INTRODUCTION
Epidemic models have been important in understanding the spread of infectious diseases and evaluating the introduction of intervention strategies like vector control and vaccination. Infectious disease dynamics are by nature nonlinear and the understanding of such nonlinear epidemiological processes is vital for any modern society, from the medical as well as the economic perspective. However, it is intrinsically mathematically difficult, and to make the urgently needed progress in improving our understanding of the dynamics of infectious diseases, concepts from various fields of mathematics as well the availability of good-quality datasets for model evaluation are needed.
Dengue fever (DF), a viral mosquito-borne infection is a major international public health concern with about 3 billion people at risk of acquiring the infection [1]. It is estimated that every year, there are 70−500 million dengue infections, 36 million cases of DF and 2·1 million cases of dengue haemorrhagic fever (DHF)/dengue shock syndrome (DSS), with more than 20 000 deaths per year [1, 2].
Infection by dengue virus causes a wide range of clinical manifestations and its classification into DF and DHF/DSS are given according to World Health Organization (WHO) guidelines. DF is an acute febrile viral disease frequently presenting with headaches, bone or joint and muscular pains, rash and leukopenia as symptoms. DHF is characterized by four major clinical manifestations: high fever, haemorrhagic phenomena, often with hepatomegaly and, in severe cases, signs of circulatory failure. Infected patients may develop hypovolaemic shock resulting from plasma leakage. This is designated DSS and can be fatal [3]. In 2009, the revised WHO case definition was proposed, classifying the illness into dengue with and without warning signs, and severe dengue [4]. The revised scheme is claimed to be more sensitive to the diagnosis of severe dengue; however, it is considered by many to be too broad, requiring more specific definition of warning signs [Reference Hadinegoro5].
Dengue fever epidemiology dynamics show large fluctuations of disease incidence and mathematical models describing transmission of disease ultimately aim to be used as predictive tools to evaluate the introduction of intervention strategies. Dengue illness is popularly known in Thailand as ‘dengue’. The English translation of the Thai word used to describe dengue illness refers to ‘fever with blood leakage’ and the English translation of the Thai language is pronounced kâi lêuat ôk. Both DF and DHF without shock can be written in the Thai language using the same combination of letters as depicted in Figure 1 [items (1) and (2)]. If DF cases are often benign or asymptomatic, DHF cases may evolve towards a group of symptoms with haemorrhagic fever leading to shock or DSS, that when written in the Thai language, uses a different combination of letters [see Fig. 1, item (3)] with the following English pronunciation: glùm aa-gaan kâi lêuat ôk chôk. Up to now, 33 years of dengue illness incidence data in Thailand are available and have been continually used by modellers to parametrize mathematical models (see e.g. [Reference Cummings6–Reference Bhatt10]).
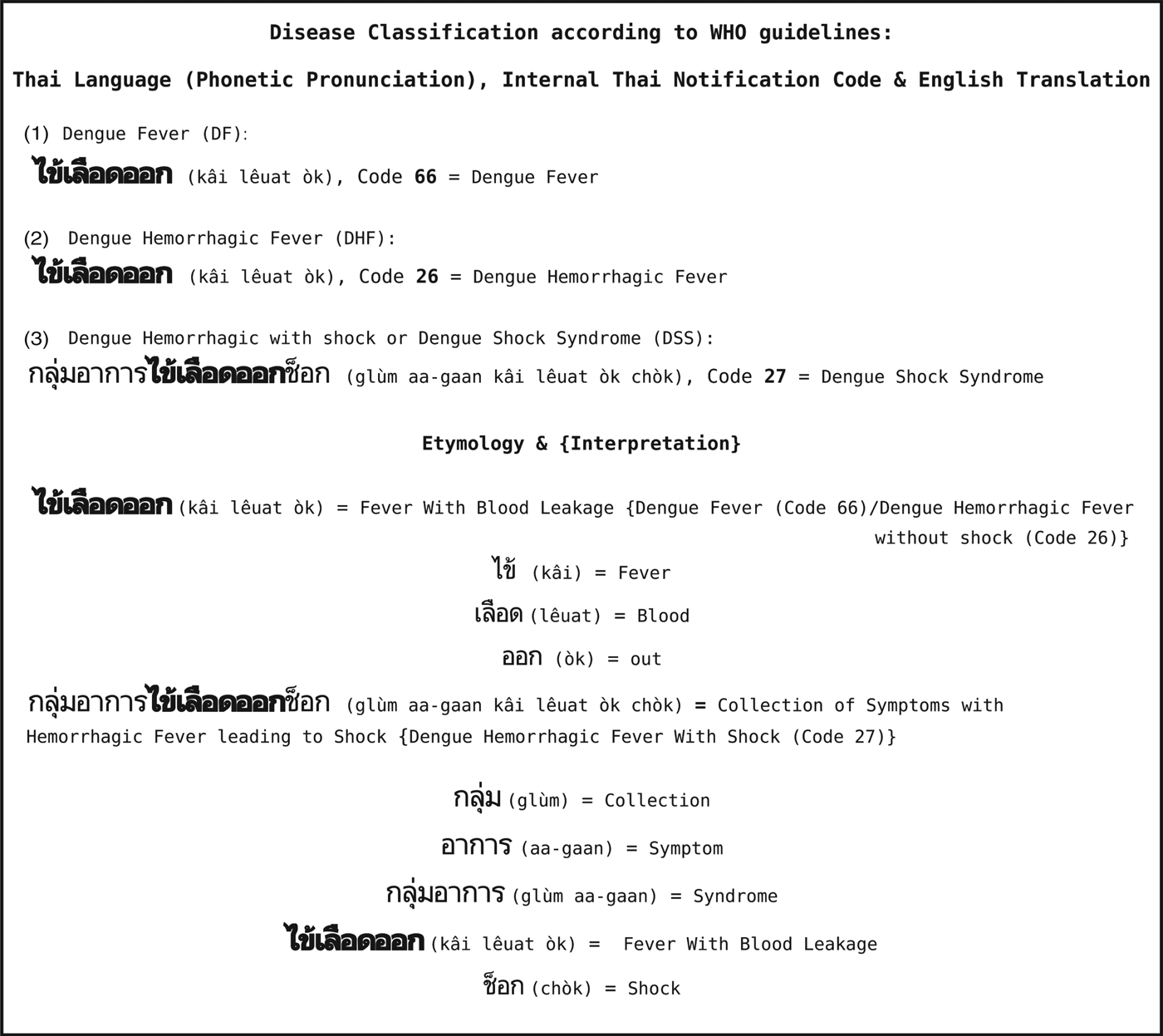
Fig. 1. Dengue illness notification diagram and etymology. Items (1), (2) and (3) give disease classification according to the WHO [3]. We present the Thai written form followed by the English pronunciation (in parentheses) and the Thai internal classification code for disease notification (for more information, see Appendix A). The Thai words for dengue fever/dengue haemorrhagic fever and dengue shock syndrome are depicted to complete the etymological study.
In this paper, a systematic data collection and its analysis were performed. Our approach consisted of gathering and analysing the dengue incidence data provided by the Bureau of Epidemiology (BoE), Ministry of Public Health (MoPH) in Thailand. The official data was cross-checked with the data used in recent publications and an inexact continuous time-series appeared to have been consistently used since 2003, affecting considerably the model dynamics and its correct application. Here, the inexact continuous time-series reflects a time-series on the incidence of the disease where only part of the official data (from 2003 onwards) has been used to continue the previous available data (from 1980 to 2002). Two different datasets, based on the interpretation of the Thai official documents, generated different model dynamics that could be used as a public health intervention tool. Numerical analysis and simulation techniques giving insights on predictability were performed and the modelling parametrization effects discussed.
METHODS
Data collection
In Thailand, a system for reporting communicable diseases including DF, DHF and DSS was considered fully operational in 1974 [Reference Chareonsook11] and the database is available at the BoE, MoPH, Bangkok, Thailand. Reasonable data from all provinces exist from the beginning of the 1980s [2, 12, 13].
From 1980 to 2005 the aggregated monthly incidence data have been publicly distributed through BoE annual epidemiological surveillance reports [12]. These data are available as a hard copy (HC) book format and the incidence of all hospital admissions for dengue cases (DF, DHF, DSS), reported to the national surveillance system, are presented as the dengue haemorrhagic fever total (DHF-total). From 2003 to the present, the data have been available as electronic files (EF) where each one of the clinical classifications of the disease (DF, DHF, DSS) are notified separately, according to WHO guidelines [3]. The aggregation of all hospital admission cases, gives rise to the HC-DHF-total incidence data and this has been available, since 2003, as BOE weekly epidemiological surveillance reports. These reports explicitly state that the DHF-total=DF+DHF+DSS (see [13]), and despite this information being publicly available, consistent underestimation of dengue cases has been used for modelling purposes, probably due to misinterpretation of the official Thai documents. We note that the HC-DHF-total cases have been overlooked by the non-Thai mathematical and epidemiological community, which considers only clinical EF-DHF cases as the natural continuation of the previously aggregated data (DF+DHF+DSS).
The official monthly incidence of dengue illness is presented for Chiang Mai (Fig. 2 a–c) and for the whole of Thailand (Fig. 2 e–g). For the epidemiological years 2003, 2004 and 2005 both sources of data are available, i.e. the HC-DHF-total and EF for DF, DHF and DSS notification cases. We listed the given numbers for HC-DHF-total, EF-DF, EF-DHF and EF-DSS cases, respectively, and observed that the number of cases notified as EF-DHF differs considerably from the numbers presented as HC-DHF-total in [12] and also available publicly in [13]. When taking into account the numbers of EF (DHF+DSS+DF) cases it can be seen that the final numbers match the original data collection of HC-DHF-total cases, confirming the origin of the lower numbers of dengue cases used in recent publications.

Fig. 2 [colour online]. Data comparison between hard copy dengue haemorrhagic fever (DHF)-total and electronic files for dengue fever (DF), DHF and dengue shock syndrome (DSS), respectively, for Chiang Mai province, in (a) 2003, (b) 2004, (c) 2005; (d) is a histogram for the underestimation of dengue cases, from 2003 to the present. Data comparison between hard copy for DHF-total and electronic files for DF, DHF and DSS, respectively, for the whole of Thailand, in (e) 2003, (f) 2004, (g) 2005; (h) is a histogram for the underestimation of dengue cases, from 2003 to the present.
Figure 2(d, h) show histograms for the underestimation of dengue cases, for Chiang Mai and Thailand, respectively, from 2003 to the present. The underestimation of cases is increasing rapidly and for any modelling interpretation based on long-term empirical incidence dengue data (from 1980 to the present), the aggregation of all hospital admissions for dengue cases (from 2003 to the present) is essential to improve model development, interpretation and its correct application. Such cross-checking of data was performed for all provinces in Thailand with similar results, leading to a large underestimation of cases for the whole of Thailand (see Fig. 2 h).
Data used in recent publications
The data used in recent publications [Reference Cummings6–Reference Aguiar9] are from 1982 to 2004. For two years, 2003 and 2004, both sources of data are available, and as shown by the blue line in Figure 3, only EF-DHF cases were used to continue the previous HC-DHF-total data from 1982 to 2002. The source of misinterpretation comes from the fact that the numbers for EF-DHF cases are not equal to the numbers for HC-DHF-total cases (see Fig. 2), generating an inexact continuous time-series used for model parametrization.

Fig. 3 [colour online]. Time-series data comparison between recent publications, the hard copy dengue haemorrhagic fever (HC-DHF)-total data and the electronic file (EF)-DHF data. Blue indicates data that have been used in recent publications [Reference Cummings6–Reference Aguiar9], black indicates the official data [from 1980 to 2003: HC-DHF-total; from 2003 to present: EF (DHF+DSS+DF)], provided by the Bureau of Epidemiology, Ministry of Public Health, Thailand, red indicates EF-DHF cases only, from 2003 to the present for (a, b) Bangkok, (c, d) Chiang Mai, (e, f) Thailand.
In Figure 3 we present the time-series comparison for Bangkok (Fig. 3 a, b) and Chiang Mai (Fig. 3 c, d) provinces, and for the whole of Thailand (Fig. 3 e, f). The blue line indicates data that have been used in recent publications [Reference Cummings6–Reference Aguiar9] giving results that could be used by the public health authorities for disease control. The black line indicates official HC-DHF-total data, and the red line indicates EF-DHF-only cases (from 2003 to the present), provided by the BoE, MoPH. Both trajectories (i.e. red and black), are approximately the same from 1982 to 2002; however, from 2003 onwards, differences begin to appear and become larger, leading up to 70% of the underestimation of the real number of cases for Chiang Mai (Fig. 2 d) and up to 50% of the underestimation of the real number of cases for the whole of Thailand (see Fig. 2 h).
Compartmental models applied to dengue fever
Almost all mathematical models for infectious diseases start from the same basic premise: that the population can be subdivided into a set of distinct classes. The most commonly used framework for epidemiological systems remains the susceptible-infected-recovered (SIR) type model, a good and simple model for many infectious diseases. Multi-strain dengue models are modelled by SIR-type models where the SIR classes are labelled for the hosts that have seen the individual strains.
The two-strain model
Retrospective dengue data and the possibility of estimating hidden states from the available data by modelling DF epidemiology [Reference Aguiar9, Reference Stollenwerk14] have been discussed, especially, primary vs. secondary infections, and symptomatic vs. asymptomatic cases that can be studied via the first available models [Reference Aguiar9, Reference Aguiar, Kooi and Stollenwerk15, Reference Aguiar, Stollenwerk and Kooi16].
A comparison between the basic two-strain dengue model, which already captures differences between primary and secondary infections, including temporary cross-immunity, with the four-strain dengue model, that introduces the idea of competition of multiple strains in dengue epidemics shows that the difference between first and secondary infections drives the rich dynamics more than the detailed number of strains to be considered in the model structure [Reference Aguiar17]. Chaotic dynamics were found to occur in the same parameter region of interest for the two- and four-strain models, being able to describe the fluctuations observed in empirical data and showing a qualitatively good agreement between empirical data and model simulation. The predictability of the system does not change significantly when considering two or four strains, i.e. both models present a positive dominant Lyapunov exponent (DLE) giving approximately the same prediction horizon in time-series. Since the law of parsimony favours the simplest of two competing models, the two-strain model is the better candidate for analysis, as well the best option for estimating all initial conditions and the few model parameters based on the available incidence data.
The seasonal two-strain model with import of infected hosts has shown a qualitatively good result when comparing empirical dengue data and simulation results, where patterns of the data behaviour were similarly found to occur in the time-series simulations [Reference Aguiar9, Reference Aguiar, Stollenwerk and Kooi16, Reference Aguiar17].
The two-strain model is represented in Figure 4 using a state flow diagram. The boxes represent the disease-related stages for the host and the arrows indicate the transition rates. This is a minimalistic model for host dynamics and the effects of the vector dynamics are only taken into account by the force of infection (FOI) parameter [Reference Aguiar9, Reference Rocha and Jesus18].

Fig. 4 [colour online]. The state flow diagram for the two-strain model. The boxes represent the disease-related stages and the arrows indicate the transition rates. The transition rate μ coming out of class R represents the death rates of all classes, S, I 1, I 2, R 1, R 2, S 1, S 2, I 12, I 21, R, entering class S as a birth rate.
In the two-strain model suggested by Aguiar [Reference Aguiar19] the population N is divided into ten classes and the model dynamics are described as follows. Individuals susceptible to strains, 1 and 2 (S) can acquire primary dengue infection with strain 1 (I 1) or strain 2 (I 2) with two possible infection rates, dependent upon who is transmitting the infection. If the host transmitting the infection is in the first infectious state, the transmission rate is β, but if the host transmitting the infection is in the secondary infectious state, the transmission rate is ϕβ. Here, the parameter ϕ is motivated by the antibody-dependent enhancement (ADE) effect and it is related to the secondary infection transmissibility factor, increasing or decreasing the transmissibility of secondarily infected individuals. For more information on the parametrization of ADE and secondary dengue infections by ϕ, see [Reference Aguiar19].
The primarily infected hosts recover with a recovery rate γ and have full and lifelong immunity against the strain they were exposed to. Individuals become susceptible again, able to get a second infection with a different strain, after a short period of temporary cross-immunity α. A susceptible individual with a previous infection with strain 1 (S 1) or strain 2 (S 2) gets the secondary infection with strain 2 (I 12) or strain 1 (I 21), respectively, at infection rate β or ϕβ, again depending on who (an individual with a primary or secondary infection) is transmitting the infection. Then, with recovery rate γ, the individuals recover (R) and become immune against all strains. For simplicity, no epidemiological asymmetry between strains is assumed, i.e. infections with strain 1 followed by strain 2 or vice versa contribute in the same way to the FOI. Significant differences between strains lead to extinction of one of the strains, hence it is not biologically relevant [Reference Mier-y-Teran-Romeroa, Schwartz and Cummings20]. Here, the difference concerning disease transmissibility is that the FOI varies according to the number of previous infections that a host has experienced. First-time infected individuals are considered asymptomatic or not admitted to a hospital. A percentage of individuals experiencing secondary infection are assumed to be a symptomatic notified case for DF, DHF or DSS.
RESULTS
In this section we discuss numerical analysis and simulation techniques giving insights on predictability that were performed to demonstrate the effects of model parametrization using different datasets. We take the province of Chiang Mai in Thailand as a case study and match the empirical dengue data with 90% of secondary infections from the model simulations. The objective is to obtain a parameter set able to describe fluctuations of dengue dynamics. The two-strain model is used to mach two different datasets. Two parameters are estimated based on the empirical data, the infection rate (β) and ADE ratio (ϕ). The other parameters are fixed for simplicity and shown in Table 1.
Table 1. Parameter values generated via data matching

Time-series parameter inference
Depending on the modelling group's interpretation of the official Thai documents, at least two possible datasets can be generated from the long-term empirical data which are available for Thailand. The first dataset, designated ‘dataset 1’, gives the most correct long-term data, and consists of all hospitalization case notifications. From 1980 to 2002 the HC-DHF-total = DF + DHF + DSS data are continued with the EF(DF + DHF + DSS) data, from 2003 to the present. The two-strain dengue model suggested by Aguiar et al. [Reference Aguiar9, Reference Aguiar, Kooi and Stollenwerk15], is able to describe dataset 1 [see Fig. 5(a, b)], when assuming β = 2γ and ϕ = 0·9.

Fig. 5 [colour online]. From 1980 to 2012 dengue incidence data for Chiang Mai province in Thailand matched with the seasonal two-strain model simulations. The birth and death rate, recovery rate, degree of seasonality and the temporary cross-immunity rate are fixed and given in Table 1. The infection rate and ratio of secondary infections contributing to the force of infection (FOI) are the parameters that may vary according to the dataset described by the model simulations. For dataset 1, empirical hard copy data [HC-dengue haemorrhagic fever(DHF)-total=dengue fever (DF)+DHF+dengue shock syndrome (DSS)] (in red) are matched with model simulation (in blue). (a) From 1980 to the present, (b) from 2003 to the present. Here, the infection rate is β = 2γ and the ADE ratio is ϕ = 0·9. Dataset 2, where empirical HC-DHF-total cases (in red) from 1980 to 2002 are continued from 2003 onwards with electronic file (EF)-DHF-only cases (in green), are matched with model simulation (in blue). (c) From 1980 to 2002, (d) from 2003 to the present. Here, the infection rate is considerably smaller, β = 1·5γ, as is the ADE ratio, ϕ = 0·7.
The second possible dataset, designated ‘dataset 2’, consists of the HC-DHF-total from 1980 to 2002, and it is continued from 2003 onwards with the EF-DHF data only. Note that the EF(DF+DSS) cases are neglected, leading to considerable underestimation of dengue cases (see Fig. 2 d). The two-strain model of Aguiar et al. [Reference Aguiar, Kooi and Stollenwerk15] is also able to describe dataset 2, but with a different infection rate (β = 1·5γ) and different ADE ratio (ϕ = 0·7).
For Chiang Mai province in Thailand, Figure 5(a, b) shows empirical dataset 1, HC-DHF-total=DF+DHF+DSS (in red) matched with the two-strain model simulation (in blue). A qualitatively good result is obtained, where patterns of irregular data occur and is predicted by the model. In Figure 5(c, d) the empirical dataset 2, the DHF-total from 1980 to 2002 (in red) continued with the EF-DHF data only (in green), is matched with the two-strain model simulation (in blue). Here, a qualitatively good match is observed from 2003 onwards (see Fig. 5 d); however, the dynamics are not able to describe the previous HC-DHF-total data, where higher outbreaks are observed (see Fig. 5 c).
For each one of the parameter sets, the model dynamics are compared and the results presented as follows.
Model dynamics and predictability
From the time-series simulations obtained by matching datasets 1 and 2 (see Fig. 5), we present the respective state space plots for the number of susceptibles vs. the logarithm of secondary infections for the two-strain model (see Fig. 6 a, b).

Fig. 6 [colour online]. Model dynamics and predictability based on the data collection used for model parametrization. Dataset 1: (a) the state space plot where a chaotic attractor is shown, (b) the Lyapunov spectrum, a fingerprint (positive DLE) for the chaotic dynamics generated by the model. Dataset 2: (c) the state space plot where a torus attractor is shown, resembling a quasi-periodicity behaviour, (d) the Lyapunov spectrum, where only periodic behaviour is confirmed to occur.
Using the state space plots in terms of the variables S and the logarithm of the total number of infected individuals I, given the dataset which is used, fixed points appear as one dot per parameter value, limit cycles appear as two dots, double-limit cycles as four dots, more complicated limit cycles as more dots, and chaotic attractors as continuously distributed dots for a single parameter value (see Fig. 6 a, b).
The attractor structures from the model dynamics, fixed point, limit cycle and more complex geometrical objects (e.g. torus) or chaotic attractor can be quantified by calculating the Lyapunov exponents [Reference Ruelle21, Reference Ott22]. Lyapunov exponents are essentially a generalization of eigenvalues determining stability vs. instability along trajectories. A negative largest Lyapunov exponent indicates a stable fixed point as attractor, a zero largest Lyapunov exponent indicates a stable limit cycle and a positive largest Lyapunov exponent indicates a chaotic attractor.
The Lyapunov spectrum for the model dynamics based on datasets 1 and 2, shown in Figure 6(c, d), respectively, are compared regarding the prediction horizon of the monthly peaks. The dynamics generated by parameter set 1 is chaotic, with a positive Lyapunov exponent, a system with a short-term predictability and a long-term unpredictability. Here, the DLE for ϕ = 0·9 is λ = 0·118, giving about 8 years of prediction horizon. For such a scenario, and knowing that stochasticity would decrease the given prediction, a long-term control strategy would not be of practical use. The alternative would be constant evaluation of the intervention measures combined with the predictability given the updated real-world data. However, by using parameter set 2, the system shows a completely different behaviour, where quasi-periodicity is observed. Here the DLE is approximately zero, giving thousands of years of prediction horizon. For such a scenario, the long-term control strategy would be effective for disease control.
From the originated model dynamics, intervention measures are suggested and implemented in order to control the disease transmissibility and to prepare the public health authorities for the next dengue season. Assuming the dynamical scenario generated by parameter set 1 (the complete dataset showing chaotic behaviour), any public health decision that was suggested would be restricted to being applied only during a short period of time where the prediction is reliable. For parameter set 2, where the incorrect interpretation of the data is used, the system shows a periodic behaviour where dengue incidence for the next seasons could be anticipated and long-term control strategies would be of practical use. Here, the long-term control strategies would probably be inefficient for dengue fever control in Thailand, where the incidence of the disease resembles chaotic behaviour where long-term unpredictability is known to occur.
DISCUSSION AND CONCLUSION
In this paper a systematic data collection and its analysis were performed. By cross-checking and analysing the overlapping epidemiological years of dengue data in Thailand, a considerable underestimation of cases was observed to be consistently used for modelling purposes, and from 2003 onwards, only part of the official data have been used to continue the HC-DHF-total data. As the time-series is updated, the underestimation of cases increases.
For Bangkok, as shown in Figure 3 b, the underestimation appears to be mild, with about 14% of cases being neglected in 2003 and 2004, and only 8% in 2005. Studying the numbers for 2010, for example, the neglected cases increase considerably, up to 30% underestimation. For Chiang Mai, as shown in Figure 3 d, and for Thailand, as shown in Figure 3 f, the underestimation is even greater, with variation from 31% in 2003 up to 65·5% in 2010 and from 29·5% up to 48% in 2010, respectively.
The time-series parameter inference shows different dynamical behaviours, depending on the data collection to be described via the modelling approaches. Dynamically, the two-strain model was able to describe the correct dataset (dataset 1), where all the admission case notifications are considered. A chaotic behaviour was found, where short-term predictability is characteristic, and for the design of any health-related interventions the particularity of this dynamical behaviour has to be considered. Here, for each recovered individual two new infections are observed (β = 2γ), and individuals in the secondary infectious stage transmit the disease 10% less (ϕ = 0·9 due to hospitalization) than individuals in the first infectious stage.
For dataset 2, where dengue cases are underestimated, the model dynamics resembles quasi-periodicity and the HC-DHF-total data, showing high outbreaks in irregular periods, can not be described by this system, promoting an ineffective control strategy to be used by the public health authorities, where up to 25% of new infections are missed and individuals with secondary infections are assumed to transmit the disease 30% less (ϕ = 0·7 due to hospitalization) than individuals in the first infectious stage.
These findings have important implications for the effectiveness of intervention measures that will be provided to public health authorities for dengue control. This study provides support for the importance of different modelling groups working with the same long-term empirical incidence dengue data in Thailand, e.g. [Reference Cummings6–Reference Bhatt10], and concludes that for any model interpretation based on Thai incidence data, dengue hospital admission cases should be aggregated (DF + DHF + DSS) to continue the previous HC-DHF-total data. This is essential to improve model development, interpretation and its correct application.
APPENDIX A
De-codifying dengue in Thailand: data interpretation
Much of the dengue data available to theoretical epidemiologists consists of time-series tracking of the evolution of a subset of state variables of an underlying dynamical system through a surveillance system. In Thailand, a system for reporting communicable diseases including DF, DHF and DSS was considered fully operational in 1974 [Reference Chareonsook11] and the database is available at the BoE, MoPH, Bangkok, Thailand. Reasonable data from all provinces exist from the beginning of the 1980s.
The surveillance system in Thailand reports hospital admissions of dengue cases, which includes all forms of dengue fever illness manifestations, hence all three possible clinical classifications according the WHO [3], i.e. DF, DHF without shock, and DSS.
From 1980 to 2005 the aggregated data at the provincial level have been publicly distributed through BoE annual epidemiological surveillance reports [12]. These data are available as a hard copy (HC) book format, where the number of cases is presented as DHF-total. From 2003 to the present the data have been available in electronic format (EF), using separate files for each one of the clinical classifications of the disease (DF, DHF, DSS). The sum of all classifications gives rise to the DHF-total monthly incidence data per province, available since 2003 in BoE weekly epidemiological surveillance reports [13]. Those reports explicitly state the DHF-total (DF + DHF + DSS); however, the English translation of the Thai documents still causes confusion when interpreting the data, underestimating the real number of dengue cases in Thailand.
In the Thai language, both the DF and DHF disease classifications are pronounced as kâi lêuat ôk, according to Thai phonetic pronunciation and DSS as glúm aa-gaan kâi lêuat ôk chôk. For the etymology of Thai words see Figure 1. Classically, the Thai word referring to a fever with blood leakage (kâi lêuat ôk), was used to describe a haemorrhagic viral disease (viral haemorrhagic fever; VHF), which was later associated with a specific group of viruses, the so-called dengue viruses. Nowadays ‘fever with blood leakage’ is occasionally specified as ‘fever with blood leakage caused by a dengue virus’, to distinguish it from ‘fever with blood leakage’ caused by other pathogens, but not in official documents, where only ‘fever with blood leakage’ is used. The abbreviation DHF-total includes all forms of hospitalized dengue fever cases, hence all three classes of the WHO classification.
The diagram presented in Figure 7 represents the separation of VHFs (shown in red) which can be caused by a dengue virus (shown in yellow, representing clinical DHF cases) and eventually a more severe case (shown in blue, representing clinical DSS cases which are DHF cases with signs of shock). Classical dengue cases without haemorrhagic symptoms (shown in green) are represented externally to the VHF class, but should be included with the class of ‘disease caused by a dengue virus’ in order to give the real overview of the number of dengue cases.

Fig. 7 [colour online]. Diagram representing the separation of viral haemorrhagic fever, in red (VHFs=1+2+4) into dengue haemorrhagic fever cases (DHF=2, in yellow), dengue shock syndrome cases (DSS=4, in blue, which are DHF cases with signs of shock) and non-dengue VHF (1, in red). External to VHF cases are the DF cases (3, in green).
Most Thai words expressing kinship have no direct translations and require additional words. There are no Thai equivalents for most daily English kinship terms, as English terms leave out much information that is natural to Thai. The Thai word used to describe dengue illness refers to haemorrhagic fever in general and its English translation can cause confusion when interpreting the data which are available for Thailand. Up to now, 33 years of dengue incidence data are available and have been continually used to parametrize mathematical models. Based on systematic data collection and its analysis, we observed a considerable underestimation of cases, where from 2003 onwards only clinical classification of ‘DHF’ cases have been considered for modelling purposes. The correct data is given by the aggregation of all admitted cases of ‘DF+DHF+DSS’ and for any interpretation based on the long-term empirical data, aggregation is essential to improve model interpretation and correct application. In the overlapping years, 2003–2005 inclusive, where both sources of data exist, the continuation of ‘HC-DHF-total’ was performed by using ‘EF-DHF’ only, leading to severely lower cases of dengue than the real number produced by ‘HC-DHF-total’. It should be noted that the official sources of the BoE emphasize that ‘DHF-total’ always refers to DF + DHF + DSS [13], but this is often overlooked by the non-Thai mathematical and epidemiological community [Reference Cummings6–Reference Bhatt10].
APPENDIX B
The two-strain model framework
Multi-strain dynamics are generally modelled with SIR-type models and have demonstrated critical fluctuations with power-law distributions of disease cases, exemplified in meningitis and dengue epidemiology [Reference Stollenwerk and Jansen23–Reference Massad25]. Dengue models including multi-strain interactions via ADE but without a temporary cross-immunity period, e.g. [Reference Ferguson, Anderson and Gupta26–Reference Billings28], have shown deterministic chaos when strong infectivity on secondary infection was assumed. The addition of a temporary cross-immunity period in such models shows a new chaotic attractor in an unexpected parameter region of reduced infectivity on secondary infection [Reference Aguiar9, Reference Aguiar, Kooi and Stollenwerk15, Reference Aguiar17], i.e. deterministic chaos was found in wider parameter regions. This indicates that deterministic chaos is much more important in multi-strain models than previously thought, and opens new ways of data analysis for existing dengue time-series, as is shown below. It offers a promising perspective on parameter-value inference from dengue case notifications.
The seasonal multi-strain model is represented in Figure 4 by using a state flow diagram, dividing the population into ten classes: susceptible to both strains 1 and 2 (S), primarily infected with strain 1 (I 1) or strain 2 (I 2), recovered from the first infection with strain 1 (R 1) or strain 2 (R 2), susceptible with a previous infection with strain 1 (S 1) or strain 2 (S 2), secondarily infected with strain 1 when the first infection was caused by strain 2 (I 21) or for being infected a second time with strain 2 when the first infection was caused by strain 1 (I 12). It should be noted that infection by one serotype confers lifelong immunity to that serotype. Finally, we have the recovered individuals from secondary infection (R). To give more reality to the dynamics of the disease, we also add a low import factor of infected individuals into the system.
To capture differences in primary infection by one strain and secondary infection by another strain we consider a basic two-strain SIR-type model for the host population, which is only slightly refined as opposed to previously suggested models for dengue fever [Reference Ferguson, Anderson and Gupta26–Reference Billings28].
The complete system of ordinary differential equations for the seasonal multi-strain epidemiological model is shown in equation (B1), and the dynamics are described as follows. Individuals susceptible to both strains can get the first infection with strains 1 or 2 with FOI βI/N, when the infection is acquired from an individual with his first infection, or ϕβI/N when the infection is acquired from an individual with his second infection (for more information on the parametrization of ADE and secondary dengue infection by ϕ, see [Reference Aguiar, Kooi and Stollenwerk15, Reference Ferguson, Anderson and Gupta26]). Individuals recover form the first infection at a recovery rate γ, conferring full and lifelong immunity against the strain they were exposed to, and also have a short period of temporary cross-immunity α against the other strain, becoming susceptible to a second infection with a different strain. A susceptible individual with a previous infection gets a secondary infection with FOI βI/N or ϕβI/N depending on who (individual with primary or secondary infection) is transmitting the infection. Then, with recovery rate γ, individuals recover and become immune against all strains. We assumed no epidemiological asymmetry between strains (β 1 = β 2 = β, ϕ 1 = ϕ 2 = ϕ), i.e. infections with strains 1 or 2 contribute in the same way to the FOI. Here, the only relevant difference concerning disease transmissibility is that the FOI varies according to the number of previous infections the host has experienced. In a primary infection, individuals transmit the disease with a FOI βI/N whereas in a secondary infection the transmission is given with a FOI ϕβI/N, where ϕ can be larger or smaller than the unit, i.e. increasing or decreasing the transmission rate.

The parameter β takes seasonal forcing into account as a cosine function and is given explicitly by

where β 0 is the infection rate, η is the degree of seasonality and ϕ the phase which becomes important only when considering empirical time-series. In this model, a susceptible individual can also become infected by contact with an infected individual from an external population (hence (β/N · S · I) goes to (β/N · S · (I + ρ · N)) contributing to the FOI with an import parameter ρ. The parameter ϕ in our model is the ratio of secondary infection contribution to the FOI. For instance, we study the region of parameter ϕ < 1, which acts to decrease the infectivity of secondary dengue infection, where hospitalization is more likely due to the ADE effect associated with the severity of disease. Individuals with a secondary infection do not contribute to the FOI as much as people with a primary infection.
The deterministic model formulation is based on the large number assumption. As a consequence, the number of individuals can be used to scale all state variables of the model. The constant population N = 100 is used for clarity so that all epidemiological components (susceptible, infected, recovered) are given as a percentage. The demography rate is denoted by μ and the parameter values are given in Table 1.
The two-strain model in its simplicity is a good model for analysis, giving the expected complex behaviour to explain the fluctuations observed in empirical data. It is minimalistic in the sense that it can capture the essential differences of primary vs. secondary infection without needing to restrict the ADE effect to one or another region in parameter space. For future parameter estimation only the two-strain model could attempt to estimate all initial conditions as well as the few model parameters. The two-strain model showed a qualitatively good result when comparing empirical dengue data and model simulations, giving insights into the relevant parameter values purely on topological information of the dynamics, and these relevant parameter values can be used for further refinement in formal parameter estimation based on the available data, which already needs relatively good initial guesses of parameters to even begin.
ACKNOWLEDGEMENTS
Dengue surveillance data were provided by the Bureau of Epidemiology, Department of Disease Control, Ministry of Public Health, Thailand. This work was supported by the European Union under FP7 in the DENFREE project and the Portuguese FCT project PTDC/MAT/115168/2009.
DECLARATION OF INTEREST
None.