounts, we searched for the specified term and then scraped all account names on August 15, 2016. Next, we manually classified all unique account names as either a real identifiable name or any other naming scheme (lab name, nonsensical string, etc.).
However, including the treatment in the recruitment process poses design challenges. We could not simply post all treatment conditions simultaneously, because users would then see eight identical surveys posted under eight different researcher names and immediately understand the purpose of the experiment. Instead, we set up the experiment such that any user could only observe one treatment condition by pre-recruiting a pool of respondents.
First, we ran a pre-survey asking only one questionFootnote 10 that captured the unique MTurk “workerID” of each respondent that opted in (N of approximately 5000). Second, we randomly assigned each of these unique identifiers to one of the eight researcher name conditions listed in Table 1. Finally, we created separate MTurk accounts under each researcher name and deployed the same survey within each account. Subjects were assigned a “qualification” within the MTurk interface, according to their assigned condition. Each survey was set such that only MTurk workers with the correct qualification could see the survey (and thus the username associated with it).Footnote 11 This meant that each potential respondent could see only one survey from their assigned researcher, and could then choose whether or not to take that survey. In summary, we posted an initial survey where we collected MTurk IDs, randomly assigned these workers to one of eight conditions where we varied the researcher name, and then only respondents in that condition could view that HIT.Footnote 12
Within the survey, respondents answered a series of questions about social and political attitudes. We drew questions from Pew, Gallup, and the American National Election Survey, specifically asking about issues for which racial and gender cues may prompt different responses.Footnote 13 We chose to ask questions about race and gender, as these are two of the main areas where prior research has demonstrated that interviewer attributes can affect subject behavior. Moreover, this is the information conveyed most prominently by researchers through their names in online surveys. After all subjects had completed all study-related activities, respondents were debriefed about the nature and purpose of the study.
RESULTS
Our design allows us to test whether researcher identity shapes the sample of respondents that agree to take the survey. We find little evidence of such an effect.Footnote 14 We find substantively small differences in the number of people who take the different surveys, and no difference in respondents’ backgrounds on a range of personal characteristics. We also do not find differences in survey completion rates across name; all rates were extremely high (above 97%). Therefore, we are not concerned about inducing selection bias by analyzing the set of completed surveys. We turn next to the content of survey responses.
Our analyses fail to reject the null hypothesis that there is no difference in how respondents answer questions when assigned to a putatively black or female researcher relative to a white or male one. We estimate all of our treatment effects using linear regression models, regressing outcome on the indicator of treatment. Robust standard errors are estimated using a bootstrapping procedure. Following our pre-analysis plan, our rejection levels for accepting that the effects differ from zero are calibrated to yield an expected number of false discoveries of α = 0.05, adjusting for multiple testing using the Benjamini–Hochberg procedure (Benjamini and Hochberg, Reference Benjamini and Hochberg1995).Footnote 15 This adjustment is important since we dramatically increase the chances of a false positive finding by testing for multiple outcomes (Benjamini and Hochberg, Reference Benjamini and Hochberg1995).Footnote 16 To avoid the appearance of “fishing” for significant p-values across many outcomes, we cannot simply follow a rule of rejecting any null hypothesis when p < 0.05. We focus on estimating only the average treatment effects of the researcher race and gender treatments, and, consistent with our pre-analysis plan, only investigate possible treatment effect heterogeneity as exploratory rather than confirmatory results.Footnote 17
Our first set of outcome questions examines whether assignment to a putatively female/black (relative to male/white) investigator changes reported affect towards, or support for policies meant to help, women/blacks.Footnote 18 For the race dimension of treatment, we estimate treatment effects on three distinct outcomes: expressed racial resentment (as measured by the 0–1 scale developed by Kinder and Sanders, Reference Kinder and Sanders1996), willingness to vote for a black president, and support for social service spending. On gender, we examine respondents’ beliefs regarding the role of women in society, willingness to vote for a woman presidential candidate, and the same social service spending outcome. In selecting our first two outcome questions, we sought questions that were both commonly used in online surveys but also directly related to each of our treatments. The social spending measure was included as a facially non-racial measure that could still have racial or gendered overtones. This allowed us to test whether respondents would think of social spending as disproportionately benefiting minorities and women, and so potentially answer in either raced or gendered ways depending on the putative race or gender of the researcher.
We designed our experiment to target a sample size of 2000 total respondents.Footnote 19 For the race treatment, we find no evidence that black versus white researcher names yield different responses on the outcome questions. Figure 1 plots the expected difference in outcomes for each of these three questions for respondents assigned to a hypothetical black researcher name relative to respondents assigned to a hypothetical white researcher name. For all three outcomes, the difference in outcomes between the two treatment groups is not statistically significant at α = 0.05. We fail to reject the null of no effect for all outcomes at the α = 0.05 level.
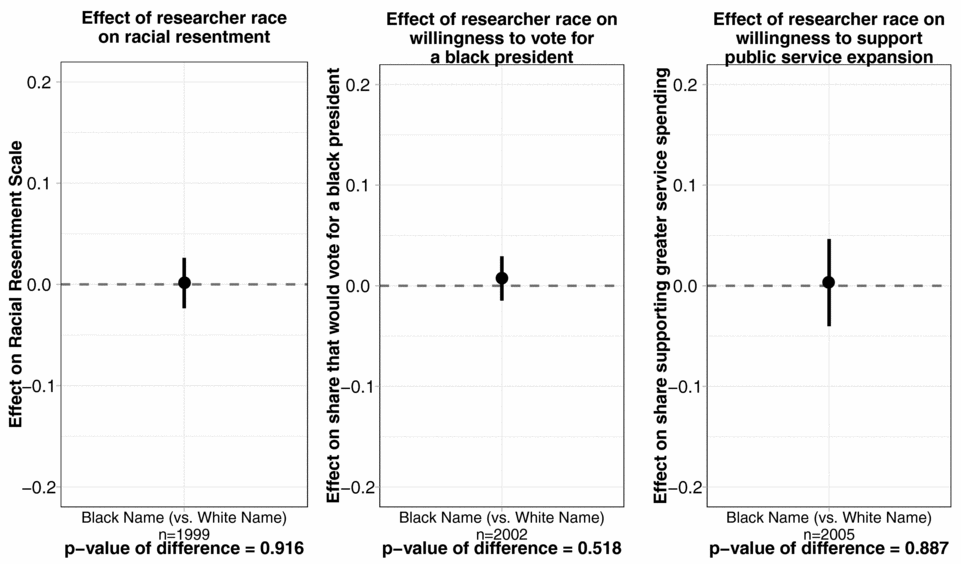
Figure 1 Difference in Policy/Attitude Outcomes for Researcher Race Treatment
For the gender treatment, when we adjust for multiple comparisons we fail to reject the null hypothesis that there is no difference between putatively male or female researchers. Figure 2 plots the difference in expected values for each of the the outcomes between the female researcher and male researcher treatment conditions. While we fail to reject the null, we should note that for all outcomes, respondents under the female researcher treatment condition were about 2–4 percentage points more likely to express affective/policy support for women. The individual p-value for the null of no effect on the gender equality outcome question fell just below the commonly used threshold of 0.05. The p-values for the null for the other two outcomes, however, fall just above the typically used threshold. Under our pre-registered design, using the Benjamini–Hochberg correction for multiple testing, we fail to reject the null for all three outcomes.Footnote 20 We cannot conclude that assignment to a putatively female researcher name significantly increased the likelihood that respondents would exhibit more woman-friendly attitudes on gender-related questions.

Figure 2 Difference in Policy/Attitude Outcomes for Researcher Gender Treatment
Despite our failure to reject the null, we note that the point estimates for the direction of the effect are consistent with our original hypothesis. In general, respondents assigned to a putatively female investigator were, in-sample, more likely to express beliefs that were more supportive of women’s equality. Given this, how concerned should researchers be about these estimates? Power calculations for our design suggest a relatively small upper bound for any “true” effect. For a study of our sample size, and accounting for the multiple comparison adjustment, we conclude that it is unlikely that we would have failed to reject had the true effect of any one of these outcomes been greater than 5 percentage points.Footnote 21 While it is not possible to “affirm” a null hypothesis, the high power of our study is such that our null finding implies any real effect is likely to be bounded close to 0.
DISCUSSION AND CONCLUSION
In this paper, we demonstrate that researchers using online survey platforms such as Amazon’s Mechanical Turk generally need not be concerned that information conveyed through their name in either the advertisement for the HIT or the informed consent page will subsequently affect their results. Our study is designed to address both elements of investigator bias: inferences about the purposes of the study and comfort with the investigator, either of which we might expect to affect the willingness of respondents to take the survey in the first place, overall effort, and the types of answers given. We fail to reject the null hypothesis that researchers’ race or gender (cued through names) have no effect on respondents’ survey behaviors. While our evidence suggests that there might be a small “true effect” of researcher gender, our power calculations demonstrate that this effect, if any, is quite small and likely not substantively meaningful for most researchers.
There are at least two plausible explanations for why the results of this paper diverge from the substantively meaningful effects found in research on other survey platforms. First, it could be the case that either the strength or substance of the treatment differs between online survey platforms and other modes of conducting surveys (such as in-person or telephone). That is, interacting in person with a black/white or male/female researcher might have a stronger effect on respondent behavior than simply reading their names. Substantively, this means that even if respondents do notice the putatively black/white or male/female name assigned to the researcher through treatment, the act of reading this name is simply not enough to change their subsequent survey responses.
Second, it could be the case that respondents in online survey platforms are less likely to take treatment.Footnote 22 If this were the case, it could be in part driven by the fact that our respondents were recruited via Amazon’s Mechanical Turk, where the financial incentives for respondents to complete tasks as quickly as possible might lead them to quickly skim through the consent page.Footnote 23 This means they would be less likely to notice the researcher name and thus less likely to respond to it. Even if respondents were prone to bias, it could be masked by the fact that few respondents actually read the names in the first place. The present study is unable to adjudicate between these two potential explanations.
For researchers conducting studies on MTurk and similar online platforms, this distinction will not matter. Nevertheless, the two different mechanisms have important implications both for the external validity of the present study as well as further research on the attributes of online survey pools. In particular, researchers should be cautious in applying the results of this study when either (1) they provide more information about themselves than simply their name in the advertisement for the survey and on the informed consent page (that is, they have a stronger treatment), or (2) respondents in their sample pay more attention throughout all stages of the survey than MTurk respondents (i.e., there is higher treatment uptake). In our experience, the first point is unlikely to occur across different survey platforms since a few platforms provide more researcher information than MTurk. However, whether and how much attention varies across different survey platforms and how this substantively affects results is an open question and interesting area of further research.
SUPPLEMENTARY MATERIALS
To view supplementary material for this article, please visit https://doi.org/10.1017/XPS.2017.25