Near-infrared spectroscopy (NIRS) has been used in medical research for more than 20 years. Based on the principles of NIRS, functional near-infrared spectroscopy (fNIRS) is a non-invasive neuroimaging technology that maps the functioning of the cerebral cortex by measuring haemodynamics (Reference Ferrari and QuaresimaFerrari 2012). Although fNIRS technology was originally used in basic neuroscience research to investigate the motor, visual, language and executive functions of healthy participants, it is increasingly been used to study a wide range of psychiatric disorders, including schizophrenia (Reference Ehlis, Herrmann and PlichtaEhlis 2007), depression (Reference Fukuda and MikuniFukuda 2012; Reference Liu, Sun and ZhangLiu 2014; Reference Takizawa, Fukuda and KawasakiTakizawa 2014), bipolar disorder (Reference Takizawa, Fukuda and KawasakiTakizawa 2014), panic disorder (Reference Nishimura, Tanii and HaraNishimura 2009), obsessive–compulsive disorder (Reference Okada, Ota and IidaOkada 2013), addiction (Reference Dempsey, Harris and ShumwayDempsey 2015), Alzheimer's disease (Reference Chou and LanChou 2013), autism spectrum disorder (Reference Fekete, Beacher and ChaFekete 2014; Reference Jung, Strother and Feil-SeiferJung 2016) and attention-deficit hyperactivity disorder (ADHD) (Reference Ehlis, Bähne and JacobEhlis 2008; Reference Inoue, Sakihara and GunjiInoue 2012; Reference Ishii-Takahashi, Takizawa and NishimuraIshii-Takahashi 2014). Most studies have attempted to delineate objective and measurable haemodynamic changes in the cerebral cortex in relation to the psychopathology of these psychiatric disorders. Hence, incorporating fNIRS into psychiatry may address the current limitations of clinical interviews and mental state examinations in establishing a psychiatric diagnosis.
Reference Martinelli and ShergillMartinelli & Shergill (2015) published a review about neuroimaging and psychiatry in BJPsych Advances. The review discussed conventional neuroimaging techniques using large, costly and immobile imaging devices, such as computed tomography (CT) scanning, magnetic resonance imaging (MRI), magnetic resonance spectroscopy (MRS), positron emission tomography (PET) and magnetoencephalography (MEG). These techniques require the participants to be in a supine position with their head restrained and without any movement of the body (Reference Martinelli and ShergillMartinelli 2015). The scanning procedures are typically conducted by radiographers, with high costs incurred by each measurement. Although Martinelli & Shergill described fNIRS as a tool primarily used with infants, its use has expanded to adult and elderly populations. The increasing popularity of fNIRS could be attributed to its advantages over conventional neuroimaging devices, i.e. that it:
-
• allows for measurements to be taken in any body position while retaining good temporal resolution
-
• has relatively low cost and volume
-
• does not require radiographers
-
• is compatible with a variety of neuroimaging instruments (Reference Ferrari and QuaresimaFerrari 2012).
Furthermore, an fNIRS device is highly portable owing to its light weight and small size, allowing measurements to be obtained in various clinical settings. Since fNIRS is not sensitive to body movement, it can also be used to measure ‘fidgety’ patients such as children with ADHD. Consequently, fNIRS has potential in assisting diagnosis, predicting clinical outcomes and evaluating treatment outcomes in psychiatry (Reference Fukuda and MikuniFukuda 2012; Reference Koike, Nishimura and TakizawaKoike 2013; Reference Ho, Zhang and HoHo 2016). A limitation of fNIRS is its inability to detect haemodynamic changes in deep neuroanatomical structures (Reference Chou and LanChou 2013). Nevertheless, understanding the theoretical background of fNIRS as well as its strengths and limitations will facilitate further development of the technology.
The purpose of this article is to introduce the theoretical background of fNIRS, key principles in the application of fNIRS and its current use in various psychiatric disorders, and to share our vision of its future applications in academic and clinical psychiatry.
Theoretical background of fNIRS
Brain–computer interface
The fNIRS system is an example of a brain–computer interface. Each fNIRS device includes the following components:
-
• a set of optical fibres (NIR light emitter/detector)
-
• a computer unit with signal processor and algorithm
-
• a computer monitor (Fig. 1).
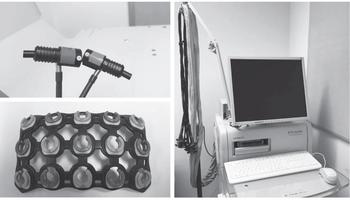
FIG 1 The components of a functional near-infrared spectroscopy (fNIRS) device: near-infrared light emitter/detector probes (upper-left), headgear to hold the probes (lower-left) and a computer unit with a signal processor (right).
The NIR light emitter and detector probe come as a set. The probe set is positioned over different areas of the head, e.g. the prefrontal cortex, frontal lobe, parietal lobe, temporal lobe or pre-supplementary motor areas (Fig. 2). The positioning of fNIRS probes over the scalp is similar to the attachment of electroencephalogram (EEG) electrodes on the scalp according to the EEG 10/20 system. A code is assigned to each probe for identification purposes. A topographical image of cerebral haemodynamics can be reconstructed and displayed on the monitor (online Fig. DS1). There are currently various different models of fNIRS devices, with some being particularly lightweight and designed for studying mobile participants, e.g. the NIRSport (online Fig. DS2).
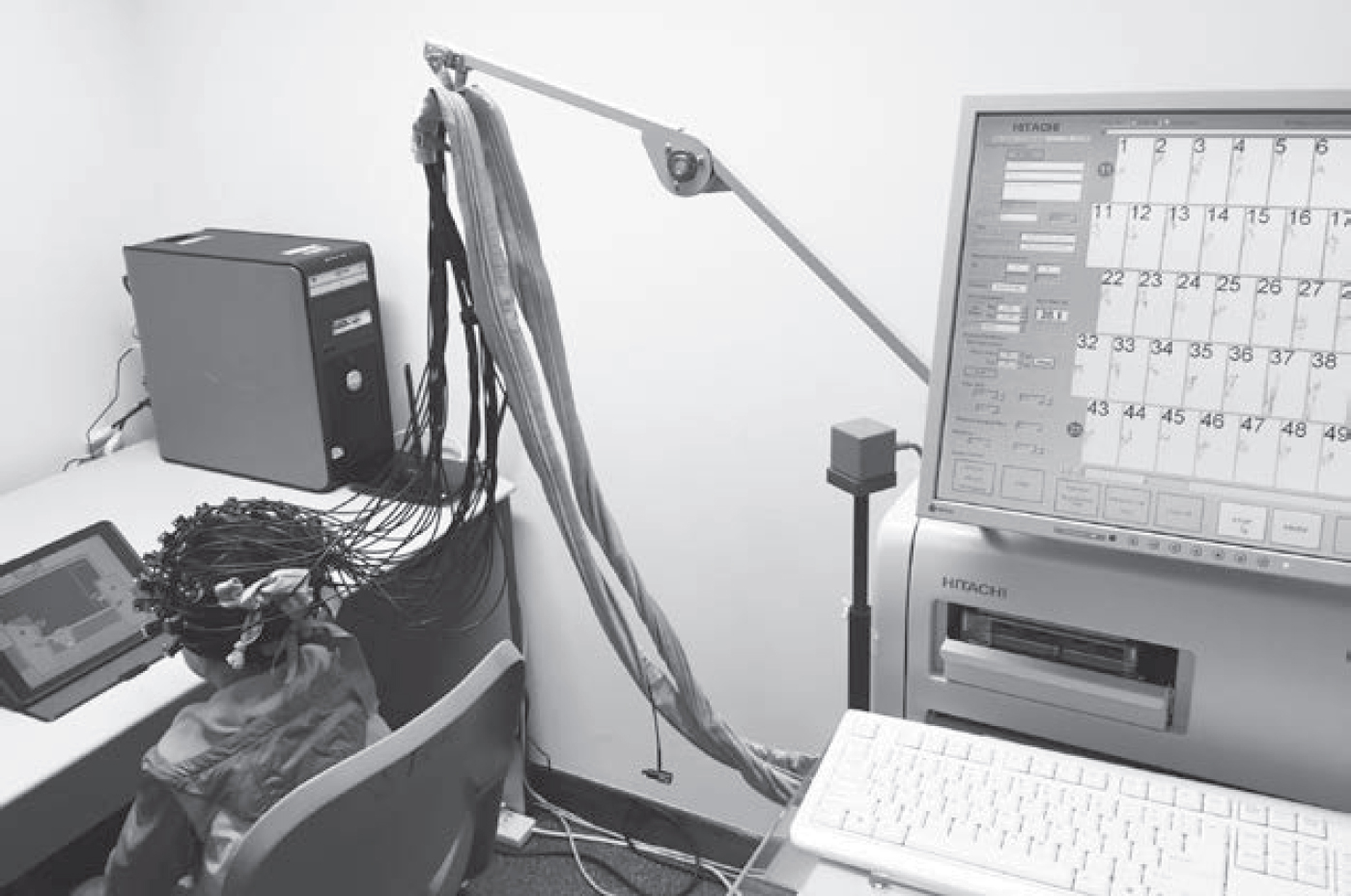
FIG 2 Data acquisition of cerebral haemodynamics during a tablet-based cognitive task.
Brain activation
Haemoglobin is a protein that forms an unstable and reversible bond to oxygen in red blood cells. In its oxygen-loaded form, it is bright red and known as oxyhaemoglobin (oxy-Hb). In its oxygen-unloaded form, it is purple/blue and known as deoxyhaemoglobin (deoxy-Hb). fNIRS can be used to examine haemodynamic activity in the brain by detecting simultaneous changes in the concentrations of oxy-Hb and deoxy-Hb. The rationale lies in a mechanism known as neurovascular coupling, whereby activation of a brain region is reflected by an increase in oxy-Hb and a corresponding decrease in deoxy-Hb levels. When there is an increase in activity within a particular brain region, there would be an increase in blood supply and oxy-Hb levels. Although deoxy-Hb would also increase owing to oxygen consumption during the brain activity, this would be compensated for by the increase in blood supply, resulting in a net decrease in deoxy-Hb levels (Reference Koike, Nishimura and TakizawaKoike 2013).
Both fMRI and fNIRS map brain activity by measuring changes in haemoglobin concentration. Studies that have used fNIRS and fMRI concurrently to measure activity during cognitive tasks have found fNIRS results to be highly correlated (r = 0.77–0.94) with fMRI blood oxygen level-dependent (BOLD) signal recordings in the same neuroanatomical regions (e.g. Reference Cui, Bray and BryantCui 2011). Hence, fNIRS can be an appropriate substitute for fMRI to study activities of the cerebral cortex when engaged in cognitive tasks. Researchers designing studies with fNIRS would need to consider:
-
• whether the spatial resolution of fNIRS is adequate to answer their question of interest
-
• whether their design accounts for weaker signal-to-noise ratio, especially in brain regions more distal from the scalp (Reference Cui, Bray and BryantCui 2011)
-
• that the fNIRS-Hb signal mainly reflects haemodynamic changes in the grey matter (Reference Sato, Yahata and FunaneSato 2013).
Nature of light
fNIRS was developed based on the principles of NIR spectroscopy. According to Reference Ferrari and QuaresimaFerrari & Quaresima (2012), human tissue is transparent to NIR light of wavelength 650–1000 nm. This light can penetrate through the skin and bone with low absorption, to reach haemoglobin in the capillaries with high absorption (Reference Koike, Nishimura and TakizawaKoike 2013). The NIR light will be scattered in tissues or absorbed by chromophores. The fNIRS instrument utilises characteristics of light absorption by haemoglobin to assess changes in oxy-Hb and deoxy-Hb concentration in various brain regions.
The mechanism of fNIRS measurement involves the emission and absorption of light. Light emitted from the source probe (emitter) is scattered or absorbed. The scattered light will then be detected by detector probe located on the surface of the scalp. The source probe and detector probe are paired by modulation of light amplitude. The different light absorption rates of oxy-Hb and deoxy-Hb allow for the concentrations of oxy-Hb and deoxy-Hb within a brain region to be measured. There are three different types of NIRS technique, each based on specific illumination methods:
-
• the continuous wave modality (providing constant tissue illumination)
-
• the frequency domain method (illuminating the head with intensity-modulated light)
-
• the time domain technique (illuminating the head with short pulses of light).
These three techniques are based on different characteristics of light emission and different detection mechanisms. Most commercial fNIRS tools adopt the continuous wave modality, which has the advantages of a higher sampling rate, relatively low cost and increased portability. However, the penetration power (depth of penetration) of continuous wave modality is lower than that of the frequency domain and time domain techniques. Furthermore, instruments using the continuous wave modality are only able to measure changes in levels of oxy-Hb and deoxy-Hb, whereas the other two techniques can measure absolute levels of oxy-Hb and deoxy-Hb (Reference Ferrari and QuaresimaFerrari 2012). Hence, the lack of absolute values of oxy-Hb and deoxy-Hb concentration are a limitation of most commercial fNIRS devices.
fNIRS has several advantages over other neuroimaging tools. First, fNIRS is not sensitive to movement and can be used on fidgety patients, young children and participants performing either sitting- or movement-based tasks. Second, the light used in fNIRS does not interfere with other tools, such as fMRI, PET and EEG. Hence, multimodal measurements can be performed on patients (e.g. fNIRS and fMRI; fNIRS and EEG; or fNIRS and PET). Third, its source probe and detector probe can be paired and located to provide spatial information regarding the brain's topography, albeit with less satisfactory spatial resolution than fMRI. Fourth, the high speed of light and high sampling rate of current models of fNIRS device (0.1 s) may provide good temporal resolution, although the temporal resolution is still lower than that of EEG. Last, as some fNIRS devices are compatible with three-dimensional (3D) brain mapping software, a 3D image could be reconstructed with measurement data based on 3D coordinates. This can be done with or without importing fMRI measures and could certainly facilitate topographical representation of brain activation to assist in the diagnostic process.
The fNIRS device has some inherent limitations owing to the characteristics of NIR light and its penetration and absorption. Brain activity measured by fNIRS is based on light absorption by haemoglobin in the blood. However, both brain activity and peripheral physiological changes may contribute to light absorption and influence measurement data. For instance, activation of the sympathetic and parasympathetic nervous systems may lead to vasodilation or vasoconstriction in the skin. It is challenging to differentiate task-induced superficial haemodynamics from cerebral haemodynamics, even with steady blood flow to the skin (Reference TakahashiTakahashi 2011; Reference Hoshi, Masamoto, Hirase and YamadaHoshi 2016), and in practice there will often be variation in the rate of blood flow across different regions of measurement within or between individuals. Skin blood flow artefacts can be removed by applying independent component analysis (ICA) if there is low correlation between skin blood flow and cerebral blood flow (Reference Takanori, Haruhide and YasuhiroTakanori 2011). Another limitation is the penetration power of light, which limits the depth of the brain region that can be reached; as a result, fNIRS may not be suitable for examining the activity of subcortical or deep brain structures (Reference Chou and LanChou 2013; Reference Hoshi, Masamoto, Hirase and YamadaHoshi 2016). For example, the pathology of Alzheimer's disease involves deficits of deep brain structures, including the parahippocampal gyrus and hippocampus, which cannot be measured by fNIRS devices of continuous wave modality.
In summary, understanding the strengths and limitations of fNIRS may help in exploring its potential in psychiatric research and clinical practice.
Principles of applying fNIRS
Applying fNIRS to the diagnosis of psychiatric disorders and monitoring of treatment progress is a relatively new area that requires further development. When establishing a clinical diagnosis, hypotheses are first generated before being tested with appropriate methods. fNIRS could be considered as a potential aid to support the diagnosis of psychiatric conditions (Reference Takizawa, Fukuda and KawasakiTakizawa 2014). Here, we highlight some practical considerations in setting up the probes and designing paradigms, and refer to previous studies that illustrate key principles in applying fNIRS for psychiatric conditions.
Single or multiple channels in measurement
The emitter and detector probes of the fNIRS device are paired to form a channel over a defined measurement area. The number of probes can be customised according to the areas of interest. For example, a 3 × 11 grid will contain 52 measurement channels and a 4 × 4 grid will contain 24. Research has used fNIRS with 2-channel (Reference Kubota, Toichi and ShimizuKubota 2005), 22-channel (Reference Ehlis, Herrmann and PlichtaEhlis 2007) and 52-channel systems (Reference Yamanaka, Tomioka and KawasakiYamanaka 2014). The advantages of using fewer channels include cost reduction, shortened preparation time and increased portability. When studying haemodynamics, the monitoring is limited to the cerebral cortex, which is a well-defined region suitable for study using a system with fewer channels. Otherwise, use of systems with fewer channels may run the risk of missing out important neuroanatomical areas (Reference Ehlis, Herrmann and PlichtaEhlis 2007).
Design of study paradigm
In general, there are two types of design paradigm: block design and event-related design (also known as trial-based design). Block design examines the pattern of brain activation across conditions, such as an alternating pattern of one or more blocks of the resting condition and the task condition. The condition could also be set as a time course (e.g. the pre-task, during-task and after-task period). By contrast, event-related design examines brain activation triggered by events that occur in a random order rather than an alternating pattern. Event-related design offers greater flexibility and measures transient changes in brain activity over short and discrete intervals.
As the fNIRS device has good temporal resolution, it is suited to assessing brain activity through event-related design (Reference Schroeter, Zysset and KupkaSchroeter 2002). Depending on the brand and model of the fNIRS device, the person conducting the examination can either set their own protocol (with or without synchronising with a task created by computerised stimulus-presentation software) or apply built-in study paradigms as appropriate.
Selecting brain areas and regions of interest
Understanding neural correlates is fundamental in fNIRS. Currently, the diagnosis of psychiatric conditions relies on mapping findings from clinical presentation and mental state examination on to predefined diagnostic criteria in the DSM-5 or ICD-10. This process can be subjective and qualitative, and it does not elucidate the underlying pathology of a psychiatric condition. The symptoms and behaviours may be caused by particular activation and deactivation patterns of the cerebral cortex when engaged in cognitive tasks. Given that brain activity over particular brain areas can be characterised and measured using fNIRS, it is necessary to define respective brain areas (regions of interest) and brain functions for investigation.
Some psychiatric illnesses were found to be correlated with dysfunctions of specific neuroanatomical areas. For instance, schizophrenia has been associated with frontal cortex dysfunction, and task-related brain activation was hypothesised to differ between patients with schizophrenia and healthy controls. Reference Ehlis, Herrmann and PlichtaEhlis et al (2007) examined frontal lobe activity in 12 patients with schizophrenia and 12 healthy controls, using fNIRS and a verbal fluency task (VFT). They found that frontal activation was significantly reduced in people with schizophrenia, compared with controls. Frontal lobe abnormalities, especially a decrease in bilateral frontotemporal oxygenation, have also been found in individuals with unipolar depression, using the VFT (Reference Noda, Yoshida and MatsudaNoda 2012). Online Fig. DS3 illustrates the differences in activation patterns during the VFT between a healthy control and a patient with depression who had received electroconvulsive therapy (ECT). Prefrontal cortex dysfunction was also found among adults with ADHD. Reference Ehlis, Bähne and JacobEhlis et al (2008) examined 13 adults with ADHD and 13 healthy controls by pairing fNIRS with a working memory task intended to assess prefrontal cortex function. The results showed that prefrontal activation was significantly lower in those with ADHD relative to controls. Reference Inoue, Sakihara and GunjiInoue et al (2012) examined the haemodynamic response of the prefrontal cortex using fNIRS and the ‘go/no go’ task. Individuals with ADHD demonstrated lower levels of brain activation in the prefrontal cortex compared with healthy controls during the inhibition response condition (no go condition), but not the execution response condition (go condition). The above examples illustrate the feasibility of using fNIRS to identify unique brain activation patterns present among psychiatric patients by pairing it with activation tasks targeting specific cognitive processes. Table 1 summarises the key findings of studies using fNIRS to assess different psychiatric disorders. These studies were identified by keyword searches (for ‘fNIRS’ and ‘specific psychiatric disorders’) in PubMed.
TABLE 1 Studies using functional near-infrared spectroscopy to assess different psychiatric disorders

Differential diagnosis of psychiatric disorders
Patients with different psychiatric illnesses may present with similar clinical features, increasing the challenge of diagnosis. It is possible that their symptomatology is related to dysfunction of particular neuroanatomical areas or reflected by distinct brain activity patterns. In 2014, Reference Takizawa, Fukuda and KawasakiTakizawa et al (2014) conducted a large-scale, multisite case–control study of group comparisons. A total of 673 patients with psychiatric disorders (major depressive disorder, bipolar disorder and schizophrenia with depressive symptoms) and 1007 healthy volunteers were examined. The study used 52-channel fNIRS and the VFT to measure relative oxy-Hb and deoxy-Hb signal changes in two brain regions: (a) frontopolar and dorsolateral prefrontal cortical regions; (b) the ventrolateral prefrontal cortex, and superior and middle temporal cortical regions. The fNIRS signal changes were measured over three periods – pre-task (baseline), task activation, and post-task – for a total of 125 seconds. This revealed that the centroid value (measured in seconds, and indicated by the position along the time course of a perpendicular line from the centroid of positive change of the NIRS signal area) in region 1 was able to correctly differentiate 74.6% of patients with major depressive disorder from healthy controls with no depression. The promise of fNIRS in assisting psychiatric diagnosis led the Japanese Health Ministry to approve fNIRS as an ‘advanced medical technology’ in 2014, and it is an insurance-covered investigation to aid differential diagnosis of depressive state (Reference FukudaFukuda 2015). There are currently no such initiatives in other countries.
The study by Reference Takizawa, Fukuda and KawasakiTakizawa et al (2014) provides insight into the applicability of fNIRS in differential diagnosis of psychiatric disorders and the feasibility of providing visual evaluation results to patients and caregivers. There is a need to develop a database of cerebral haemodynamic patterns of various psychiatric disorders to serve as a reference for future comparisons. fNIRS can augment clinical interviews and mental state examination in establishing a clinical diagnosis. It is possible that clinical symptoms in psychiatric disorders are secondary to low/exaggerated cortical or subcortical function together with suboptimal brain arousal state and other physiological dysregulation (e.g. autonomic dysfunction). As fNIRS can be simultaneously combined with other devices, future diagnostic approaches could involve multimodal measures (measuring other biological signals, such as EEG waves and autonomic activity) as a diagnostic tool in clinical psychiatry. Although fNIRS cannot produce detailed pictures of neuroanatomical structures, and hence cannot replace structural MRI, its portability and cost-effectiveness support it as a potential diagnostic tool to verify fMRI findings (e.g. functional hypofrontality in schizophrenia). Nevertheless, it needs to be emphasised that although fNIRS has the potential to be a supplementary diagnostic tool, it cannot replace conventional history-taking and mental state examination in establishing a psychiatric diagnosis. In the near future, more assessment paradigms need to be developed and training in clinical application of fNIRS should be offered to psychiatrists.
Conclusions
This article provides a brief review of the theoretical background and principles of applying fNIRS in psychiatric research. fNIRS is a tool that can potentially facilitate the clinical diagnostic process and identify stages of psychiatric illnesses by providing objective and quantifiable evidences of brain changes (Reference Nishimura, Tanii and HaraNishimura 2009; Reference Fukuda and MikuniFukuda 2012). However, this will require specific cerebral haemodynamic patterns to be validated in larger clinical populations with specific psychiatric disorders. Large-scale studies are now possible, owing to advances in the fNIRS technology and improvements in its measurement accuracy. Additionally, it will be important to develop standardised paradigm protocols and data analysis methodologies to ensure reproducibility of results. As recommended by Reference Koike, Nishimura and TakizawaKoike (2013), establishing an international community of NIRS researchers may promote more active use of fNIRS and advance expertise in this challenging area. This will, in turn, shape developments in the role of fNIRS in complementing psychiatric diagnosis and management in years to come.
MCQs
Select the single best option for each question stem
-
1 fNIRS is unlikely to be affected by:
-
a body movement
-
b skin integrity
-
c skull thickness
-
d thick scalp hair
-
e none of the above.
-
-
2 The light used in fNIRS is:
-
a gamma radiation
-
b infrared light
-
c radio waves
-
d sunlight
-
e ultraviolet light.
-
-
3 A limitation of fNIRS compared with fMRI is that it:
-
a is portable
-
b is more expensive
-
c has lower temporal resolution
-
d has lower spatial resolution
-
e cannot be combined with EEG devices.
-
-
4 fNIRS cannot be used to assess the:
-
a frontoparietal cortex
-
b hippocampus
-
c occipital lobe
-
d prefrontal cortex
-
e superficial cortex.
-
-
5 The correlation coefficient for fNIRS activity and fMRI signal during the same cognitive task is in the range:
-
a 0.17–0.24
-
b 0.27–0.34
-
c 0.37–0.54
-
d 0.47–0.74
-
e 0.77–0.94.
-
MCQ answers

1 | a | 2 | b | 3 | d | 4 | b | 5 | e |
eLetters
No eLetters have been published for this article.