Low-carbohydrate diets (LCD), which restrict carbohydrate in favour of increased protein or fat intake, or both, have recently received great interest and become a popular strategy for short-term weight loss(Reference Liu, Zhang and Ye1). An increasing number of randomised controlled trials and meta-analyses showed improvements in body weight and multiple markers of cardiometabolic health, including lowering fasting glucose, homoeostatic model assessment for insulin resistance (HOMA-IR), systolic and/or diastolic blood pressure and TAG, after implementation of LCD for 6 months or longer in overweight(Reference Sackner-Bernstein, Kanter and Kaul2,Reference Meckling, O’Sullivan and Saari3) , obese(Reference Willems, Sura-de Jong and van Beek4,Reference Silverii, Cosentino and Santagiuliana5) and metabolically unhealthy populations(Reference Goldenberg, Day and Brinkworth6,Reference Angelidi, Papadaki and Nolen-Doerr7) .
Nonetheless, whether LCD can decrease LDL-cholesterol, a known risk factors for CVD, remains controversial. For example, several studies(Reference Mansoor, Vinknes and Veierod8,Reference Bueno, de Melo and de Oliveira9) found significant increase in LDL-cholesterol after following LCD, while some studies refuted these findings(Reference Ebbeling, Knapp and Johnson10,Reference Gjuladin-Hellon, Davies and Penson11) . Besides the dietary composition of carbohydrate, the quality and types of macronutrients may play an important role in human health(Reference Ludwig, Willett and Volek12–Reference Shan, Guo and Hu14). For example, intakes of whole grains, legumes and fruits were associated with lower risk of CVD or mortality(Reference Aune, Keum and Giovannucci15–Reference Wang, Ouyang and Liu17), whereas high intakes of carbohydrates from refined grains and added sugars seemed harmful(Reference Lim, Tai and van Dam18–Reference Sawicki, Jacques and Lichtenstein20). Similarly, replacing saturated fat with unsaturated fat or replacing animal protein with plant protein was associated with reduced CVD incidence or CVD-related mortality(Reference Li, Hruby and Bernstein21–Reference Zeng, Li and Zhang24). These findings raise the question of whether the inconsistent results on LCD and lipid profile can be due to different food sources and type of macronutrients in the diet. In addition, there have been limited studies on the associations between LCD and markers of cardiometabolic risk in the US free-living population, although few observational studies(Reference Ha, Joung and Song25–Reference Nakamura, Ueshima and Okuda27) have examined the associations in East Asia, in which the average percentage of energy from carbohydrate intake was approximately 61 %, higher than this in the USA and Europe (∼50 %)(Reference Seidelmann, Claggett and Cheng28).
Herein, we aimed to evaluate the cross-sectional associations between LCD and plasma markers of cardiometabolic risk by considering quality and types of macronutrients in a free-living population from the US National Health and Nutrition Examination Survey (NHANES). LCD was defined according to the method suggested by Halton et al.(Reference Halton, Willett and Liu29) in the analysis. Moreover, healthy (emphasising unsaturated fat, plant protein and less low-quality carbohydrates) and unhealthy (emphasising saturated fat, animal protein and less high-quality carbohydrate) LCD scores were developed to distinguish between different dietary quality(Reference Shan, Guo and Hu14,Reference Li, Li and Zeng30) .
Methods
Study population
The NHANES programme includes a series of cross-sectional nationally representative health examination surveys, aiming to describe the health and nutritional status. About 5000 persons were recruited by the National Center for Health Statistics (NCHS) every year since 1999. Details on study protocol and data collection have been described elsewhere(31). Dietary information was collected by a single 24-h dietary recall from 1999 to 2002, and collected twice (i.e. two 24-h recalls) since 2003, in which the first 24-h recall was administered in person in the Mobile Examination Center (MEC), and the second recall was conducted by telephone 3–10 d later. In line with previous studies(Reference Li, Zeng and Wang32–Reference Li, Chen and Zhang34), we only included the 2003–2018 survey cycles with two 24-h recalls in the analysis. Participants were excluded if they were younger than 18 years old (n 32 549), had missing data on diet (n 5294), had implausible energy intake (< 2510 or > 14 644 kJ/d for women and < 3347 or > 17 573 kJ/d for men, n 1753), had only one 24-h dietary recall (n 4654) or did not have any biochemical data (n 1277, online Supplementary Fig. 1). No statistically significant differences in macronutrient intake between those with single 24-h dietary recall and those with two 24-h recalls (n 34 785, 88·2 %) were found. The NCHS ethics review board approved this study, and written informed consent was obtained from all participants.
Development of low-carbohydrate diet scores
The NHANES programme used multiple-pass method(35) to collect dietary data, which may improve the complete and accurate data collection and reduce the respondent burden. Nutrients were estimated using cycle-specific versions of the USDA Food and Nutrition Database for Dietary Studies(36). The National Cancer Institute method was used to estimate the usual intake of nutrients based on 2-d dietary recall, which can overcome several limitations inherent in the analysis of 24-h recall data(37). We used the percentage of energy from each macronutrient rather than absolute intake to reduce measurement errors and to represent the dietary composition(Reference Shan, Guo and Hu14).
The development of overall, healthy and unhealthy LCD was reported elsewhere(Reference Shan, Guo and Hu14,Reference Halton, Willett and Liu29) . Unlike clinical trials, it is difficult to dichotomise participants in observational studies into those who are on and those who are not on an LCD. Halton et al.(Reference Halton, Willett and Liu29) originally construct a system to classify participants according to their relative levels of fat, protein and carbohydrate intake and created a summary score designated the LCD score. In this analysis, participants were divided into eleven sex-specific strata by percentage of energy from carbohydrate, protein and fat, and their subtypes. Participants in the highest stratum of fat and protein intake were assigned 10 points, those in the lowest stratum were assigned 0 points. For carbohydrate, participants in the highest stratum were given 0 point, and those in the lowest stratum were given 10 points. Overall LCD score was obtained by summing up the scores of carbohydrates, fat and protein, which ranged from 0 to 30 points.
To distinguish between the quality and types of macronutrients in LCD, Shan et al. (Reference Shan, Guo and Hu14) further developed two types of LCD, healthy and unhealthy LCD, based on the food sources of carbohydrate (high-quality carbohydrate: carbohydrate from whole grains, whole fruit, legumes and non-starchy vegetables v. low-quality carbohydrate: carbohydrate from refined grains, added sugar, fruit juice, potato, other starchy vegetables and other sources) and types of fat (saturated fat v. unsaturated fat) and protein (plant protein v. animal protein). Specifically, healthy LCD score was calculated according to the percentages of energy from low-quality carbohydrate (reverse scoring), unsaturated fat (positive scoring) and plant protein (positive scoring), whereas unhealthy LCD score was calculated according to the percentages of energy from high-quality carbohydrate (reverse scoring), saturated fat (positive scoring) and animal protein (positive scoring). Similarly, plant-based LCD score was calculated according to percentages of energy from carbohydrate (reverse scoring), plant fat (positive scoring) and plant protein (positive scoring), and animal-based LCD score was calculated according to percentages of energy from carbohydrate (reverse scoring), animal fat (positive scoring) and animal protein (positive scoring, online Supplementary Table 1)(Reference Liu, Yang and VoPham38).
Measurement of biomarkers
Fasting blood samples were collected and processed in the MEC following the first 24-h recall and then were analysed at the CDC laboratory. The methods used to assay plasma markers, including insulin, C-peptide, C-reactive protein (CRP), TAG, total cholesterol (TC), HDL-cholesterol, LDL-cholesterol and apoB, were described elsewhere(39). HOMA-IR was calculated using the following formula: fasting serum insulin (µU/ml) × fasting plasma glucose (mmol/l)/22·5(Reference Wang, Chen and Zhang33,Reference Zhu, Peng and Lu40) . LDL-cholesterol levels were calculated based on levels of TC, TAG and HDL-cholesterol according to the method by Friedewald(Reference Freeman41).
Assessment of covariates
Socio-demographic and lifestyle information, such as age, sex, race/ethnicity, marital status, education, smoking status, alcohol drinking, physical activity and family income, was collected using self-reported questionnaire. Height and body weight were measured at the MEC by trained health technicians following standardised protocols. Physical activity was measured in metabolic equivalent tasks-h/week. Family income was measured by the ratio of family income to poverty. BMI was calculated by dividing body weight (kg) with squared height (m2). Hypertension, CVD and cancer were defined if participants had ever been told by a healthcare professional of such diseases and/or records of medication prescription due to these diseases. Individuals were considered as having diabetes if they had a self-reported history of diabetes or presented with HbA1c ≥ 6·5 % or fasting glucose ≥ 126 mg/dl.
Ethics approval
The NCHS approved the NHANES study protocol, and the written informed consent was obtained from all participants. Since this analysis used public dataset, human subjects’ approval was waived.
Statistical analysis
The 2-d dietary sample weights were applied to overcome the limitations of non-response and oversampling(42). All biomarkers were logarithmically transformed to normalise the distribution. We used multiple linear regression to examine the associations between LCD-adherence scores and the plasma levels of biomarkers. Model 1 was adjusted for survey cycle, age, sex, race/ethnicity, marital status, education level, ratio of family income to poverty, physical activity, smoking status, alcohol drinking, total energy intake, hypertension, diabetes, cancer, CVD, family history of CVD, family history of diabetes and survey cycle. Model 2 was additionally adjusted for BMI, considering the potential intermediate role of obesity. When examining inflammatory markers, we further adjusted for aspirin use in the analysis. A missing value indicator was created for each covariate if possible.
We presented percentage changes and 95 % CI in biomarker concentrations comparing LCD score in different categories with the reference group, expressed as (exp(β) − 1) × 100 %. Restricted cubic spline was used to assess the potential non-linear relationships between LCD scores and biomarker concentrations. Subgroup analysis was conducted by age, sex, race/ethnicity, education level, ratio of family income to poverty, smoking status, drinking status, physical activity, BMI and diabetes. Wald test was used to evaluate whether the cross-product terms between these subgroup variables and LCD were statistically significant.
In sensitivity analysis, we repeated analysis among participants who are free of major CVDs. In line with previous studies(Reference Willems, Sura-de Jong and van Beek4,Reference Frisch, Zittermann and Berthold43) , we also defined LCD as a diet that contains less than 40 % of energy from carbohydrates and investigated its associations with markers of cardiometabolic risk. All statistical tests were two-sided, and we conducted the statistical analyses using SAS version 9.4 (SAS Institute Inc, Cary, NC). Because of many tests being conducted, we used the Bonferroni correction to define the statistical significance as P < 0·001 (0·05/(5 exposures × 10 outcomes)) for main analysis and P < 0·0001 (0·05/(5 exposures × 10 outcomes × 9 groups)) for subgroup analysis allowing for multiple comparisons.
Results
Baseline characteristics
A total of 34 785 participants (mean age, 48·4 (sd 18·9) years) were included in the analysis. On average, about 49 % of energy intake was from carbohydrate, 35 % from fat and 16 % from protein. Participants with higher healthy LCD scores were older, had higher prevalence of diabetes, were better educated, had higher ratio of family income to poverty, were more physical active, were more likely to be current drinkers and less likely to be current smokers. Participants with higher unhealthy LCD scores were younger, had higher BMI and prevalence of diabetes, were less educated and physical active and were more likely to be current smokers (Table 1). The age-adjusted characteristics of the participants by quartiles of overall, plant-based and animal-based LCD scores are shown in online Supplementary Table 2.
Table 1. Age-adjusted characteristics of the participants by quartiles of healthy and unhealthy LCD in NHANES (2003–2018) (n 34, 785)*
(Percentages and interquartile ranges; mean values and standard deviations)
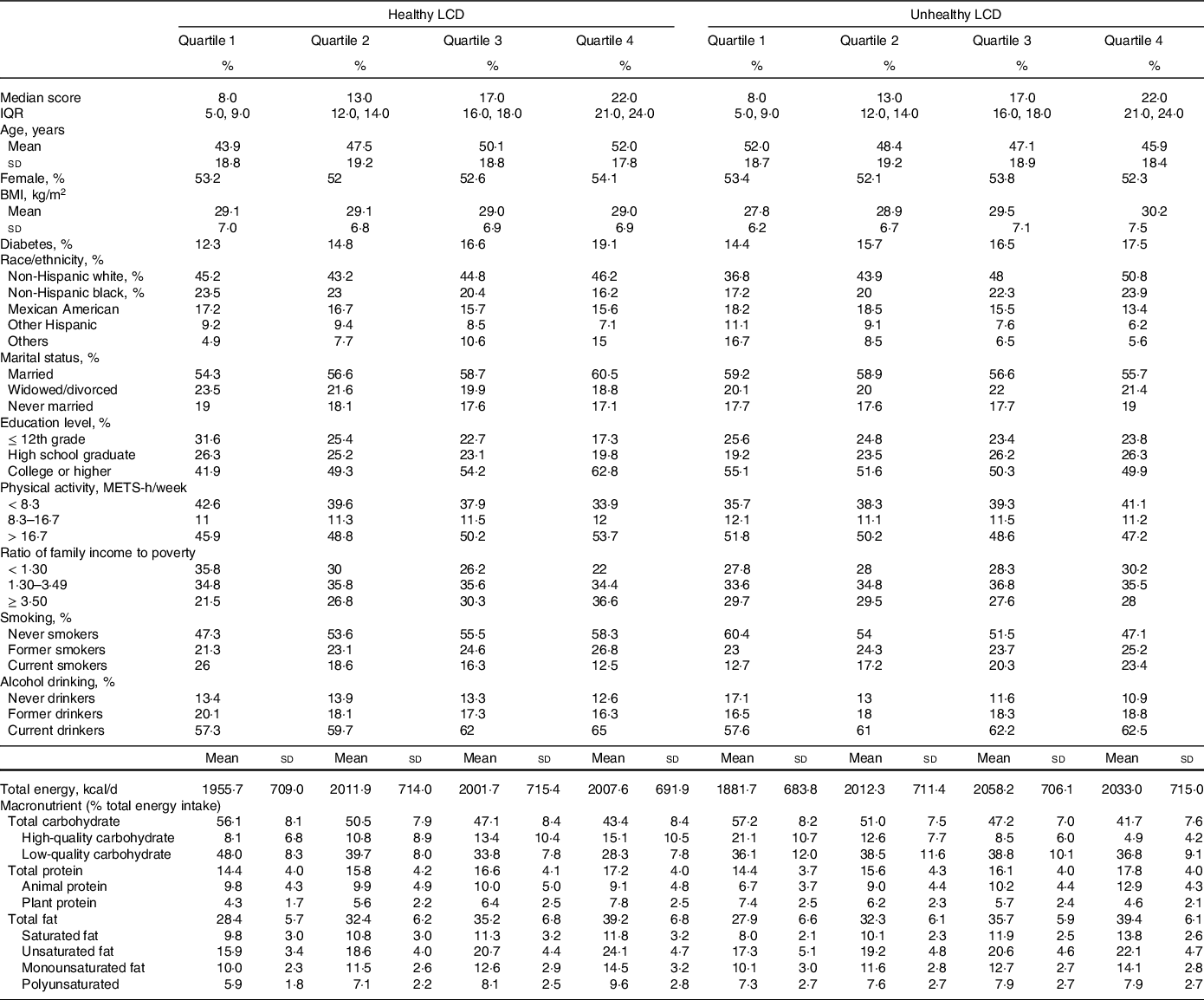
IQR, interquartile range; LCD, low-carbohydrate diet; METS, metabolic equivalent tasks; NHANES, National Health and Nutrition Examination Survey.
* Continuous variables were presented as means (sd) if they were normally distributed, otherwise median (IQR) estimate was used. All variables were standardised to the age distribution of the study population except for age and LCD scores. Of note, the summing proportions for some categories are not 100 % due to missing values or rounding.
Low-carbohydrate diets and metabolic profile
Participants in the highest quartile of healthy LCD score had 5·91 % (95 % CI −10·29 %, −1·32 %) lower insulin, 6·16 % (95 % CI −10·70 %, −1·39 %) lower HOMA-IR and 9·13 % (95 % CI −14·87 %, −3·00 %) lower CRP, compared with those in the lowest quartile (all P trend < 0·001) (Table 2). For the same comparison, higher unhealthy LCD score was associated with 7·53 % (95 % CI 3·14 %, 12·10 %), 9·44 % (95 % CI 4·53 %, 14·58 %) and 17·56 % (11·36 %, 24·12 %) higher levels of insulin, HOMA-IR and CRP, respectively (all P trend < 0·001). A non-significant difference in these markers of metabolic profile was observed for overall, plant-based or animal-based LCD (online Supplementary Table 3).
Table 2. Percentage change (%) and 95 % CI in plasma markers on metabolic profile by quartiles of LCD in the NHANES (2003–2018)
(Percentages and 95 % confidence intervals)

LCD, low-carbohydrate diet; NHANES, National Health and Nutrition Examination Survey; HOMA-IR, homoeostatic model assessment of insulin resistance; CRP, C-reactive protein.
* Model 1 was adjusted for age (18–29, 30–39, 40–49, 50–59 and ≥ 60 years), sex (male, female), total energy intake (kcal/day, tertile), race/ethnicity (non-Hispanic white, non-Hispanic black or other races), education (≤ 12th grade, high school graduate or more than high school), marital status (married, widowed/divorced/separated or never married), ratio of family income to poverty (< 1·30, 1·30–3·49 or ≥ 3·50), physical activity (< 8·3, 8·3–16·7 or > 16·7 METS-h/week), smoking (never smoker, former smoker or current smoker), alcohol drinking (never drinker, former drinker or current drinker), hypertension (yes/no), diabetes (yes/no), cancer (yes/no), CVD (yes/no), family history of CVD (yes/no), family history of diabetes (yes/no) and survey cycle (assigned values from 1 to 8).
† Model 2 was additionally adjusted for BMI (< 18·5, 18·5–24·9, 25·0–29·9 and ≥ 30·0).
‡ Linear trend test was conducted by treating each LCD as a continuous variable in the models.
Low-carbohydrate diets and lipid profile
Participants with higher adherence to healthy LCD had 9·71 % (95 % CI −13·39 %, −5·86 %) lower TAG and 7·60 % (95 % CI 6·26 %, 8·95 %) higher HDL-cholesterol than those in the lowest quartile (all P trend < 0·001). Unhealthy LCD was associated with 1·97 % (95 % CI 0·99 %, 2·95 %) higher TC and 4·11 % (95 % CI 2·02 %, 6·25 %) higher LDL-cholesterol (all P trend < 0·001) (Table 3). Overall and plant-based LCD was associated with lower TAG and higher HDL-cholesterol levels (all P trend < 0·001), while animal-based LCD was associated with lower concentrations of TAG and higher concentrations of TC and HDL-cholesterol (all P trend < 0·001) (online Supplementary Table 4).
Table 3. Percentage change (%) and 95 % CI in plasma markers on lipid profile by quartiles of LCD in the NHANES (2003–2018)
(Percentages and 95 % confidence intervals)

LCD, low-carbohydrate diet; NHANES, National Health and Nutrition Examination Survey; TC, total cholesterol.
* Model 1 was adjusted for age (18–29, 30–39, 40–49, 50–59 and ≥ 60 years), sex (male, female), total energy intake (kcal/d, tertile), race/ethnicity (non-Hispanic white, non-Hispanic black or other races), education (≤ 12th grade, high school graduate or more than high school), marital status (married, widowed/divorced/separated or never married), ratio of family income to poverty (< 1·30, 1·30–3·49 or ≥ 3·50), physical activity (< 8·3, 8·3–16·7 or > 16·7 METS-h/week), smoking (never smoker, former smoker or current smoker), alcohol drinking (never drinker, former drinker or current drinker), hypertension (yes/no), diabetes (yes/no), cancer (yes/no), CVD (yes/no), family history of CVD (yes/no), family history of diabetes (yes/no) and survey cycle (assigned values from 1 to 8).
† Model 2 was additionally adjusted for BMI (< 18·5, 18·5–24·9, 25·0–29·9 and ≥ 30·0).
‡ Linear trend test was conducted by treating each LCD as a continuous variable in the models.
Subgroup and sensitivity analysis
In subgroup analysis (online Supplementary Fig. 2–4), we observed no differential associations according to stratified factors, with the exception of race/ethnicity. The positive association between healthy LCD and HDL-cholesterol seemed stronger in non-Hispanic whites than in other racial/ethnic groups. We found a positive association between unhealthy LCD and HDL-cholesterol in other racial/ethnic groups but not in non-Hispanic whites (all P interaction < 0·0001). When restricting analysis within subjects who were free of CVD, the results were not essentially changed (online Supplementary Table 5–6). When defined LCD as a diet with less than 40 % of energy from carbohydrate, LCD was associated with lower insulin, HOMA-IR and TAG, but high levels of TC and HDL-cholesterol, compared with a diet with ≥ 60 % of energy from carbohydrate (online Supplementary Table 7).
Discussion
In this large nationwide cross-sectional study among US adults, we found that healthy LCD was associated with lower levels of insulin, HOMA-IR, CRP and TAG and higher concentrations of HDL-cholesterol, while unhealthy LCD was associated with higher concentrations of insulin, HOMA-IR, CRP, TC and LDL-cholesterol. These results indicate that the association between LCD and cardiometabolic risk markers appears to depend on the quality and types of macronutrients in the diet.
We found that healthy LCD may have positive and unhealthy LCD may have negative impacts on CRP and metabolic and lipid profiles, whereas overall LCD generally showed a null association with most of cardiometabolic risk markers. Moreover, when defined LCD as a diet with less than 40 % of energy from carbohydrate and did not consider dietary quality, LCD was associated with high levels of TC in our analysis. These findings indicate that besides composition, the food sources and types of macronutrients in LCD may play an important role in cardiometabolic health. In line with these findings, few existing cohort studies(Reference Shan, Guo and Hu14,Reference Halton, Willett and Liu29,Reference Akter, Mizoue and Nanri44) showed an inverse association between healthy or plant-based LCD and long-term risk of CVD incidence or mortality, a positive association for unhealthy or animal-based LCD and a non-significant association for overall LCD. These results may also partly explain the inconsistent findings in the effect on lipid file among previous randomised controlled trials(Reference Willems, Sura-de Jong and van Beek4,Reference Mansoor, Vinknes and Veierod8) , in which neither the food sources nor the types of macronutrients in LCD were considered.
Different impact on metabolic profile between healthy and unhealthy LCD could be partly explained by different quality of carbohydrate and varied subtypes of fat and protein in each LCD. Previous studies(Reference Yeo, Yoon and Kim45,Reference Musa-Veloso, Poon and Harkness46) showed that high-quality carbohydrates, such as non-starchy vegetables, whole grains and whole fruits, were associated with lower postprandial blood glucose response and HOMA-IR, whereas low-quality carbohydrates such as refined grains and added sugars with high glycaemic load could be associated with high postprandial glucose and insulin. A meta-analysis of nineteen studies showed that replacing 5 % of the energy from carbohydrates or saturated fat with energy from polyunsaturated fat was associated with lower levels of HOMA-IR(Reference Imamura, Micha and Wu47).
Similarly, we observed that healthy LCD showed an anti-inflammatory and unhealthy LCD showed a pro-inflammatory property. We did not find a significant association between overall LCD and CRP levels, which was in line with the only population-based study investigating LCD and inflammatory markers among 1097 participants aged 40–59 years in Japan(Reference Nakamura, Ueshima and Okuda27). Consistently, the anti-inflammatory or pro-inflammatory properties have been reported for several nutrient and food components in LCD. For example, green leafy vegetables, whole grains, fruits and nuts were inversely associated with levels of hs-CRP and IL-6(Reference Nettleton, Steffen and Mayer-Davis48). Fibre and n-3 fatty acids have been associated with decreased concentrations of markers of inflammation, whereas saturated fat has been associated with increased levels of inflammation(Reference Nieman, Anderson and Cifelli49). In addition, carbohydrates have been identified as key source of oxidative stress, and restriction of carbohydrate can also reduce a number of pro-inflammatory cytokines, chemokines and adhesion molecules(Reference Sharman and Volek50). Notably, plant- and animal-based LCD were not associated with the markers on metabolic profile in the analysis, indicating that the categorisation of LCD into healthy and unhealthy LCD appeared superior to the categorisation of LCD into plant- and animal-based LCD on differentiating the quality of LCD.
Consistent with our observations, few existing studies showed that overall LCD was positively associated with HDL-cholesterol(Reference Ha, Joung and Song25,Reference Nakamura, Ueshima and Okuda27) in general population, though these studies did not investigate healthy and unhealthy LCD separately. The increased levels of HDL-cholesterol in participants with high adherence to overall LCD might be partly due to high dietary fat intake(Reference Kris-Etherton, Etherton and Yu51), which was confirmed in a meta-analysis of sixty randomised controlled trials, showing that SFA, MUFA and PUFA increased HDL-cholesterol more than carbohydrates did(Reference Mensink, Zock and Kester52). We found that overall and healthy LCD were associated with lower TAG levels. Consistently, a recent meta-analysis of twelve randomised controlled trials conducted among participants who were at least 18 years old and had no specific diseases demonstrated a decrease of TAG levels in LCD group compared with the control group(Reference Dong, Guo and Zhang53). Such beneficial effects of LCD could be due to improved postprandial hyperinsulinaemia, which can lead to inhibition of lipolysis, and enhance delivery of NEFA for hepatic esterification.
We found that the positive association between healthy LCD and HDL-cholesterol levels was more prominent among non-Hispanic whites but not among other racial/ethnic groups. By comparison, the percentage of energy from low-quality carbohydrates (i.e. starchy vegetables, refined grains, and fruit juice) and animal protein for non-Hispanic whites was 37·0 and 9·0 %, respectively, which were lower than those from other racial/ethnic groups (i.e. 38·1 and 9·4 %, respectively). The percentage of energy from dietary fat for non-Hispanic whites was 34·6 %, which was higher than those from other racial/ethnic groups (33·0 %). Therefore, the differential associations could be partly due to the subtle difference in how healthy LCD score was comprised. Another possible explanation is that non-Hispanic whites generally have lower inflammation levels(Reference Dinwiddie, Zambrana and Doamekpor54) and prevalence of cardiovascular risk factors(Reference Pool, Ning and Lloyd-Jones55) compared with other racial/ethnic groups. Alternatively, the findings might be due to chance. Future studies are needed to confirm our findings and to elucidate the underlying mechanisms.
Strengths of the present study include the use of a large nationally representative sample of US adults, the comprehensive assessment of plasma markers of cardiometabolic risk and the use of LCD that distinguish between different subtypes and food sources of macronutrients. There are several limitations to our study. First, cross-sectional design cannot determine causality. Second, the single measure of biomarkers may cause some measurement error. Last, diet was measured by 24-h recalls which may lead to misreporting and might not represent the usual intake, although we used several methods, such as multiple-pass method and dietary sampling weights to reduce the measure error and to improve estimates.
In conclusion, adherence to healthy LCD was associated with favourable concentrations of many cardiometabolic risk markers, while unhealthy LCD had a negative impact on these biomarkers. These findings support a potential benefit in emphasising high-quality carbohydrates, unsaturated fat and plant protein in the diet for type 2 diabetes and CVD prevention, which underscore the need to consider the quality and types of macronutrients in future LCD studies. Due to the cross-sectional design, cohort studies or clinical trials are warranted to validate our findings.
Acknowledgements
This study uses data from the NHANES. The authors thank the NCHS, the US Centers for Disease Control and Prevention (CDC) for their financial support for data collection and analysis. The authors thank the study participants and staff.
This work was supported by the National Natural Science Foundation of China (82073651), Anhui Provincial Natural Science Foundation (2008085MH262 and 2108085QH357), Anhui Provincial Education Department (gxyqZD2021099) and grants from Anhui Medical University (2021xkjT007, 2020lcxk033, XJ201935 and 2019xkj161).
Huang, Xie and Li had full access to all the data in the study and take responsibility for the integrity of the data and the accuracy of the data analysis. Study concept and design: Yang and Huang. Acquisition, analysis or interpretation of data: all authors. Drafting of the manuscript: Huang and Yang. Critical revision of the manuscript for important intellectual content: all authors. Statistical analysis: Huang, Li. Obtained funding: Yang, Tang and Zhang. Administrative, technical or material support: Yang. Study supervision: Yang.
The authors disclose no conflicts.
Supplementary material
For supplementary material referred to in this article, please visit https://doi.org/10.1017/S0007114522003038