1. Introduction
A tipping point is where a small intervention leads to large and long-term consequences for the evolution of a complex system, profoundly altering its mode of operation (Gladwell, Reference Gladwell2000; Lenton et al., Reference Lenton, Held, Kriegler, Hall, Lucht, Rahmstorf and Schellnhuber2008). Such highly non-linear response is usually self-propelling and hard to reverse. Tipping points can interact across systems, spatial and temporal scales (Lenton, Reference Lenton2020). Crucial to their occurrence is the presence of strongly reinforcing positive feedback within a system, which can amplify a small initial change and turn it into a large consequence.
The recognition of ‘negative’ tipping points in the climate, ecological and biogeochemical systems was key to identifying and setting several of the ‘planetary boundaries’ (Rockström et al., Reference Rockström, Steffen, Noone, Persson, Chapin, Lambin, Lenton, Scheffer, Folke, Schellnhuber, Nykvist, de Wit, Hughes, van der Leeuw, Rodhe, Sörlin, Snyder, Costanza, Svedin and Foley2009). Recently, evidence that such tipping points may be approaching has underpinned declarations of a climate and ecological emergency (Lenton et al., Reference Lenton, Rockstrom, Gaffney, Rahmstorf, Richardson, Steffen and Schellnhuber2019). This in turn has led to increasingly ambitious targets to tackle climate change and reverse biodiversity decline – notably the target of limiting global warming to 1.5 °C. But such targets demand transformative rates of societal change – including a continuous, roughly 7% per year average global decline of greenhouse gas emissions from now on (Otto et al., Reference Otto, Donges, Cremades, Bhowmik, Hewitt, Lucht, Rockström, Allerberger, McCaffrey, Doe, Lenferna, Morán, van Vuuren and Schellnhuber2020a) exceeding 10% per year in advanced economies (Anderson, Broderick, & Stoddard, Reference Anderson, Broderick and Stoddard2020). Hence, there is a growing consensus that some social actors need to identify and trigger ‘positive tipping points’ (or ‘sensitive intervention points’) to accelerate progress to achieve the required, transformative rates of change for everyone (Farmer et al., Reference Farmer, Hepburn, Ives, Hale, Wetzer, Mealy, R., Srivastav and Way2019; Jordan et al., Reference Jordan, Sala, Stafford, Bubier, Crittenden, Cutter, Kay, Libecap, Moore, Rabalais, Shepherd and Travis2010; Lenton, Reference Lenton2020; Otto et al., Reference Otto, Donges, Cremades, Bhowmik, Hewitt, Lucht, Rockström, Allerberger, McCaffrey, Doe, Lenferna, Morán, van Vuuren and Schellnhuber2020a; Sharpe & Lenton, Reference Sharpe and Lenton2021; Tàbara et al., Reference Tàbara, Frantzeskaki, Hölscher, Pedde, Kok, Lamperti, Christensen, Jäger and Berry2018; Totten, Reference Totten2012; van Ginkel et al., Reference van Ginkel, Botzen, Haasnoot, Bachner, Steininger, Hinkel, Watkiss, Boere, Jeuken, Sainz de Murieta and Bosello2020; Westley et al., Reference Westley, Olsson, Folke, Homer-Dixon, Vredenburg, Loorbach, Thompson, Nilsson, Lambin, Sendzimir, Banerjee, Galaz and van der Leeuw2011).
A defining quality of such positive tipping points is that they are intentional. Transformative change can happen in any sufficiently complex adaptive system (Levin, Reference Levin1998) without anyone willing it to happen. It requires innovations occurring within a system that instigate feedback processes and are subject to some filtering or ‘selection’ process (Lenton et al., Reference Lenton, Kohler, Marquet, Boyle, Crucifix, Wilkinson and Scheffer2021). For example, in Earth history there were several pivotal ‘revolutions’ – meaning transformative changes in the identity and functioning of a complex system (here the Earth system) – long before humans evolved (Lenton & Watson, Reference Lenton and Watson2011). Several ‘revolutions’ in human history also appear unintentional, for example, the Neolithic revolution or the industrial revolution. These arguably hinged on changes in norms triggering reinforcing feedbacks, including innovation based on already-known technologies. Whether the social and technological innovators intended to revolutionise the world is unclear. Other large-scale human ‘revolutions’ are portrayed as intentional, notably the ‘green revolution’ (driven by a relatively small group of actors intent on radically increasing food production) – but there was still a rich mix of intentional and unintentional change at play. Now that we are explicitly considering collective, intentional transformation towards global sustainability, we are entering arguably unique territory (Lenton & Latour, Reference Lenton and Latour2018). As such we need to draw some sort of ‘map’ to guide the initiation of positive tipping points, and we should be aware that existing ‘maps’ are likely to have limitations. We define the goal of ‘global sustainability’ as the persistence of all components of the biosphere, including ensuring all humans have a quality of life beyond mere survival (Brown, Hanson, Liverman, & Merideth, Reference Brown, Hanson, Liverman and Merideth1987).
The emerging body of work identifying candidate ‘positive tipping points’ has recently focused on social-technological systems (or ‘socio-technical systems’) (Farmer et al., Reference Farmer, Hepburn, Ives, Hale, Wetzer, Mealy, R., Srivastav and Way2019; Otto et al., Reference Otto, Donges, Cremades, Bhowmik, Hewitt, Lucht, Rockström, Allerberger, McCaffrey, Doe, Lenferna, Morán, van Vuuren and Schellnhuber2020a; Sharpe & Lenton, Reference Sharpe and Lenton2021) – that is, systems comprising interacting social and technological elements. However, earlier work identified multiple positive tipping points in social-ecological systems (Marten, Reference Marten2005) – that is, systems comprising interacting social and ecological/natural elements. By including ecological change, the opportunities for positive tipping are enriched because ecological systems can have their own tipping dynamics (Scheffer, Reference Scheffer2009), and other living things in those systems also have the agency to tip change (Latour & Lenton, Reference Latour and Lenton2019). Transformation towards global sustainability must necessarily occur across coupled social-technological-ecological systems – that is, systems comprising interacting social, technological and ecological elements (Ahlborg, Ruiz-Mercado, Molander, & Masera, Reference Ahlborg, Ruiz-Mercado, Molander and Masera2019) – and these may have novel reinforcing feedbacks and tipping points in their coupled dynamics.
Positive tipping points offer hope for accelerating change to avert climate and ecological emergency, but crucial practical questions are how to identify and trigger them. The emerging literature is rather theoretical, speculative and rarely specific enough to guide actions. Mathematical theory highlights multiple potential tipping mechanisms and associated reinforcing feedbacks in social-technological systems (Zeppini, Frenken, & Kupers, Reference Zeppini, Frenken and Kupers2014), but needs to be translated into specific contexts to guide deliberate tipping. Recent examples of positive tipping points in power generation, personal transport and lighting can provide useful clues to effective action (Kamat, Khosla, & Narayanamurti, Reference Kamat, Khosla and Narayanamurti2020; Sharpe & Lenton, Reference Sharpe and Lenton2021). A much larger set of previous socio-technical transitions can also provide guidance – even if they were not towards a more sustainable state. That is the foundation of ‘Transitions Management’ theory (Rotmans, Kemp, & van Asselt, Reference Rotmans, Kemp and van Asselt2001) and the associated ‘Multi-Level Perspective’ (Geels, Reference Geels2002). It highlights how transformation usually starts in niches before accelerating up an ‘S curve’ trajectory (and eventually saturating as the new normal). However, in being guided by how change has happened in the past it may foreclose the possibility that change can happen differently (and faster) in the future (Sovacool, Reference Sovacool2016). A synthesis of transitions theory and social movement theory may help elucidate how social innovations in niches – ‘free social spaces’ – can sometimes give rise to abrupt social mobilisations and construction of new political processes and structures (Törnberg, Reference Törnberg2018).
Other established frameworks can help operationalise positive tipping points, in particular systems thinking and Donella Meadows' ‘leverage points’ framework of places to intervene in a system (Abson et al., Reference Abson, Fischer, Leventon, Newig, Schomerus, Vilsmaier, von Wehrden, Abernethy, Ives, Jager and Lang2017; Leventon, Abson, & Lang, Reference Leventon, Abson and Lang2021; Meadows, Reference Meadows1999, Reference Meadows2008; Schlaile, Urmetzer, Ehrenberger, & Brewer, Reference Schlaile, Urmetzer, Ehrenberger and Brewer2021). We focus on strengthening reinforcing positive feedbacks as a key leverage point, but recognise that weakening negative feedbacks exerts comparable leverage, and the most powerful leverage points are those that change the intent of a system (Meadows, Reference Meadows1999, Reference Meadows2008). Meadows' conception of ‘intent’, derived from cybernetics, does not imply conscious purpose on the part of a system – but trying to instigate positive tipping points is all about putting some conscious ‘intent’ into social-technological-ecological systems. This needs to be combined with an understanding of complex adaptive systems, but complexity science rarely references leverage points (Holland, Reference Holland2014). The ‘panarchy’ framework begins to bring them together, recognising that in a ‘reorganisation phase’, small (chance) events can have a disproportionate impact in a system, because many system connections were previously broken in a preceding ‘release phase’ – usually as a result of some crisis (Gunderson & Holling, Reference Gunderson and Holling2002).
Our aim here is to start to operationalise positive tipping points by synthesising established theory and lessons from past examples, to offer guidance on finding potential future tipping points and associated interventions and actors that could trigger them, and to frame a further research agenda. The paper is structured as follows: Section 2 synthesises existing theory pertinent to identifying positive tipping points. Section 3 draws out a recipe to begin to operationalise the theory – offering some initial guidelines for those keen to trigger positive tipping points. Section 4 discusses an agenda for further research. Section 5 concludes.
2. Positive tipping points theory
First, we use integrative literature review (Torraco, Reference Torraco2016) to synthesise relevant existing theory and models of tipping points, relating them to some specific examples. After outlining the challenge, we take a particular mathematical entry point to conceptualising positive tipping points (Figure 1), recalling that ‘all models are wrong, but some are useful’ (Box, Reference Box, Launer and Wilkinson1979) and recognising the limitations of our chosen approach.
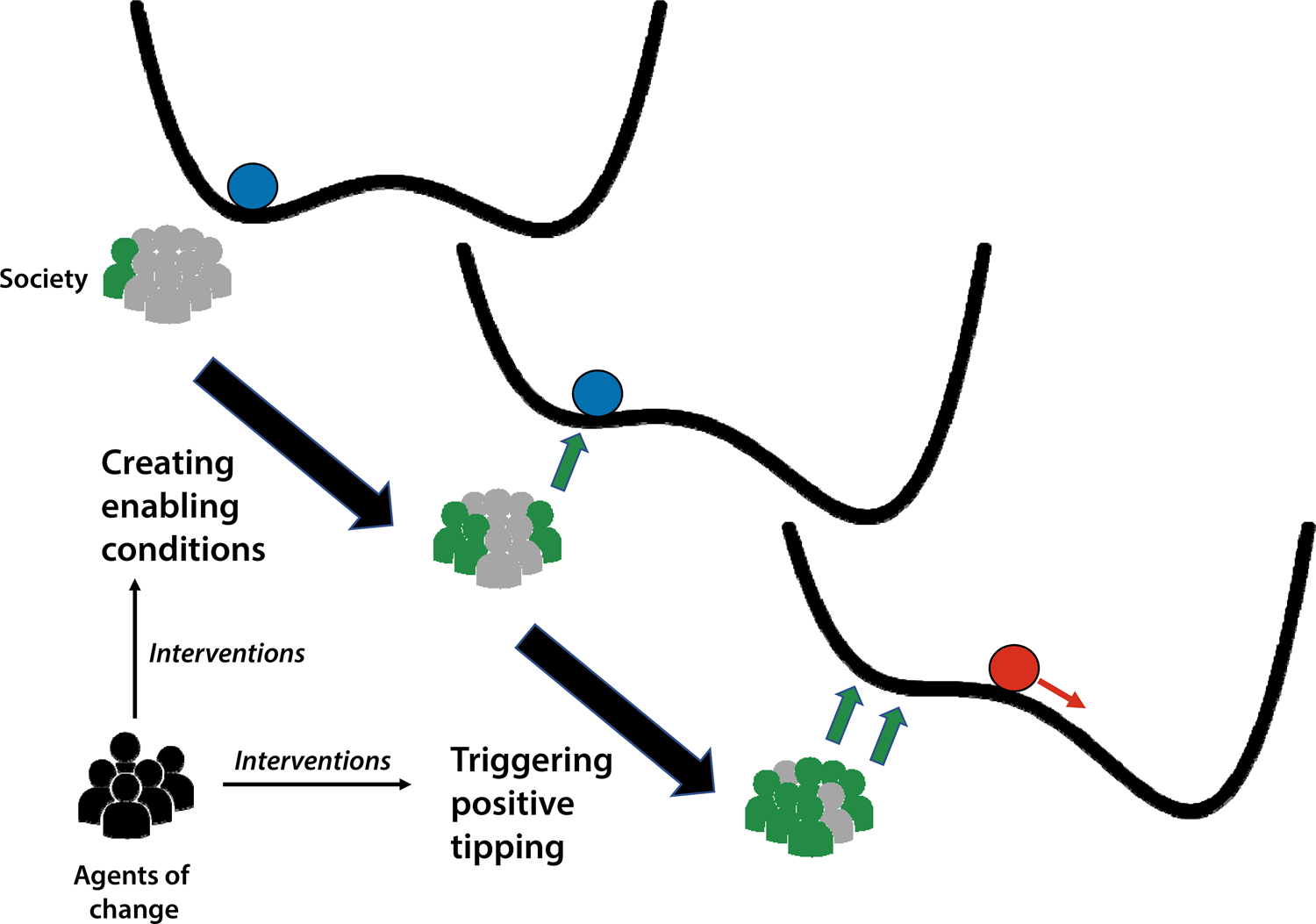
Fig. 1. A dynamical systems conceptualisation of positive tipping points.
2.1 Intent and the need for speed
It would appear critical to global sustainability transformations that some human actors are discontent with the current state of a system and have a vision of a desired state they want to transform towards – such as net zero greenhouse gas emissions. They may also have some specific goals they want to achieve to get towards their vision – such as halving greenhouse gas emissions by 2030 and reaching net zero by 2050. Meanwhile, other actors argue the transformation needs to go even faster than that (Anderson et al., Reference Anderson, Broderick and Stoddard2020). Having a vision of an alternative state and associated goals – especially if they are widely and democratically agreed upon – is a potentially powerful motivation for transformative change (Schot & Steinmueller, Reference Schot and Steinmueller2018). But just wanting change to happen is not sufficient to make it happen – that needs practical action guided by a valid model of how transformative change can occur. This must recognise that there are usually other actors with different intents – including those who oppose change – exercising their agency as well (Smith & Christie, Reference Smith and Christie2021).
The ‘Transitions Management’ literature argues that such systemic change is inherently slow, providing estimates ranging from >20 years for sustainable technology innovations (Gross, Hanna, Gambhir, Heptonstall, & Speirs, Reference Gross, Hanna, Gambhir, Heptonstall and Speirs2018), to ‘one generation or more’ for a socio-technical paradigm shift (Grin, Rotmans, & Schot, Reference Grin, Rotmans and Schot2010). That sounds like a counsel of despair because sustainability transformation must now happen considerably faster than that. However, in past socio-technical transitions, there were things that diverse actors could and did do to accelerate change (Victor, Geels, & Sharpe, Reference Victor, Geels and Sharpe2019) and there are past examples of rapid change (Sovacool, Reference Sovacool2016). Hence their pace was not predetermined. Looking ahead much work has already been done on multiple fronts towards sustainability transformation – but we still need to trigger it! This brings us to an approach to modelling positive tipping points.
2.2 Dynamical systems approach
At a tipping point, a small perturbation can trigger a large response from a system, sending it into a qualitatively different future state (Figure 1). The notion that a system has alternative dynamically stable states (or ‘attractors’) and can transition between them means that it contains both negative and positive feedback loops and their relative strength can change. A predominance of negative feedback creates and maintains a dynamically stable state. At a tipping point, positive (reinforcing) feedbacks get sufficiently strong to (temporarily) dominate the dynamics, propelling self-amplifying change between alternative stable states. Thus, any attempt at deliberate tipping needs to seek to trigger strongly reinforcing positive feedback loops – and it may also seek to weaken negative feedbacks that maintain the incumbent state.
A tipping point is a special ‘place’ or point – we should not expect a system to naturally reside close to one – unless it belongs to a special class of systems that exhibit ‘self-organised criticality’ (Bak, Tang, & Wiesenfeld, Reference Bak, Tang and Wiesenfeld1987). To bring a system to a tipping point typically requires some forcing – that is, a change in boundary conditions – in a direction that weakens balancing negative feedbacks maintaining the initial state and/or strengthens reinforcing positive feedbacks that amplify change (Meadows, Reference Meadows1999, Reference Meadows2008). Hence there is typically an important phase that precedes the ‘triggering’ of a positive tipping point: work first needs to be done to create enabling conditions (Figure 1). Once nearby, a tipping point may be triggered by a further small change in these boundary conditions or by a small change in a relevant feature of the system. Here we distinguish between system features in which a tipping point change may occur, and control variables which can bring a system feature to (and past) a corresponding tipping point (noting that ‘control’ is meant mathematically and does not imply we can control such complex systems).
A key challenge in identifying tipping points is thus to identify the critical control variable(s) and features of a particular system. This may seem daunting because there are usually many variables in a complex system. But crucially, the behaviour of a complex system near a tipping point can simplify dramatically such that often just one control variable dominates the behaviour (in mathematics this is the centre manifold theory (Carr, Reference Carr1982)). That control variable itself may be a mathematical combination of other variables.
Clearly this conceptualisation (Figure 1) is an incomplete representation of complex, co-evolving social-technological-ecological systems. It does not capture their endogenous evolution, and it treats the intentional actions of agents within the system who want to trigger positive change as if they were external forcing factors. Whilst we can talk mathematically about ‘control’ variables, we cannot assume that such highly complex and interconnected systems can be ‘controlled’. Rather they may be ‘influenced’ to a limited extent, within particular (bounded) contexts of action or systems of reference. But it is meant to be viewed as a partial model of the key dynamics of tipping points, which helps agents within the system start to formulate interventions.
2.3 Existing tipping point models
We now turn to identifying different models of tipping points that can occur in social-technological-ecological systems, their critical control variables, and the reinforcing feedback loops that can propel them (Table 1). There are several models, each capturing different yet overlapping aspects of tipping point dynamics. These models are reasonably well known in their respective academic disciplines, but rarely considered together. In Figure 2, we schematically summarise and synthesise several of the different underlying tipping mechanisms, which we describe in the following sub-sections. We start in the social-technological domain, with models of tipping point dynamics in the ‘diffusion’ of new norms, behaviours, and technologies through society – elegantly summarised by Zeppini et al. (Reference Zeppini, Frenken and Kupers2014). Then we move to the social-ecological domain.

Fig. 2. Schematic synthesis of different mechanisms that can underlie positive tipping points across social-technological-ecological systems – explained in text Section 2.3.
Table 1. Relevant tipping point models, building on Zeppini et al. (Reference Zeppini, Frenken and Kupers2014)

2.3.1 Critical mass, diffusion of innovations and social contagion
Several models of innovation diffusion display a tipping point in terms of a critical mass of individuals that once reached can tip most (or all) of the population to adopt. The critical mass is a sufficient number of adopters of a new idea, technology or innovation such that the rate of adoption becomes self-sustaining and creates further growth. The population denoted in Figure 2 are shown at critical mass (the vertical dashed line) with those who have adopted shaded dark and those who have yet to adopt unshaded. The concept of a critical mass is central to Diffusion of Innovations theory (Rogers, Reference Rogers1962), which crystallised from studies of the spread of agricultural technologies in the United States in the 1920s and 1930s. It characterises the uptake of innovations as an ‘S-curve’ and classifies human populations into successive fractions defined in terms of their propensity to adopt innovations (Table 2). New ideas, products or behaviours start with innovators, then early adopters, followed by an early majority, then a late majority and finally the laggards. Along this trajectory, the products mature, and their functionality improves as a result. In Figure 2, we show the population (middle panel) and technology (bottom panel) following S-curves of adoption.
Table 2. Diffusion of innovation theory classification of populations, following Rogers (Reference Rogers1962)

The original mathematical formulation of a critical mass tipping point was in a model of metropolitan segregation (Schelling, Reference Schelling1971).Footnote 1 It was later generalised to a wide range of social phenomena (Granovetter, Reference Granovetter1978; Schelling, Reference Schelling1978). The underlying positive feedback of social contagion occurs when adoption of a norm or behaviour makes it easier for the next person to adopt it, through imitation (Granovetter, Reference Granovetter1978). In Figure 2, this mechanism of contagion is shown with small arrows from one adopter to multiple others. Information, like diseases, can spread through simple contagion, only requiring contact with one other person to spread, whereas change of behavioural norms typically occurs through complex contagion requiring contact with multiple people (Centola & Macy, Reference Centola and Macy2007). In Granovetter's (Reference Granovetter1978) model, people are assumed to be heterogeneous with different individual thresholds for adoption of a new norm/behaviour (above which it is beneficial to them), and that threshold depends on how many others have adopted – that is, people vary in the extent to which they are influenced by others. (In Figure 2, we denote the movable threshold for one individual who has just adopted.) Adoption does not depend on any qualities of the norm/behaviour. The model can produce cascades within a population, including where one person adopting is sufficient to trigger the whole population to ultimately adopt, but just a small variation in another individual's threshold can prevent this (Granovetter, Reference Granovetter1978). Variants of the model consider no preferential influence, or social structure where friends have a higher influence on individual decisions (Granovetter, Reference Granovetter1978). The critical mass tipping point for complex social contagion depends on the distribution of individual thresholds for adoption and on social network structure (Centola & Macy, Reference Centola and Macy2007; Centola, Becker, Brackbill, & Baronchelli, Reference Centola, Becker, Brackbill and Baronchelli2018). Social contagion underlies accelerating adoption of some sustainable behaviours – for example, the uptake of rooftop solar photovoltaic (PV) systems (Graziano & Gillingham, Reference Graziano and Gillingham2014) and other household energy behaviours (Wolske, Gillingham, & Schultz, Reference Wolske, Gillingham and Schultz2020).
Most of us require a sense of self-efficacy (ability to deal individually with a problem) to adopt pro-environmental behaviour, and a sense of collective efficacy (ability as a group to deal with a problem) can reinforce self-efficacy (Jugert et al., Reference Jugert, Greenaway, Barth, Büchner, Eisentraut and Fritsche2016). Values endorsed by our peers can be more important than facts or ‘experts’ in changing our beliefs and actions. Once a critical mass tipping point is reached, pressure to conform to a collective identity can propel social contagion. Controls on the steepness of the S-curve have been widely studied, for example, in marketing science, and support social contagion as one mechanism (van den Bulte & Stremersch, Reference van den Bulte and Stremersch2004). Specifically, greater income inequality, and cultural factors including greater collectivism, power distance and masculine values can steepen the S-curve,Footnote 2 meaning change starts later but happens faster once underway (van den Bulte & Stremersch, Reference van den Bulte and Stremersch2004). However, competing technological standards are more powerful than cultural or economic effects in deterring early adoption but supporting faster change once underway (van den Bulte & Stremersch, Reference van den Bulte and Stremersch2004). This is consistent with the qualities of norms/behaviours/technologies affecting adoption. This leads to other models with a critical mass tipping point that hinge on different reinforcing feedbacks (Zeppini et al., Reference Zeppini, Frenken and Kupers2014), to which we now turn.
2.3.2 Increasing returns, coordination and herding
The positive feedback of increasing returns to adoption occurs when a behaviour or technology becomes more attractive (in quality or price) the more fellow users have already adopted it (Arthur, Reference Arthur1989). Several important positive feedbacks can underlie increasing returns, including learning by doing – the more something is done/made, the better it can be done/made (Arrow, Reference Arrow1962); economies of scale – the more something is done/made, the more efficiently/cheaply it can be done/made (Bejan, Almerbati, & Lorente, Reference Bejan, Almerbati and Lorente2017); and technological reinforcement – the more something is used, the more technologies emerge that make it more useful. In Figure 2, we denote the process of learning by doing in both technology developers (bottom panel) and the population of early adopters (middle panel) as an accumulation of knowledge about a new option in their minds as adoption proceeds – recognising that early adopters serve as early testers, providing feedback and helping in developing a solution. We denote economies of scale as a declining S-curve of price (bottom panel), mirroring the rising S-curves of adoption (middle panel) and quality/performance (bottom panel). We denote technological reinforcement as the cogs of core and supporting technologies coming together up the S-curve (bottom panel). A critical mass of adopters of a new technology can trigger a tipping point where increasing returns and associated adoption becomes self-propelling (middle panel). That critical mass depends on any difference in quality to the incumbent technology and the strength of increasing returns (Zeppini et al., Reference Zeppini, Frenken and Kupers2014). Striking examples of increasing returns in sustainable technology uptake are the declining price of renewable energy with increasing deployment (Green, Reference Green2019; Kavlak, McNerney, & Trancik, Reference Kavlak, McNerney and Trancik2018), and of the batteries used in electric vehicles (EVs) (Nykvist & Nilsson, Reference Nykvist and Nilsson2015). An increasing returns tipping point can be affected by technology developers, governments supporting them and consumers acting as the critical mass.
Technological reinforcement can also lead to a distinct critical mass coordination tipping point. This can occur thanks to the network effect whereby different members of a population must coordinate on a new technology suite to get a superior payoff from it than an existing technology suite. Think of EVs and charging points compared to petrol/diesel cars and refuelling garages. The ‘players’ in such a ‘game’ could be technology developers, deployers or users. Accordingly, in Figure 2, we denote coordination as something spanning and connecting the realms of society and technology (as both people and technologies must be coordinated). The critical mass of coordinators required depends on the benefit and cost of the new technology relative to the payoff for coordinating on an old technology suite (Zeppini et al., Reference Zeppini, Frenken and Kupers2014), where the benefits and costs of a new technology may be subject to positive feedbacks of increasing returns. A committed minority of ‘zealots’ can tip a contagious spread of cooperation (Masuda, Reference Masuda2012), which may also be sensitive to social network structure (Cardillo & Masuda, Reference Cardillo and Masuda2020). A coordination tipping point can be affected by firms coordinating, government helping them coordinate and policy incentives altering the payoffs (e.g. by altering subsidy regimes). Also, if governments coordinate internationally to align their policies in support of the same set of technologies, this strengthens increasing returns.
A different type of critical mass tipping point occurs with informational cascades leading to herding behaviour (Banerjee, Reference Banerjee1992; Bikhchandani, Hirshleifer, & Welch, Reference Bikhchandani, Hirshleifer and Welch1992). Here positive feedback can occur when each potential adopter of a behaviour forms an expectation (of the payoff) based on the decisions of previous adopters. It can be rational for an individual to behave (e.g. adopt a new technology) based purely on the behaviour of those before them, disregarding their own information. We denote this in Figure 2 (middle panel) as some of those about to adopt looking at and being influenced by those who have just adopted. The strength of the positive feedback decreases with adoption. Hence herding cascades can be both initiated by information and stopped by new public information (Bikhchandani et al., Reference Bikhchandani, Hirshleifer and Welch1992). This suggests the creators (e.g. researchers) and broadcasters (e.g. media) of new public information have the agency to change the tipping dynamics. There is evidence of informational cascades in the adoption of organic farming practices (Chatzimichael, Genius, & Tzouvelekas, Reference Chatzimichael, Genius and Tzouvelekas2014).
A crucial question is in which fraction of the population is critical mass reached (Figure 2, middle panel)? Clearly, it depends on the model and various contextual factors highlighted. It can be just one person (Granovetter, Reference Granovetter1978), but typically it is in the range 10–40% of the population (Rogers, Reference Rogers1962), and for complex contagion ~25% of a population can tip a change in social convention (Centola et al., Reference Centola, Becker, Brackbill and Baronchelli2018; Rogers, Reference Rogers1962). Thus, it could lie within the innovators, the early adopters, or the early majority (Table 2). Importantly, early adopters ‘help’ in developing a solution, buying into the promise, serving as early testers, and providing feedback. In contrast, the early majority typically need evidence of an innovation's worth to adopt. Thus, new behaviour/technology itself changes as it finds more adoption.
2.3.3 Percolation, co-evolution and missing models
Other models of social-technological tipping points are not defined in terms of critical mass.
The spread of new norms or behaviours by information transfer through social networks can lead to a percolation threshold if people vary in their willingness to adopt (Solomon, Weisbuch, de Arcangelis, Jan, & Stauffer, Reference Solomon, Weisbuch, de Arcangelis, Jan and Stauffer2000). Past such a tipping point, an innovation can diffuse throughout a networked population, whereas below it cannot (Zeppini et al., Reference Zeppini, Frenken and Kupers2014). The percolation threshold can depend on the cost of adoption, the number of starting seeds from which the innovation spreads, and the network connectivity. Any of us can be starting seeds for the spread of innovation and thus affect the number of those. Network connectivity is itself endogenously evolving with the Internet and social media. The critical level of cost can depend on other reinforcing feedbacks of, for example, increasing returns.
In the development of interdependent technologies, there can also be co-evolution tipping points between different stable states in the ‘fitness landscape’ of a suite of coupled technologies. In this model, the fitness of a specific technology depends on the design state of other coupled technologies (Kauffman & Johnsen, Reference Kauffman and Johnsen1991; Kauffman & Macready, Reference Kauffman and Macready1995). For example, the fitness of wind and solar power depends on the design state of energy storage and grid technologies. The model system readily gets locked into local fitness maxima but changes in governance can trigger tipping points to higher fitness maxima. Importantly, neither top-down or more localised governance interventions are universally more effective, outcomes are path-dependent (Zeppini et al., Reference Zeppini, Frenken and Kupers2014).
Some observed social-technological tipping points are challenging to interpret through any of the above models, suggesting additional models may need to be developed. For example, a recent coal to gas tipping point in the UK power sector hinged on a change in their relative cost for power generation, which triggered withdrawal of investment from coal, and ultimately destruction of coal power stations (Sharpe & Lenton, Reference Sharpe and Lenton2021).
2.3.4 Tipping mechanisms involving ecology
Reinforcing feedbacks and co-evolution are of course not limited to the social-technological realm – they have a much longer history in the ecological realm. Ecosystems contain diverse positive feedbacks some of which can get strong enough to generate tipping points, for example, in coral reefs, rangelands and lakes (Scheffer, Carpenter, Foley, Folke, & Walker, Reference Scheffer, Carpenter, Foley, Folke and Walker2001). These are often called ‘regime shifts’ and there is a database of numerous examples (Biggs, Peterson, & Rocha, Reference Biggs, Peterson and Rocha2018). There are also many models of specific ecosystems and their specific tipping points. Most of the literature focuses on negative tipping points triggered by human activities, for example, lake eutrophication, but positive feedback can always operate in either direction. Positive tipping points towards preferred ecological states have been achieved, for example, restoring lakes to a clear water state. Notably, however, the positive tipping point for recovery usually requires a much larger change in a critical control variable than what caused the original, negative tipping point. This is because they are different tipping points that bound a region of ‘bi-stability’ where two alternative states are stable.
There are also social-ecological positive feedbacks – reinforcing interactions between changes in society and ecology (Marten, Reference Marten2005), sometimes described as ‘virtuous cycles’ (Morrison, Reference Morrison2016; Selman & Knight, Reference Selman and Knight2006). For example, marine-protected areas rejuvenate fisheries causing people to create additional marine-protected areas (Mascia & Mills, Reference Mascia and Mills2018). Environmental stewardship improves reef ecology, enhancing social values and motivating further stewardship (Turnbull, Clark, & Johnston, Reference Turnbull, Clark and Johnston2021). If positive feedback is strong enough, this can generate tipping points that are not present in either system alone. For example, a modelled tipping point between a state of over-exploitation of a common-pool resource (such as fish stocks) without human cooperation, and avoidance of over-exploitation through a cooperative social norm and social ostracism of non-complying harvesters (Lade, Tavoni, Levin, & Schlüter, Reference Lade, Tavoni, Levin and Schlüter2013). In Figure 2 (upper panel), we capture positive tipping to a preferred social-ecological state as going up an S-curve, involving learning by doing, into a state in which humans and other species have positively reinforcing interactions. We denote coordination spanning and connecting the realms of society and ecology, because people must coordinate with each other and must attune with other species in this form of positive tipping. Other generic models consider how tipping points in both social and ecological systems that are coupled interact (Mathias et al., Reference Mathias, Anderies, Baggio, Hodbod, Huet, Janssen, Milkoreit and Schoon2020), or how co-evolving environmental and strategy dynamics in the presence of an ecological tipping point can sometimes lead to persistent oscillations (Tilman, Plotkin, & Akçay, Reference Tilman, Plotkin and Akçay2020; Weitz, Eksin, Paarporn, Brown, & Ratcliff, Reference Weitz, Eksin, Paarporn, Brown and Ratcliff2016).
Finally, there must be positive feedbacks where reinforcing changes in society, technology and ecology are all coupled together (hinted at by the interconnected nature of Figure 2). However, there is relatively little literature on social-technological-ecological systems (Ahlborg et al., Reference Ahlborg, Ruiz-Mercado, Molander and Masera2019) and hence a shortage of models of associated tipping points. We suggest the ‘green revolution’ would make an instructive historical case study where increasing population, food production and proliferation of new technologies all fed back to amplify each other. So too would contemporary initiatives co-opting ‘ecosystem engineers’ and utilising technology to trigger positive tipping in ecosystems harvested for food – such as blue mussel beds (Schotanus et al., Reference Schotanus, Walles, Capelle, van Belzen, van de Koppel and Bouma2020).
2.4 Upward-scaling tipping cascades
Sometimes, in interconnected complex systems, the activation of one tipping point can increase the likelihood of triggering another at a larger scale, and so on (Lenton, Reference Lenton2020). Such an ‘upward scaling tipping cascade’ can progress in time (towards a greater degree of permanence), in space (expanding to affect a larger geographical area) or across system boundaries (e.g. from a product, to an economic sector, to an economy of many sectors) (Sharpe & Lenton, Reference Sharpe and Lenton2021). This can result in very large-scale, rapid change (Lenton, Reference Lenton2020; Sharpe & Lenton, Reference Sharpe and Lenton2021).
Current theory and models of upward-scaling tipping cascades are quite abstract and more focused on avoiding damaging cascades (Dekker, von der Heydt, & Dijkstra, Reference Dekker, von der Heydt and Dijkstra2018; Klose, Karle, Winkelmann, & Donges, Reference Klose, Karle, Winkelmann and Donges2020). Nevertheless, experience provides some clues as to the type of intervention that can trigger positive cascades. In ecosystems, the reintroduction of a single ‘keystone species’ can trigger a positive upward-scaling ‘trophic cascade’ – a famous example being the reintroduction of wolves in Yellowstone national park (Ripple & Beschta, Reference Ripple and Beschta2012). Several past ‘socio-technical transitions’ started with disruptive technological innovations in niches that cascaded upwards through tipping points to society-wide change (Smith, Stirling, & Berkhout, Reference Smith, Stirling and Berkhout2005). The EV revolution appears to be following this pattern: Norway's tipping point to EVs dominating new car sales was initially reversible without the maintaining of policy incentives, but is now becoming irreversible, spreading across Europe and worldwide, and starting to reinforce the transition to renewable energy through availability of cheap battery storage (Sharpe & Lenton, Reference Sharpe and Lenton2021).
3. Operationalising positive tipping points
Having reviewed and synthesised available models (Figure 2), we now outline a research agenda to operationalise positive tipping points (Figure 3). Crucial determinants of whether positive tipping can occur are the sensitivity of a system to being tipped, and the leverage of different actors and actions to bring about tipping. We conceptualise operationalising positive tipping points in three phases of action: creating enabling conditions; sensing the potential for positive tipping; and triggering positive tipping points. In each phase, changes already underway may be harnessed towards positive outcomes, and learning will be crucial to success. First, we draw out some initial guidelines on the enabling conditions for positive tipping points. Second, we consider how the potential for positive tipping can be sensed using models and data. Third, we offer some initial guidelines on who can trigger positive tipping and how.

Fig. 3. Summary of framework for triggering positive tipping points.
3.1 Identifying and creating enabling conditions for positive tipping points
Recall the distinction between system features in which a tipping point change may occur, and control variables which may bring a system feature to (and past) a tipping point. The systems features are the ‘what’ that tips, whilst the control variables help identify the ‘how’ of tipping. For example, the number of adopters is a system feature, and at a critical mass tipping point one more adopter will trigger a self-propelling cascade of further adoption. However, to create enabling conditions, the focus should be on identifying and affecting the control variables (the ‘how’) that can bring a system to a tipping point. In the example, those are the factors affecting adoption and altering the value of the critical mass.
From the general models (Table 1, Figure 2) and from past examples, we have identified some key enabling conditions that may control positive tipping, depending on the context (Figure 3). We have sought to describe these in terms understandable to a wide range of practitioners. We offer them as a non-exhaustive list, proceeding from social to more social-technological variables, noting that ecological variables that can enable positive tipping tend to be context-specific (e.g. reducing nutrient loading of a eutrophic lake or reducing harvesting intensity of a fish stock).
3.1.1 Population size
Where individual thresholds are homogeneous (as in increasing returns and coordination models), more people are needed to reach a critical mass tipping point in a larger population. Conversely, where individual thresholds are heterogeneous (as in social contagion or informational cascades), larger populations can have a greater chance of some small subset group passing a tipping point (Granovetter, Reference Granovetter1978). For example, social contagion of rooftop solar PV uptake has been observed to start in sub-populations. More generally, a ‘sweet spot’ of population size for triggering action to scale Project Drawdown's climate solutions has been identified at the community scale (~10,000 people) (Bhowmik, McCaffrey, Ruskey, Frischmann, & Gaffney, Reference Bhowmik, McCaffrey, Ruskey, Frischmann and Gaffney2020). This suggests targeting smaller/subset populations as a strategy to enable tipping, for them at least – but it then depends on the existence of an upward-scaling tipping cascade for that to escalate towards global change.
3.1.2 Social network structure
Social network structure can affect the tipping points for social contagion, informational cascades, percolation and co-evolution. For example, greater friendship between people can enable social contagion (Granovetter, Reference Granovetter1978). Weak ties across a social network can promote simple contagion but inhibit complex contagion (Centola & Macy, Reference Centola and Macy2007). Complex contagion, for example, of social movements, benefits from clusters of strong local ties, hence efforts to trigger it could target tightly knit residential networks (Centola & Macy, Reference Centola and Macy2007) and committed minorities (Centola et al., Reference Centola, Becker, Brackbill and Baronchelli2018). Bottom-up social movements typically gestate in ‘free social spaces’ which protect them from mainstream society and generate new social networks that can be drawn upon to promote collective action (Törnberg, Reference Törnberg2018). Hence supporting a diversity of such spaces within society should increase the potential for positive tipping. Clearly, context matters, but recognising the nature of a contagion phenomenon can help to draw out general rules.
3.1.3 Information/capability
Adopters need the right information to use an alternative, or act on a behaviour. Increased exposure to information can enable social contagion (Hodas & Lerman, Reference Hodas and Lerman2014), and public information can initiate or stop an informational cascade (Bikhchandani et al., Reference Bikhchandani, Hirshleifer and Welch1992). For example, the ‘TIST’ smallholder tree planting schemeFootnote 3 has spread to over 100,000 farmers in 15 years because it was designed to facilitate information sharing in a scalable way, while providing smallholder farmers with the capability to access international voluntary carbon markets. Clearly, context defines what information is key, so it is hard to draw out general rules.
3.1.4 Price
A competitive price signals to consumers the availability of a plausible alternative to an incumbent technology while stimulating demand. A critical price can enable or prevent a percolation tipping point. Equally, critical mass tipping points involving increasing returns and/or coordination can be influenced by price. For example, across Europe, where electric cars have reached purchase price parity with equivalent petrol/diesel cars – notably in Norway – this leads to a highly non-linear increase in market share (Sharpe & Lenton, Reference Sharpe and Lenton2021) (despite EVs being considerably cheaper to use). Thus, interventions reducing price can help bring a technology alternative to a positive tipping point.
3.1.5 Performance/quality
When an alternative has equivalent or better quality/performance than an incumbent technology this can attract demand. Difference in quality affects the critical mass at which increasing returns on adoption reaches a tipping point (as does benefit from a new technology in a coordination game). For example, EV access to bus lanes in Norway markedly cut urban school/work commuting times helping propel EV uptake (Figenbaum, Reference Figenbaum, Contestabile, Tal and Turrentine2020). EVs also typically have superior acceleration to ICE vehicles, and differences in range are dwindling rapidly. Another example is accelerating uptake of plant-based meat alternatives – for example, the Beyond Meat and Impossible burgers – that mimic the taste and texture (quality), and experience (performance) of a beef burger. Thus, interventions that improve quality/performance of an alternative can enable positive tipping.
3.1.6 Desirability/symbolism
A new alternative needs to be desirable to potential adopters, sometimes irrespective of price or performance, and may provide social distinction to owners – thus acting as a ‘positional good’ (valued because of its limited supply) (Hirsch, Reference Hirsch1976). Willingness to adopt is crucial to allowing percolation through a population. There can be strong cultural attachment to an incumbent behaviour that makes it hard to give up. For example, meat consumption is heavily ingrained in tradition in some cultures – for example, Argentina, France – such that even if plant-based alternatives are cheaper and taste as good, uptake may be resisted. Conversely, sometimes, using a minority technology positively signals being different from the majority and this can overwhelm concerns about being left with a technological orphan of little functional value (van den Bulte & Stremersch, Reference van den Bulte and Stremersch2004). This can be crucial for early adopters, although the effect clearly weakens if the product takes off. Thus, interventions that make an alternative more desirable can help enable positive tipping.
3.1.7 Accessibility/convenience
Adoption of a new behaviour or sustainable product benefits from being convenient to access. Agent-based modelling suggests that pro-environmental behaviours are limited by having access to sufficient opportunities for behaving sustainably and emerge systemically as a product of not only infrastructural, social, but also individual factors (Kaaronen & Strelkovskii, Reference Kaaronen and Strelkovskii2020). For example, food choices are typically based on convenience and access. Meat is often given preferential shelf space in supermarkets, listed at the top of menus, and provided as default in catering facilities, whereas alternative proteins are often placed in the back of supermarkets, hidden on menus, and only provided on special request. Increasing accessibility can enable positive tipping.
3.1.8 Complementarity
A new suite of strongly reinforcing technologies that are well coordinated can more readily reach a critical mass where they displace an old technology suite. Conversely, a go-alone strategy often fails when complementary innovations by other parties fail to occur. A positive example is the complementary development of the EV market and the charging network in Norway (Figenbaum, Reference Figenbaum, Contestabile, Tal and Turrentine2020). Coordinating complementary technologies can enable positive tipping.
Some innovations may change the structure of a system, not just the value of control parameters. For example, social network structure can be altered by changes in information technology, notably social media. By introducing new feedbacks this can alter the existence of tipping points, as well as proximity to them. For example, the climate protest movements appear to have reached a critical mass partly enabled by social media (Otto et al., Reference Otto, Donges, Cremades, Bhowmik, Hewitt, Lucht, Rockström, Allerberger, McCaffrey, Doe, Lenferna, Morán, van Vuuren and Schellnhuber2020a). Technically, evolving structural change goes beyond the simple dynamical systems approach adopted above (Figure 1), but it is recognised in the leverage points framework.
3.2 Sensing the potential for positive tipping
Sensing the potential for positive tipping of some specific system is likely to involve some combination of data and modelling. For the result to translate into action, those intent on triggering positive tipping ideally need to be participants in the sensing activity. This invites the formulation of a general co-design methodology that can be applied to specific cases.
When faced with a specific system and problem, one approach is to bring stakeholders together through workshops to identify potential tipping points, as done for, for example, the Jordan River region (Rodriguez Lopez et al., Reference Rodriguez Lopez, Tielbörger, Claus, Fröhlich, Gramberger and Scheffran2019). This process can be aided by developing a system map, which involves drawing the interconnections and feedback loops in a system, and comparing the result to known system ‘archetypes’ (Stroh, Reference Stroh2015). Here this means looking for strong reinforcing feedback loops and the controls on them. We have been co-creating such a systems map in the lead author's home city, utilising the ‘kumu’ software platform, as part of initiating an ‘Exeter living laboratory’.Footnote 4 This is the start of developing a participatory model of a specific system. Additional exploration of different visions of a city's future through software such as ‘Visionmaker’Footnote 5 could help converge on a shared vision for positive tipping. Participatory methods such as citizens' assemblies and juries could both help sense the potential for positive tipping and reach resolutions for action to try and trigger it. Once multiple examples are available for different systems then one can look for (and learn from) examples of comparable systems elsewhere that have tipped (Sharpe & Lenton, Reference Sharpe and Lenton2021).
The next step may be to formalise a mathematical model of a specific system, as a tool for experimentation and learning. Where a comparable tipping point has happened elsewhere, one could try and fit a general model (Table 1) to that specific experience and hope it is translatable (Granovetter, Reference Granovetter1978). For social contagion, one can retrospectively look at the distribution of individual thresholds within a population that has tipped. Then accounting for some uncertainty and variability, one can explore the range of possible outcomes for comparable populations. A complementary approach is to undertake controlled experiments to predict social tipping points of norm change (Andreoni, Nikiforakis, & Siegenthaler, Reference Andreoni, Nikiforakis and Siegenthaler2021).
Even where a relevant experience of tipping is missing, one may compare a system map with the general models (Table 1) and adopt relevant core mechanism(s) as the basis of a specific model. Such models have been developed to examine specific opportunities for positive tipping. Some operate in an experimental mode where uncertain parameters are varied over plausible ranges to explore how they affect the outcome. For example, a global model of dietary change that couples psychological theories of behaviour change to their land use consequences reveals the conditions for a self-amplifying shift to sustainable diets (Eker, Reese, & Obersteiner, Reference Eker, Reese and Obersteiner2019). Or an agent-based model that reveals the degree of intervention needed for positive tipping to cooperation over groundwater management in different national contexts (Castilla-Rho, Rojas, Andersen, Holley, & Mariethoz, Reference Castilla-Rho, Rojas, Andersen, Holley and Mariethoz2017). More specific models have examined, for example, the role of reinforcing feedbacks in achieving a green growth trajectory for the EU (Tàbara et al., Reference Tàbara, Mangalagiu, Kupers, Jaeger, Mandel and Paroussos2013), the conditions for a UK tipping point in EV uptake (Shepherd, Bonsall, & Harrison, Reference Shepherd, Bonsall and Harrison2012), or city scale reinforcing feedbacks between solar PV power and EVs (Shepero, Munkhammar, Widén, Bishop, & Boström, Reference Shepero, Munkhammar, Widén, Bishop and Boström2018). The challenge is that more detailed models need more data to constrain them and risk overfitting.
We may not understand a complex system well enough to build a specific model of it or have enough data to calibrate a detailed model. However, we can still sense something about the proximity of a system to a tipping point directly from observations of that system because different complex systems approaching tipping points show common dynamical behaviour. Specifically, their recovery from perturbations slows down – or in ecologists' terms, they lose resilience. This is because the negative feedbacks maintaining the stability of an incumbent state are getting weaker before a tipping point is reached where positive feedback takes over (and the system never recovers from perturbation). This ‘critical slowing down’ behaviour can be detected if a system is subject to known perturbations and we can measure its response, or if a system is subject to continual variability it shows up in changes in the statistical properties describing the system's response – notably, rising temporal autocorrelation and variance (Held & Kleinen, Reference Held and Kleinen2004; Lenton et al., Reference Lenton, Held, Kriegler, Hall, Lucht, Rahmstorf and Schellnhuber2008; Scheffer et al., Reference Scheffer, Bacompte, Brock, Brovkin, Carpenter, Dakos, Held, van Nes, Rietkerk and Sugihara2009).
This approach has been successfully tested on a range of climate and ecological systems, to reveal whether they are heading towards (or away from) a tipping point (Lenton et al., Reference Lenton, Held, Kriegler, Hall, Lucht, Rahmstorf and Schellnhuber2008; Scheffer et al., Reference Scheffer, Bacompte, Brock, Brovkin, Carpenter, Dakos, Held, van Nes, Rietkerk and Sugihara2009). Rarely is it translated into a statistical forecast of likelihood/ease of crossing a tipping point, but that can be done (Held & Kleinen, Reference Held and Kleinen2004). Where the approach needs more testing is in the social realm. Tantalising studies suggest early warning signals before past stock market bubbles (Diks, Hommes, & Wang, Reference Diks, Hommes and Wang2019) or electricity grid blackouts (Ren & Watts, Reference Ren and Watts2015). A hypothesis that could be tested by analysing social media data is whether spikes of collective attention on new technologies and associated products, for example, EVs or alternative proteins, are getting more resilient (i.e. decaying more slowly) over time. Equally, the potential for tipping public values, attitudes and opinions may be sensed by looking at the strength of sentiment and its decay rate for particular topics in social media. This might be further explored by the deliberate experimental introduction of social media messages. Whilst this approach may tell us something about when a system is more amenable to being positively tipped, we need understanding of that specific system to tell us anything about how to intervene.
3.3 Who and how to tip positive change?
What actions, by whom can create different types of positive tipping? Actions that trigger a tipping point, by definition, can be very small right at the tipping point, but variable amounts of effort may be needed to create the enabling conditions (Section 3.1) and to sense the potential for positive tipping (Section 3.2).
3.3.1 Who?
We should all feel a sense of agency and autonomy to be part of tipping positive change – and this includes collective and strategic agency as well as individual and everyday agency (Otto et al., Reference Otto, Wiedermann, Cremades, Donges, Auer and Lucht2020b). Indeed, reinforcing feedbacks can overturn conventional notions of who has power – witness the school strike movement. That said, different actors (and coalitions of actors) have differing power to affect change in different contexts. It is hard to resist dividing this into ‘top-down’ and ‘bottom-up’ actors and actions, but that presupposes a questionable model of society. Instead an actor-network view seems apt (Latour, Reference Latour2005).
Previous studies have catalogued the actors and coalitions that can be involved in sustainability transitions (Farla, Markard, Raven, & Coenen, Reference Farla, Markard, Raven and Coenen2012; Smith & Christie, Reference Smith and Christie2021), albeit without a specific focus on triggering tipping points. To give a (non-exhaustive) indication: Policymakers and public authorities are a major focus given their role in setting and enacting societal rules. Financial actors have considerable leverage to change the global economy. The third sector (e.g. NGOs) can hold them all to account. Citizens forming social movements can trigger positive tipping points and start upward-scaling tipping cascades (Smith, Christie, & Willis, Reference Smith, Christie and Willis2020). Researchers and technological innovators are the creators of novel alternatives and entrepreneurs can help propel their upscaling. Citizens are key to the uptake of alternative behaviours and products. Firms can actively engage in innovation trajectories and help build an innovation ‘ecosystem’ that learns by doing (Khoo, Reference Khoo and Charter2018). Public sector organisations can provide a protective environment for innovation niches to develop. Marketing can help tip change in public attitudes. Experts and knowledge institutions can provide authoritative information. The media can help communicate it. The faith sector can help tip hearts and minds. Coalitions between different types of actor – united by common interests, ideas or values – can be key to triggering sustainability transitions, because rarely does a single actor have the necessary resources and usually there are other actors opposing transformation (Roberts et al., Reference Roberts, Geels, Lockwood, Newell, Schmitz, Turnheim and Jordan2018). A historical example is the coalition of doctors and engineers that helped trigger the transition in wastewater management from cesspools to sewers in the Netherlands (Geels, Reference Geels2006). More recently, a coalition from academia, NGOs and local constituencies started a ‘new water culture’ movement that triggered policy change in Spain's Ebro river basin (Tàbara & Ilhan, Reference Tàbara and Ilhan2008).
3.3.2 How?
Tipping points can be triggered by different types of intervention from different actors and coalitions. Section 3.1 suggested a broadly defined set of actions to create enabling conditions: target smaller populations, reduce price, improve quality/performance, increase desirability, increase accessibility, coordinate complementary technologies, provide relevant information and alter social network structure. From integrative literature review and past examples, we offer a non-exhaustive list of actions to trigger positive tipping (Figure 3), linked to particular actors and coalitions. We start by considering innovation within the social, technological and ecological realms, and across them (Figure 2), before turning to public and private institutions and capital, and changing information:
Social innovation: Coalitions of committed actors can tip complex social contagion, for example, protest movements that in turn trigger political change (Kuran, Reference Kuran1989; Smith et al., Reference Smith, Christie and Willis2020). Notably, what started with the lone protest of Greta Thunberg outside the Swedish parliament became, within 18 months, a worldwide protest movement mobilising millions of people. Political declarations of a climate emergency followed (although the connection to action is questionable). The movement successfully mobilised the ‘new power’ of online crowd-sourcing, radical transparency and leaderless structures (Heimans & Timms, Reference Heimans and Timms2019).
Technological innovation: Technological innovators can create sustainable alternatives and through learning-by-doing improve their quality/performance, desirability, accessibility and price – all of which may take an innovation past a critical mass tipping point. For example, precision fermentation and mycoprotein technology can use plant protein to create products with the same or similar sensory properties as conventional meat. Technology firms can also coordinate to achieve complementarity of interdependent technologies, potentially triggering coordination and co-evolution tipping points.
Ecological intervention: Human intervention in ecosystems can trigger ecological positive feedback(s) and tipping points in a desired direction – for example, the rapid recovery of a eutrophic lake (Mehner et al., Reference Mehner, Diekmann, Gonsiorczyk, Kasprzak, Koschel, Krienitz, Rumpf, Schulz and Wauer2008). This approach can co-opt other species of ‘ecosystem engineers’ to do the tipping, for example, introducing blue mussels to recover coastal mud flats (Schotanus et al., Reference Schotanus, Walles, Capelle, van Belzen, van de Koppel and Bouma2020). Whilst small in scale, such examples could be emulated worldwide and positive tipping of coastal mud flats, salt marsh and mangrove ecosystems could sequester significant amounts of carbon from the atmosphere.
Social-technological-ecological innovation: Innovations in social-ecological systems (Olsson & Galaz, Reference Olsson, Galaz, Nicholls and Murdock2012) can trigger positive feedback(s) in a desired direction (Marten, Reference Marten2005; Pereira, Drimie, Maciejewski, Tonissen, & Biggs, Reference Pereira, Drimie, Maciejewski, Tonissen and Biggs2020a). For example, feeding schoolchildren in Kenya boosted community agroforestry (Borish, King, & Dewey, Reference Borish, King and Dewey2017). Recreation of earthen dams to trap monsoonal rains in Rajasthan, refilled aquifers, supporting dry-season irrigation, successful cash crop production and community rejuvenation.Footnote 6 Social-technological innovations can also create virtuous cycles. For example, the Net-Works partnership between a business and a charity has created a supply chain that engages coastal communities in the Philippines and Cameroon in collecting and selling discarded fishing nets, which are recycled into nylon yarn for carpet manufacture (Khoo, Reference Khoo and Charter2018). This also benefits ecology because abandoned fishing nets can continue to trap marine life. Such innovations have the potential to trigger positive tipping points.
Policy interventions and public investment: National governments can invest in innovation and R&D, redirect public finance (e.g. subsidies) from incumbents to disruptors, tax unsustainable incumbents, and reconfigure markets and institutions – all of which may help take sustainable innovations past a critical mass tipping point. For example, subsidising renewable energy deployment and introducing a carbon tax specifically on power generation was critical to tipping coal burning out of UK power generation (Sharpe & Lenton, Reference Sharpe and Lenton2021). National governments can also invest in information and communication technologies (ICT) in a way that makes social network structures more amenable to tipping. The Internet began through government-funded research (Leiner et al., Reference Leiner, Cerf, Clark, Kahn, Kleinrock, Lynch, Postel, Roberts and Wolff2009), and ongoing investment in ICT has sometimes had unintended consequences – for example, contributing to the loss of a 40-year monopoly on power in Malaysia (Miner, Reference Miner2015). Multiple governance levels can help coordinate complementary technologies – potentially triggering coordination and co-evolution tipping points. Regional and local government can target smaller populations more amenable to tipping, for example, cities providing free, accessible EV charging infrastructure.
Private investment and markets: Private sector investments tend to come after government investment as an innovation is starting to take off. They can help propel increasing returns and trigger critical mass tipping points – for example, the rapid expansion and declining price of solar PV (Green, Reference Green2019). Withdrawing private finance from unsustainable incumbents, or at least making it harder to access, can also play a critical role.
Public information: Many actors and institutions, including media and government, can generate and spread public information that can trigger social contagion. For example, the BBC ‘Blue Planet II’ series tipped a persistent change in media coverage and political attention on plastic pollution (Males & Van Aelst, Reference Males and Van Aelst2021), although there is little evidence it has led to behaviour change (Dunn, Mills, & Veríssimo, Reference Dunn, Mills and Veríssimo2020). The movement from anonymous global food supply chains to food labelling and certification has triggered a shift to alternative food networks (Pereira et al., Reference Pereira, Drimie, Maciejewski, Tonissen and Biggs2020a). Equally, educational campaigns to expose and counter ‘discourses of climate delay’ could weaken these well-funded and well-organised negative feedbacks that oppose transformational change (Lamb et al., Reference Lamb, Mattioli, Levi, Roberts, Capstick, Creutzig, Minx, Müller-Hansen, Culhane and Steinberger2020).
Behavioural nudges: ‘Behavioural nudges’ – and ‘hyper-nudges’ using big data (Yeung, Reference Yeung2017) – subtly editing the choice architecture for citizen behaviour through suggestions and positive reinforcement can erode an existing norm and may trigger a social contagion tipping point beyond which a new norm takes hold in society (Brescia, Reference Brescia2019; Sunstein, Reference Sunstein2014). For example, smaller plate sizes reduce over-consumption and food loss and waste (Wansink & van Ittersum, Reference Wansink and van Ittersum2013). However, ‘green nudges’ have variable effectiveness (Schubert, Reference Schubert2017), small levies on single-use plastic shopping bags have had variable effects (Nielsen, Holmberg, & Stripple, Reference Nielsen, Holmberg and Stripple2019; Rivers, Shenstone-Harris, & Young, Reference Rivers, Shenstone-Harris and Young2017), and nudging can sometimes trigger shifts in the unintended (less sustainable) direction (Zorell, Reference Zorell2020).
4. Discussion
We have tried to synthesise and operationalise knowledge of positive tipping points, identifying different models and compiling lists of actors and actions – but these should not be treated as independent if we aim to create transformative change across social-technological-ecological systems. A first application of the framework to food and land system transformations is the subject of a separate report (FOLU/GSI, 2021). Looking ahead, the coupled dynamics of social-technological-ecological systems including their potential positive tipping points is a key target for research. So too is considering how intentional change is initiated (Smith et al., Reference Smith, Christie and Willis2020), particularly the role of coalitions of shared interest (Victor et al., Reference Victor, Geels and Sharpe2019), and how different agents and interventions can most effectively work together to create system-wide positive tipping points (Stadelmann-Steffen, Eder, Harring, Spilker, & Katsanidou, Reference Stadelmann-Steffen, Eder, Harring, Spilker and Katsanidou2021). For example, through systems entrepreneurship (Schlaile et al., Reference Schlaile, Urmetzer, Ehrenberger and Brewer2021), policy packaging (Fesenfeld, Wicki, Sun, & Bernauer, Reference Fesenfeld, Wicki, Sun and Bernauer2020) and systems innovation (Hekkert & Negro, Reference Hekkert and Negro2009). Who and how to work together most effectively can depend on the problem and the phase, and the most effective way to unlock system-wide positive tipping may be to intervene in multiple places simultaneously. The ‘Breakthrough Agenda’ launched at COP26 puts such thinking into action with leaders of countries covering 70% of world GDP committing to work together to trigger positive tipping points in each emitting sector.Footnote 7 Research should also consider how we can recombine what we already have in novel ways to trigger positive tipping (rather than necessarily coming up with new things). At a higher-level of synthesis, a transdisciplinary horizon scanning exercise could consider which systems are ready for tipping, which are not but need to be, and what past case studies can tell us about tipping pathways. Some actors are more attuned to the type of thinking outlined herein than others and may be natural instigators (e.g. ‘Extinction Rebellion’ already applied the principles of critical mass in a designed effort to mobilise public protest). Not all aspects of a desired sustainability transformation may be amenable to positive tipping points and it is part of the research agenda to identify these.
Within this more systemic view, it is vital to consider the political economy of positive tipping points. Perceived injustices can be a trigger of tipping. For example, in Chile a small increase in public transport fares in Santiago triggered a social outburst (Estallido Social) ultimately leading to constitutional change. Conversely, any attempt to tip positive change is likely to meet resistance from the incumbent way of doing things. Existing regimes, whether social, technological or ecological, are stabilised by damping feedbacks that resist change and restore the status quo. This can take many forms, including in the social realm, cultural norms, sunk costs, subsidies, ease of raising finance and lobbying groups. We have focused on strengthening positive feedbacks but weakening the negative feedbacks that maintain an incumbent state can be just as powerful as a leverage point (Meadows, Reference Meadows1999, Reference Meadows2008). For example, shifting subsidies from fossil fuel extraction to renewable power, and helping workers, communities and local governments benefit from transformation.
When transformative change takes off, the original incumbent way of doing things must decline – often in an accelerating way propelled by reinforcing feedbacks. There will be losers as well as winners. Hence it is vital to consider whether and how this exacerbates or reduces current inequalities and what social safety nets can help ensure a just transformation (Newell & Mulvaney, Reference Newell and Mulvaney2013; Newell & Simms, Reference Newell and Simms2021; Sovacool, Reference Sovacool2021; Winkler, Reference Winkler2020). Good intentions do not necessarily translate into good outcomes. Apparently well-intentioned rapid transformations can turn out to be immensely exploitative for many stakeholders – in the business realm, think of Uber or AirBnB. Thus, part of the research agenda is to consider; how are people going to game the transformation? Who owns the transformation, who has access to it, and who reaps the benefits of it, will be critical to determining the outcome. Public bodies have a huge role to play in value creation – a much bigger one than big business would like us to believe (Mazzucato, Reference Mazzucato2011). Sadly, public bodies do not generally invest in their own capacities and increasingly outsource everything to companies and consultancies. But when all the knowledge is outside of public bodies who is left in the driver's seat? If it is private institutions without the public good at their heart it could readily lead to negative outcomes.
Given the urgent need for a transformation to global sustainability, not acting is not an option. To help mitigate the risk that well-intended positive tipping points pave a road to hell, the ‘panarchy’ framework emphasises the value of adaptive management as a co-evolutionary process to damp negative outcomes and amplify positive ones (Gunderson & Holling, Reference Gunderson and Holling2002). Key to this approach is the recognition that we can never fully predict the consequence of intervening in a complex adaptive system, but we can experiment and learn by doing, in ‘transformative spaces’ (Pereira et al., Reference Pereira, Frantzeskaki, Hebinck, Charli-Joseph, Drimie, Dyer, Eakin, Galafassi, Karpouzoglou, Marshall, Moore, Olsson, Siqueiros-García, van Zwanenberg and Vervoort2020b) or ‘real world laboratories’ (Schäpke et al., Reference Schäpke, Stelzer, Caniglia, Bergmann, Wanner, Singer-Brodowski, Loorbach, Olsson, Baedeker and Lang2018). This is already occurring widely with cities' experiments to tackle climate change (Castán Broto & Bulkeley, Reference Castán Broto and Bulkeley2013, Reference Castán Broto, Bulkeley, Turnheim, Berkhout and Kivimaa2018), where frontrunners have triggered reinforcing feedbacks across networks of cities (Irvine & Bai, Reference Irvine and Bai2019). This suggests a systemic strategy to initiate positive tipping points in niches using public investment and policy levers, and thus retain an option to reverse the tipping (if it proves undesirable) by rapidly withdrawing those levers.
5. Conclusion
Multiple actors have the agency to contribute together to triggering positive tipping points of sustainability transformation. Multiple types of action can trigger them, and multiple reinforcing feedbacks can propel them. Actions that can create enabling conditions for positive tipping include targeting smaller populations, altering social network structure, providing relevant information, reducing price, improving quality/performance, increasing desirability, increasing accessibility and coordinating complementary technologies. Actions that can trigger positive tipping include social innovation, technological innovation, ecological intervention, social-technological-ecological innovation, policy interventions, public investment, private investment, broadcasting public information and behavioural nudges. This prototype recipe for triggering positive tipping points can help counter widespread feelings of disempowerment in the face of global challenges and help unlock apparently widespread ‘paralysis by complexity’. The proposed initial framework needs testing and refining, especially to create a more systemic recipe for positive tipping, to ensure positive tipping points are aligned with a just transformation, and to link theory of positive tipping points to empirical studies, to enrich both theory and practice. But there is no better way forward than to learn by doing. Continuing to delay action to accelerate a just transformation towards global sustainability will only accentuate the need to find and trigger even more dramatic positive tipping points in the future.
Acknowledgements
We thank Lukas Fesenfeld, Diana Liverman, Paul Lussier, Michael Obersteiner and David Tàbara for stimulating conversations that helped shape our thinking.
Author contributions
T.M.L. conceived and designed the study. T.M.L. wrote the article with input from all co-authors.
Financial support
This work was supported by the Leverhulme Trust (T.M.L., grant number RPG-2018-046); and the Alan Turing Institute (T.M.L., Turing Fellowship).
Conflict of interest
None.
Data availability
The article contains no new data.