Impact statement
This review provides a snapshot in time covering some of the most exciting and innovative uses of aerial drone technology for mapping and capturing data in coastal ecosystems. We discuss how several of the defining characteristics of coasts make them particularly well-suited to drone technologies. For example:
-
1. Coasts are inherently spatially heterogeneous, but drones can acquire data with unprecedented detail from low altitudes, capturing the heterogeneity at multiple scales.
-
2. Coastal ecosystems are structurally complex, which is both a cause and effect of various processes including erosion and deposition. The structural complexity can be modeled in three dimensions using what is now considered routine computer processing of drone mapping data to further understand coastal environments and their associated biophysical processes.
-
3. Coastal environments are temporally dynamic and constantly changing in response to environmental conditions, but drones can be flown on flexible time schedules to capture data and account for this.
-
4. Coasts support many lives and livelihoods, which makes them the perfect breeding ground for democratizing technology such as drones to take flight.
In reviewing current applications of drone technology, combined with the growth trajectory and emerging trends in technology more broadly, we offer perspectives for the future of using drones in coastal ecosystems. We suggest that advances in sensor engineering, platform availability, and automation in terms of data capture and processing are most likely to be game-changing technologies over the next five years. While the technology advances will be instrumental in enabling broader adoption of drones and their data, exciting and transformative change in coastal monitoring and management is also likely to occur in local communities from a grassroots level. It is the combination of technology with people that will drive sustainable lives and livelihoods in coastal environments for the future.
Introduction
With nearly half of the global population living within 100 km of a coastline, it comes as no surprise that these regions are important for a wide range of environmental, sociocultural, and economic reasons. At the interface between land and sea, coastal environments support coral reefs, seagrass beds, mangrove and kelp forests, and the many species inhabiting those ecosystems. Their beaches, foreshores, permafrost, and rocky cliffs are host to a myriad of cultural and social activities, promoting health and well-being in the local community (Nicu et al., Reference Nicu, Rubensdotter, Stalsberg and Nau2021; Satariano, Reference Satariano2021). Coasts are also at the forefront of tourism, real estate, and international trade. However, sometimes the importance of these ecosystems and our heavy reliance on them means that we may be loving our coasts to death.
Heavy reliance on any resource will inevitably place pressures upon it. In coastal ecosystems, we see the compounding effects of primary anthropogenic pressures with secondary impacts related to climate change. In fact, Williams et al. (Reference Williams, Watson, Beyer, Klein, Montgomery, Runting, Roberson, Halpern, Grantham, Kuempel, Frazier, Venter and Wenger2021) suggest that only 15.5% of global coastlines have low levels of human pressure and that more than 50% of most countries’ coasts are degraded. This degradation may take the form of shoreline change (including erosion and deposition) (Splinter and Coco, Reference Splinter and Coco2021); sea level rise (Cazenave et al., Reference Cazenave, Palanisamy and Ablain2018); impacts from natural disasters such as cyclones (Fabricius et al., Reference Fabricius, De’ath, Puotinen, Done, Cooper and Burgess2008), earthquakes (Hart et al., Reference Hart, Pitman and Byun2020); tsunamis (Marras and Mandli, Reference Marras and Mandli2021); increased runoff and pollution (Kroon et al., Reference Kroon, Thorburn, Schaffelke and Whitten2016); as well as the presence of marine debris (Olivelli et al., Reference Olivelli, Hardesty and Wilcox2020). Yet in spite of – or perhaps because of – the significant challenges faced in coastal regions, they remain areas of population growth and connection, with considerable opportunities for building sustainable lives and livelihoods into the future.
Of the many exciting opportunities for and with coastal ecosystems, many focus on innovations that will hopefully result in greater quality of life for their local communities. These opportunities are generally linked to deriving greater benefit from the vast number of ecosystem services that coastal regions are recognized as providing (Heckwolf et al., Reference Heckwolf, Peterson, Jänes, Horne, Künne, Liversage, Sajeva, Reusch and Kotta2021). For example, mangroves, salt marshes, and seagrass ecosystems have become the foundation of blue carbon economies (Bertram et al., Reference Bertram, Quaas, Reusch, Vafeidis, Wolff and Rickels2021), while permafrost-rich Arctic coastlines store >1,300 Pg of organic carbon, Earth’s largest terrestrial carbon sink for centuries (Tanski et al., Reference Tanski, Bröder, Wagner, Knoblauch, Lantuit, Beer, Sachs, Fritz, Tesi, Koch, Haghipour, Eglinton, Strauss and Vonk2021). There is enormous potential to incorporate innovative engineering, urban planning, and policy frameworks to assist vulnerable coastal communities facing the impacts of climate change, particularly in developing countries with the greatest need for adaptation strategies (Le, Reference Le2020). We also acknowledge that there is a growing need for cross-cultural comanagement to recognize the value First Nations people bring in understanding coastal systems (Ireland, Reference Ireland2021) and how traditional practices can enhance and sustain local industries such as aquaculture and fisheries (Anbleyth-Evans et al., Reference Anbleyth-Evans, Hiriart-Bertrand, Leiva, Iwama and Henriquez-Antipa2022). To determine the enduring benefit of responding to such opportunities, and to discover new opportunities, it is critical to ensure the ecosystems are appropriately monitored and to allow management intervention to be triggered if and when required.
Remote sensing technologies have supported coastal management practices by providing baseline and ongoing monitoring data for decades (Splinter et al., Reference Splinter, Harley and Turner2018; Román-Rivera and Ellis, Reference Román-Rivera and Ellis2019; Kennedy et al., Reference Kennedy, Roelfsema, Lyons, Kovacs, Borrego-Acevedo, Roe, Phinn, Larsen, Murray, Yuwono, Wolff and Tudman2021). In addition to the many freely available optical satellite datasets (e.g., Landsat and Sentinel), increasing opportunities are arising to incorporate high-fidelity aerial drone imagery into mapping, modeling, monitoring, and management routines (Joyce et al., Reference Joyce, Duce, Leahy, Leon and Maier2018). With more than five million drones shipped globally in 2020 alone (Laricchia, Reference Laricchia2022), there are more drones in circulation than kilometers of coastline around the world. It is therefore timely to document some of the most exciting trends in drone data collection in coastal ecosystems.
Drone applications in coastal ecosystems
To situate this review in the recent literature (past 10 years), we conducted a systematic search of published and in press peer-reviewed journal articles using the Scopus database. We included all articles with the following words contained within their abstract, keywords, or title:
-
1. “Coast*” (wildcard used to include coasts, coastline, coastal); AND
-
2. “Drone*” (wildcard used to include plural); OR
-
3. “Unmanned” or “uncrewed” or “unoccupied” or “remotely piloted” “a*” (wildcard used to include aircraft or aerial, rather than underwater).
This returned 778 articles at the time of searching in May 2022. For reference, the same search conducted for “drone” (or variants of this word as above) without including “coast*” returned 32,846 articles. Thus the coastal research and application component represents approximately 2% of the broader discipline. The coastal drone research publications have been derived from a diverse 78 countries, though more than 50% originate from just four countries: the United States (n = 199), China (n = 74), Italy (n = 69), and Australia (n = 68) as determined by first author location. Note that this does not necessarily indicate the study site location.
Evaluating the keywords as nominated by the authors (Figure 1) reveals that the most common applications for using drones in coastal environments include for erosion (n = 85); animals (n = 54); environmental monitoring (n = 50); landforms (n = 45); and vegetation (n = 44). There is a clear clustering of words associated with photogrammetry including structure from motion (n = 56); digital elevation model (n = 62); Digital Terrain Model (n = 16); and 3D modeling (n = 11). Many of these terms are likely to also be associated with the coastal erosion theme as they provide supporting data or products to evaluate morphology, erosion, and deposition.
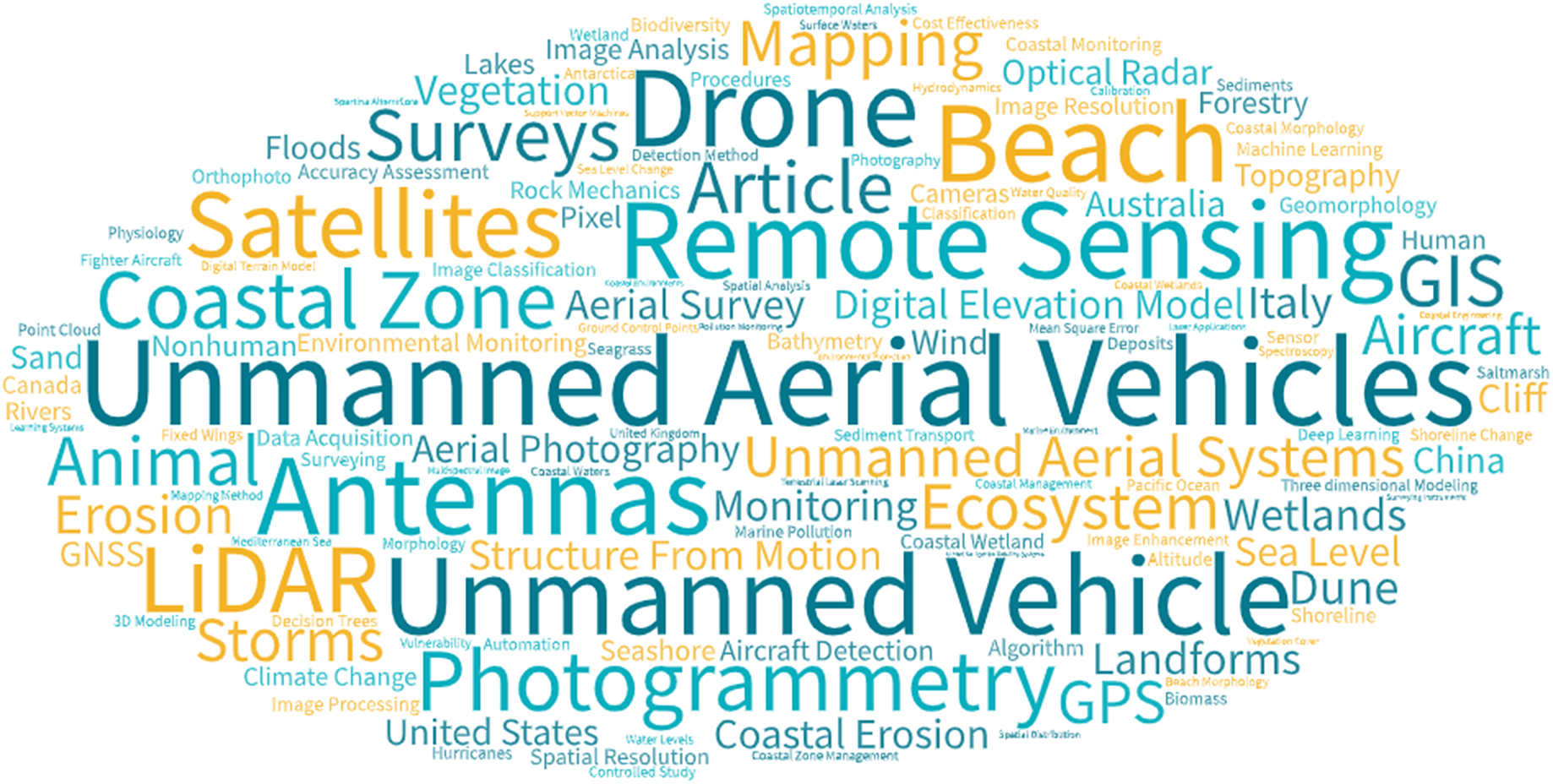
Figure 1. Keywords within peer reviewed articles related to coastal drone applications. Size of the word or phrase is relative to the number of papers using that term within their keywords, i.e. larger words indicate a higher frequency of use. Note also the movement towards using gender neutral language and favouring ‘uncrewed’ or ‘unoccupied’ rather than ‘unmanned’ (Joyce et al., Reference Joyce, Anderson and Bartolo2021) will see a change in the prevalence of these search terms in the near future.
Using a subset of the literature extracted from that identified in the above meta-analysis, combined with more specific searches, the following sections delve deeper into the unique value proposition that aerial drones offer as data collection tools specifically for mapping coastal ecosystems.
Why are drones so well-suited for coastal applications?
Notwithstanding the significant value that satellite and other airborne based (such as balloons and aircrafts) remote sensing offers for monitoring coastal ecosystems, aerial drones as a data collection platform have a unique value proposition. With a platform significantly closer to the features of interest (typically less than 120 m or 400 ft compared to several thousand feet for traditional survey aircraft and hundreds of kilometers for a satellite), drones capture imagery at least an order of magnitude higher in spatial resolution (<5 cm) than achievable with traditional survey and commercial satellites (between 30 cm to >1 km). This is of particular importance in narrow coastal zones where the features to be monitored are often small and heterogeneous (Figure 2a). Drones can also be used to model the three-dimensional structure of coastal ecosystems and their components using structure from motion (SfM) photogrammetry software (Figure 2b; Casella et al., Reference Casella, Rovere, Pedroncini, Mucerino, Casella, Cusati, Vacchi, Ferrari and Firpo2014, Reference Casella, Rovere, Pedroncini, Stark, Casella, Ferrari and Firpo2016, Reference Casella, Collin, Harris, Ferse, Bejarano, Parravicini, Hench and Rovere2017, Reference Casella, Drechsel, Winter, Benninghoff and Rovere2020; Lowe et al., Reference Lowe, Adnan, Hamylton, Carvalho and Woodroffe2019). For instance, drone-collected high-resolution orthomosaics and digital terrain models (DTMs) enable improved characterization of dynamic landscapes and volumetric changes such as those experienced by coastal systems after a storm or urban development (see Lowe et al., Reference Lowe, Adnan, Hamylton, Carvalho and Woodroffe2019). Further, aligning the ideal tide with optimal sun angle and environmental conditions creates a narrow window of opportunity appropriate for capturing mapping data, often not achievable with satellites, but possible with drones (Joyce et al., Reference Joyce, Duce, Leahy, Leon and Maier2018; Figure 2c). Drones are therefore flexible in terms of the types of data they can capture, as well as the ways in which they can be used. It is this very flexibility that has contributed to the wide uptake of drones as democratizing technology for remote sensing and mapping more broadly. Given the high density of population along our coastlines (e.g., Figure 2d), there are more than enough people and drones to capture their stories. The ability of drones to address the combination of spatial heterogeneity, structural complexity, temporal dynamics, and population hubs makes them truly valuable as data collection tools in coastal environments.
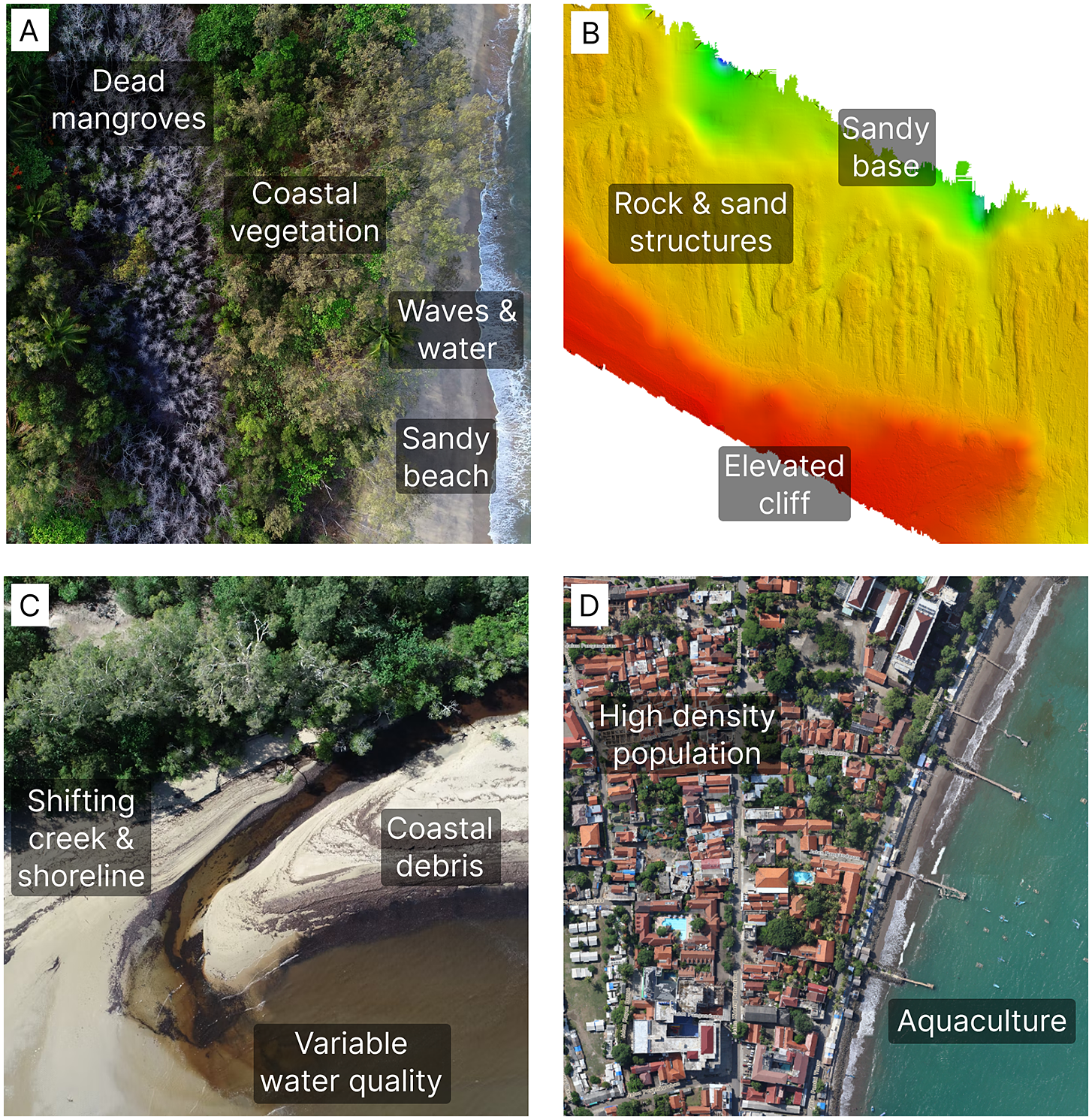
Figure 2. a) Spatial heterogeneity at various scales demonstrating that a small area can cover many different features and microhabitats (Joyce, Reference Joyce2020); b) Structural complexity of a rocky and sandy coastline seen in a digital terrain model (DTM) constructed using drone imagery (Joyce, 2019); c) Dynamic tidal flows and shifting coastal sands following a large storm event (Joyce, Reference Joyce2021); and d) Many lives and livelihoods are supported in this densely populated Indonesian coastal town (Nugroho, Reference Nugroho2016).
Coastal ecosystems are spatially heterogeneous
From individual corals to entire shorelines, coastal ecosystems vary widely in their spatial scale and heterogeneity both within the broader coastal zone (i.e., landscape heterogeneity) and within ecosystems (e.g., species diversity). The ability to dynamically scale both spectral and spatial resolutions in a way that coarser-scale satellite sensors cannot, makes drones an ideal technology to capture variability within the coastal zone.
Among the most endangered ecosystems on the planet due to climate change (Hughes et al., Reference Hughes, Baird, Bellwood, Card, Connolly, Folke, Grosberg, Hoegh-Guldberg, Jackson, Kleypas, Lough, Marshall, Nyström, Palumbi, Pandolfi, Rosen and Roughgarden2003), coral reefs hold tremendous biodiversity (Plaisance et al., Reference Plaisance, Caley, Brainard and Knowlton2011) and the spatial complexity of these habitats can indicate such diversity (Richards, Reference Richards2013). Mapping and monitoring coral reef heterogeneity with remote sensing technology helps enable informed management action at fine spatial scales (Roelfsema et al., Reference Roelfsema, Phinn, Jupiter, Comley and Albert2013; Bennett et al., Reference Bennett, Younes and Joyce2020; Nolan et al., Reference Nolan, Kim, Hoegh-Guldberg and Beger2021). For example, Bennett et al. (Reference Bennett, Younes and Joyce2020) used drone-collected red, green, and blue (RGB) imagery to identify and classify coral reefs at a 0.5 cm spatial scale in the southern Great Barrier Reef, allowing insight into the spatial distribution of coral habitat features, and in particular identifying live coral. This level of detail is not possible with satellite data where the inherent heterogeneity occurs at a subpixel level.
Similar to coral reefs, high levels of accuracy and precision in mapping seagrass meadows and kelp forests have been difficult to achieve with satellite imagery due to the complex mixture of both aquatic and vegetative spectra and timing of image capture (O’Neill and Costa, Reference O’Neill and Costa2013; Reshitnyk et al., Reference Reshitnyk, Costa, Robinson and Dearden2014; Cavanaugh et al., Reference Cavanaugh, Cavanaugh, Bell and Hockridge2021; Mora-Soto et al., Reference Mora-Soto, Capsey, Friedlander, Palacios, Brewin, Golding, Dayton, Van Tussenbroek, Montiel, Goodell, Velasco-Charpentier, Hart, Macaya, Pérez-Matus and Macias-Fauria2021). However, drones have allowed researchers to more accurately map these ecosystems with increased spatial resolution and explore how biotic and abiotic environmental variability influences classification (Nahirnick et al., Reference Nahirnick, Reshitnyk, Campbell, Hessing-Lewis, Costa, Yakimishyn and Lee2019).
Augmented by the increased spatial resolution drones provide, advanced classification techniques, such as machine and deep learning also add enhanced contextual information (Hamylton et al., Reference Hamylton, Morris, Carvalho, Roder, Barlow, Mills and Wang2020a), including mapping down to the individual organism level in sea cucumber populations (Li et al., Reference Li, Duce, Joyce and Xiang2021). When increasing spatial resolution alone is not enough to map and monitor heterogeneous coastal ecosystem features, advanced data types such as hyperspectral (Cornet and Joyce, Reference Cornet and Joyce2021; Jaud et al., Reference Jaud, Sicot, Brunier, Michaud, Le Dantec, Ammann, Grandjean, Launeau, Thouzeau, Fleury and Delacourt2021) and Light Detection and Ranging (LiDAR) (Kramer et al., Reference Kramer, Mücher and van der Hagen2021) can be employed and are especially useful on drones due to their cost reduction and advances in sensor miniaturization in current technology.
Coastal ecosystems are structurally complex
In coastal ecosystems, fine-scale, three-dimensional structural variability can act as an indicator for ecosystem health (Rombouts et al., Reference Rombouts, Beaugrand, Artigas, Dauvin, Gevaert, Goberville, Kopp, Lefebvre, Luczak, Spilmont, Travers-Trolet, Villanueva and Kirby2013), restoration success (Levin and Talley, Reference Levin and Talley2002), and vulnerability to climate hazards (van Loon-Steensma et al., Reference van Loon-Steensma, Hu and Slim2016). In salt marshes, for example, both landscape and individual vegetation structures are important parameters in assessing condition (Morzaria-Luna et al., Reference Morzaria-Luna, Callaway, Sullivan and Zedler2004). As a low-cost way to measure salt marsh structure, DiGiacomo et al. (Reference DiGiacomo, Bird, Pan, Dobroski, Atkins-Davis, Johnston and Ridge2020) found that combining multispectral drone imagery with SfM modeling, achieved similar accuracy and higher consistency when compared to higher-cost, crewed LiDAR data of vegetation height predictions in coastal salt marsh sites and with significantly finer spatial resolution compared to satellite imagery. From measures of structure through drone-collected data, biophysical parameters (e.g., biomass) can then also be quantified (Doughty and Cavanaugh, Reference Doughty and Cavanaugh2019). These techniques also scale up forested wetlands, such as the coastal swamp oak forest (Conroy et al., Reference Conroy, Hamylton, Kumbier and Kelleway2022) and mangroves (Jones et al., Reference Jones, Raja Segaran, Clarke, Waycott, Goh and Gillanders2020). Scaling down from wetlands, with increased spatial resolution drones can also help map the structure of fine-scale features and ecosystems such as coral reefs (Casella et al., Reference Casella, Collin, Harris, Ferse, Bejarano, Parravicini, Hench and Rovere2017, Reference Casella, Lewin, Ghilardi, Rovere and Bejarano2022) and oyster reefs (Windle et al., Reference Windle, Puckett, Huebert, Knorek, Johnston and Ridge2022).
In addition to mapping ecological features, drone mapping images can be processed using SfM photogrammetry techniques to build digital terrain models (DTMs) and digital surface models (DSMs). This enables fine-scale, three-dimensional information to help map abiotic features in coastal systems both on land and underwater (Casella et al., Reference Casella, Drechsel, Winter, Benninghoff and Rovere2020; Alevizos and Alexakis, Reference Alevizos and Alexakis2022; Kreij et al., Reference Kreij, Scriffignano, Rosendahl, Nagel and Ulm2018), including tracking topographic change over time (Casella et al., Reference Casella, Rovere, Pedroncini, Stark, Casella, Ferrari and Firpo2016; Lowe et al., Reference Lowe, Adnan, Hamylton, Carvalho and Woodroffe2019; Volpano et al., Reference Volpano, Zoet, Elmo Rawling and Theuerkauf2022). Combining the digital elevation models (DSM or DTM) with an RGB orthomosaic of the region provides a unique perspective of coastal ecosystems that is simpler and more cost-effective to create than using more traditional aerial survey and satellite techniques.
Coastal ecosystems are temporally dynamic
Coastal ecosystems are the nexus between the world’s terrestrial landscapes and the open ocean and therefore subject to the dynamics of upland and upstream processes as well as offshore oceanic drivers. Situated in a transitional ecological matrix, coastal ecosystems often experience fine-scale temporal variability ranging from hourly and daily tidal regimes or real-time emergency hazards, such as tsunamis and storm surges originating offshore, to water quality emergencies that emerge from adjacent urban or freshwater areas. With their ability to be deployed near-instantaneously and with greater flexibility, drones are an excellent resource to document time-sensitive processes in coastal zones (Hart et al., Reference Hart, Pitman and Byun2020). Using drones, researchers, scientists, and local resource managers are able to capture data when and where they need. For example, to monitor intertidal reefs, Murfitt et al. (Reference Murfitt, Allan, Bellgrove, Rattray, Young and Ierodiaconou2017) chose to collect imagery exclusively during low tide to increase the spatial area of the exposed intertidal reef; a temporal versatility not afforded by the majority of satellite sensors. Similar flexibility also allows for better control over environmental parameters that introduce noise into spectral reflectance measurements such as clouds, which can be a particular problem for optical satellite sensors in temporally sensitive ecosystems (Whitcraft et al., Reference Whitcraft, Vermote, Becker-Reshef and Justice2015), and sun glint (Nahirnick et al., Reference Nahirnick, Reshitnyk, Campbell, Hessing-Lewis, Costa, Yakimishyn and Lee2019; Giles et al., Reference Giles, Davies, Ren and Kelaher2021; Windle and Silsbe, Reference Windle and Silsbe2021).
In temperate regions, permafrost thawing has caused rapid shifts in Arctic coastlines, mobilizing large amounts of sediments, organic matter, and nutrients from the permafrost (Wegner et al., Reference Wegner, Bennett, Vernal, de Forwick, Fritz, Heikkilä, Łacka, Lantuit, Laska, Moskalik, O’Regan, Pawłowska, Promińska, Rachold, Vonk and Werner2015). Optical satellite image coverage in high-latitude regions has been historically limited to coarse temporal and spatial resolution. Instead, frequent use of lightweight drones coupled with image-based modeling in such environments has allowed land managers to self-service their data needs, providing timely information on rapidly changing features (Cunliffe et al., Reference Cunliffe, Tanski, Radosavljevic, Palmer, Sachs, Lantuit, Kerby and Myers-Smith2019). In challenging environments, researchers and practitioners are turning to drones to meet their time-sensitive data acquisition needs.
In time-sensitive coastal emergencies, drones can be deployed instantaneously to aid in activities like aquatic search and rescue (Burke et al., Reference Burke, McWhirter, Veitch-Michaelis, McAree, Pointon, Wich and Longmore2019; Del-Real and Díaz-Fernández, Reference Del-Real and Díaz-Fernández2021), sharks tracking for coastal recreation risk assessment (Stokes et al., Reference Stokes, Apps, Butcher, Weiler, Luke and Colefax2020), or monitoring water quality emergencies such as oil spills and harmful algal blooms (Filippi et al., Reference Filippi, Hanlon, Rypina, Hodges, Peacock and Schmale2021; Windle and Silsbe, Reference Windle and Silsbe2021). As these emergencies dynamically unfold, drones are ideally suited to capture relevant data, allowing relevant authorities to act on timely data-driven decisions. Drones, therefore, provide support to first responders by uniquely providing time-sensitive data in dynamic situations.
Coastal ecosystems support many lives and livelihoods
An estimated 600+ million people live within 10 m of sea level (Neumann et al., Reference Neumann, Vafeidis, Zimmermann and Nicholls2015) placing both tremendous pressure as well as value on coastal ecosystems. As the hub of many population centers and travel destinations, this means that coasts also provide a critical mass of people with the ability to undertake grassroots monitoring of their local environments. This can take place within independent groups, or supported by a variety of citizen science initiatives that are popular in easily accessible locations (Kelly et al., Reference Kelly, Fleming, Pecl, von Gönner and Bonn2020). Thus coastal regions hold a combination of a suitably sized population base to support activated and environmentally conscious citizens with readily accessible drone technology that is ideally suited to address coastal challenges.
Understanding that drones allow people to take remote sensing and mapping into their own hands, it would be remiss of our review to exclude the applications absent from – and perhaps rarely captured – within the peer-reviewed literature. For example, many First Nations groups are using drones as part of their standard practice to monitor and manage their land and sea country. Most First Nations drone experiences occurred since 2014 and were often located in developing countries, particularly South and Central America (Vargas-Ramírez and Paneque-Gálvez, Reference Vargas-Ramírez and Paneque-Gálvez2019). They are typically using drones for coastal erosion, damage caused by feral animals and development, documenting sites of significant cultural heritage, weeds, habitat mapping, and fire monitoring.
Drones have also been used to help Indigenous communities assess the risk from climate change and mapping land ownership. For example, the Guna, an Indigenous people who live along the Panamanian coast, are facing rapid land loss due to sea level rise. They used drones to identify targets for the potential relocation of their homes. In Cebu Province, Philippines in 2016, several communities used multirotor drones to formalize land ownership rights by facilitating the identification of plots and resolving local disputes related to the property (Pablo and Petzold, Reference Pablo and Petzold2016). Many of these applications have been identified above as well-suited to drone technology, and local Ranger networks are now using the technology as part of their standard operations. The democratizing nature of drone technology is therefore returning power to these groups to document landscapes from their point of view.
Personnel within the military are also less likely to contribute in great detail to the peer-reviewed literature, but still hold considerable infrastructure and expertise related to coastal applications of drones. Relevant military operations are often focused on developing situational awareness associated with the littoral zone as a subset of the coastal environment. Drones are ideally suited to capturing data in this narrow region on a rapid and as-needs basis. They also have the added benefit of providing sovereign data suitable for creating highly detailed digital elevation and slope models to inform troop and vehicular movement plans.
What are the challenges with using drones?
Throughout this review, we have discussed how drone-based remote sensing offers significant possibilities to acquire data for coastal applications in a fast and relatively cost-effective way. Yet as with all technology, drones are not necessarily the ideal solution under all scenarios. They come with their own range of challenges that we encourage readers to consider prior to building a drone capability. Paradoxically, the very benefits presented above can also be the real challenges in their uptake. We present these considerations within three categories based on logistics, finance, and expertise.
Drone data capture is not always simple
While capturing data is often not a challenge, capturing analysis-suitable data requires drone operators to be cognisant of a range of environmental and logistical challenges. For example, local regulations vary and often require consultation with aviation authorities, governments and councils, and Indigenous land custodians. The legislation, licensing, and compliance requirements continue to change and in many cases are not transferable across jurisdictions. Following approvals to operate, as with most passive remote sensing, the quality of drone data is highly dependent on the local weather conditions. Specific to coastal ecosystems, Duffy et al. (Reference Duffy, Cunliffe, DeBell, Sandbrook, Wich, Shutler, Myers-Smith, Varela and Anderson2018) note that drones are sensitive to complex sea breezes and salt spray, while tides and changing water levels can mean that finding suitable take-off and landing sites is difficult. The effects of the long-standing challenge with sunglint when imaging over water bodies (Duffy et al., Reference Duffy, Cunliffe, DeBell, Sandbrook, Wich, Shutler, Myers-Smith, Varela and Anderson2018; Joyce et al., Reference Joyce, Duce, Leahy, Leon and Maier2018; Giles et al., Reference Giles, Davies, Ren and Kelaher2021) is also amplified in high spatial resolution imagery such as that captured by a drone. Coarser resolution satellite imagery tends to smooth out sunglint effects, however, they are clearly apparent in drone imagery, acting to obscure or distort subsurface features. Careful timing and planning can reduce the impact of these complications on resultant data.
Thorough planning is also necessary to ensure adequate site coverage. While drones can cover a greater area than in situ field survey (in the order of several hectares rather than m2), they are still limited by the operator’s ability to keep the drone within line of sight (regulation requirement), and the drone battery life. Although battery technology is constantly improving, flight durations for the most readily available consumer drones are typically limited to <40 min (or longer for fixed-wing platforms). To increase the area coverage within that time, the operator can choose to fly at a higher altitude (usually limited by the regulations of up to 400 ft, or 120 m), but will compromise the spatial detail in doing so. Thus drones are often better suited to local scale mapping and monitoring rather than regional or national programs.
Drones are not always a cheap alternative
It is imperative to consider the full scope of the project and data needs before estimating the budgetary requirements for building a drone capability. While the initial outlay for a drone platform with mapping capabilities might be as low as US$500, large and more advanced platforms are found in the order of thousands or tens of thousands of dollars. In addition, incorporating advanced sensors such as those equipped with hyperspectral, LiDAR, or thermal capabilities will further increase the cost of acquisition, sometimes well over $100,000. Alongside the hardware costs, annual software license fees for data processing and storage solutions need to be taken into account. There are however open-source options for data processing, and cloud storage is constantly reducing in price.
Drone data does not equate to knowledge
The number of research papers referenced in this review alone attests to the many diverse challenges in translating drone data into knowledge and actionable insights. From the variety of software packages and number of parameters to consider even in the preprocessing stages of photogrammetry (Pell et al., Reference Pell, Li and Joyce2022), deriving analysis-ready data such as orthomosaics and digital elevation models is not always a simple process. After conducting the initial processing, users need to develop skills in manipulating large volumes of data, building reliable and reproducible workflows, and translating downstream products to communicate their meaning. Data capture therefore often becomes the simplest aspect of a workflow while operationalizing the opportunity of drones and meaningful insights present the real challenge.
Future perspectives for using drones in coastal ecosystems
After reflecting on the status of using aerial drones as data collection tools in coastal environments, there have been significant advances in the past five years. This is the period where peer-reviewed publications on the topic started to grow considerably. So, while it may be difficult to forecast the future of the technology given the rate of growth, there are some clear emerging trends that will generate exciting applications and new perspectives for coastal ecosystems over the next five years. Our understanding of the various coastal features and processes currently targeted for mapping will benefit from a variety of drone and technology enablers (Figure 3). We consider these key enablers below within the context of our four grounding statements, being that coastal ecosystems are spatially heterogeneous; are structurally complex; have fine-scale temporal dynamics; and support many lives and livelihoods.

Figure 3. Range of coastal features and processes currently targeted for mapping activities as key indicators of ecosystem health, surrounded by the drone and associated technology components identified as significant enablers of future directions in understanding coastal ecosystems.
Spatial heterogeneity at all scales remains a challenge, but also provides many opportunities for technology development in coastal ecosystems. While camera systems are generally well-suited to capture heterogeneity, we will continue to see higher quality sensor systems including those with multispectral and hyperspectral capabilities penetrate the market due to consumer demand. However, the greatest growth trajectory lies in developing software and algorithms to process these data in a timely manner. With increasing data resolution (spatial, spectral, temporal domains), manual interpretation will no longer be an option. The ability to ingest and fuse large volumes of data, in combination with machine and deep learning analytics will be instrumental in making informed decisions in coastal ecosystems.
As climate change models predict we could lose up to half of the world’s sandy beaches by the end of this century (Vousdoukas et al., Reference Vousdoukas, Ranasinghe, Mentaschi, Plomaritis, Athanasiou, Luijendijk and Feyen2020), and erosion rates are exacerbated along Arctic coastal landscapes with shrinking ice cover, storm surge effects, and thawing permafrost (Irrgang et al., Reference Irrgang, Lantuit, Gordon, Piskor and Manson2019), the onus is on scientists, environmental managers, and policymakers to ensure we are making the right data-driven decisions for communities affected. Three-dimensional models of topography are a fundamental component of this supporting data. While drones already enable us to capture much-needed information to document structural and morphological changes along coastlines (Splinter et al., Reference Splinter, Harley and Turner2018; Lowe et al., Reference Lowe, Adnan, Hamylton, Carvalho and Woodroffe2019; Hamylton et al., Reference Hamylton, Nurdin, Carvalho, Jompa, Akbar, Fitrah, Lanuru and Amri2020b), the sensors acquiring these data are becoming more sophisticated and accurate. General consumers are already able to enjoy LiDAR technology in some of the latest handheld mobile devices, so it is likely that this miniaturized level of technology will become available on drone platforms as well, replacing some of the heavier equipment currently available. This will greatly supplement photogrammetry software techniques that are commonly used for generating 3D models, but are limited by vegetation cover. Real-time kinematic (RTK) global navigation satellite systems (GNSS) have also started to move into the consumer-grade drones, enhancing the positional accuracy of captured imagery. As that becomes more widespread, digital elevation models will have significantly higher levels of accuracy in x–y–z domains which are critical to accurately document shoreline changes.
However, thoroughly understanding shoreline change requires more than 3D information. Here, frequent and repeated data capture becomes a fundamental component to document and predict change. This is where the growth of the drone market comes in. Current projections suggest there may be a fourfold increase in the drone market by the end of the decade, placing many more drones in circulation for both personal and commercial use. This opens opportunities for increased frequency of data capture.
Yet many drone operators capturing data still may not create the transformative change possible in monitoring fine-scale coastal dynamics. Instead, the growing number of operational drones (e.g., delivery platforms) equipped with sensors that can also capture timely mapping data as a secondary purpose is an even larger market opportunity. These platforms are long-range, can fly beyond visual line of sight (BVLOS), and spend extended periods in the air. This means that they can cover vast areas and capture huge amounts of data in a near continuous manner. Coupling this technology with what has been termed “drone in a box” solutions placed in strategic coastal locations of interest, the future of coastal monitoring with drones becomes increasingly autonomous.
As we capture significantly more data, we must also look to the opportunities for data storage, autonomous data processing, and data or information sharing. Amazon Web Services (AWS), Microsoft, and Google compete strongly for dominance in cloud storage and processing services, and the rise of Google Earth Engine, Microsoft Planetary Computer, and associated applications has helped process and reprocess vast quantities of open satellite remote sensing data. As developers create new web and mobile applications off the back of these services, this opens opportunities for coastal managers and decision-makers to derive insights from remote sensing data without needing to conduct the analysis themselves (Dinerstein et al., Reference Dinerstein, Joshi, Vynne, Lee, Pharand-Deschênes, França, Fernando, Birch, Burkart, Asner and Olson2020). With drone data considered relatively new to the portfolio of remote sensing options, processing data and delivering information in this manner is currently in its infancy and being tested by early adopters (e.g., Bennett et al., Reference Bennett, Younes and Joyce2020). Developing and operationalizing these types of applications with robust artificial intelligence algorithms tuned to and driven by drone data will result in even greater uptake in drone technology in coastal ecosystems. The future of interacting with drone data in this manner will be available on the cloud, with hybrid cloud, or edge computing solutions, resulting in rapid information flow to the people and systems needing it most.
Although we may head toward greater autonomy, we must not underestimate the role of people and community. We have seen how drones have become democratizing technology that is readily available to communities around the world, even in highly regional and remote areas (Vargas-Ramírez and Paneque-Gálvez, Reference Vargas-Ramírez and Paneque-Gálvez2019; Colloredo-Mansfeld et al., Reference Colloredo-Mansfeld, Laso and Arce-Nazario2020; Álvarez Larrain et al., Reference Álvarez Larrain, Greco and Tarragó2021). In 2017, communities in the Maabaidhoo Island, Maldives, used multirotor drones to better prepare for challenges posed by climate change, by identifying eroded coastlines, developing risk maps, and restoring coral reef systems and mangroves (United Nations Development Programme, 2017). Coupled with a groundswell of interest in citizen science activities and community-led initiatives (Hecker et al., Reference Hecker, Haklay, Bowser, Makuch, Vogel and Bonn2018), drones and their data support people taking action in their local areas aligned with ecosystems they are passionate about (Colloredo-Mansfeld et al., Reference Colloredo-Mansfeld, Laso and Arce-Nazario2020; Merlino et al., Reference Merlino, Paterni, Locritani, Andriolo, Gonçalves and Massetti2021; Bunting et al., Reference Bunting, Theuerkauf, Rabins, Konsoer, Leitner and Lewis2022; Theuerkauf et al., Reference Theuerkauf, Bunting, Mack and Rabins2022). In 2022, Indigenous community members from the Hudson and James Bay Lowlands in northern Ontario, Canada were trained to use drones to document land use change across their respective communities. Encouraging and supporting these activities will promote sustainability, comanagement, and equitable decision-making about coastal ecosystems, by those who rely directly on them (Bax et al., Reference Bax, Novaglio, Maxwell, Meyers, McCann, Jennings, Frusher, Fulton, Nursey-Bray, Fischer, Anderson, Layton, Emad, Alexander, Rousseau, Lunn and Carter2022), while grounding data collection in FAIR (findable, accessible, interoperable, and reusable) principles will ensure the broader community can leverage these efforts.
Further, we must look to the role of First Nations people – in particular women – for their critical contributions in achieving sustainable, inclusive development, and climate resilience (Löw, Reference Löw2020; Hale et al., Reference Hale, Gerhardt, Day and Heron2022; Kandrot et al., Reference Kandrot, Hayes and Holloway2022) in line with the United Nations Sustainable Development Goals. An example of this is found in Guyana, South America where drones are used by Indigenous girls to map and monitor carbon stocks of mangroves for potential conservation designation near their community (Persaud, Reference Persaud2021). Given the importance of diversity in mapping and the considerable concerns of embedded biases if this is not taken into account (Gardner et al., Reference Gardner, Mooney, De Sabbata and Dowthwaite2020; Hsu et al., Reference Hsu, Pruckner, Satterthwaite, Weatherdon, Hadley and Nguyen2021), we cannot afford to disregard wide-ranging viewpoints and participation in mapping. Maintaining and enhancing practices of technology transfer from research to operational, and from areas of privilege to underserved communities will bring entirely new ways to work with coastal environments for enhanced lives and livelihoods in the future.
Conclusion
Global climate models predict that our coastal areas are facing challenging times ahead. We can either balk at these challenges or embrace the opportunities that arise as a consequence. Our review has clearly demonstrated that we have the people and technology projections to embrace innovations and provide new solutions to coastal ecosystem challenges. In the inevitably changing future, coasts will remain spatially heterogeneous, structurally complex, temporally dynamic, and supportive of many lives and livelihoods. We offer here that drones have a unique value proposition in monitoring and managing these multidimensional complexities for future sustainability. Supported by scalable analytics as well as cloud and edge infrastructure, drones will continue to play a role as data collection tools in monitoring and managing coastal ecosystems until such time that the next great technological advancement makes them obsolete.
Open peer review
To view the open peer review materials for this article, please visit http://doi.org/10.1017/cft.2022.7.
Data availability statement
The data that support the findings of this study are available from the corresponding author (K.E.J.) upon reasonable request.
Acknowledgements
Icons used to create Figure 3 courtesy Integration and Application Network (ian.umces.edu/media-library).
Author contributions
All authors have contributed substantially to every aspect of conceptualizing, researching, reviewing, and editing. All authors have read and agreed to the published version of the manuscript.
Financial support
This research received no specific grant from any funding agency, commercial, or not-for-profit sectors.
Competing interest
The authors declare none.
Comments
No accompanying comment.