1. Introduction
Climate change is no longer a concept but reality. Confronting Southeast Asia where millions of residents earn their livelihoods through agriculture, it is among the greatest challenges threatening development and poverty eradication (Asian Development Bank, 2009). Vietnam is among the countries to be worst affected (Dasgupta et al., Reference Dasgupta, Laplante, Meisner, Wheeler and Yan2007). Engendering increased temperatures, rainfall fluctuations, and increased weather extremes, climate change poses huge threats to the agricultural sector because of its direct exposure and reliance on weather conditions. Paddy rice, of course, is not an exception.
Paddy rice plays a crucial role in the nation's economy in that it sustains food security, creates rural employment, and serves as a source of export revenues. Rice farming is at least one source of income, if not the only, for more than 75% of poor households and 48% of nonpoor households (Yu et al., Reference Yu, Zhu, Breisinger and Hai2010). As the second-largest shipper, Vietnam alone accounts for approximately 20% (by quantity) of the total world rice trade.Footnote 1 Its economic significance and climatic vulnerability have urged researchers to study how changes in climate would affect rice yield/production. A question that is of at least equal importance but has not received sufficient attention is how potential yield impacts affect the rice market. This study was purposely conducted in an attempt to answer that question. We first determine the long-run effects of climate change on rice yield using the cointegration framework. Estimated parameters are then plugged into an equilibrium displacement model (EDM) to simulate impacts of climate change on Vietnam's rice market. In determining the effects of climate change on rice yields, we focus on temperatures and precipitation as they are most likely to be altered under climate change.
The remainder of the article begins with a brief literature review of relevant studies (Section 2). This is followed by sections on data and variables (Section 3) and cointegration tests to determine yield impacts of temperatures and precipitation (Section 4). In Section 5, a model is built for Vietnam's rice market, on which simulations are performed (Sections 6 and 7). The article concludes with a summary of key findings (Section 8).
2. Literature Review
As for many other regions throughout the world, temperatures and precipitation play a crucial role in the production potential of major crops in Southeast Asia. The effects of these factors are, however, highly country specific because one country is distinguishable from others with regard to its sensitivity to weather conditions.
Using data from farmer-managed irrigated rice fields in six important rice-producing countries in tropical/subtropical Asia, Welch et al. (Reference Welch, Vincent, Auffhammer, Moya, Dobermann and Dawe2010) reached the conclusion that rice yields plummet as nighttime temperatures are higher. Daytime temperatures up to a point are found to enhance yields. That being said, they cautioned that any gains caused by higher daytime temperatures are likely to be outweighed by losses resulting from higher nighttime temperatures as temperatures are rising faster at nights. Additionally, if daytime temperatures become too high, this will impede rice yields, inflicting more loss. In Malaysia, Alam et al. (Reference Alam, Talib, Siwar and Toriman2014) reported that rice yields plunge by 3.44% in the current season and 0.03% in the next season in response to a 1% increase in temperature. Utilizing the crop model ORYZA 2000, Vaghefi et al. (Reference Vaghefi, Shamsudin, Makmom and Bagheri2011) predicted a decline of 0.36 t/ha in rice yield under the scenario of a 2°C increase in temperature at a CO2 concentration of 383 ppm. If the level of CO2 concentration rises to 574 ppm, the loss is 0.69 t/ha. In the Philippines, Peng et al. (Reference Peng, Huang, Sheehy, Laza, Visperas, Zhong, Centeno, Khush and Cassman2004) reported a drop of 10% in rice yields for every 1°C increase in minimum temperature during the dry cropping season (January to April), whereas maximum temperatures were insignificant. In the Mekong Delta in Vietnam, it is estimated for dry-season crops that if temperatures in January fall below 19°C, every 1°C drop would cause a loss of 0.12 t/ha. For wet-season crops, each 1°C increase beyond 35°C would lead to a plunge of 0.38 t/ha (Nhan, Trung, and Sanh, Reference Nhan, Trung, Sanh, Stewart and Coclanis2011).
Regarding precipitation, it is reported that rice yields in Malaysia plunge by 0.12% in current season and 0.21% in the following season as a result of 1% increase in precipitation (Alam et al., Reference Alam, Talib, Siwar and Toriman2014). In Indonesia, the Philippines, and Laos, the 2009–2010 El Niño,Footnote 2 for instance, caused rice yields to drop, respectively, 3%, 6.6%, and 6.74% relative to 2008–2009 (Shean, Reference Shean2014). Naylor et al. (Reference Naylor, Battisti, Vimont, Falcon and Burke2007) estimated that a 1-month delay in the onset of the rainy season during El Niño years would upset rice production in the wet season (January–April) in Indonesia in that production in West/Central Java would fall by approximately 6.5% and in East Java/Bali by 11.0%. In the Mekong Delta, wet-season rice production would lose 0.2 t/ha for each 100 mm increase in precipitation beyond 250 mm, and 0.6 t/ha for each 100 mm decrease below 50 mm (Nhan, Trung, and Sanh, Reference Nhan, Trung, Sanh, Stewart and Coclanis2011).
Although studies that evaluate climate change impacts on rice production are plenty, studies that go further to assess impacts on rice market are few. Particularly for Vietnam, the study by Furuya, Kobayashi, and Yamauchi (Reference Furuya, Kobayashi and Yamauchi2014) is, to the best of my knowledge, the first and only one that touches on how the rice market would react in response to changes in climate. The variable of interest in their study is, however, not temperature nor precipitation but evapotranspiration (ET). The reasoning behind the study is as simple as this: Climate change leads to changes in ET. Changes in ET in turn result in changes in rice yields and farming areas. At the end of the day, climate change would shock the rice market through changes in the rice supply. The following two simulations were conducted in the study: (a) the baseline, which assumes that ET after 2000 is the average of ET from 1995 to 1999; and (b) the CC-B2, in which ET is presumed to accord with the Intergovernmental Panel on Climate Change (IPCC) B2 scenario. By contrasting the CC-B2–simulated results with those of the baseline, the authors ended up with effects of climate change on the rice market. It is reported that in the Mekong Delta climate change would depress rice production in the wet and dry seasons, respectively, by 1.76% and 2.19% between 2026 and 2030 relative to the baseline. Consequently, farm prices in the CC-B2 simulation are 8.31% higher. For the country as a whole, the 2026–2030 average farm price in the CC-B2 is 415,000 dong/t higher than that in the baseline because climate change is expected to decrease the total production of rice. In spite of higher forecasted rice prices, the CC-B2 yields an almost identical projection of rice demand to that in the baseline. Per capita rice consumption would step up steadily from 229.2 kg in 2010 to 258.6 kg in 2030. There is no apparent difference in the 2026–2030 average per capita rice consumption between the two simulations. Such likeness can be explained as follows: The surge in per capita consumption is the net outcome of the positive effect of income growth and the negative effect of rice price increase. The two simulations make use of the same projections of income growth (provided in the IPCC's special report on emissions scenarios B2; see Gaffin et al., Reference Gaffin, Rosenzweig, Xing and Yetman2004) but differ in their own projections of rice prices. Because the effect of income overwhelmingly outweighs that of price, both simulations end up with nearly identical projections of per capita rice consumption.
3. Data and Variables
Vietnamese farmers, as a general practice, cultivate three rice crops a year, which are traditionally named spring, autumn, and winter.Footnote 3 Yield data measured in metric tons per hectare are available for each cropping season on a yearly basis from 1975 to 2014 collected by the General Statistics Office of Vietnam. Although crop lengths vary over years, the spring crop across the country starts and finishes roughly between January and April, the autumn crop between May and September, and the winter crop between September and December. We also see this “grouping” adopted in Furuya et al. (Reference Furuya, Meyer, Kageyama and Jin2010), which they note is based on the cropping calendars of the U.S. Department of Agriculture (1994). Figure 1 provides a snapshot of rice yields of each of the three cropping seasons. In all three seasons, yields have been soaring since 1975 with the spring crop being notably more productive than the other two.

Figure 1. Rice Yields (t/ha) in Three Cropping Seasons in Vietnam 1975–2014
Data on maximum and minimum temperatures and precipitation are obtained from the Climatic Research Unit (University of East Anglia) and are 0.5° × 0.5° gridded station data. Available from 1975 to 2014 on a monthly basis, maximum and minimum temperatures (°C) are monthly averages, and precipitation is the total amount per month (millimeters per month). In the forthcoming analysis, months are grouped according to the length of each cropping season. After being grouped, maximum/minimum temperatures and precipitation are seasonal averages. The spring cropping season is characterized by the lowest maximum/minimum temperatures and precipitation (Figures 2, 3, and 4). The autumn cropping season is hottest and receives the highest amount of precipitation. The temperature gap between the spring and autumn cropping seasons is roughly 5°C.

Figure 2. Maximum Temperatures (°C) in Three Cropping Seasons in Vietnam

Figure 3. Minimum Temperatures (°C) in Three Cropping Seasons in Vietnam

Figure 4. Precipitation (mm/month) in Three Cropping Seasons in Vietnam
Yield and weather variables enter the empirical analysis in logarithms. We use a double-log specification in order to obtain the elasticities of yields with respect to temperatures and precipitation, which are ultimately used to simulate the economic model for Vietnam's rice market.
4. Empirical Analysis
4.1. Unit Root Tests
Times series are prone to be nonstationary, which renders usual regressions spurious. The cointegration concept (Granger, Reference Granger1981) provides a framework to deal with nonstationary time series. A cointegrated relationship implies that the two series have a long-run equilibrium, and therefore, they cannot drift too far away from each other (Ender, Reference Ender2004). A cointegration test demands that the series be integrated to the same order. The first step is therefore to check for unit roots in all variables at their levels and first differences. Table 1 presents the τ-statistics of the unit root tests (Dickey and Fuller, Reference Dickey and Fuller1979). At 5% level of significance, all variables are integrated to order 1.
Table 1. Unit Root Tests

Notes: Critical value for unit roots tests with constant at the 5% level is −2.893 (MacKinnon, Reference MacKinnon, Engle and Granger1991). FD, first difference.
4.2. Cointegration Tests
Engle and Granger (1987) were the first to devise a method to test for cointegration. This two-step method is, however, suitable when the test involves two variables (Kennedy, Reference Kennedy2008). For three or more variables, the Johansen method (Johansen, Reference Johansen1988) is preferred. Prior to the tests, the choice of the number of lags in the underlying vector autoregressive (VAR) is made; in this article, the choice is guided by the Akaike information criteria. Table 2 presents the Johansen's trace statistics for each of the three cropping seasons. For the spring crop, the trace statistic at rank = 1 is 23.52, which is smaller than the 1% critical value. We therefore cannot reject the null hypothesis that there is one cointegrating vector among interested variables. In the same manner for the autumn crop, the trace statistic at rank = 2 fails to exceed the critical value, implying two cointegrating vectors. Multiple cointegrating vectors do not imply multiple long-run equilibria, but rather multiple sector equilibria in a long-run equilibrium (Kennedy, Reference Kennedy2008). Researchers often discard those vectors that make no economic sense. For the winter crop, the trace test gives the conclusion that there exists no integration among interested variables.
Table 2. Johansen Trace Tests for CointegrationFootnote a

a Five lags are used in the test for the spring crop, six lags for the autumn crop, and three lags for the winter crop.
Note: Asterisk (*) indicates the number of cointegrating vectors among variables at 1% level of significance.
For the spring and autumn crops, the long-run relationships between yields and weather variables are presented in Table 3. Maximum temperatures have negative effects on yields of both spring and autumn crops, whereas minimum temperatures have a positive effect on the spring crop but a negative effect on the autumn crop. In response to a 1% increase in maximum temperatures, spring crop yield would reduce by 28.8% and autumn crop yield 43.87%. A 1% increase in minimum temperatures would boost spring crop yield by 17.12% but reduce autumn crop yield by 0.32%. As it is remarkably warmer in the autumn cropping season, temperatures inflict greater harm and precipitation yields greater benefit to the autumn crop than they do to the spring crop. In both cropping seasons, precipitation is positively linked to yields. A 1% increase in precipitation would ramp up spring crop yield by 1.81% and autumn crop yield by 4.31%.
Table 3. Effects of Temperatures and Precipitation on Rice Yields

a Lagrange-multiplier test for serial correlation up to 10 lags.
Notes: P values are in parentheses. LHS, left-hand side; RHS, right-hand side.
Gonzalo (Reference Gonzalo1994) indicates that underspecifying the number of lags in the underlying VAR can significantly increase the finite-sample bias in the parameter estimates and lead to autocorrelation. We therefore perform Lagrange-multiplier tests to check for autocorrelation up to 10 lags. The tests fail to reject the null hypothesis of no autocorrelation, which implies that the number of lags is correctly specified. We also test the normality of the residuals using the Jarque-Bera tests. The tests conclude that the null hypothesis of normally distributed errors cannot be rejected.Footnote 4
5. Vietnam Rice Market Model
Here we specify a structural model for the Vietnam rice market that is built on Wailes and Chavez's (2011) work. The model consists of the supply sector, the demand sector (domestic demand and export), stocks, and price linkage equations:

where YS , YA , and YW are, respectively, yields in spring, autumn, and winter crops;Footnote 5 AS , AA , and AW are farming areas; TSMin , TAMin , TWMin , TSMax , TAMax , TWMax , RS , RA , and RW represent minimum/maximum temperatures and precipitation; Q is total milled rice production in three seasons; EST and BST represent milled rice ending and beginning stocks, respectively; D and E are, respectively, domestic and foreign demand; and P, PF , and FOB are wholesale, farm, and export prices, respectively.
Equations (1)–(7) characterize the supply sector. Yields are specified as functions of temperatures and precipitation. Farming areas are predicated on farm prices. We assume that harvested areas equal farming areas. Total milled rice production is the sum of the products of yields and farming areas scaled down by the paddy-milled rice conversion rate (0.667).Footnote 6 Equations (8) and (9) model the demand sector in which the domestic demand responds to wholesale prices, and the export demand to FOB prices. Ending stock (equation 10) is a function of total milled rice and export prices. Farm price is linked to wholesale price (equation 11), and wholesale price is modeled as a function of FOB price (equation 12). The market-clearing condition (equation 13) requires that ending stocks be the residual of total milled rice plus beginning stocks net of demand and export. Beginning stocks are simply ending stocks of the previous year. The model contains 13 endogenous variables (YS , YA , YW , AS , AA , AW , Q, D, E, EST, FOB, PF , and P) and 10 exogenous variables (TSMin , TAMin , TWMin , TSMax , TAMax , TWMax , RS , RA , RW , and BST). All other exogenous variables that affect rice yields and supply and demand of rice are not in the scope of this study and are therefore suppressed.
Climate change is associated with fluctuations in temperatures and precipitation. In order to simulate the impacts of climate change on the rice market, the structural model is first expressed in the equilibrium displacement form as follows:
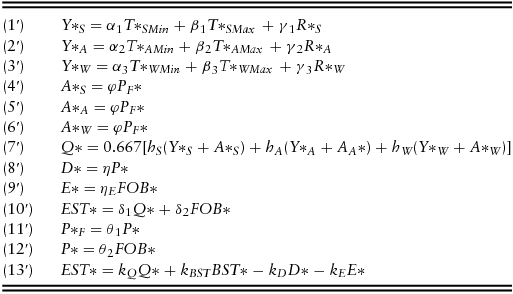
where the variables with asterisks indicate percentage changes (X* = dlnX = dX/X); α i , β i , and γ i (i = 1, 2, 3) are the elasticities of yields with respect to minimum temperatures, maximum temperatures, and precipitation in each cropping season, respectively; and φ is the elasticity that reflects farmers’ sensitivity to farm prices in their decisions on farming areas. Here, we assume that farmers are equally price sensitive across three cropping seasons; hj = (Yj *Aj )/Q j = S, A, W are the quantity shares of spring, autumn, and winter rice production in total milled rice; δ1 and δ2 are, respectively, the elasticities of ending stocks with respect to total milled rice and FOB prices; η and η E are price elasticities of domestic and export demand, respectively; θ1and θ2 are, respectively, the wholesale-farm and FOB-wholesale price transmission elasticities; kQ = Q/EST, kBST = BST/EST, kD = D/EST, and kE = E/EST are, respectively, the ratios of total milled rice, beginning stock, domestic demand, and export to the ending stock.
6. Parameterization
Our study benefits from Wailes and Chavez's (2011) in the sense that most of the key elasticities are retrieved from their study. Specifically, the elasticities of ending stocks with respect to milled rice (δ1), and FOB prices (δ2) are, respectively, 2.386 and −1.178.Footnote 7 The domestic demand price elasticity (η) is set to −0.2. The price elasticity of farming areas (φ) is 0.007. The price transmission elasticities (θ1, θ2) are, however, not readily available. Wailes and Chavez (Reference Wailes and Chavez2011) estimated that P* = 0.987P* F and P* F = 0.541FOB*. Thus, the value of θ1 is simply equal to 1/0.987 = 1.013 and θ2 is 0.987 × 0.541 = 0.534.
The elasticities of yields with respect to temperatures and precipitation (α i , β i , γ i ) are set to the estimated parameters in Table 3.Footnote 8 The quantity shares of spring, autumn, and winter rice production in total milled rice (hj ) are set to their 2009–2013 averages as given in Table 4. The export demand elasticity (η E ) is −30, taken from Minot and Goletti (Reference Minot and Goletti2000). The parameters kQ , kBST , kD , and kE take on their 2008–2012 mean values (Table 4).
Table 4. Definitions and Baseline Values for Model Parameters

a These are 2009–2013 mean values computed from data provided by the General Statistics Office of Vietnam.
b These are 2008–2012 mean values computed from data obtained from the U.S. Department of Agriculture.
7. Simulation
The EDM model (equations 1′–13′) can be equivalently expressed in matrix form as XY = Z, where Y is the column vector of percentage changes in endogenous variables relative to an initial equilibrium; X is the matrix of parameters corresponding to endogenous variables; and Z is the column vector of zeros and percentage change in exogenous variables along with their parameters. The percentage changes in endogenous variables in response to a 1% change in exogenous variables can be obtained by Y = X −1 Z.
Table 5 presents the effects of spring temperatures and precipitation on the rice market. It is no surprise that the effects of maximum temperatures outweigh those of minimum temperatures and precipitation. For instance, a 1% increase in maximum temperatures in the spring cropping season, viewed in isolation, would reduce total milled rice by 9.02%. In contrast, every 1% increase in minimum temperatures and precipitation would increase total milled rice by 5.36% and 0.57%, respectively. The variable that boosts/shrinks total milled rice would depress/increase prices. The price effects at farm and wholesale levels are almost identical, which is explained by the price transmission elasticity of 1.013. The overall impression is that effects on the export market overwhelmingly exceed those on the domestic market. For instance, every 1% increase in maximum temperatures, viewed in isolation, would increase domestic prices (farm and wholesale prices) by 0.94% while increasing FOB prices by 3.51%. Consequently, domestic consumption would fall by 0.19% compared with a drop of 27.95% in exports. That domestic consumption falls far less than exports is partially ascribed to the magnitudes of the corresponding price elasticities. While that of the domestic demand is −0.3, that of the export demand is −30. Similar analysis applies to the effects of autumn temperatures and precipitation on the rice market (Table 6).
Table 5. Effects of 1% Change in Spring Temperatures and Precipitation on Rice Market

Table 6. Effects of 1% Change in Autumn Temperatures and Precipitation on Rice Market

In order to determine effects of climate change on the rice market, we utilize projected changes in temperatures and precipitation under the low emission scenario (B1) compiled by the Vietnamese Ministry of Natural Resources and Environment (2009). The report, however, provides projected changes for mean temperatures, not maximum or minimum temperatures (Table 7). We, therefore, assume that maximum and minimum temperatures would increase by the same Celsius degrees as mean temperatures. This might be a very strong assumption, but these are the best-bet projected changes for maximum and minimum temperatures. Percentage changes are then computed by dividing absolute change (°C) by the corresponding 1980–1999 baseline values. For precipitation, projected changes are already expressed in percentage changes in the report. Table 8 summarizes projected changes in temperatures and precipitation.
Table 7. Projected Changes (°C) in Mean Temperatures 2020–2040Footnote a

a These changes are under the B1 emission scenario compared with the 1980–1999 baseline.
Source: Author's calculations based on the Vietnamese Ministry of Natural Resources and Environment (2009).
Table 8. Projected Changes (%) in Maximum and Minimum Temperatures and Precipitation 2020–2040Footnote a

a For spring, the 1980–1999 baseline values for maximum and minimum temperatures are 26.86°C and 18.53°C, respectively. For autumn, these figures are 31.05°C and 23.67°C.
Source: Author's calculations based on Vietnamese Ministry of Natural Resources and Environment (2009).
Projected changes (%) in temperatures and precipitation in each cropping season are multiplied by their corresponding 1%-change effects (Tables 5 and 6). Intermediate results are summed across weather variables and then across cropping seasons. The final results that reflect the effects of climate change on the rice market are summarized in Table 9.Footnote 9 Thus, without the application of interventions and new cropping advancements, results suggest a plunge of 17.89% in total milled rice by 2030. In response, farm and wholesale prices each increase by 1.86%. Being price inelastic (−0.2), domestic demand consequently falls by 0.38%. The export sector would experience a rise of 6.94% in export prices and a drop of 55.36% in export quantities. Farmers would suffer from a sales loss of 16.02%, whereas wholesalers would see a sales gain of 1.48%.Footnote 10 For exporters, their sales loss would amount to 48.42%.
Table 9. Effects of Climate Change on Rice Market 2020–2040 (% change)

The effects on the export sector are quite substantial partly because of the large price elasticity of export demand (−30). This parameter value is taken from Minot and Goletti's study that dated back to Reference Minot and Goletti2000. As we do not want to overestimate the effects of climate change on this sector, a naturally arising question would be how these effects will alter if the export demand happens to be more/less price elastic. Basic microeconomic theory advises that as demand becomes less price elastic, a shift in the supply curve would lead to greater change in price but smaller change in quantity. Here, we therefore expect that a less price elastic export demand would amplify the effects on FOB prices while rendering the effects on export quantities less pronounced. Additionally, sales loss would become less severe. In an attempt to check our economic intuition, we experimentally set the export demand price elasticity alternatively to −20, −10, and −5 and redo the simulation. For simplicity, we only report changes by 2030 (Table 10). Toward the decline of η E (in absolute terms), the effects on FOB prices are enhanced, indicating greater increase in prices. In contrast, the effects on exports are less pronounced, indicating less reduction in quantities exported. Finally, less negative effects on exporters’ sales imply that exporters experience fewer losses.
Table 10. Climate Change Effects on Exports with Various Export Demand Price ElasticitiesFootnote a

a These are projected percentage changes by 2030.
8. Conclusion
This study aims to quantify effects of climate change on rice yield and, more importantly, the rice market in Vietnam. We approach the research objective by first determining yield effects of maximum/minimum temperatures and precipitation. Given the estimated parameters, we simulate an EDM for Vietnam's rice market to obtain effects of a 1% change in temperatures and precipitation on rice market. Finally, by combining these 1%-change effects with projected changes in temperatures and precipitation under the low emission scenario (B1), we are able to quantify effects of climate change on the rice market.
Results suggest that temperatures and precipitation are tied to rice yields in the spring and autumn crops. In the event of climate change, these two climatic elements can probably wreak havoc on Vietnam's rice economy if no interventions or improvements in growing techniques are put in place. Rice production would drop by as much as 18% by 2030. The largest loss is accrued to exporters as they see their export quantities and sales drop by a half by 2030. In the domestic market, because of a plunge in rice production, consumers would have to pay more. For farmers, increase in farm prices is not sufficient to neutralize reduction in production, resulting in a loss of sales. Only wholesalers benefit as they would see a rise in sales. This gain is, however, far too modest compared with losses that accrued to farmers and exporters. Interventions and/or advancements in growing techniques are, therefore, a must in order to mitigate these adverse effects.
It is well known that temperatures (and perhaps precipitation) affect rice yields differently in different growth stages. Thus, a potential extension to this study is to include as explanatory variables temperatures and precipitation of each of every month during the crop length instead of seasonal averages. This way, one could achieve more precise and detailed insights of the effects of these weather factors on rice yields. This approach, however, demands a longer time series to compensate for the increase in the number of regressors.
Another issue that should be addressed in future studies is the use of aggregated data (country averages). As Vietnam straddles a wide range of latitudes (8° to 23° north of the equator), the northern parts are distinguishable from the southern parts in terms of climate conditions. Southern Vietnam experiences a tropical climate with two distinguished seasons—namely, the dry and wet seasons—whereas the northern parts enjoy a subtropical climate with more distinct seasonal variations. Thus, the use of aggregated data has blurred climatic distinctions among regions of the country. Although acknowledging the disadvantage of using aggregated data, we are unable to tackle the issue in the current study because regional yield data are available only for 1991–2014, which does not provide sufficient observations for the cointegration analysis.