A fundamental tension continues to exist between the need for quality benefit-cost analysis (BCA) in evaluations of social policy interventions for children, and the difficulty in doing so. In their paper from half a decade ago, Vining and Weimer summarized the challenges of carrying out BCA of social policy interventions as well as the primary research needs that, if accomplished, would facilitate efforts in the future (Vining & Weimer, Reference Vining and Weimer2010). Their review emphasized both needs to improve upon current shadow prices as well as where new ones should be developed. Comprehensive BCA is particularly difficult for programs directed toward children, especially where targeted skills and behaviors are not directly monetized and where subjects are not followed for a long enough time to allow measurement of adult outcomes (Foster, Dodge & Jones, Reference Foster, Dodge and Jones2003; Crowley, Hill, Kuklinski & Jones, Reference Crowley, Hill, Kuklinski and Jones2013). Research will be vital for informing development of the necessary shadow prices that should be based on causal associations, involve multiple studies, and recognize uncertainty in any cost projections. Such requirements make it so that determining shadow prices in a valid and reliable manner will be a gradual and challenging process. Developing such estimates is worth pursuing, given the importance and relevance of BCA for effective programs for children.
In simplest terms, the existing literature has considered the skills and behaviors targeted through social policy intervention for children to fall under the dichotomy of “cognitive” versus “noncognitive,” especially for programs delivered within school settings where both areas may be addressed. A rich literature discusses the frameworks from which we can consider different aspects of human development and personality in terms of these two umbrella terms, albeit often in terms of certain outcome categories (Almlund, Duckworth, Heckman & Kautz, Reference Almlund, Duckworth, Heckman, Kautz, Hanushek, Machin and Woessmann2011; Committee on Defining Deeper Learning and 21st Century Skills, Reference Pellegrino and Hilton2012; Farrington et al., Reference Farrington, Roderick, Allensworth, Nagaoka, Keyes, Johnson and Beechum2012; Lippman, Ryberg, Carney & Moore, Reference Lippman, Ryberg, Carney and Moore2015). Shadow prices for cognitive skills have been employed in BCA of programs for children and youth. This is especially the case if we consider educational attainment as a manifestation of cognitive ability. For instance, the shadow price of a high school diploma can be used where an effective program may decrease school drop-out rates.
Research entities, such as the Washington State Institute for Public Policy (WSIPP), have taken the next step to determine costs associated with proximal outcomes linked to high school graduation, namely achievement scores (Lee et al., Reference Lee, Aos, Drake, Pennucci, Miller and Anderson2012). Others have developed shadow prices for intelligence quotient (IQ) points (Vining & Weimer, Reference Vining and Weimer2009). Shadow prices for early achievement recognize the value of stages of cognitive development that are vital in young students. In contrast, BCA researchers have rarely taken the opportunity to monetize noncognitive skills (e.g., personal competencies and characteristics including mental health/emotion management, social skills, self-discipline, motivation, personality characteristics, and dozens of other “soft skills” identified in research). This is unfortunate given the wealth of evidence that shows that noncognitive skills have great influence on economic outcomes related to crime, attainment, substance abuse, labor market success, and health (among other things). It is also unfortunate given that many social policy intervention efforts target noncognitive skills in children. Further, in the context of growing evidence-based policy initiatives, the field increasingly prioritizes public investment toward cognitive capacities (Levin & Belfield, Reference Levin and Belfield2014).
In this paper, we review the state of the inclusion of noncognitive skills in economic evaluation of programs for children and youth. This is not a review of BCA and use of shadow prices in social policy in general (see Vining and Weimer (Reference Vining and Weimer2009) and Weimer and Vining (Reference Weimer and Vining2009)). Despite well-intentioned dissuasion (e.g., Calkins, Reference Calkins2015; Vander Ark, Reference Vander Ark2012), we employ the term noncognitive here, given its common use across research disciplines for covering many important competencies in human development (other terminology has been proposed including soft skills (Lippman et al., Reference Lippman, Ryberg, Carney and Moore2015), social–emotional skills (Miyamoto, Huerta & Kubacka, Reference Miyamoto, Huerta and Kubacka2015), and character skills (Heckman, Humphries & Kautz, Reference Heckman, Humphries and Kautz2014)). We first consider the broader definitional issues of noncognitive skills in research and for the sake of BCA in social policy. In the second section, we focus on measurement issues that are vital to how noncognitive skills and behaviors can be incorporated into economic evaluation. Finally, we consider valuation issues for representing noncognitive ability in BCA, once defined and measured. In this last section, we review the current state of inclusion of noncognitive skills in economic evaluation of programs for children. We dually consider what skills have been represented through shadow prices as well as what noncognitive skills are targeted in programs for children, enabling us to summarize what has been left out so far. We also consider what noncognitive skills have the most potential for inclusion in future benefit-cost models. Finally, we review the research and organized efforts that could help facilitate further development of shadow prices, based on variable linkages or contingent valuation.
Defining noncognitive skills: embracing the complexity
Various intra- and interpersonal competencies in human development related to personality, emotions, and social skills are often represented as “noncognitive” ability. These are skills that are distinct from those thought of as more cognitive in nature that encompass innate intelligence, learned academic ability, critical thinking, and creativity (Committee on Defining Deeper Learning and 21st Century Skills, Reference Pellegrino and Hilton2012). For the sake of BCA, noncognitive skills deserve attention, given they are potentially improved by an effective program and are often targeted in social policy interventions. The “cognitive” versus “noncognitive” terminology is well established but misinforming, as is often expounded in other reviews (Duckworth & Yeager, Reference Duckworth and Yeager2015). A primary misrepresentation with the label “noncognitive” is that these skills involve cognitive processes throughout. For instance, cognitive functioning is central to the ability to manage one’s state and resolve problems. Additionally, the two cannot be thought of as independent entities. In a school setting the capacity to complete work and achieve relies on cognitive ability but also requires self-discipline and motivation. Of course, strengthening cognitive skills has been emphasized in school systems, given long-held beliefs in the role of learned knowledge toward attaining educational degrees and subsequent labor market success. Traditionally, in education less importance has been directed toward complementary noncognitive skills that cover other aspects of individuals’ daily lives including self-control, social competence, emotion management, and attitudes. Many interventionists consider this a reason to boost noncognitive ability through extra programming efforts within or outside of school (Durlak, Weissberg, Dymnicki, Taylor & Schellinger, Reference Durlak, Weissberg, Dymnicki, Taylor and Schellinger2011; Greenberg et al., Reference Greenberg, Weissberg, O’Brien, Zins, Fredericks, Resnik and Elias2003).
Frameworks from multiple research disciplines
Much research attention is directed toward the importance of noncognitive skills in human development. This includes their role in increasing the likelihood for educational attainment, increased productivity in the labor market, decreased likelihood for criminal activity, and better health/mental health (Heckman, Reference Heckman2000; Carneiro, Crawford & Goodman, Reference Carneiro, Crawford and Goodman2007; Brunello & Schlotter, Reference Brunello and Schlotter2011; Tough, Reference Tough2013). Importantly, research highlights the value of addressing noncognitive ability early, given the crucial role early skills play in developmental cascades for success in school and healthy state management (Masten et al., Reference Masten, Roisman, Long, Burt, Obradovic, Riley, Boelcke-Stennes and Tellegen2005). This research supports the value of targeting noncognitive skills at a young age, thereby setting the stage for long-term well-being and increased economic success. Of course, the role of noncognitive ability in child development is complicated, given the joint influence with cognitive ability, physiology, parental investment, and other contextual factors all shaped over time (Cunha & Heckman, Reference Cunha and Heckman2008). Collectively, these factors influence how one functions and makes decisions across the behavioral spectrum (e.g., ability to be productive in school or on the job, deciding not to be delinquent). Defining noncognitive ability for BCA must acknowledge the complicated interplay of several factors in development.
Characterization of noncognitive skills in studies differs across research disciplines. In education, noncognitive skills are considered for their impact on achievement in school (Farrington et al., Reference Farrington, Roderick, Allensworth, Nagaoka, Keyes, Johnson and Beechum2012). Psychology studies focus on the role of characteristics such as executive function and self-regulation in childhood. The study of noncognitive skills in economics is often in relation to the impact on labor market outcomes or productivity in general. A well-known structure for representing noncognitive skills has been the “Big Five” taxonomy in personality psychology, providing classification into a handful of domains – agreeableness, extraversion, openness to experience, conscientiousness, and neuroticism – that envelope the vast list of more specific skills. Other characterizations have been proposed to represent personality, often extending the breakdown beyond five categories (for an overview, see Almlund et al. (Reference Almlund, Duckworth, Heckman, Kautz, Hanushek, Machin and Woessmann2011)). Important frameworks have also been proposed for considering fundamental categories of social and emotional learning (SEL) (Collaborative for Academic Social and Emotional Learning, 2015), intra- and interpersonal competencies (Committee on Defining Deeper Learning and 21st Century Skills, Reference Pellegrino and Hilton2012), and for skills relevant to future workforce success (Lippman et al., Reference Lippman, Ryberg, Carney and Moore2015).
The variation in frameworks across disciplines is reflected in social policy through the nature of what programs are aiming to change, for example, enhancing skills to improve future success versus building skills to reduce the likelihood for problems. Classifications of noncognitive ability from an educational or workforce perspective place emphasis on how skills can increase success (Farrington et al., Reference Farrington, Roderick, Allensworth, Nagaoka, Keyes, Johnson and Beechum2012; Lippman et al., Reference Lippman, Ryberg, Carney and Moore2015). Other classifications may be more general toward well-being, with attention toward skills that improve personal functioning. The Collaborative for Academic, Social and Emotional Learning (Collaborative for Academic Social and Emotional Learning, 2015), for instance, classifies SEL skills into five categories that emphasize skills to increase success as well as to respect others and avoid problems (self-awareness, self-management, social awareness, relationship skills, responsible decision making). The Big Five personality characteristics include the negatively valenced neuroticism, recognizing the value of healthy mental states. Clearly programs that can increase the likelihood of success (e.g., future educational attainment, employment) and programs that can reduce the likelihood for future problems (e.g., preventing crime or substance abuse outcomes) are both relevant for BCA.
Definitional needs for BCA
The frameworks proposed in research are useful for helping organize and consider important human skills and traits (for a thorough review of the many frameworks proposed, see (Lippman et al., Reference Lippman, Ryberg, Carney and Moore2015)). Yet BCA requires more specificity for the underlying construct and how it is measured. A clearer delineation of noncognitive skills that balances theoretical and practical matters can help researchers understand what can be incorporated into an economic analysis. This can start through observation of what skills and behaviors are targeted through social policy intervention, and how they are measured. As we emphasize below, some skills are manifested through observable behaviors, while others are more latent and may require assessments to gauge them. Valuation of assessed skills in programs involving younger children is difficult, and program evaluation usually does not follow program participants long enough to observe future monetized behaviors that result. If it is important to reach children early in the developmental period, then it is critical to understand how these early states impact future well-being reflected in late adolescent or adult outcomes. In that sense, we can contemplate noncognitive competency or ability that one has versus a state or condition or behavior (outcome) that eventually occurs. Benefit-cost analysis is more likely to involve values determined for the latter, whereas programs for children, especially preventive or skill-building interventions, are aimed at the former.
Attention should also be directed toward distributional characteristics of the specific skills or behaviors in question, especially as they are related to certain distal outcomes that could be monetized. This may be important given that certain programs may intend to induce change categorically (e.g., from an at-risk state to not at-risk state) while others aim to induce more continuous change (increasing the level of some competency in general). For BCA we may make the assumption that an improvement of a skill (for instance) is linearly related to an outcome, such as an increased likelihood to graduate from college. The linkage may not always be that simple, however, especially considering programs trying to prevent afflictions, such as substance dependence or a mental health condition. For a certain skill, change at one end of the distribution may mean something different than an equivalent change in the middle of the distribution (akin to how change in a measure of health such as cholesterol means something different at different parts of the spectrum). Relatedly, it is important to understand how noncognitive ability interacts with other key factors (e.g., physiological, cognitive, other noncognitive skills) in influencing an outcome. For instance, self-regulation could include developing state management skills toward knowing when to seek professional help for a mental health condition that is innate and/or driven by environmental stressors. More generally, research may demonstrate that noncognitive ability has different implications among different subgroups. Where possible, definitions of noncognitive ability for BCA should recognize relevant contextual issues that can make a big difference in how shadow prices are formed.
Considering a conceptual structure for BCA
There are various frameworks and the number of noncognitive skills featured in research within these classifications is extensive. The information from various studies and reviews is useful but it is challenging to assimilate. However, to further the discussion for the sake of BCA, we adopt a list defined by Lippman and colleagues, given its breadth considering various research disciplines as well as being up to date (Lippman et al., Reference Lippman, Ryberg, Carney and Moore2015). While the report emphasizes five critical “soft skills” when considering labor market outcomes (social skills, communication, higher order thinking, self-control, positive self-concept), we include their broader defined set of skills in Table 1. In parentheses in the table we list related terms from other disciplines; this includes the Big Five categories as well as executive function, a broader term for skills related to attention, working memory, and inhibitory control (Anderson, Reference Anderson2002). Further breakdown into interpersonal versus intrapersonal skills as proposed through other frameworks (Committee on Defining Deeper Learning and 21st Century Skills, Reference Pellegrino and Hilton2012) is useful both for considering the nature of these skills and for how they can be measured. For definitional purposes, the conceptualization of interpersonal competencies may be simpler than for intrapersonal competencies. This is reflected in current proposed frameworks for noncognitive skills where the majority of subcompetencies are intrapersonal (e.g., Collaborative for Academic Social and Emotional Learning (2015), Miyamoto et al. (Reference Miyamoto, Huerta and Kubacka2015), Committee on Defining Deeper Learning and 21st Century Skills (Reference Pellegrino and Hilton2012), Hess (Reference Hess2015), Farrington et al. (Reference Farrington, Roderick, Allensworth, Nagaoka, Keyes, Johnson and Beechum2012)); this includes
$3/5$
of the Big Five and
$4/5$
of the noncognitive factors defined for shaping academic performance (Farrington et al., Reference Farrington, Roderick, Allensworth, Nagaoka, Keyes, Johnson and Beechum2012). Interpersonal and intrapersonal characteristics are intertwined; the ability to interact with others is greatly influenced by how one manages internal emotional states, and vice versa. The distinction is useful conceptually, however, to consider what skills are targeted by interventions, how they are measured, and their potential role in impacting future costs.
Table 1 Noncognitive categories for skills targeted by social policy interventions, classified by intrapersonal versus interpersonal.

Note: Skills defined through framework proposed in Lippman et al. (Reference Lippman, Ryberg, Carney and Moore2015).
Noncognitive skills targeted by intervention
The competencies shown in Table 1 are relevant to BCA given that many social policy interventions aim to improve them, and it is plausible that these skills can impact current and future costs. It is not hard to conceive how interpersonal characteristics are linked to economic outcomes. Improved ability to work with others translates into success in school and the workplace. Better relationships with others positively impacts mental health and in turn, functioning in daily activities. More broadly, those who gain respect for others and for societal customs are less likely to commit interpersonal crime. Programs that target interpersonal skills may aim to improve the ability to work with others to complete tasks (e.g., in school settings) or to develop positive supportive relationships. In addition, evidence exists that these skills can be identified at an early age. For example, prosocial behavior in young children has been shown to be linked to future outcomes across multiple domains of adult functioning (Jones, Greenberg & Crowley, Reference Jones, Greenberg and Crowley2015). Intrapersonal competencies that are targeted by interventions include state management (and other aspects of mental health), substance abuse resistance, learning strategies, and motivational tools. It may not be hard to draw the link between intrapersonal ability and monetized outcomes. For instance, attention skills can be improved and have been shown to be uniquely important to success in school (Duncan et al., Reference Duncan, Dowsett, Claessens, Magnuson, Huston, Klebanov, Pagani, Feinstein, Engel and Brooks-Gunn2007). Several studies have demonstrated the role of positive self-esteem in school and labor market success (Drago, Reference Drago2011; Murnane, Willett, Braatz & Duhaldeborde, Reference Murnane, Willett, Braatz and Duhaldeborde2001). Self-control has been linked to several long-term outcomes including avoidance of crime in adulthood (Pratt & Cullen, Reference Pratt and Cullen2000; Moffitt et al., Reference Moffitt, Arseneault, Belsky, Dickson, Hancox, Harrington, Houts, Poulton, Roberts and Ross2011). Much study is directed toward the ability of individuals to achieve or thrive when faced with challenges or contextual risk factors (i.e., overcome obstacles in order to be productive). This characteristic may appear in research as perseverance, grit, resiliency, or motivation (Duckworth, Peterson, Matthews & Kelly, Reference Duckworth, Peterson, Matthews and Kelly2007). For considering BCA of programs directed at children, it is important to recognize both those competencies that are targeted through effective programs as well as the evidence for how these targeted skills matter in development.
In general, the most successful programs can have a lasting impact on individuals’ productivity at school and the workplace, improve personal relationships, and increase resiliency to environmental stressors. Programs that are shown to be effective at improving noncognitive skills in children are listed through resources such as the Blueprints for Healthy Youth Development (Institute of Behavioral Science, 2015) or the Top Tier Evidence initiative (Coalition for Evidence-Based Policy, 2015). Although many effective programs are identified as effective at improving the skills listed in Table 1, it is also clear that these skills are not readily monetized. Conventional BCA of programs for children would rely on valuing the indirect influence of these skills, enabled through tracking individuals long enough for measuring the economic outcomes that are eventually realized (i.e., cost savings or costs generated). A better understanding of the role of these proximal skills in influencing future costs would greatly improve the use of BCA of programs for children. Moreover, a clearer mapping of the various skills can help us understand where links have been determined for the sake of BCA and where more research could be helpful (considered further below, related to valuation).
Issues in measurement: quantifying noncognitive skills for BCA
There is growing cross-disciplinary agreement that noncognitive skills matter in shaping future adult outcomes. Much depends, however, on our capacity to measure these skills in evaluations of programs for children. Certain skills may manifest through eventual behaviors or states that involve costs (e.g., a diagnosis or criminal act), and are useful measures for BCA. Certain skills in Table 1 may best be considered as proximal to a future outcome that can be monetized (e.g., improved academic work habits increase the likelihood to graduate from high school on time). These are vital to consider given they may be targeted outcomes in social policy intervention and may be malleable through effective programs. Measurement of both proximal states (e.g., substance use attitudes) and hypothesized relevant outcomes (e.g., substance use) is common in evaluation of program effectiveness. These same issues of measurement are central to determining how best to capture noncognitive skills for BCA.
Following Table 1, we introduce Table 2 adding a separate distinction in terms of measurement: observed versus assessed. This includes skills from Table 1 but also common behaviors/outcomes related to noncognitive ability (based on a survey of prior BCAs in social policy for child and youth programs). The former are usually assessed in studies, while the latter are more often observed. We note that this is not a distinction based on measurement sources. That is, assessed skills may be more likely to be self-reported but could feasibly rely on another informant or observer (e.g., teacher, parent). Observed competencies are apparent through behaviors or actions that would be apparent to others (thus suitable for observer report or records) but could also be self-reported (or maybe parent-reported if the subject is a child).
Table 2 Examples of noncognitive skills and related outcomes based on their nature (interpersonal vs. intrapersonal) and measurement type (assessed vs. observed).

Note: Italicized
$=$
not monetized in BCA.
It is important to make such distinctions in order to consider measures that are useful to BCA but also for capturing noncognitive ability in children. Observed skills are simpler to conceive in terms of scale although not free of measurement error given that they might be based on recall (by self or another informant). Observed measures related to noncognitive skills are often counts, such as the number of visits to an emergency department or the number of academic milestones reached. Here we also include clinical determinants (conditions, diagnoses) that are well established. A diagnosis for conduct disorder, for instance, would be an observed outcome given that it is an observed condition, albeit determined by another person (mental health professional). Skills that are assessed could be gathered in research or clinical settings, much in the way cognitive ability is assessed in school. Assessed skills may include artificially generated behavior, or an indication of how one would respond, given situational specifics (McDaniel, Morgeson, Finnegan, Campion & Braverman, Reference McDaniel, Morgeson, Finnegan, Campion and Braverman2001). For assessments there are usually many instruments to choose from dependent on setting and research intentions. This is understandable but creates difficulty in getting consistent results that would be useful for BCA. Measurement theory is central here, as the goal is to tap something latent that cannot be perfectly measured but is ideally approximated.
The utility of observed measured behaviors for BCA is clear, if they are measurable in the timeframe of the study. Cost of illness research and studies of public costs of crime and delinquency enable shadow prices for mental health problems and extreme behavior problems that may be partially a result of noncognitive deficiencies (e.g., state management). Completion of tasks such as obtaining a degree reflects noncognitive competence and is more directly valued. Such economically relevant observed measures could be obtained for older children, especially within an evaluation of a program directed toward adolescents that is thus better suited for BCA. For illustration we italicize skills in Table 2 that are not found in BCAs currently. This demonstrates how measured observed behaviors or states – to the extent that they represent the manifestation of noncognitive skills – are already incorporated into the BCA of programs for children.
Table 2 also maintains the breakdown between inter- and intrapersonal noncognitive skills, given the implications for the sake of measurement. (As discussed above, the distinction is not perfect; certain skills/behaviors involve both inter- and intrapersonal processes to some degree, such as those resulting in observed crime/delinquency outcomes; the classification is based on what is more salient.) The lengthy list in the bottom left quadrant (intrapersonal, assessed) may be important in human development, but is not easily valued. The potential greater impact from reaching children early, however, could increase the likelihood for long-term well-being (Conti & Heckman, Reference Conti and Heckman2013; Cunha, Heckman & Schennach, Reference Cunha, Heckman and Schennach2010; Barnett & Frede, Reference Barnett and Frede2010). Observational measurement may rely on situations that may not have transpired yet for an individual. And important early noncognitive skills (e.g., early self-regulation) may be better captured through reliable established measurement instruments than through measured behaviors. An advantage of assessed over observed measurement is that responses can be obtained based on one’s capacity to produce an outcome, and thus is not dependent on outcomes observed or reported to have occurred naturally.
Measurement of interpersonal skills
Observed interpersonal skills are relevant to economic outcomes, and can represent different aspects of well-being and functioning. For instance, observed interpersonal competence may be represented through relationships with intimate partners or family. A healthy long-term relationship could have additional mental and emotional benefits that increase productivity and help decrease other costly behavioral or health problems. Many preventive interventions aim to reduce risky sexual behavior in adolescents, to deter sexual disease or unwanted pregnancy. Interpersonal skills in school or the workplace may also be relevant. Perhaps a new frontier for observation of interpersonal skills involves the social influence of an individual. Relatively simple measures can capture this, such as peer nominations of social friendships. For youth programs this could involve either positive or negative influence. Economic benefits could be linked to one’s ability to improve productivity among a group or otherwise related to that individual’s influence that may translate into greater success in the labor market. Negative influence could be characterized, for instance, if one coerces peers toward antisocial acts such as substance abuse, delinquency, or gang involvement. Social network analysis techniques (Scott, Reference Scott2012) can derive statistical estimates of the influence of individuals, which could feasibly be useful for BCA.
Assessments of interpersonal skills in evaluations of youth programs can also provide important information on general social skills, relationship skills, and other attitudes or beliefs, albeit in a less natural manner. Externalizing and aggressive behavior can be assessed through composite measures that can be obtained relatively easily. For instance, a well-known measure such as the Child Behavior Checklist can provide aggression scores on a continuum that is useful in some studies where a yes–no diagnosis is less preferred or impractical (Achenbach, Reference Achenbach1991). Assessment can also involve seeking answers from children for how they would handle certain social situations or interactions with peers or family. This enables data for aspects of interpersonal skills that may not be observable, given situational needs. Characterizing interpersonal skills from assessment may be challenging, but the most promise for BCA would come from latent skills validated to be causally linked to monetized outcomes. In this case, combination of multiple assessments (through measurement models) could be useful for representing interpersonal noncognitive states.
Of course, measurement of interpersonal skills in a single snapshot provides limited information. For instance, while strong supportive interpersonal relationships can improve an individual’s mental health and influence the ability to succeed in school or work, it may be hard to capture the degree or permanence of this interpersonal bond. Plus, it may depend on support systems that are hard to characterize simply. Data from multiple sources or across multiple occasions could help with better characterization of interpersonal noncognitive functioning. Newer data collection methods (such as through smartphones) could also provide better information on interpersonal skills across varying settings and time periods. Data on neurobiological mechanisms are also becoming more common, and could provide more information on interpersonal noncognitive skills, which could be especially useful for abstract processes where the informant report is unreliable. (We note that our discussion of innovative data collection methods pertain to intrapersonal skills as well.) Such approaches could provide richness to investigations of causal influence on economic outcomes, especially related to teamwork and productivity at the workplace.
Measurement of intrapersonal skills
Intrapersonal skills manifest through observed behaviors in many ways that reflect personal functioning. A primary example of the manifestation of intrapersonal skill is measured in schools, especially related to academic behavior (Farrington et al., Reference Farrington, Roderick, Allensworth, Nagaoka, Keyes, Johnson and Beechum2012). This could include attainment, given the importance of noncognitive ability for working toward completing a degree and other educational milestones (Heckman & Rubinstein, Reference Heckman and Rubinstein2001), but also task completion and attendance. Poor academic behavior may be measured through required extra resources in school for more extreme issues, such as repeating grades or requiring special education services. In general, observable school outcomes such as these – positive and negative – provide good representation of noncognitive ability to complete educational requirements. Intrapersonal noncognitive factors related to antisocial behavior would involve behavior that is not directed toward other persons. More severe cases of antisocial behavior that likely include propensity to be violent may require more intensive services to prevent long-term problems (although the pay-off for averting a violent offender is very large in terms of both personal and societal costs). It may also be the case that interventions can better address some level of antisocial behavior but not ameliorate more extreme conditions. If we are able to distinguish delinquency that is not interpersonal, valuation for the sake of BCA can occur directly through direct costs of criminal acts, including property loss, criminal justice system costs (lower than for violent offenders), and costs to victims from damage or loss of property. Much can be gained for BCA from understanding what noncognitive processes influence various levels of antisocial behavior (intra- versus interpersonal).
Substance abuse represents another manifestation of intrapersonal noncognitive competence. Many social policy interventions and school programs aim to bolster skills (i.e., related to state management and self-control) that are influential to preventing substance abuse. Substance abuse behavior can be measured in multiple ways, including assessment of the age of first initiation, counts of recent use, whether an extreme more illicit drug had been tried, and whether risky associated behaviors (e.g., driving while intoxicated) occurred. Intrapersonal problems related to limited self-control or poor mental health can manifest in substance abuse at an early age.
Many intrapersonal skills do not manifest in more natural observed outcomes within the timeframe of the evaluation, and thus assessments are required to measure the effectiveness of programs for children. The measures used depend on what skills or behaviors are targeted by programs. A variety of established and validated measures are available to assess intrapersonal skills shown in Table 2. However, there are challenges for BCA given the lack of evidence for these skills to be linked to costs (reflected in the number of italicized skills in the bottom left quadrant of Table 2). Currently, assessed intrapersonal skills that are most useful to BCA are those that are parallel constructs to current or future behaviors that are more readily monetized. For instance, this could include a measure of emotion management or internalizing problems that can be linked to a future mental health condition (as with the Child Behavior Checklist referenced above). Other assessments are relevant to BCA if prior studies indicate causal links with success in school or the labor market, or as realized through avoidance of personal problems such as substance abuse. Typically, assessed intrapersonal competencies are not planned for use in eventual BCA, although such measures have been used retrospectively in BCA (Aos et al., Reference Aos, Lee, Drake, Pennucci, Klima, Miller, Anderson, Mayfield and Burley2011).
A specific concern with measurement of noncognitive ability is how to sort through the number of measures available to assess it. Given the complexity of noncognitive skills (evident in the various ways it is classified, as discussed above), there is an assortment of possible measures for various skills, and different studies rely on different instruments. Benefit-cost analysis is facilitated if there is consensus for what measure best captures a particular skill. If evidence for indirect links with costs is based on different measures than those available in a specific study, generalization concerns can arise. Analysts must consider whether certain measures are better suited for use in BCA. Part of this may rely on what measures are shown to be (a) most valid for representing the construct and (b) provide the best evidence for links with monetized outcomes. If BCA is part of the plan in evaluation, the researcher could consider what options exist to also obtain observed intrapersonal measures that are part of the logic model. Where evidence emerges for causal links between the measured skills shown in Table 2 and current or future costs, the options will increase for shadow prices that are useful in the evaluation of programs for children.
Monetizing noncognitive skills
Currently, noncognitive skills are represented in BCA implicitly through monetized outcomes that they influence. To a limited degree, certain skills and behaviors in Tables 1 and 2 have been valued based on determined linkages with other monetized outcomes (i.e., indirectly). But generally, shadow prices for these skills are very rarely attempted; thus BCA of programs for children usually relies on whatever monetized outcomes are available. Other targeted outcomes are left unvalued, providing an incomplete picture of the potential economic benefit if such outcomes are truly causally linked to future costs. We begin our discussion on valuation issues by first summarizing where noncognitive factors have been incorporated into BCA either through outcomes they influence or through indirect valuation, that is, where causal linkages are evident. Then we consider where more research will be needed to further the possibility of shadow price development. To review the state of valuation of noncognitive skills, we present Table 3 showing the tiers of valuation for noncognitive competencies in BCA currently. We define three levels to summarize evidence from prior published research.
Table 3 State of shadow price and valuation evidence for noncognitive behaviors and skills.
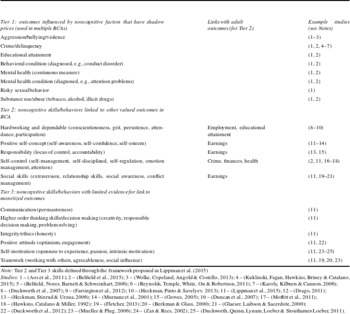
Note: Tier 2 and Tier 3 skills defined through the framework proposed in Lippman et al. (Reference Lippman, Ryberg, Carney and Moore2015) Studies: 1 – (Aos et al., Reference Aos, Lee, Drake, Pennucci, Klima, Miller, Anderson, Mayfield and Burley2011); 2 – (Belfield et al., Reference Belfield, Bowden, Klapp, Levin, Shand and Zander2015); 3 – (Wolke, Copeland, Angold & Costello, Reference Wolke, Copeland, Angold and Costello2013); 4 – (Kuklinski, Fagan, Hawkins, Briney & Catalano, Reference Kuklinski, Fagan, Hawkins, Briney and Catalano2015); 5 – (Belfield, Nores, Barnett & Schweinhart, Reference Belfield, Nores, Barnett and Schweinhart2006); 6 – (Reynolds, Temple, White, Ou & Robertson, Reference Reynolds, Temple, White, Ou and Robertson2011); 7 – (Karoly, Kilburn & Cannon, Reference Karoly, Kilburn and Cannon2006); 8 – (Duckworth et al., Reference Duckworth, Peterson, Matthews and Kelly2007); 9 – (Farrington et al., Reference Farrington, Roderick, Allensworth, Nagaoka, Keyes, Johnson and Beechum2012); 10 – (Heckman, Pinto & Savelyev, Reference Heckman, Pinto and Savelyev2013); 11 – (Lippman et al., Reference Lippman, Ryberg, Carney and Moore2015); 12 – (Drago, Reference Drago2011); 13 – (Heckman, Stixrud & Urzua, Reference Heckman, Stixrud and Urzua2006); 14 – (Murnane et al., Reference Murnane, Willett, Braatz and Duhaldeborde2001); 15 – (Groves, Reference Groves2005); 16 – (Duncan et al., Reference Duncan, Dowsett, Claessens, Magnuson, Huston, Klebanov, Pagani, Feinstein, Engel and Brooks-Gunn2007); 17 – (Moffitt et al., Reference Moffitt, Arseneault, Belsky, Dickson, Hancox, Harrington, Houts, Poulton, Roberts and Ross2011); 18 – (Hawkins, Catalano & Miller, Reference Hawkins, Catalano and Miller1992); 19 – (Fletcher, Reference Fletcher2013); 20 – (Berkman & Glass, Reference Berkman and Glass2000); 21 – (Glaeser, Laibson & Sacerdote, Reference Glaeser, Laibson and Sacerdote2000); 22 – (Duckworth et al., Reference Duckworth, Duncan, Kokko, Lyyra, Metzger and Simonton2012); 23 – (Mueller & Plug, Reference Mueller and Plug2006); 24 – (Zax & Rees, Reference Zax and Rees2002); 25 – (Duckworth, Quinn, Lynam, Loeber & Stouthamer-Loeber, Reference Duckworth, Quinn, Lynam, Loeber and Stouthamer-Loeber2011).
-
∙ Tier 1 includes outcomes known to be influenced by noncognitive factors that have been monetized in multiple BCAs, at least based on determined shadow prices. Valuation is relatively straightforward if data are available. These include behaviors that may be greatly driven by many influences (cognitive, contextual, physiological) but for which noncognitive factors are known to play a role in how they manifest (e.g., mental health conditions, educational attainment).
-
∙ Tier 2 includes skills or behaviors where studies indicate indirect links with costs or with other outcomes that have shadow prices, ideally based on demonstrated causal associations. We consider skills listed in that section as potentially monetized for BCA in the near future.
-
∙ Tier 3 includes targeted skills known to be important to the field of social interventions for children and youth, but for which valuation for the sake of BCA will be more involved until further information on the causal linkages becomes available.
The table is intended to be comprehensive for important outcomes to social policy interventions, that is, Tiers 2 and 3 include noncognitive skills corresponding to our discussion above. This distinction requires some subjectivity, but enables us to reflect on the state of valuation for BCA. It is important to consider a broad set of skills that may be targeted through effective programs regardless of how difficult they are to monetize. Ideally, BCAs of programs for children will acknowledge what important targeted program outcomes cannot be valued (what may fall into tier 3) at least based on conventional approaches.
Valuation of noncognitive skills in recent BCAs
Currently, BCA of programs that target noncognitive competencies is facilitated if such observed measures of study impact are available, such as those shown in Tier 1. Observed outcomes found in BCA of programs aimed at youth related to crime can be monetized. Interpersonal criminal acts are costly, given costs incurred by the victim (e.g., due to lost productivity, injury) as well as potentially greater costs to process interpersonal offenses that are apprehended. This includes domestic crime as well. A vast literature has documented costs of child abuse and intimate partner violence that are more likely to occur in aggressive children who do not change their ways by adulthood (Fang, Brown, Florence & Mercy, Reference Fang, Brown, Florence and Mercy2012; Wang, Holton & Prevent Child Abuse America, Reference Wang and Holton2007). If data are available, BCA can involve valuation based on costs of arrest, court costs, and incarceration costs (e.g., Reynolds et al., Reference Reynolds, Temple, White, Ou and Robertson2011). Costs of child abuse can incorporate long-term costs linked to the child victim. Costs to victims can be explicitly measured if data are available. Willingness-to-pay (WTP) estimates have been published that can help valuation of crime-related outcomes that are not directly monetized; for example, see Cohen and Piquero (Reference Cohen and Piquero2009). Data may be sought through observed public court records or they might be reported through informants within a study as well.
When considering BCA for programs for children relevant to crime, this pertains mostly to prevention. In this case, of course, the BCA will directly monetize crime-related outcomes only if follow-up periods sufficiently cover when eventual criminal activity or domestic abuse could be observed. Delinquency costs observable during adolescent periods would involve school resources needed to process offenders. A more specific focus is the costs of bullying in schools, involving school resources needed to address these concerns; costs to victims would also need to be projected. Attention toward understanding the potential economic benefits of programs to prevent bullying have included potential WTP values (Persson & Svensson, Reference Persson and Svensson2013).
Observed mental health conditions determined through professional diagnosis could be considered as partially resulting from early noncognitive skills. Cost of illness studies provide data that can determine shadow prices for associated costs of diagnosis of conditions such as attention deficit hyperactivity disorder (ADHD). This includes costs of services that might be gauged by measuring health care and medication needs. Costs associated with long-term health problems and lower productivity in school or the workplace may also be factored into shadow prices. Programs that reduce the likelihood for diagnosable mental health conditions can be found to produce monetary savings using BCAs without much need for projections.
Valuation of intrapersonal skills in studies of social policy interventions for children is challenging unless they are realized through economic outcomes. However, studies have recognized the economic value of these skills and shadow prices have been employed for certain intrapersonal skills, especially for those observable in outcomes such as educational attainment, employment, mental health, or substance use behaviors. For instance, in economic evaluation of programs aimed at preventing substance use, valuation is sensible given the costs associated with untreated problems. For BCAs, monetization using such data can involve shadow prices for the costs of long-term problems that can be linked to the age of initiation and early or extensive substance abuse.
Demonstrated links to monetized outcomes
Representation of observed behaviors that are influenced by noncognitive ability is relatively common in BCA and precedence from prior work enables the use of these measures in economic evaluation for social policy interventions. In contrast, data on assessed noncognitive factors are not well suited for BCA. Valuation depends on validated links between skills and costs or other outcomes that have shadow prices. However, Tier 1 examples of the use of projected costs in BCA involve both interpersonal and intrapersonal noncognitive competencies (e.g., mental health, early behavior issues) as well as both observed and assessed measurement. Where assessed measurement was used, most of these examples involve links between early measures and eventual outcomes for corresponding constructs (e.g., early aggression to crime).
In their efforts to evaluate programs that serve children in the state of Washington, WSIPP has demonstrated a systematic effort for valuing early assessed skills. Their approach relies on collective evidence from prior research to determine these links, and thus far projected costs have been based on externalizing composite scores, measures of mental health (including mental health, related to employment and wages). (Given their approach of multiprogram evaluation and use of meta-analyses for the valuation process, actual measures differ across studies; see Aos et al. (Reference Aos, Lee, Drake, Pennucci, Klima, Miller, Anderson, Mayfield and Burley2011).) WSIPP also incorporates valuation of risky sexual behavior through teen births (connected to costs related to public assistance, reduced educational attainment, and child abuse) and substance use measures (employment and wages, educational attainment, crime costs); see (Lee et al., Reference Lee, Aos, Drake, Pennucci, Miller and Anderson2012). Given the coverage of several programs in their efforts, WSIPP has become a primary resource for demonstrating where indirect linkages (between proximal outcomes and costs) can be used for valuation of programs for children. Several of the links shown in Table 3 are based on precedence set by their evaluations over the past decade.
OtherexamplesofvaluationinBCAofprogramsforchildrenhave also involved assessed skills. For instance, in the BCA of Communities That Care methods adapted from WSIPP’s approach enabled long-term costs linked to substance use and delinquency through high school (Kuklinski et al., Reference Kuklinski, Fagan, Hawkins, Briney and Catalano2015). In their multiprogram evaluation of SEL interventions, Belfield et al. valued several early outcomes for intrapersonal skills. Their BCA included important shadow prices that captured the value of effective programs aimed at improving early noncognitive competence. This included attention problems (health costs), beliefs about aggression and violence (personal behaviors), continuous measures of mental health, early substance use and misuse (future substance abuse), and risky behavior/sexual behavior (delinquency, health) (Belfield et al., Reference Belfield, Bowden, Klapp, Levin, Shand and Zander2015).
Other research has determined where linkages are evident using retrospective analysis of contributing factors toward program effects. A primary example of this involved determination of the role of noncognitive skills in the long-term effects from the landmark Perry Preschool study (Heckman et al., Reference Heckman, Pinto and Savelyev2013). Specifically, they found that program impact on early behavior (aggression) and on academic motivation (among participating girls) was instrumental toward an overall positive net benefit (Heckman, Moon, Pinto, Savelyev & Yavitz, Reference Heckman, Moon, Pinto, Savelyev and Yavitz2010). Reynolds and colleagues carried out an assessment of the potential influence of noncognitive skills on adult outcomes within the Child-Parent Center intervention in Chicago, finding that a substantial amount of variance in future criminal activity was explained by early noncognitive skills collectively (Reynolds, Temple & Ou, Reference Reynolds, Temple and Ou2010). As with studies generally focused on causal links between early skills and adult outcomes, such evidence from rich experimental data (i.e., randomized groups, long-term follow-up, sufficient measurement coverage) can be vital toward informing valuation.
Monetizable proximal outcomes: challenges and promise
Clearly, incorporation of noncognitive factors has increased for BCA in social policy over the past several years, thanks largely to systematic applied methods by such as WSIPP. The valuation techniques employed have involved careful use of research evidence across multiple studies and allowed BCA to be based not just on direct costs. However, the noncognitive competencies featured in Tables 1 and 2 are still largely excluded from BCA. There are fundamental challenges that impede further valuation for evaluations.
A primary challenge is that the link between noncognitive skills/behaviors and monetized outcomes is always indirect, and many of these links are not yet validated. Valuing a noncognitive skill could be based on the degree to which improvement increases the likelihood for attainment, income or avoidance of costly problems and services. The development of shadow prices relies on understanding these paths as well as how to represent the constructs (i.e., see measurement discussion). The link between something like IQ (a “cognitive” skill) and future attainment is easier to ascertain, partly due to universally accepted measurement processes for IQ. For noncognitive ability related to self-management (mental health), valuation occurs more readily given known associated costs of a diagnosis related to health needs and impact on productivity.
There is also a lack of sufficient data to inform the first issue. The ideal data would include both well-measured noncognitive skills and economic outcomes observed across the life span. Datasets with both long term tracking of respondents and measurement of representative constructs are rare. Data that provide broad inclusion of noncognitive skills are often limited in the ages of follow-up and thus not easily connected to adult outcomes. Further, certain datasets that cover long spans of time may include outdated measurement of early noncognitive skills. An additional concern is the effect of measurement error, especially when relying on informant reports for more abstract attributes. Data are always limited in terms of what and how the constructs were measured. Researchers must make the best efforts with what they have, hoping to face manageable limitations in available data.
Promising directions
In Tier 2 (Table 3), we list categories of competencies that have not been included in BCA for social policy, but for which evidence may exist for inclusion in the short-term future. Citations to studies linking these measures to economic outcomes are also provided, as well as some of the relevant adult outcomes. Of course, careful aggregation of results would be required, with attention to study sample characteristics and other contextual factors and factoring in uncertainty in cost projections. Methodological approaches established by others, notably WSIPP, could help increase confidence in linkages.
Given the interrelationships among the competencies listed, researchers should also account for double counting among linkages and strive for unique causal associations where possible. If appropriate steps can be taken, certain competencies that are common to programs for children may provide good candidates for shadow prices. A good example is characteristics representing self-control or self-regulation. For instance, Moffitt and colleagues demonstrated the long-term link between self-control in children and adult outcomes across multiple domains including crime, personal finances, and health (Moffitt et al., Reference Moffitt, Arseneault, Belsky, Dickson, Hancox, Harrington, Houts, Poulton, Roberts and Ross2011). New research can help strengthen the evidence for causal links between early skills and future costs. The best place to start is where existing evidence already indicates patterns of associations. This is especially important regarding the proximal outcomes that are targeted by interventionists, shown to be both causally linked to future monetized outcomes but also malleable through effective programs.
To illustrate this further, we provide Table A1 that summarizes linkages between noncognitive skills and one outcome category – earnings – in studies using large datasets (skills are listed as they appear in the study). This table represents efforts currently under way to synthesize results from prior studies that will enable shadow prices for possible use in BCA (Karoly, Cannon & Muchow, Reference Karoly, Cannon and Muchow2015). Through this researchers can track the magnitude of the linkages, the methodology used in the study as well as other details about the results and sample; such collective evidence can be important in determining shadow prices for social policy. Clearer delineation of these constructs (how to measure them) and evidence of causal pathways would better enable shadow price development. Where this can occur, it would enable more complete BCA of programs for children. Collective use of the same measure can also help strengthen the available evidence. For instance, the same measure for self-esteem (Rosenberg Self-Esteem Scale) is used in all studies as represented in Appendix A for that variable. Going forward, organized research efforts such as this could help accelerate the process.
Further investigation
The third tier in Table 3 includes noncognitive competencies identified in the review by Lippman et al. that are not viable for BCA currently, and there exists limited evidence for linkages for costs that will enable shadow prices. Of course, these skills are likely good candidates for enhancement through effective programming efforts, given their identified role as important noncognitive factors. They are also considered as important fundamental competencies by early childhood interventionists, educators, and parents. A broader effort toward shadow price development may be necessary to make sufficient progress in the field. The process is not just challenged by insufficient research examining links between these skills and economic outcomes. More clarification may be necessary for best ways to measure. The more abstract the skill, the harder it will be to establish a clear linkage. This is especially true for intrapersonal skills where limited measurement options exist and the competency is not stable in development. Greater focus is warranted toward skills that are likely malleable (i.e., not representing more fixed personality traits) and skills where unique influence can be determined.
Data, methodology, and collaboration for moving forward
Despite these obstacles, it will be important to progress in this area. Original research could be carried out to help further the evidence necessary for developing shadow prices that could facilitate BCA of programs for children in the coming years. Longitudinal data are available that allow us to examine links between early skills and future costs. Well-known publically available data include the National Longitudinal Study of Youth (Bureau of Labor Statistics, 2002) and the Panel Study of Income Dynamics (Hofferth, Davis-Kean, Davis & Finkelstein, Reference Hofferth, Davis-Kean, Davis and Finkelstein1997) among others. Ideally these data allow us to examine a full set of late adolescent and/or adult outcomes as well as include good coverage of noncognitive skills in early development. In many available datasets (especially older ones) the latter may be limited. Other data from studies in the behavioral sciences (such as intervention evaluations) can provide better coverage of noncognitive skills but may not involve a representative sample or follow participants long enough. Research organizations recognize the importance of assessing how these early skills impact current and future well-being, so there is much attention directed toward what current data can provide and what new data may be useful. For instance, the Organisation for Economic Co-operation and Development has plans to lead an international data collection effort to examine the long-term role of social–emotional skills in healthy development among adolescents (Miyamoto et al., Reference Miyamoto, Huerta and Kubacka2015). However, research toward understanding these links for BCA must rely on what data are available now. Efforts toward determining links between early skills and economic outcomes must acknowledge all data limitations in order to represent uncertainty appropriately. Decisions also must be made for how to represent skills over time, especially in terms of what ages. For instance, prior important work has involved averaging measurement across several formative years (e.g., Moffitt et al., Reference Moffitt, Arseneault, Belsky, Dickson, Hancox, Harrington, Houts, Poulton, Roberts and Ross2011). This can be a useful strategy, especially if efforts are made to standardize for other important factors such as situational incentives (Heckman & Kautz, Reference Heckman and Kautz2012).
Irrespective of good data, it is challenging to identify causal influences in these linkages. Regression models can demonstrate important unique predictive relationships but cannot determine causality per se. Randomized-control trials could provide important information on causal paths but only if the mechanism involves program impact on limited skills. Typically programs aim at improving multiple noncognitive skills; thus available data may not provide a clear picture unless isolated effects occur. Other methodological approaches could be employed for observational data (without experimental conditions) that could include instrumental variables or propensity scores, even considering continuous factors (Lippold, Coffman & Greenberg, Reference Lippold, Coffman and Greenberg2014). However, the issue of sorting through multiple skills is still challenging with such models. Another approach could involve mediational models, where temporal ordering of skills allows one to consider development and assess multiple mediators at a time (e.g., unique indirect effect of school engagement on future attainment mediated through more proximal achievement in midgrades).
Still there are various approaches and data that could help provide information to build on through a series of analyses, using transparent methodology and clearly stating limitations. Individual pathways between noncognitive skills and costs are unlikely to occur in isolation. For the sake of BCA, however, separate shadow prices for separate skills will be very useful. (Adjustments for double counting can be employed in the valuation process.) More immediate value could come from determining links between skills that are more clearly aligned with subsequent economic outcomes. The first step could involve mapping of what important linkages should be validated for BCA. The skills listed in Table 2 could be considered for their possible influence on current or future costs, for example, substance use attitudes to later substance abuse problems, early emotion management skills to longer term likelihood for mental health conditions, and early school engagement to attainment in school. Ideally something like early antisocial behavior (measured on a continuous scale) can be studied for future likelihood of crime and additionally whether this involves interpersonal crime (i.e., with larger economic implications). As has occurred through WSIPP’s efforts, focus should go on where links are best demonstrated based on repeated evidence, either from prior research or new analyses.
Contingent valuation methods (CVMs) for noncognitive skills will be challenged by the same measurement issues discussed above. Continuous measures have been valued before for BCA, for instance based on IQ points for assessing potential environmental hazards (von Stackelberg & Hammitt, Reference von Stackelberg and Hammitt2009). Increased emphasis on the importance of early skills such as self-control and self-esteem could make contingent valuation surveys more meaningful (e.g., for parents). Still CVM will be more readily applicable to observed noncognitive skills, where measurement units are interpretable. Behavioral scientists could make an effort to instigate CVM surveys from some of the more widely perceptible noncognitive behaviors such as early substance use, delinquent acts in school, or early indication of mental health issues. Prior studies demonstrating links with key behaviors could help inform CVM survey procedures. For instance, if substance use attitudes are shown to predict subsequent substance use behavior, researchers could frame how much change in attitudes implies a certain interpretable difference in behavior. In this way, both data and CVM could aid in the derivation of shadow prices.
Working across disciplines can also better ensure standardization across studies (Karoly, Reference Karoly2012), especially important for projecting costs. Different procedures and approaches through different BCAs will only serve to muddle the process. Toward this end, current organizations through committees and task forces are encouraging application of BCA in the behavioral sciences promoting increased standardization, communication, and transparency in the process. This includes recent efforts through the Institute of Medicine (Board on Children Youth and Families, 2014), the Pew-MacArthur Results First Initiative (Pew-MacArthur Results First Initiative, 2013), the Society for Prevention Research’s Taskforce on Economic Analyses of Prevention, and the National Institutes of Health supported Prevention Economics Planning and Research Network. Such efforts can help promote methodologies that involve representation of uncertainty in cost estimation, through the use of Monte Carlo techniques, for instance (Weimer & Vining, Reference Weimer and Vining2009). Ideally it will also involve acknowledgment of contextual and demographic factors for the target population as well as the source of any derived shadow prices. Bounded estimates of cost projections will still provide important information in the economic evaluation of social policy.
Conclusion
The potential for valuation of noncognitive skills has increased in the past several years but the research demonstrating the importance of these factors continues to outpace their inclusion in BCA. However, this is starting to change and progress in valuing these competencies will be necessary in order to best evaluate the full economic impact of childhood interventions. Shadow prices may not be achievable for many noncognitive skills currently, yet research may provide more evidence for costs in the future. Interventionists should consider their logic models and the potential impact of the program beyond the planned evaluation design (beyond planned measurement periods or spillover impact, for instance). A benefits map (see Belfield et al., Reference Belfield, Bowden, Klapp, Levin, Shand and Zander2015) can provide a way for researchers to consider what can and cannot (at least currently) be monetized for BCA of social policy intervention. Further progress toward an agreed upon strategy for defining, measuring, and valuing noncognitive skills can increase the likelihood that their inclusion in BCA is more feasible. This should be done with care (robust methodology, consistency across studies, control for uncertainty, etc.), but any new progress can better enable the evaluative process to inform policy on the potential economic benefits from effective programs for children and youth.
Appendix A. Studies demonstrating links between noncognitive skills and earnings
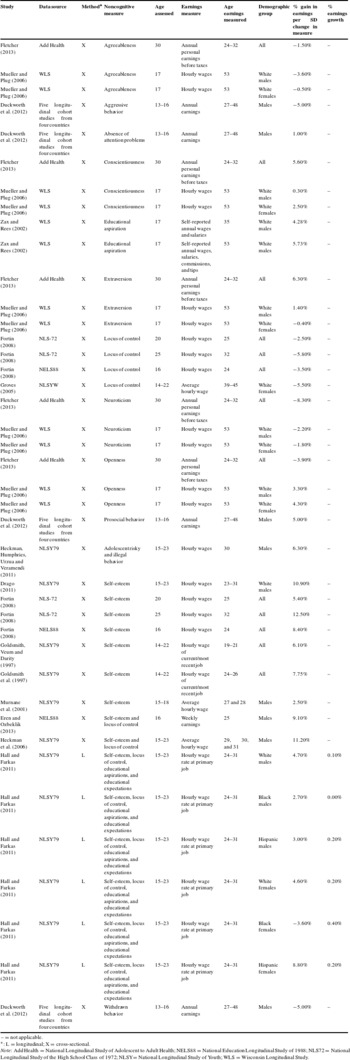