INTRODUCTION
Recently we reviewed microbial risk assessment theory application to Pseudomonas aeruginosa swimming pool infection management [Reference Rice1]. Information gaps identified included limited application of dose–response theory to dermal infection in line with previous reviews [Reference Mena, Gerba and Whitacre2, Reference Barna and Kádár3] and lack of a full mechanistic description of infection [Reference Julien4]. In response, we further reviewed pool folliculitis and acute otitis externa aetiology, epidermis ecology, and infection dose–response theory, and constructed a theoretical framework [Reference Roser5]. The next steps we proposed included developing modern dose–response models recognizing the issues raised in [Reference Rice1]. The current paper proposes the first such dose–response algorithms for swimming pool folliculitis, using a ‘meta-analysis’ style approach for interrogating available data and assesses the uses, limitations and implications of the resulting algorithms.
The background context is detailed in [Reference Roser5]. A summary of our rationale is as follows. Increasingly infectious disease management is based on quantitative risk assessment. Central to risk characterization are dose–response algorithms. When combined with exposure assessment information, dose–response algorithms can provide estimates of infection and illness likelihood and disease burden (disability-adjusted life years) for exposed populations. These risk estimates can be used to develop infection management policies, e.g. tolerable microbial contamination of recycled water. This approach has yet to be extended to dermal infections but P. aeruginosa folliculitis provides a good starting place as it is one of the best known opportunistic pathogens and folliculitis outbreaks are common.
To develop dose–response models for ingested pathogens, the current procedure has been to collect infection and attack rate data and collate this with concurrent pathogen density measurements. Data pairs are then fitted to exponential, β-Poisson, hypergeometric and other models [Reference Schmidt6]. Data are obtained mainly from food epidemiology and volunteer and animal trial literature [Reference Huang and Haas7–Reference Crockett9]. By this approach, ingested dose estimates are derived by combining the volume or mass, of water or food consumed, with microbial density estimates.
The first constraint on applying this approach to pool pathogens is that there is no equivalent of water volume consumed. However, our review [Reference Roser5] identified two alternative candidate algorithm forms which relate epidermal infection likelihood and epidermal lesion density to water-borne pathogen density. Both originated in the same research as the widely used exponential ingestion model of Furomoto & Mickey [Reference Furumoto and Mickey10]. Their equations [(4) and (15)] were originally developed for plant epidermises and so seem logical candidates for application to human skin infection. Further, their theoretical analysis appears to have been satisfactorily validated [Reference Furumoto and Mickey11]. The equations are as follows:


where A and B are algorithm constants, C = density of pathogen, N l = lesions per unit area, P inf = probability of infection, and r C is a constant.
A second constraint, was that suitable experimental data for the curve fitting, appeared scant and ambiguous [Reference Roser5]. Some outbreak data were available. However, it was doubtful that P. aeruginosa density/disease likelihood data provided valid matched pairs because of: (i) delays between infection, folliculitis outbreak detection and water testing of several days [Reference Birkhead, Vogt and Hudson12, Reference Hopkins, Abbott and Wallace13]; (ii) pool environment stresses (chlorine dosing, filtration) leading to unknown increases/reductions; and (iii) P. aeruginosa cells potentially being in a viable but non-culturable state in the pool, but able to grow and be pathogenic once in the host [Reference Colwell14]. P. aeruginosa skin inoculation trials [Reference Leyden, Stewart and Kligman15] did not fully satisfy Koch's postulate in that classical folliculitis symptoms could not be induced upon exposure of skin to outbreak isolates and involved different exposure conditions to pools [Reference Roser5]. Animal and human volunteer experiments conceptually offer a solution; however, no P. aeruginosa/folliculitis model was identified. Moreover, it is questionable whether human experiments [Reference Leyden, Stewart and Kligman15, Reference Buck and Cooke16] could now be ethically undertaken because P. aeruginosa causes serious/fatal systemic disease, is notoriously resistant to antibiotics, and high challenge doses are required to induce disease [Reference Mena, Gerba and Whitacre2].
Accordingly, other approaches for estimating P. aeruginosa densities associated with folliculitis need consideration. From re-examining the P. aeruginosa literature we concluded that the required data might be obtained via a meta-analysis style [Reference Kiecolt and Nathan17] literature interrogation which integrated ‘the findings from the universe (or a sample) of investigations of some phenomenon … Meta-analysis involves using published research to compute an overall level of significance, or comparable test statistics'.
Although this approach promised less precise algorithms than have been obtained with enteric pathogens we considered it worth exploring on several grounds. ‘Natural bathing’ in freshwater containing P. aeruginosa and lacking disinfectant is increasingly popular and this bacterium poses a clear risk [Reference Casanovas-Massana and Blanch18]. Price & Ahearn's [Reference Price and Ahearn19] minimum infection concentration (MIC) approach is out-dated [Reference Buchanan20]. Therefore, a reformulation based on more modern dose–response theory is needed. Dose–response algorithms efficiently integrate pathogen density, epidemiology and other dose–response data and generate testable hypotheses about infection risks.
Finally ‘single-hit’ theory based dose–response algorithms could provide reality checks for future mechanism-based dose–response formulations [Reference Roser5] which we suggest might be called second- and third-generation models, respectively (the minimum infective dose concept being first generation). Single-hit theory derived dose–response algorithms [Reference Teunis and Havelaar21] have been central to the revolution in risk assessment-based water and food quality management. Recently their use has stimulated recognition of the need to more deeply understand dose–response mechanisms. This is reflected in the Key Events Dose–Response Framework (KEDRF) analysis [Reference Julien4] which identified six different steps not captured in current model formulation: (i) intake/exposure, (ii) biological interaction/process, (iii) interaction/process (transport/distribution/excretion), (iv) interaction/process (metabolism), (v) target issue interaction, and (vi) ultimate effect. Separately, Teunis & Havelaar [Reference Teunis and Havelaar21] explain how current microbial dose–response formulations can deviate from the underlying ‘single-hit’ theory.
METHODS
Previously identified information [Reference Rice1, Reference Roser5] was re-evaluated, supplemented with new data and collated. Likely densities of P. aeruginosa associated with varying likelihood of folliculitis were estimated. As different studies report cells densities variously as c.f.u./ml, c.f.u./100 ml or c.f.u./l [Reference Price and Ahearn19, Reference Ratnam22–Reference Van Asperen24] we standardized on c.f.u./ml.
Because of the diversity of the information considered, the limited number of references supporting each body of data, and uncertainties about how outbreaks correspond to reported P. aeruginosa density (e.g. sample timing), it was not possible to employ an ‘odds-ratio’ style meta-analysis used with ingested pathogens (e.g. [Reference Teunis, Ogden and Strachan8]). Therefore, input estimates were deduced from first principles, pool ecology, experimental and outbreak data, and epidemiology reports. Findings of this meta-analysis style interrogation were organized into ‘Information sets’ (Table 1): Set I reported P. aeruginosa pool outbreak densities; Set II, P. aeruginosa pool densities where no folliculitis was detected; Set III, P. aeruginosa growth/blooms in waters comparable to pools; Set IV, growth promotion by pool organic matter; Set V, susceptibility of exposed human populations; Set VI, folliculitis attack rates relative to exposure time; Set VII, folliculitis lesion density in clinical disease presence and absence; and Set VIII, inocula and strains inducing disease experimentally. We reasoned that dose–response algorithms should be consistent with all these lines of evidence. For each ‘Information set’, an interpretation was developed supporting estimation of bacterial density/infection likelihood and bacterial density/lesion density data pairs. Where possible, interpretations were quantitative (e.g. disease likelihood and severity, bacterial density), and related to outbreak/infection scenarios. From this analysis we deduced P. aeruginosa densities, infection likelihoods, and disease severity (lesion density), corresponding to folliculitis outbreak severity (Table 2).
Table 1. Information supporting estimation of folliculitis dose–response algorithm coefficients
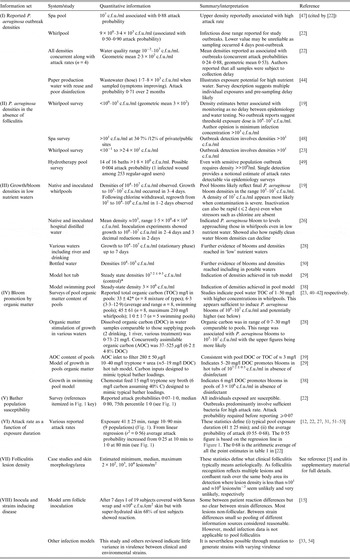
* ‘±’ = 1 standard deviation.

Equations (1) and (2) coefficients (r C , A, B) were estimated by minimizing the overall difference between data pairs in Table 2 and model estimates obtained using mainly iterative trial-and-error methods. Three programs were employed and compared, Microsoft's Excel ‘Solver’ wizard (Microsoft Corp., USA), Matlab 2012 (Mathworks, USA) and Palisade™ ‘@Risk Optimizer’ (Palisade Corp., USA), to the primary and log-transformed model. In the case of equation (1) we also estimated r C by transforming this expression into its linear form: ln[1/(1 – P inf)] = r C .C and applying Excel's least squares line fit. Prior to fitting the data we first obtained approximate estimates of the model coefficient values for the two equations from trial-and-error plots of each set of five input value pairs [X = P. aeruginosa density, Y = infection probability; equation (1) or Y = lesion density; equation (2)].
With Solver the curve fits were optimized by iteratively varying the coefficient estimates to obtain a minimum root mean square value for Y estimates vs. their algorithm estimated counterparts. In the case of Matlab we used a maximum likelihood estimator (MLE) [Reference Koshal25] assuming the ‘noise’ followed a Gaussian or Poisson distribution, as well as the program's nonlinear least squares ‘nlinfit’ estimator. In the case of Optimizer we applied the maximum likelihood method assuming noise was distributed in a Gaussian fashion. In applying these programs we assessed the sensitivity of the exponential model [equation (1)] coefficients to our assumptions about 0% and 100% attack probability.
RESULTS AND DISCUSSION
Input data set development and interpretation
Information Set I indicated that pool P. aeruginosa bloom up to densities of ≈107 c.f.u./ml. However, delays in sampling mean post-outbreak densities likely do not reflect those at the time of exposure. The data are complemented by Set II which suggests that P. aeruginosa can bloom to up to 103–104 c.f.u./ml without inducing outbreaks; and Set III, especially Favero et al. [Reference Favero26], which showed how rapidly P. aeruginosa can bloom and decline (>3 log10 in ⩽2 days). Set III also showed that P. aeruginosa blooms to ≈106–107 c.f.u./ml even in clean waters before die-back, at rates consistent with folliculitis outbreaks following a bloom and bust time-course [Reference Favero26, Reference Vogt27]. Rates of decline in real pools appear uncharacterized. However, the lack of pool detections in Hopkins et al.'s and Vogt et al.'s [Reference Hopkins, Abbott and Wallace13, Reference Vogt27] outbreak investigations and two other studies reviewed by Ratnam et al. [Reference Ratnam22] are consistent with this picture.
Peak densities are likely to vary in response to organic carbon concentrations [Reference Vital28, Reference Goeres, Loetterle and Hamilton29]. Set IV indicated that pool organic carbon concentrations are sufficient for bloom peaks of 105–107 c.f.u./ml. From these data we suggest plausible minimum and most likely densities causing outbreaks are 105 and 3 × 106 c.f.u./ml, respectively, and a density associated with maximum infection is ≈1·8 × 107 c.f.u./ml (Tables 1 and 2). For comparison, an earlier example of this meta-analysis style data interrogation to guide estimation of hazardous levels of pathogens in support of risk management is Haas & Rose [Reference Haas and Rose30].
From Set V the maximum infection probability appears to be 1·0 while the minimum attack probability where outbreaks are recognized is ≈0·07. Modal infection probability appears >0·5. From Ratnam et al. ([Reference Ratnam22], table 1 column 3), average ± standard deviation (0·68 ± 0·30) and median (0·80) attack probability can be estimated. From their own study these authors suggested ‘average’ exposure times for children (55 min) and adults (15 min). Based on their data, as well as additional literature identified in Table 1 (Set VI, nine populations), we estimated exposure times associated with outbreaks to average 41 ± 25 min. From linear regression of these data (Fig. 1), the corresponding mean attack probability was 0·55 at 41 min and 1·0 for exposures >80 min (Set VI, Fig. 1). Although women may be more susceptible to folliculitis than men [Reference Hudson31], attack probabilities appear generally high for both sexes given sufficient exposure duration. A notable feature of the data identified in this paper and our previous reviews [Reference Rice1, Reference Roser5] was that most detailed folliculitis outbreak reports were from the 1980s. We suggest that although outbreak data is still being collected on a large scale it occurs as part of routine surveillance, e.g. [Reference Craun, Calderon and Craun32], and so does not merit in-depth scrutiny which could have informed our meta-analysis.

Fig. 1. Percentage of pool users acquiring folliculitis as a function of exposure time.
Set VII indicated that lesion densities associated with the most severe, median, least and undetectable folliculitis occurrences were ≈104, 103, 2 × 102 and 100–101 lesions/m2, respectively. Finally Set VIII suggested there is little difference in infectivity risk posed by clinical compared to environmental P. aeruginosa strains and most strains can induce lesions and disease [Reference Leyden, Stewart and Kligman15, Reference Vives-Flórez and Garnica33]. In light of this and the practice of pooling data from multiple experiments and strains of algorithm construction practice with enteric pathogens (e.g. [Reference Crockett9]) we concluded it was reasonable in this first instance to combine data from different reports to construct generic dose–response algorithms. Nevertheless, we recognize that strains with different pathogenicity may be identified in the future and modify the dose–response picture.
Dose–response algorithms
From the Information sets (Table 1) we deduced data pairs (Table 2) for estimating coefficients of equations (1) and (2) (Fig. 2). Equation (1) coefficient estimates varied by ~1 order of magnitude depending on which estimator was used and whether the outer (P = 0 or 1) values were included. Matlab estimates were consistently 4·3 × 10−7 c.f.u./ml (MLE with Gaussian noise function) and 3·3 × 10−7 c.f.u./ml (nlinfit). The latter was comparable to a least squares-based estimate calculated independently by one of this paper's referees. Some Solver-derived values were higher (e.g. 5·1 × 10−7 c.f.u./ml) but varied markedly depending on upper input attack probability (A value of 0·99–0·9999 needed to be assumed as the method did not work if a P value of 1·0 was used). As the MLE-based estimate is more conservative than, and reportedly preferred by statisticians over, least squares estimators we propose an r C coefficient of 4·3 × 10−7 c.f.u./ml P. aeruginosa estimate be selected when using equation (1) for estimating clinical folliculitis likelihood in the event of bathing in swimming pools or spas with variable P. aeruginosa densities.

Fig. 2. Exponential and log-linear dose–response curve fits. Data points from Table 2.
Figure 2 also shows estimates of the log-linear algorithm, equation (2), coefficients describing how disease severity varies. For unclear reasons none of the Matlab fit estimators converged on a solution. However, Solver and @Risk Optimizer converged on comparable A and B values. The slightly more conservative Optimizer values were: A, 2.51644 × 107 lesions/m2; and B, 2.28011 × 10−11 c.f.u./ml P. aeruginosa. One of the referees pointed out that applying the least squares objective function over the organism densities of interest yields an alternative simpler algorithm: Nl = 5·4959 × 10−4 C (coefficient units, lesions per c.f.u./ml per m2). For organism densities across the range 10−1–108 c.f.u./ml the algorithm predicted lesion densities only 4% less than with the log-linear equation (2). For completeness these two algorithms are plotted side by side in Figure 2. Given the trivial difference in their lesion density estimates we suggest either could be used for P. aeruginosa folliculitis severity, but suggest the use of equation (2) with the A and B coefficient values above in line with our use of Furomoto & Mickey's theory.
These log-linear and linear algorithms are notable in that they predict lesions will be too few to detect where P. aeruginosa densities are <103 c.f.u./ml, i.e. ≈1 lesion over a whole body which would be clinically unrecognizable (Fig. 2). An important qualification on these equation coefficients is that they apply to ‘typical bathing’. Specifically Set VI indicates these algorithms correspond to average exposure of ≈41 min. Speculatively, Figure 1 suggests folliculitis likelihood might be decreased by bathing for only 10–15 min and approach 1·0 where exposure exceeds 1 h. We have included these and estimates of exposure duration because on theoretical grounds [Reference Roser5] and from experimental data [Reference Birkhead, Vogt and Hudson12, Reference Hudson31], infection likelihood depends on exposure duration and the algorithm coefficients which could differ in other circumstances.
Uncertainties
Uncertainties associated with our algorithms include the following. Dose–response coefficient estimates have four significant figures and R 2 values of 0·9. These reflect curve fit precision not risk estimate accuracy. We suggest the accuracy of equations (1) and (2) is about ± 1 order of magnitude reflecting the 105–107 c.f.u./ml P. aeruginosa cell density range bathers are likely exposed to during outbreaks (Set IV, Table 1). We have not included confidence intervals (cf. fig. 1 in [Reference Crockett9]) as there were insufficient data to warrant a full formal meta-analysis. Hot tubs operate at higher temperatures than swimming pools ([Reference Kush and Hoadley23, table 5·1], [Reference Kanan34]) and have a smaller volume-to-person ratio and likely more organic matter. These factors could result in faster growth and inactivation, higher P. aeruginosa densities, more rapid infection, more rapid disease induction and higher infection likelihood. However, because of the limited data and understanding of P. aeruginosa ecology in pool waters, we combined the two datasets. Our estimates of clinical folliculitis severity as lesions/m2 were based on a small primary dataset [Reference Roser5, supplementary material therein]. How time (Fig. 1, Set VI) or temperature impacts on infection, is uncertain but three moderating biophysical processes are likely operating concurrently and synergistically: wetting of the skin, occlusion and likelihood of P. aeruginosa skin attachment [Reference Roser5]. The influence of water and occlusion are illustrated by Leyden et al.'s study of skin response to P. aeruginosa inocula [Reference Leyden, Stewart and Kligman15] and Toll et al.'s [Reference Toll35] observations of microsphere migration into follicles during drying. The complexity of attachment to any surface including skin is illustrated by numerous biofilm studies which often use P. aeruginosa itself as a model [Reference Katsikogianni and Missirlis36]. Our algorithms necessarily apply only to P. aeruginosa folliculitis and not acute otitis externa, nor do they apply to other causes of folliculitis, e.g. Staphylococcus aureus, although the principles and approach here may be applicable to quantifying risk from other folliculitis agents.
Complementing Price & Ahearn's [Reference Price and Ahearn19] MIC formulation
A test of the value of the modelling is whether it can generate new insights, hypotheses, questions and/or management recommendations. Price & Ahern [Reference Price and Ahearn19] provided a useful benchmark that can be applied to the models presented here and indeed, theirs appears to be the only other P. aeruginosa pool MIC estimate identified to date. Their MIC was well above the recommended water quality benchmark of <10−2 c.f.u./ml raising questions of whether their proposal was reasonable and current guidelines are too onerous? Beyond this, few quantitative risk conclusions were drawn. Our analysis complements theirs with estimates of infection inducing densities, folliculitis likelihood and exposure duration and indicates their MIC is consistent with dose–response models of Furumoto & Mickey [Reference Furumoto and Mickey10], exposure and attack probabilities, and other information compiled in Table 1. Our integration, captured in equation (1), is also a step needed for quantifying folliculitis disease burden (e.g. as disability-adjusted life years). Separately, equation (2) posits a model for how folliculitis severity and likelihood vary in response to pool, ablution, environmental bathing, and industrial water exposure.
Water quality guidelines and monitoring
Current P. aeruginosa management includes monitoring of pool water densities using selective media to verify that pool disinfection and pool hygiene are satisfactory. A common benchmark density is <10−2 c.f.u./ml [37]. Exceedence triggers follow-up monitoring, pool closure, super-chlorination and dumping of pool water. Our algorithms and analysis indicate, consistent with Price & Ahern [Reference Price and Ahearn19], that for folliculitis, the <10−2 c.f.u./ml benchmark is very conservative, and considerably higher densities in the range 100–102 c.f.u./ml pose little immediate risk. Additionally equation (2) and its underlying inputs are consistent with a cell density of ≈103 c.f.u./ml being required to induce a single, probably undetectable, lesion over a whole body (≈1 lesion/m2 of skin, Fig. 2).
Nevertheless, routine monitoring still appears useful. In respect to folliculitis, detection of <102 c.f.u./ml can trigger pool closure while still allowing health authorities to credibly reassure the public that infection is unlikely. Repeatedly elevated P. aeruginosa densities in disinfected pool water, may indicate a chronic contamination source (e.g. biofilms on filters with 109 c.f.u./g [Reference Goeres, Loetterle and Hamilton29]) and bloom/outbreak potential in the event of disinfection loss (Sets III and IV). Hence detection of P. aeruginosa in the range 10−2–102 c.f.u./ml appears a credible trigger for preventive action, e.g. biofilm reservoir identification and elimination. Post-outbreak culture-based monitoring also appears useful for detecting contamination sources, e.g. poolside carpet [Reference Hopkins, Abbott and Wallace13]. However because of the high variability of P. aeruginosa populations in terms of infectivity, c.f.u. data appears of questionable value for inferring risk levels unless densities exceed to104 c.f.u./ml (Set I, Table 1).
Pool management
One driver for our reviews of P. aeruginosa in swimming pools [Reference Rice1, Reference Roser5] was a series of queries from a pool manager about the significance of, and appropriate responses to, repeated P. aeruginosa detections. They were concerned that dumping >1 million litres during a drought was wasteful and wanted clearer justification. Our analysis and algorithms provided insights into management best practice and raised questions about the benefits of water dumping and quarantining pool socializing areas from bathers.
Even clean drinking, bottled and distilled waters are capable of promoting blooms of P. aeruginosa to densities >104 c.f.u./ml [Reference Favero26, Reference Vital28] when ≈1 mg/l total organic carbon (TOC) is present. Chlorination normally controls pool densities [Reference Rice1] but makes the pool vulnerable to P. aeruginosa blooms because it also inactivates potential competitors. Constant re-introduction of P. aeruginosa can probably not be eliminated because of high bather carriage [Reference Rice1, Reference Roser5] and accumulation within pool biofilms [Reference Goeres, Loetterle and Hamilton29, Reference Goeres38]. This highlights the need for active pool management to control the levels of P. aeruginosa such that infective doses are not reached.
Conversely, the importance of disinfectant maintenance and minimizing P. aeruginosa bloom-enhancing factors, especially biofilms’ available organic matter, was affirmed. Whether biofilms are more associated with filters, pool surfaces or surrounds remains to be determined. However, the desirability of controlling organic carbon seems conclusive, as with disinfection by-products [Reference Uhl and Hartmann39–Reference Chu and Nieuwenhuijsen42].
The value of water dumping is unclear. Conceptually, dumping might reduce assimilable organic carbon (AOC) concentrations. However, the organic carbon content of even clean drinking water appears high enough [1–10 mg/l dissolved organic carbon (DOC)] to support blooms [Reference Vital28] before the added input of DOC from bathers. Conceptually, a carbon absorbing filter might be used with small pools. However, a 1 million litres Olympic size pool with 20 mg/l TOC would need filters capable of removing 20 kg of organic matter to prevent a 106–107 bloom during disinfection loss which our algorithms indicate would be a concern. Further, such filters would need careful management as they would be ideal for biofilm development. We conclude that organic matter management is desirable but probably needs technology development or adaptation of existing technology to cope with the large amount of carbon needing removal.
Other implications
Zichichi et al. [Reference Zichichi, Asta and Noto43] reported a folliculitis outbreak linked to showering. Assuming lesion densities were comparable to clinical pool-related folliculitis and exposure was sporadic, our algorithms suggest P. aeruginosa densities averaging 105–107 c.f.u./ml of bathing water. If pipe biofilms were the source, this outbreak suggests very extensive organic matter build-up, bacterial sloughing and/or massive disinfection failure. No source was identified, but a candidate contamination reservoir is illustrated in Figure 3, i.e. potable header tanks. This source reflects a common water supply situation, a lack of 24-h pressure and vulnerable buffering. Another example of high P. aeruginosa exposure resulting from poor management is high organic matter content industrial cleaning tanks [Reference Hewitt44]. This latter study illustrates how folliculitis is a risk wherever high loads of organic carbon accumulate and intimate exposure can occur. Peak P. aeruginosa density of 8 × 103 c.f.u./ml was close to the ≈ 104 c.f.u./ml which combined with a modest order of magnitude bloom would be sufficient to affect exposed workers.

Fig. 3. Header tanks, a potential domestic water contamination source. (a) Poor tank management (top lost or discarded); (b) tank contaminant accumulation (algae and cyanobacteria provide carbon for biofilms); (c) bath water.
A converse exposure scenario is ‘natural bathing’ in freshwater streams, pools and lakes. P. aeruginosa is recognized to pose a risk [Reference Casanovas-Massana and Blanch18, Reference Van Asperen24] but there do not appear to have been reports of folliculitis outbreaks or precursor densities from our reviews. For example, for four lakes meeting the Dutch and the European Commission's mandatory standards for thermotolerant coliforms the median (10th and 90th percentile) densities were 4 (1 and 63) and ×10−3 c.f.u./ml, respectively [Reference Van Asperen24]. The lack of outbreaks is consistent with expectations based on our dose–response algorithms. In natural bathing situations P. aeruginosa might be controlled by natural environmental processes, e.g. cooler water, intense sunlight, microbial competition for DOC. Nevertheless the risk of blooms remains, as illustrated by E. coli blooms in natural waters [Reference Power45, Reference Ashbolt, Dorsch, Banens, Kay and Fricker46] and P. aeruginosa in drinking water [Reference Vital28].
RECOMMENDATIONS AND FUTURE WORK
Monitoring
Routine culture-based water quality monitoring still appears useful, pre- and post-outbreak, but would be improved upon by meta-genomic studies to better describe potentially suppressive or promoting microbial communities. Furthermore, increased data collection appears desirable. Based on our data analyses we suggest the following testing also be considered:
-
• qPCR analyses of water, and water filters for 16S rRNA gene copy numbers and strain markers to follow blooms and avoid cell injury/viable but non-culturable cell detection constraints and provide an indication of bloom peaks. (Large sample volumes should not be needed where blooms occur. As cost reduces, supplement this with 16S rRNA meta-genomic analyses of community structures, both pool water and skin.)
-
• Organic matter analysis, especially DOC.
-
• Photographic documentation of pool outbreak environments.
-
• Detailed records of folliculitis morphology especially lesion density.
-
• Biopsies of infections for skin lesion vs. pool strains.
We again suggest developing pool ‘Water Safety Plans’ [Reference Rice1]. Based on our meta-analysis and algorithm to interpret culture-based monitoring data we propose the following as a guide. For single/sporadic detections at 10−2–100 c.f.u./ml, check basic bathing system vulnerabilities then re-test. Do not panic or close a pool unless there is breakdown of critical treatment barriers, e.g. chlorinator, identified. For single 100–102 c.f.u./ml or repeat 10−2–100 c.f.u./ml detections suspend pool access. Then re-test immediately including a check of DOC concentration (preferably <10 mg/l). Check for system vulnerabilities and re-test. Finally if clear, return to a normal monitoring programme. If not clear undertake wider testing, e.g. organic carbon, filter, source water and remediate and re-test as required. For single results >102 c.f.u./ml or repeats >100 c.f.u./ml suspend access, and determine contamination source and solution, experimentally or via survey. Then implement remediation and undertake high-frequency routine monitoring for a period to confirm efficacy.
Following outbreaks, we suggest closing the pool indefinitely and undertaking an array of testing including DOC, qPCR of water and niches where P. aeruginosa could originate, as well as detailed epidemiology, and including satisfactory replicate and control tests. Then the pool management system should be revised in light of outbreak lessons. If necessary install new treatment equipment and increase management action frequencies. Publish this information. Increase routine monitoring frequency for several months to ensure the problem is solved.
Research directions
Following the current study we now plan an analogous analysis of data applicable to mechanism-based dose–response algorithms. Separately, we suggest that follow-up risk management studies be undertaken to test equations (1) and (2) and our interpretation. Given ethics considerations, we suggest such work should focus on improved monitoring complementing current testing e.g. qPCR, DOC, testing rationale, data interpretation. Another useful direction would be further studies of P. aeruginosa and opportunist bloom ecology generally modelled [Reference Favero26, Reference Vital28, Reference Goeres, Loetterle and Hamilton29, Reference Goeres38, Reference Power45, Reference Ashbolt, Dorsch, Banens, Kay and Fricker46] in support of prevention and source identification.
ACKNOWLEDGEMENTS
We thank the referees for their helpful comments in sorting the curve fits. We also thank Hoori Ajami for help with Matlab analysis.
DECLARATION OF INTEREST
None.