Highlights
-
• Native English speakers and learners of Kaqchikel gave semantic associates to words.
-
• Networks were created of semantically related words for both groups.
-
• English networks had structures similar to networks of creative individuals.
-
• Kaqchikel networks had structures similar to networks of less creative individuals.
-
• Different semantic network structures may account for the foreign language effect.
1. Introduction
Network science is an interdisciplinary field drawing from mathematics, physics, computer science, and other related fields to represent complex systems as networks (Watts & Strogatz, Reference Watts and Strogatz1998). Nodes in the network represent individual entities in the system; edges represent a relationship between two nodes. The complex networks used in the present study should not be confused with artificial neural network models (Rogers & McClelland, Reference Rogers and McClelland2014) where the terms network, nodes, and connections are also used.
Complex networks have been used in various domains, including physics, biology, and the social sciences (Newman, Reference Newman2008). These computational and mathematical techniques have also been used to study different aspects of cognitive and language processing (Siew et al., Reference Siew, Wulff, Beckage and Kenett2019). Words in the mental lexicon are represented as nodes, and edges represent phonological (for a review see Vitevitch, Reference Vitevitch2021), semantic (Gravino et al., Reference Gravino, Servedio, Barrat and Loreto2012), or other types of relationships between words (e.g., Levy et al., Reference Levy, Kenett, Oxenberg, Castro, De Deyne, Vitevitch and Havlin2021). Importantly, the structure of the emerging network influences the efficiency of processes in that system (Kleinberg, Reference Kleinberg2000), making it useful to analyze the overall structure of various types of cognitive networks (e.g., Marko & Riečanský, Reference Marko and Riečanský2021).
One common way to examine the mental lexicon is to create a network of semantic associates obtained via a word association task (De Deyne et al., Reference De Deyne, Navarro, Perfors, Brysbaert and Storms2018). In a word association task, participants are given a cue word and asked to state the first word(s) that come to mind. Nodes represent the cue and response words, and (directed) edges connect the words given as responses to each cue. In many areas of the social and behavioural sciences, much research has involved participants from western, educated, industrialized, rich, and democratic (WEIRD) societies (Henrich et al., Reference Henrich, Heine and Norenzayan2010). Further, much psycholinguistic research has focused on written, institutionally-supported, standardized, and prestigious (WISP) languages (Mallinson et al., Reference Mallinson, Cahill, Francis, Namboodiripad, Labrada and Bender2021). To examine a broader range of languages, to assess language learning in a lesser-taught language, and to raise awareness of indigenous languages (note that 2022–2032 was declared the International Decade of Indigenous Languages by the United Nations), we, in the present study, elicited semantic associates to a set of cue words from monolingual English speakers, and from learners of Kaqchikel.
Kaqchikel is a moderately synthetic language from the K’ichean branch of the Mayan language family (Garzon et al., Reference Garzon, Brown and Becker Richards1998). It is spoken by approximately 500,000 people in the central highlands of Guatemala (in the regions between Guatemala City and Lake Atitlán). The Kaqchikel language has limited syllable structure, uses many fusional affixes, has pro-drop agreement markers that replace pronouns, a highly interconnected morphology and syntax, and has a relatively free word order (Tummons, Reference Tummons2010). The number of Kaqchikel speakers has been declining—making it an endangered language (Instituto Nacional de Estasística, 2018 census)—in part because many families are teaching their children Spanish exclusively due to the perceived social and economic advantages of speaking Spanish in the region. However, many native Kaqchikel speakers, Guatemalan government and education sectors, and other professionals and scholars are engaging in language revitalization efforts in Guatemala (Garzon et al., Reference Garzon, Brown and Becker Richards1998).
In the present study, we used the techniques of network science to construct and compare the semantic networks of monolingual English speakers and learners of Kaqchikel. In these networks, nodes represented the cue words and the responses, and directed edges connected the cue word to the responses.
2. Methods
2.1. Participants
The study reported here was approved by the Institutional Review Board at the University of Kansas. Participation in this study via Qualtrics was completely voluntary, and participants provided informed consent prior to beginning the word association task. The 90 (self-reported) native English speakers who completed the task were recruited from a General Psychology course at the University of Kansas (KU) and received course credit for their participation. Nine students in Intermediate or Advanced Kaqchikel Maya courses at the University of Kansas completed the task and received extra credit for the class for their participation. The amount of time that each student spent learning Kaqchikel ranged from roughly 5 months (i.e., a little more than a semester of coursework) to 5 years (n = 1, via immersive experience). Given that the English speakers and Kaqchikel learners were all students enrolled at KU in the same semester, any social/cultural/and so forth differences typically observed in two independent groups of language users were reduced.
2.2. Procedure
The Kaqchikel learners received 71 Kaqchikel words (from Moore, Reference Moore2021; see Appendix A) and presented one word at a time on a computer screen as the cue words in a word association task. Participants were asked to respond with the first word that came to mind when they saw the Kaqchikel cue word. Based on vocabulary lists from the Intermediate and Advanced Kaqchikel Maya courses at KU, 57 words should have been known, and 14 words might be novel for students in those courses. Participants were encouraged to respond with Kaqchikel words that they knew and not simply translate the cue words to English. However, some responses were provided in English (indicated with “_E” on the node label) or in Spanish (indicated with “_S” on the node label).
The monolingual English speakers received the same list of 71 Kaqchikel words (translated into English). Participants were asked to respond with the first English word that came to mind when they saw the cue word.
3. Results and discussion
As many have noted (e.g., De Deyne et al., Reference De Deyne, Navarro, Perfors, Brysbaert and Storms2018), there is variability across studies using the semantic associate task in the number of responses that participants are asked to provide (e.g., the first, more than one, as many as possible in a set amount of time, etc.), and in the criteria used to “count” a response (e.g., 2 or more participants provide the response, etc.). Given the limited number of Kaqchikel learners and the small number of cue words (relative to other studies) in the present study, we created our networks (using the Gephi software; Bastian et al., Reference Bastian, Heymann and Jacomy2009) by having nodes represent the 71 cue words with directed edges connecting to nodes representing all of the (real-word) responses provided by participants (regardless of the number of participants who provided that response). Table 1 lists several commonly used measures for the two resulting networks. See Appendix B for definitions of each measure.
Table 1. Network measures of the Kaqchikel learners and monolingual English speakers
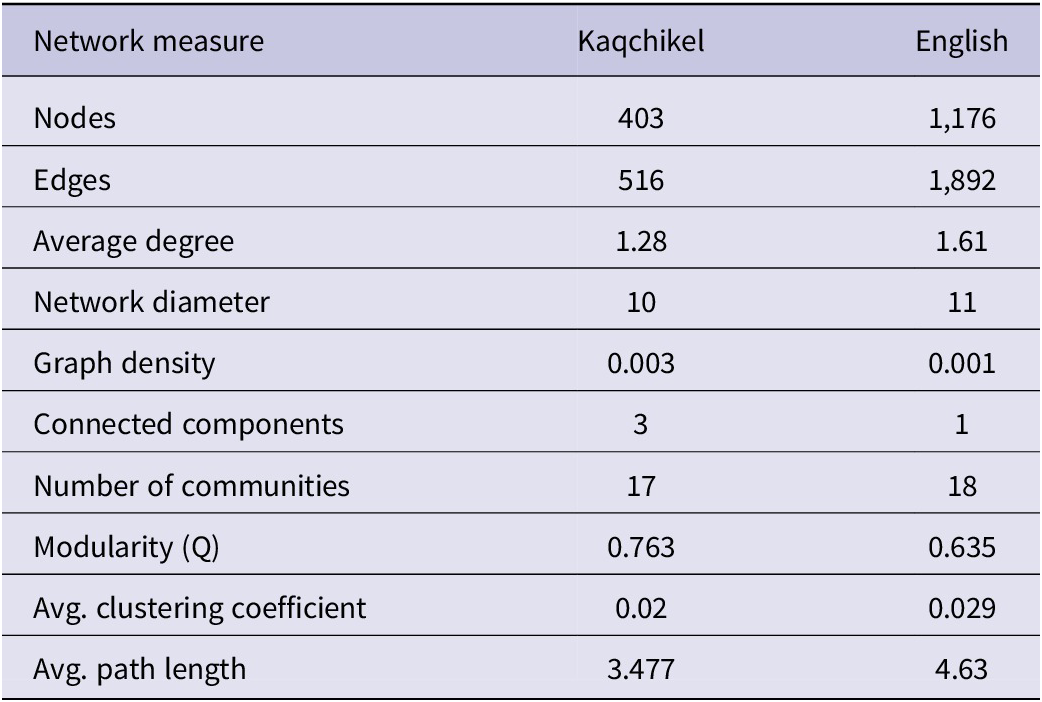
Many of the values reported in Table 1 are “points,” not mean values of a distribution. Because there are no degrees of freedom in such measures, conventional statistics cannot be used to assess the statistical significance of observed differences. Previous studies (e.g., Watts & Strogatz, Reference Watts and Strogatz1998; Vitevitch et al., Reference Vitevitch, Castro, Mullin and Kulphongpatana2023) used an informal heuristic of a value in one network being “different” if it is more than 1.5 times greater than the same measure from the other network. Using that heuristic, none of the measures differ “significantly,” except nodes, edges, graph density and connected components.
Although the monolingual English speakers and the Kaqchikel learners received the same 71 cue words, the results in Table 1 show that the network created from the responses from the English speakers had approximately 3 times as many nodes (i.e., words) and edges than the network created from the responses from the Kaqchikel learners. Similarly, when we consider the out-degree of just the 71 cue words we again see a statistically significant difference (using an independent samples t-test, t (140) = 24.68, p < .001), with the English speakers producing more responses to each cue word (mean = 26.69 words, SD = 6.20) than the Kaqchikel learners (mean = 7.27 words, SD = 2.36).
The larger number of responses from the monolingual English speakers (n = 90) compared to the Kaqchikel learners (n = 9) is of course due in part to the difference in sample size for each group. However, the difference in the number of responses from the two groups is also indicative of a difference in the variety of the responses provided by each group. Consider for example the responses to the cue word Ixïm (corn) for the Kaqchikel learners and the monolingual English speakers (Figure 1).
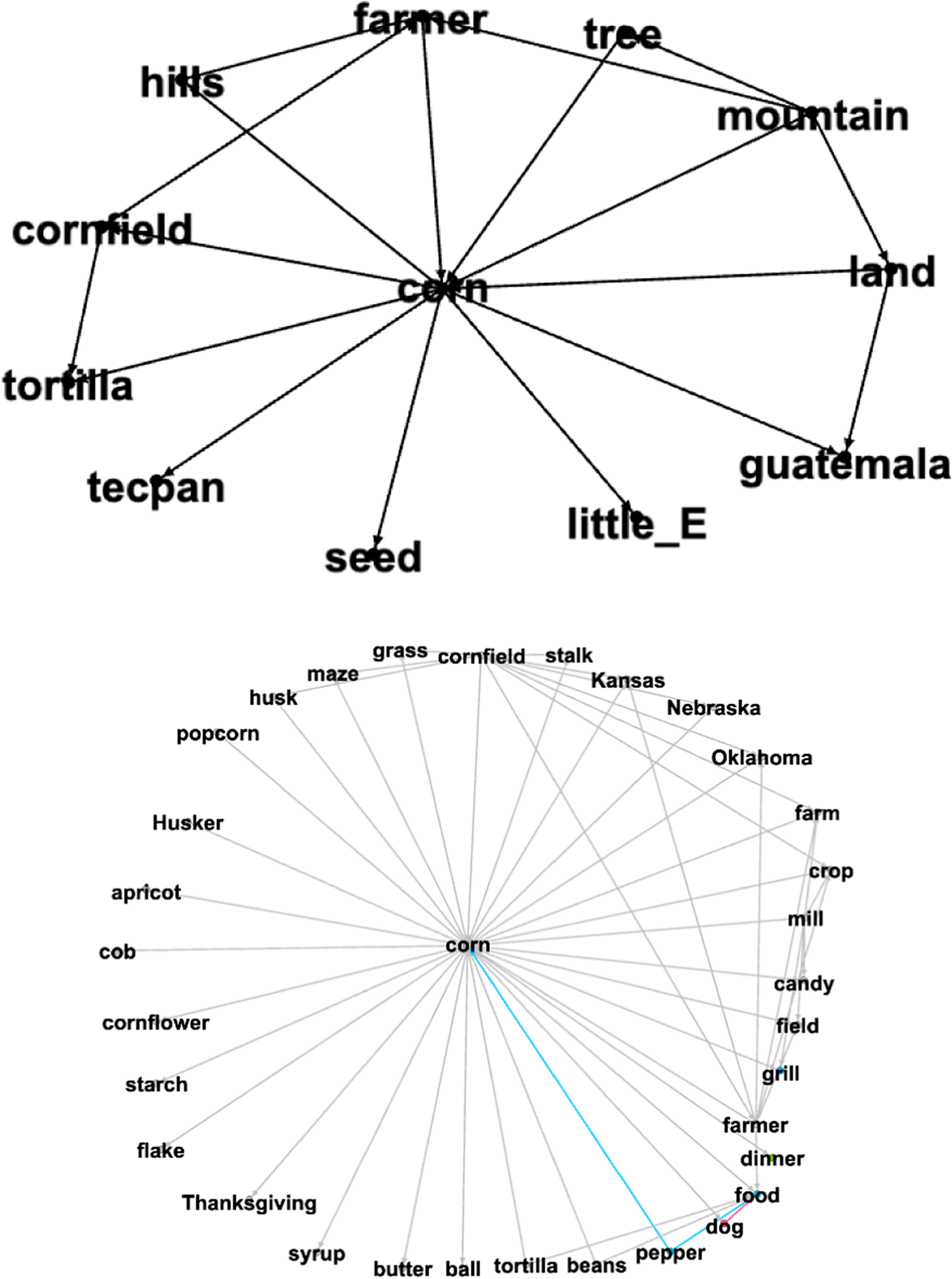
Figure 1. The top panel shows the ego network for Ixïm (corn) from Kaqchikel learners (with a density of 0.144). The bottom panel shows the ego network for corn from monolingual English speakers (with a density of 0.054).
Notice (in the examples in parentheses) that both groups produced responses related to locations where corn is grown (English: farm, crop, Kansas, Nebraska, field, cornfield; Kaqchikel: Guatemala, tecpán (Tecpán = a town in Guatemala), land, field, hills, mountain), food items related in some way to corn (English: tortilla, beans, syrup; Kaqchikel: tortilla), components of corn (English: cob, husk, stalk; Kaqchikel: seed), and occupations related to corn (English: Husker, farmer; Kaqchikel: farmer). Thus, both groups tended to focus on similar characteristics or features of the cue words.
However, the responses provided by the Kaqchikel learners appear to be more direct or utilitarian, whereas the responses provided by the English speakers appear to be more original or “creative” in nature (e.g., (corn)dog, (corn)flake, candy(corn), Thanksgiving, maze, (corn)starch, popcorn, etc.). Previous studies suggest that speakers of a foreign language make decisions about moral dilemmas that are more utilitarian in nature than native speakers of a language (Costa et al., Reference Costa, Foucart, Hayakawa, Aparici, Apesteguia, Heafner and Keysar2014; Planchuelo et al., Reference Planchuelo, Hinojosa and Duñabeitia2024; cf., Rühle & Lev-Ari, Reference Rühle and Lev-Ari2024). This so-called “foreign language effect” may have contributed to the difference we observed in how utilitarian (or creative) the responses were from Kaqchikel learners (or English speakers).
The observation that both groups focused on similar characteristics or features of the cue words casts some doubt on an explanation based completely on the foreign language effect. Further doubt is cast on the foreign language effect by findings of a diminished foreign language effect in bilinguals who are proficient in both languages (Degner et al., Reference Degner, Doycheva and Wentura2011; Dylman & Champoux-Larsson, Reference Dylman and Champoux-Larsson2020), suggesting that proficiency in L2 may influence the extent to which a response is utilitarian/creative in the present study. Recall that the participants in the present study were (self-reported) native English speakers with 18+ years of exposure to the language and learners of Kaqchikel enrolled at the same Midwestern university with approximately 5 months of exposure to the language. The more proficient English speakers had a richer, more nuanced semantic knowledge to draw upon for each cue word than the Kaqchikel learners who knew a limited number of words in that language. This enabled the English speakers to produce unique and creative responses, whereas the limited vocabulary of the Kaqchikel learners restricted them to common, rigid, utilitarian responses.
The hypothesis that the size or structure of the semantic network in novice/proficient language users might influence the utilitarian/creative nature of their responses is consistent with other work that examined the semantic networks of people who were high or low in creativity (Kenett et al., Reference Kenett, Anaki and Faust2014). Kenett et al. found that less creative individuals had semantic networks that were rigid, and had few connections between nodes. The semantic networks of more creative individuals had broader connections (connecting seemingly distant concepts) and were more spread out. Consistent with the idea that novice language users might produce less creative/utilitarian responses and proficient language users might produce more creative responses is the density of the Kaqchikel and English networks. As shown in Table 1 the Kaqchikel network (density = 0.003) was more rigidly connected than the English network (density = 0.001) where seeming disparate concepts might be connected. A difference in network density can also be seen in the ego-networks of the cue word Ixïm/corn shown in Figure 1 (with the density values reported in the caption).
Additional support for the hypothesis that the structure of the semantic network of novice/proficient language users might influence the utilitarian/creative nature of their responses can be seen in the number of components in each network. The English network had only 1 component, meaning that every node was connected in some way to every other node in the network. The implication of such a structure is that even disparate concepts can be reached and might appear to be related in some way.
In contrast, the network of the Kaqchikel learners had 3 components: a giant component (containing 409 of the 421 nodes that were connected to each other), and 2 smaller components that were not connected to the other components. (One of the small components contained the cue word taluwäch/television and 6 responses, and the other small component contained the cue word ti’ij /meat and 5 responses.) The implication in this case is that there is a literal “gap” in semantic knowledge (i.e., 3 components that are disconnected from each other), which makes it impossible to get from some concepts to others, and makes it increasingly difficult to use words in a flexible, novel, or original way to produce a “creative” response. The observation that learners of a language might have a semantic network with several disconnected components also suggests that language instructors might target vocabulary words that bridge together the components that are disconnected from each other to aid acquisition by better approximating the semantic structure of proficient/native speakers.
Consider further the responses from the proficient/native English speakers (in the bottom panel of Figure 1). Notice that several of the responses are compound words with complex compositional meanings (e.g., corndog, cornflake, candy corn, cornstarch, popcorn). The Common European Framework of Reference (CEFR) states that having “…a good familiarity with idiomatic expressions and colloquialisms…” is indicative of CEFR-level C2 “proficiency” (from the Spoken Interaction criterion) in a foreign language. Perhaps a related marker of proficiency in a foreign language might relate to the facility that speakers have in not only understanding compound words with complex compositional meanings but in creating novel, humorous concepts, such as cabbage cage, by pairing existing concepts (Siew et al., Reference Siew, Engelthaler and Hills2022). Further investigation of such responses from the English speakers in the present study may be useful in determining how a mechanism known as “generators” develops in the lexical network of a native speaker or an L2 speaker to model the creation of such novel concepts or relationships between existing concepts (Hills & Kenett, Reference Hills and Kenett2022).
More broadly, further investigation of the responses from the English speakers in the present study might contribute to questions about the way that morphology is represented and processed in the mental lexicon (Embick et al., Reference Embick, Creemers, Goodwin Davies, Papfragou, Trueswell and Gleitman2022). The investigation of Kaqchikel learners in the present study also highlights the importance of considering less taught/non-WISP languages with morphological systems that differ significantly from the English language when addressing questions about morphological processing, or when attempting to use networks to model the lexicon (Vitevitch et al., Reference Vitevitch, Martinez and England2024).
The present study demonstrates a novel way to use a semantic association task and the mathematical techniques from cognitive network science to study the mental lexicon of native speakers and language learners. For other examples of network science being used to investigate bilingualism see Borodkin et al. (Reference Borodkin, Kenett, Faust and Mashal2016)), Tiv et al. (Reference Tiv, Gullifer, Feng and Titone2020)), and Luef (Reference Luef2022).
Perhaps unsurprising, the results of the present analysis show that the semantic network of language learners is smaller than the semantic network of native speakers of a language. Crucially, however, the present analysis suggests that the more “loosely” connected structure of the semantic network of native speakers of a language may facilitate the production of linguistically creative responses (i.e., associating seemingly disparate concepts). In contrast, the rigid and disconnected structure of the semantic network of (novice) language learners may constrain their responses to be more common, straightforward, and utilitarian in nature.
Although the utilitarian decisions made by people in L2 compared to their native language (known as the “foreign language effect”) are often attributed to the reduced emotional response elicited by the L2 (Costa et al., Reference Costa, Foucart, Hayakawa, Aparici, Apesteguia, Heafner and Keysar2014), the present observation suggests an alternative interpretation. Namely, the size and structure of one’s semantic network may allow an experienced speaker to creatively connect disparate concepts (see Kenett et al., Reference Kenett, Anaki and Faust2014), or constrain a less experienced speaker (with a smaller and disconnected network) to produce responses that are more utilitarian and less creative in nature.
The present analysis also draws attention to less taught/non-WISP languages, and illustrates how studying such languages can lead to insights that might help researchers better understand how all languages (even L1) are learned (e.g., Luef, Reference Luef2022). For example, language instructors might use the tools of network science to map out the semantic or phonological knowledge of their students and target new vocabulary words that would bridge the “gaps” between disconnected clusters of related words. Finally, the present analysis provides another way in which the tools of network science can be used to understand another aspect of bilingualism (see also Borodkin et al., Reference Borodkin, Kenett, Faust and Mashal2016 and Tiv et al., Reference Tiv, Gullifer, Feng and Titone2020).
Acknowledgements
AM was supported in part by an Undergraduate Research Award from the Center for Undergraduate Research at the University of Kansas, and by the TRIO McNair Scholar Program from the Center for Educational Opportunity Programs at the University of Kansas.
Competing interest
The author(s) declare none.
Appendix A
Table A1. The 71 cue words used in the semantic associate task
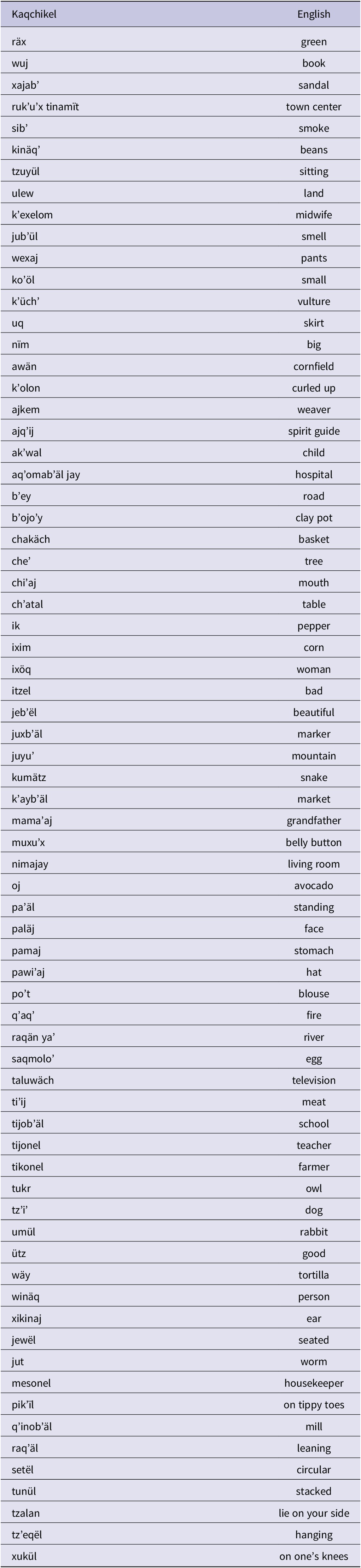
Appendix B
Definitions of common network measures. For formal definitions and information on how to calculate these measures, see Bastian, Heymann, and Jacomy (Reference Bastian, Heymann and Jacomy2009).
Nodes. Nodes represent individual entities in the system being studied, such as people who are friends with each other in a social network. In the present study, nodes represented words (i.e., cue words and responses).
Edges. Edges are connections placed between nodes that are related in some way. In a social network, edges would connect nodes/people who are friends with each other. The absence of an edge between two nodes/people would indicate that those two people do not know each other. Edges can be directed (as in the present study) indicating that the cue word/node elicited various response words. Edges can also be undirected indicating a bidirectional flow of information/goods/knowledge etc. In some networks, edges can be weighted to indicate the strength of the relationship between two nodes. For example, A and B might be friends since elementary school (and have a larger weight on the edge), whereas A and C met only recently (and would have a smaller weight on the edge). The edges in the present study were unweighted, meaning the strength of the relationships among nodes was assumed to be similar.
Average Degree. The average degree refers to the mean number of edges per node in the whole network.
Network Diameter. The network diameter refers to the shortest number of connections that must be traversed to connect the two nodes that are farthest from each other in the network.
Graph Density. Graph density is the ratio between the actual number of edges and the number of possible edges in the network. Values closer to 0 indicate that there is a small number of connections (compared to the number that is possible); such networks are said to be sparsely connected. Values closer to 1 indicate that there is a large number of connections (compared to the number that is possible); such networks are said to be densely connected. A value of 1 indicates that the network is fully connected (i.e, every node is connected to every other node in the network).
Connected Components. Connected component refer to nodes that are connected in some way to each other. The giant component is the component with the largest number of nodes connected to each other in some way. In the context of language networks, smaller components are sometimes referred to as “lexical islands” (Vitevitch, Reference Vitevitch2021).
Number of communities. A community is a group of nodes that are more connected to each other than they are to nodes in other communities. Communities are not the same thing as a connected component. Within a connected component, there may still be subgroups of (connected) nodes that tend to be more connected to each other than to other nodes in the component, thereby forming a community.
Modularity (Q). Modularity is a measure of how strong the division between components is in a network. Values closer to 0 indicate that the community structure is weak, whereas values closer to 1 indicate that the community structure is better defined.
Average Clustering Coefficient. The average clustering coefficient measures the extent to which nodes that are neighbors to a given nodes are also neighbors of each other. Values close to 0 indicate that the neighboring nodes tend to just be connected to a given node, whereas values close to 1 indicate that the neighboring nodes connect to the given node and have a high probability of being connected to each other as well.
Average Path Length. Path length refers to the shortest number of steps it takes to get from one node to another node. The average path length is the mean value for all possible pairings of nodes in the network.