There is a growing body of literature showing that just as phonological information is activated during the processing of written language (see, e.g., Frost, Reference Frost1998, for a review), orthographic information is activated during the processing of spoken language (see Frost & Ziegler, Reference Frost, Ziegler and Gaskell2007, for a review). There are, however, fewer studies on the role of orthography in second language (L2) spoken word processing. In this study, we are interested in how L2 learners with a formal instruction background use orthographic information in spoken word recognition.
Late L2 learners differ from native language (L1) speakers because they already use one phonological system that can influence the learning of another system (e.g., Best & Tyler, Reference Best, Tyler, Bohn and Munro2007), and because they are already familiar with the grapheme–phoneme correspondences of their L1, which can have a strong impact on the perception and learning of L2 sounds (Bassetti, Reference Bassetti2006; Escudero, Hayes-Harb, & Mitterer, Reference Escudero, Hayes-Harb and Mitterer2008; Escudero & Wanrooij, Reference Escudero and Wanrooij2010; Hayes-Harb, Nicol, & Barker, Reference Hayes-Harb, Nicol and Barker2010; Showalter & Hayes-Harb, Reference Showalter and Hayes-Harb2015). In addition, unlike L1 speakers who learn orthographic word forms only after the phonological forms have been established, literate L2 learners in formal instruction are exposed to written word forms early on in the learning process. The present study investigated how these L2 learners map L2 spoken words onto their written counterparts, specifically the extent to which this mapping is mediated by orthographic or phonological information, and to which L1 grapheme–phoneme correspondences are activated in this process. For this purpose, we conducted two experiments where spoken word forms had to be matched with their printed referents while participants’ eye movements were monitored. We also set out to evaluate the role of L2 proficiency in this matching process.
Even though orthographic information is not necessary in L1 spoken language processing, it is known to be activated even during (non-metaphonological) language processing tasks such as lexical decision that do not demand a special focus on the phonotactic or orthotactic structure of the word forms (Grainger, Diependaele, Spinelli, Ferrand, & Farioli, Reference Grainger, Diependaele, Spinelli, Ferrand and Farioli2003; Grainger & Ferrand, Reference Grainger and Ferrand1996; Salverda & Tanenhaus, Reference Salverda and Tanenhaus2010; Ventura, Morais, Pattamadilok, & Kolinsky, Reference Ventura, Morais, Pattamadilok and Kolinsky2004; Ziegler & Ferrand, Reference Ziegler and Ferrand1998, but see, e.g., Mitterer & Reinisch, Reference Mitterer and Reinisch2015, for the lack of orthographic effects in the perception of conversational speech). These orthographic effects have been explained by a simultaneous coactivation of phonological and orthographic representations (e.g., Grainger et al., Reference Grainger, Diependaele, Spinelli, Ferrand and Farioli2003) or by an activation of orthographically restructured phonological representations (Taft, Castles, Davis, Lazendic, & Nguyen-Hoan, Reference Taft, Castles, Davis, Lazendic and Nguyen-Hoan2008) during the processing of spoken words.
In the L1, the written forms of words are learned after their spoken forms, but in L2 instructed learning environments, the two modalities are learned in parallel. As a result of this co-structuration of orthographic and phonological information (Veivo & Järvikivi, Reference Veivo and Järvikivi2013), orthography may have a more important role in the L2 lexicon than in the L1 lexicon. Further, there is evidence that if the orthographic system of the L2 is incongruent (i.e., if the phonemes can be represented by several different graphemes or vice versa), parallel acquisition of orthography and phonology can be a hindrance to the acquisition of the L2 phonological system (Escudero, Simon, & Mulak, Reference Escudero, Simon and Mulak2014).
In L2 spoken word processing, the activation of orthographic information has been studied especially from the point of view of the parallel activation of the L1. For example, Bartolotti, Daniel, and Marian (Reference Bartolotti, Daniel and Marian2013) showed that during spoken word recognition in a newly acquired L2, orthographically similar L1 word forms are activated even if they are pronounced differently from the target words. This result is complementary to studies showing that phonologically similar words of both languages of bilingual or second language speakers compete for recognition in parallel (Blumenfeld & Marian, Reference Blumenfeld and Marian2007; Marian & Spivey, Reference Marian and Spivey2003a, Reference Marian and Spivey2003b; Spivey & Marian, Reference Spivey and Marian1999).
The role of orthographic input for the learning of L2 phonology has been widely studied (for reviews, see Bassetti, Reference Bassetti, Thorsten and Young-Scholten2008; Young-Scholten, Reference Young-Scholten, Burmeister, Piske and Rohde2002). There is evidence that orthography can help to acquire new phonemic categories of the L2 (Escudero et al., Reference Escudero, Hayes-Harb and Mitterer2008, Reference Escudero, Simon and Mulak2014; Showalter & Hayes-Harb, Reference Showalter and Hayes-Harb2013; Simon, Chambless, & Kickhöfel Alves, Reference Simon, Chambless and Kickhöfel Alves2010), but can also have a negative impact on the acquisition of L2 phonology (Bassetti, Reference Bassetti, Guder, Jiang and Wan2007; Bassetti & Atkinson, Reference Bassetti and Atkinson2015; Young-Scholten & Langer, Reference Young-Scholten and Langer2015), especially when the grapheme–phoneme relations of the L2 are different from the L1 (Escudero & Wanrooij, Reference Escudero and Wanrooij2010; Hayes-Harb et al., Reference Hayes-Harb, Nicol and Barker2010). Furthermore, there is evidence that late L2 learners in instructed learning environments can have an orthographic bias in their lexical knowledge, especially in the recognition of decontextualized word forms (Veivo, Suomela-Salmi, & Järvikivi, Reference Veivo, Suomela-Salmi and Järvikivi2015). At the same time, words for these learners can have imprecise phonological representations (Cook & Gor, Reference Cook and Gor2015; Cook, Pandža, Lancaster, & Gor, Reference Cook, Pandža, Lancaster and Gor2016), which may lead not only to the activation of false semantic content (Cook et al., Reference Cook, Pandža, Lancaster and Gor2016) but also to increased lexical competition (Broersma & Cutler, Reference Broersma and Cutler2011).
If the phonological representations of L2 words are more imprecise and unstable than those for L1 words, they may also be less well connected to their orthographic counterparts. As proficiency in the L2 increases, phonological representations are likely to become more accurate (Darcy, Daidone, & Kojima, Reference Darcy, Daidone and Kojima2013) and the orthographic bias in accessing semantic content decreases (Veivo et al., Reference Veivo, Suomela-Salmi and Järvikivi2015). Taken together, the lexicon of late L2 learners in instructed learning could be orthographically biased so that orthographic representations may be more robust than phonological representations. Moreover, this relative bias might decrease as proficiency increases. In the present study, we evaluated this orthographic bias hypothesis by examining the flow of information from spoken word forms to written word forms in late L2 learners at different proficiency levels.
Previous studies have shown that proficiency can influence orthographic activation in L2 spoken word processing: orthographic information during spoken word processing is activated more rapidly and more strongly in more proficient than in less proficient L2 learners (Mitsugi, Reference Mitsugi2016; Veivo & Järvikivi, Reference Veivo and Järvikivi2013; Veivo, Järvikivi, Porretta, & Hyönä, Reference Veivo, Järvikivi, Porretta and Hyönä2016). Specifically, Veivo et al. (Reference Veivo, Järvikivi, Porretta and Hyönä2016) used the visual world paradigm with printed referents and observed a significant effect for the degree of orthographic overlap of the vowels in targets and competitors (target: <mince> /mɛ̃s/ “slim” vs. O+ competitor: <mite> /mit/ “moth” or O– competitor: <mythe> /mit/ “mythe”), but only for higher proficiency participants. This suggests that orthographic information modulates L2 spoken word identification at least for higher proficiency learners. However, Veivo et al. (Reference Veivo, Järvikivi, Porretta and Hyönä2016) did not contrast the two types of within-language L2 competitors in the same experiment or investigate the activation of between-language competitors from the participants’ L1 to evaluate the activation of L1 orthography in L2 spoken word processing. The present study was designed to fill this gap.
CURRENT STUDY
In the present study, our main objectives were to investigate the mapping of spoken L2 words onto their written referents, and to evaluate whether this mapping is mediated mainly via orthographic or phonological information. For this purpose, we used the visual world eye-tracking paradigm (Allopenna, Magnuson, & Tanenhaus, Reference Allopenna, Magnuson and Tanenhaus1998; Cooper, Reference Cooper1974; Tanenhaus, Spivey-Knowlton, Eberhard, & Sedivy, Reference Tanenhaus, Spivey-Knowlton, Eberhard and Sedivy1995; for a review, see Huettig, Rommers, & Meyer, Reference Huettig, Rommers and Meyer2011) in a task where spoken words are matched with their written counterparts (cf. Huettig & McQueen, Reference Huettig and McQueen2007, Reference Huettig and McQueen2011; McQueen & Viebahn, Reference McQueen and Viebahn2007). We studied Finnish learners of French with a wide range of proficiency levels. The task in both experiments consisted of listening to spoken instructions in French (“cliquez sur le mot cidre”) and clicking on one of the four words (target, competitor, and two unrelated distractors) that appeared on the computer screen 200 ms before the acoustic onset of the target word. The spoken target words were accompanied by a high orthographic low phonological overlap (OH-PL) competitor (e.g., <cidre> /sidʀ/ “cider” vs. <cintre> /sɛ̃tʀ/ “coat hanger”) or a low orthographic high phonological overlap (OL-PH) competitor (e.g., <cidre> /sidʀ/ vs. <cycle> /sikl/ “cycle”) either in the L2 (Experiment 1) or in the L1 (Experiment 2).
If orthographic input in L2 acquisition leads to an orthographic bias in the lexical knowledge of late L2 learners (e.g., Young-Scholten, Reference Young-Scholten, Burmeister, Piske and Rohde2002; for a review, see Bassetti, Reference Bassetti, Thorsten and Young-Scholten2008), we expect orthographically similar competitors to delay the mapping more than phonologically similar competitors. If the precision of phonological representations depends on proficiency (Darcy et al., Reference Darcy, Daidone and Kojima2013), proficiency will affect the speed of the mapping process. Based on previous results (Veivo & Järvikivi, Reference Veivo and Järvikivi2013), lower proficiency learners might activate sublexical grapheme–phoneme correspondences of the L1, which would show as increased activation of phonologically similar L1 competitors in Experiment 2.
We started by investigating in Experiment 1 the matching of French spoken and written L2 word forms in the presence of within-language orthographic and phonological competitors.
EXPERIMENT 1
Method
Participants
Sixty-four students from the University of Turku participated for course credit or volunteered. They reported no hearing impairment or language deficits and had normal or corrected-to-normal vision. All participants were native speakers of Finnish who had learned French as a foreign language in instructed learning. None of the participants had acquired French or any other language besides Finnish before the age of 3. Their age of onset for L2 French varied between 5 and 45 (median = 14). This means that they were all either literate or had started to acquire literacy in their L1 when they began to learn the L2. The participants represented a wide range of proficiency levels ranging from beginners to highly proficient. They evaluated their proficiency in French for five subskills (listening, reading, spoken interaction, spoken production, and writing) with the CEFR self-assessment grid (2001, pp. 26–27). Each subskill was self-assessed on six levels, which were assigned values from 1 to 6. The maximum score for proficiency for each participant was therefore 30.Footnote 1 Participant-related background information is summarized in Table 1.Footnote 2
Table 1. Background information for participants (n = 64) in Experiments 1 and 2.

Materials
The visual displays comprised four words: target, competitor, and two distractors. There were 20 target words (e.g., <cidre>) each associated with either a OH-PL overlap competitor (e.g., <cintre>) or a OL-PH overlap competitor (e.g., <cycle>). In the OH-PL condition, targets and competitors had a word initial orthographic overlap of two letters so that the nucleus vowel of the first syllable was always spelled similarly but pronounced differently (e.g., <cidre> /sidʀ/ “cider” vs. <cintre> /sɛ̃tʀ/ “coat hanger”).Footnote 3 In the OL-PH condition, targets and competitors always had a word-initial phonological overlap of two sounds so that the nucleus vowel of the first syllable was pronounced similarly but spelled differently (e.g., <cidre> /sidʀ/ vs. <cycle> /sikl/ “cycle”). Each target (e.g., <cidre> /sidʀ/) and its competitors (vs. <cintre> /sɛ̃tʀ/ and <cycle> /sikl/) were associated with two distractor words that were orthographically, phonologically, and semantically unrelated.
The two competitors were matched for frequency (Lexique 3; New, Pallier, Ferrand, & Matos, Reference New, Pallier, Ferrand and Matos2001) as well as possible. The mean frequency of the OH-PL competitors was 43.7 per million and of the OL-PH competitors 47.9 per million. In addition, distractors in each display were matched for frequency with the target, 32.6 and 35.3 per million, respectively. Targets, competitors, and distractors were also matched for written length.Footnote 4 The 20 target word sets are listed in Appendix A. In addition to the target displays, 50 filler displays were constructed. In order to avoid the participants developing test-taking strategies and recognizing the target displays on the basis of formal similarity between the words, 20 of the filler displays had an overlap between the distractor words. In 10 of these filler displays, the distractors had an OH-PL overlap, and in 10 displays, the distractors had an OL-PH overlap. The remaining 30 filler sets comprised four words with no orthographic, phonological, or semantic overlap. In sum, Experiment 1 consisted of 70 trials (20 target word displays, 20 manipulated filler displays, and 30 filler displays).
Each target word was embedded in a French sentence instructing the participant to click on the target word (e.g., “cliquez sur le mot cidre”). These sentences were recorded digitally using the SANAKO Lab100 hardware in the Learning, Age and Bilingualism laboratory at the University of Turku. A female native speaker of French, unaware of the aims of the study, read the sentences in a randomized order with a brief prosodic break before each target word. The mean duration for target words was 616 ms.
Design and procedure
Each trial consisted of responding to the spoken instruction sentence (e.g., “cliquez sur le mot cidre”), by choosing the target word with a mouse click among the four words appearing on the computer screen. The position of each type of word was randomized for each display. For the target word displays, the competitors in the two experimental conditions were counterbalanced between two lists so that each list contained an equal number of OH-PL (10) and OL-PH (10) overlap competitors. The order for the presentation of the 70 trials was randomized for each participant, and the participants were assigned to the two experimental lists in the order of appearance.
Participants’ eye movements were monitored using a head-mounted SR EyeLink II eye-tracker (www.sr-research.com) sampling at 500 Hz. Each trial started with drift correction where the participants fixated on a small cross appearing in the center of the screen for the experimenter to accept the gaze accuracy. After that, the spoken instruction to click on the target word was given via headphones. The visual display (see Figure 1) appeared on the screen 200 ms before the onset of the target word (cf. Huettig & McQueen, Reference Huettig and McQueen2007; McQueen & Viebahn, Reference McQueen and Viebahn2007; Salverda & Tanenhaus, Reference Salverda and Tanenhaus2010). As it takes about 200 ms to program and launch a saccade after a stimulus is presented (Matin, Shao, & Boff, Reference Matin, Shao and Boff1993), this assured that the participants were not able to read the target words and have access to the phonological form via orthography before hearing the targets. The written words were presented in lowercase Times New Roman font being approximately 3 to 4 degrees wide, with the center of each word appearing approximately 8 degrees from the center of the screen (Figure 1).

Figure 1. An example of the visual display used in Exp. 1 and Exp. 2 (target: route, competitor: rouva, distractors: kansa & frère).
Before the main experiment, participants were familiarized with the task by presenting a practice block of 10 displays consisting of unrelated words. After that, they were presented with Experiments 1 and 2. The order of the experiments was counterbalanced between participants.
Results and discussion of Experiment 1
Five trials (0.5% of the data) were removed from the analyses because the participants clicked on the competitor word instead of the target word.Footnote 5 The proportion of looks to the targets, to the competitors, and to the distractors was determined for each trial and for each participant by calculating the number of looks to each word in 20-ms time bins. Mean proportions of looks to each type of word in the two experimental conditions for a 1200-ms period starting from target word onset are presented in Figure 2.

Figure 2. Mean proportion of looks to each type of word in the two experimental conditions in Exp. 1.
Proportions of looks to each type of word do not differ at word onset, but as Figure 2 shows, looks to distractors start to diverge from target and competitor looks in both experimental conditions at about 300 ms after the onset of the target word. Looks to competitors increase until around 500 ms, and looks to targets increase until reaching the asymptote around 1000 ms after onset. Therefore, we examined the data more in detail within a time window ending at this latter time point (200–1000 ms after target word onset). The proportions of fixations were logit-transformed for statistical analyses (Fox & Weisberg, Reference Fox and Weisberg2011),Footnote 6 providing an unbounded measure in which zero represents 50% of looks (Barr, Reference Barr2008).
Visual world eye-tracking data is inherently time-series data and usually presents nonlinearly over time (see Figure 2). In addition, it is possible that the time course interacts with other continuous variables, such as proficiency (cf. Veivo et al., Reference Veivo, Järvikivi, Porretta and Hyönä2016), which may also be nonlinear. We therefore used generalized additive mixed modeling (GAMM; Baayen, Vasishth, Kliegl, & Bates, Reference Baayen, Vasishth, Kliegl and Bates2017; Hastie & Tibshirani, Reference Hastie and Tibshirani1990; Wood, Reference Wood2006), which does not assume a linear relationship between predictors and the response variable and is capable of modeling interactions between continuous variables (here, time and proficiency; see Baayen et al., Reference Baayen, Vasishth, Kliegl and Bates2017; Baayen, van Rij, de Cat, & Wood, Reference Baayen, van Rij, de Cat, Wood, Speelman, Heylan and Geeraerts2018; Veivo et al., Reference Veivo, Järvikivi, Porretta and Hyönä2016). Furthermore, given the time series nature of the data, GAMM also allows for the control of autocorrelation in the data (see, e.g., Porretta, Kyröläinen, van Rij, & Järvikivi, Reference Porretta, Kyröläinen, van Rij, Järvikivi, Czarnowski, Howlett and Jain2018). Autocorrelation relates to the correlation between data points in a time series; a measurement at time point t is correlated to differing degrees with a measurement at time point t-i, depending on the lag. Autocorrelation is particularly problematic because it can greatly increase overconfidence of the model estimates.
In order to understand how online target word processing is modulated by proficiency and overlap, we modeled logit transformed looks to the target word as a function of time (200–1000 ms after target onset), proficiency (ranging from A1 to C2), and overlap condition (OH-PL vs. OL-PH). In addition, list and trial were included in the analysis as control variables. Finally, to control for individual variation in looking behavior, we created the variable event. Here, event represents the combination of participant and trial, capturing participants’ variable responses to different items in the experiment. Event was included in the model as a random effect, allowing each unique time series to have its own intercept in the model (Baayen et al., Reference Baayen, van Rij, de Cat, Wood, Speelman, Heylan and Geeraerts2016; Nixon, van Rij, Mok, Baayen, & Chen, Reference Nixon, van Rij, Mok, Baayen and Chen2016; Porretta, Tucker, & Järvikivi, Reference Porretta, Tucker and Järvikivi2016).
It is reasonable to expect that proficiency (a continuous variable) may influence the time course of processing nonlinearly. To allow for this, we used a tensor product (Wood, Reference Wood2006) for a nonlinear relationship between time and proficiency. Further, also using a tensor product, a difference surface (Baayen, Reference Baayen and Olson2010; Wood, Reference Wood2006) was included for overlap condition. This approach allows for the evaluation of the significance of the factor relative to the interaction of time and proficiency. In this case, the difference surface informs how and where OH-PL is different from the overall effect by adding an additional smoothing parameter on top of the main trend (Zuur, Ieno, Walker, Saviliev, & Smith, Reference Zuur, Ieno, Walker, Saveliev and Smith2009). Finally, trial order was included as a smooth term, and list was included as a parametric term.
The model was fitted to the data through a series of steps in order to assess the contribution of each variable. First, we fitted a full model (i.e., all the predictors, as described above). Second, autocorrelation was estimated from the data (ρ = 0.895, indicating a fairly high correlation between subsequent time points), and the model was refitted including this parameter to adjust the confidence of the estimates. Third, we evaluated the contribution of the individual predictors in the model. For this, two criteria were used: the p value of the term (indicating whether a given effect is not zero) and maximum likelihood (ML) score comparison between model variants (indicating whether the inclusion of the predictor improved the fit of the model; Zuur et al., Reference Zuur, Ieno, Walker, Saveliev and Smith2009). This process was done iteratively in a backward stepwise fashion until the model contained only predictors that were statistically significant and contributed to the model fit. Trial and the difference surface for overlap condition were removed through the fitting process, indicating that the order of presentation of the targets was not significant, χ2 (2) = 1.017, p = .362, nor was the type of overlap between targets and competitors, χ2 (5) = 1.182, p = .797.
ML score comparisons with chi-square tests between variant models justified including proficiency as an input variable, χ2 (3) = 30.192, p < .001. The resulting model contained the following predictors: event, experimental list, Time × Proficiency, and explained 30.6% of the deviance. The statistics for the parametric and smooth terms of the model with the best fit are summarized in Table 2. The significant effect of proficiency over time is depicted in Figure 3.
Table 2. Generalized additive mixed model with best fit for target looks in Experiment 1: Parametric coefficients and estimated degrees of freedom (Edf), reference degrees of freedom (Ref. df), F values, and p values for the tensor product


Figure 3. The effect of Proficiency over Time for target looks in Exp. 1.
In interpreting the GAMM results, visual inspection of the figures is essential, perhaps even more so than in other types of data analysis. Figure 3 presents the interaction between proficiency and time as a regression surface, showing that overall, as time progressed, participants were generally more likely to look at the target. Here darker shades of gray represent fewer looks to the target, whereas lighter shades of gray represent more looks to the target, and the contour lines indicate the rate of change.
More interesting, as proficiency increased, the participants were more likely to look at the target. Lower proficiency learners looked at the targets later than higher proficiency learners. Proficiency especially influenced processing in participants with proficiency scores under 15 (equal to CEFR-levels A1, A2, and B1) and did so in a graded fashion. For example, if we follow the time course for participants with proficiency scores 5 and 20, we find that lower proficiency participants were less likely to fixate the targets (between 400 ms and 600 ms). However, we can also see that the effect of proficiency was not linear along the proficiency continuum. This is evidenced by the shape of the contour lines, which indicate a strong effect of proficiency for participants with scores under 15 and little to no effect for participants with scores over 15.
The results of Experiment 1 fail to provide evidence that the OH-PL overlap between targets and within-language competitors delays the mapping between spoken and written forms more than OL-PH overlap. This suggests that when both orthographic and phonological competitors are present at the same time, both orthographic and phonological information is used in the matching process to a similar degree. We will return to this issue in detail in the General Discussion. However, our results confirm that the speed of target identification depends on L2 proficiency in a nonlinear fashion: more proficient L2 listeners fixate the targets faster than less proficient learners, and the influence of proficiency is more pronounced in the lower half of the proficiency scale.
As we were interested in how the L1 modulates L2 performance, we next moved on to investigate the activation of orthographic and phonological information from the participants’ L1 in the recognition of L2 word forms in Experiment 2. This experiment was designed to examine the impact of L1 orthography on the mapping process of the L2 at different proficiency levels.
EXPERIMENT 2
Method
Participants
Participants were the same as in Experiment 1.Footnote 7 Therefore, the two experiments were presented in a counterbalanced order between the participants.
Materials
As in Experiment 1, the visual displays consisted of four words: the target, the competitor, and two distractor words. Experiment 2 comprised 28 target words. Each French target word (e.g., <paume> /pom/ “palm”) was associated with a Finnish competitor with either a OH-PL overlap (e.g., <pauhu> /pauhu/ “roar”) or a OL-PH overlap with the target word (e.g., <pommi> /pom:i/ “bomb”). In the OH-PL condition, targets and competitors had a word-initial orthographic overlap of three letters so that the nucleus vowel of the first syllable was always spelled similarly but pronounced differently according to L1 phoneme–grapheme correspondences (cf. <paume> /pom/ vs. <pauhu> /pauhu/).Footnote 8 In the OL-PH condition, targets and competitors always had a word-initial phonological overlap of two sounds so that the nucleus vowel of the first syllable was always pronounced as similarly as possible (the vowel systems of the two languages not being the same) but spelled differently (cf. <paume> /pom/ vs. <pommi> /pom:i/). Each target (e.g., <paume> /pom/) and its competitors (e.g., <pauhu> /pauhu/ and <pommi> /pom:i/) were associated with two orthographically, phonologically, and semantically unrelated distractors, one in the L1 and the other in the L2. The visual display therefore always consisted of two words in the L2 (French), the target and the first distractor, and of two words in the L1 (Finnish), the competitor and the second distractor. Competitors were matched as well as possible for written frequencies reported in the unpublished Turun Sanomat newspaper lexical database (comprising 22.7 million word tokens) using the WordMill Lexical Search Program (Laine & Virtanen, Reference Laine and Virtanen1999). The mean frequency was 12.6 per million for the OH-PL overlap competitors and 29.1 per million for the OL-PH overlap competitors. Distractors were matched for frequency with the target. The mean frequency of the target words was 118.8 per million; the mean frequency of the distractor words was 109.7 per million (101.8 per million for the L1 distractors and 117.6 per million for the L2 distractors). The 28 target word sets are listed in Appendix B. In addition to the target set, we constructed a 28-item filler set that consisted of four words with no orthographic, phonological, or semantic overlap. In the filler sets, the target and another word were in the L2 (French) and two additional words were in the L1 (Finnish). The procedure for recording the targets was as in Experiment 1. The mean duration of target words was 592 ms.
Design and procedure
The design and procedure were identical to those in Experiment 1.
Results and discussion of Experiment 2
Before the analyses, 6 trials (0.3% of the data) were removed from the data because the participants erroneously clicked on the competitor word. As in Experiment 1, the proportion of looks to the targets, to the competitors, and to the distractors was determined for each trial and for each participant by calculating the number of looks to each type of word in 20-ms time bins. The mean proportion of looks to each word in the display for 1200 ms, starting from the target word onset, is depicted in Figure 4.

Figure 4. Mean proportion of looks to each type of word in the two experimental conditions in Exp. 2.
Visual inspection of the plots shows that looks to distractors start to diverge from competitor and target looks at about 300 ms and that looks to competitors start to decline around 600 ms after target word onset. Looks to targets increase until reaching the asymptote around 1000 ms. As in Experiment 1, the proportions of looks in each 20-ms bin were logit-transformed for statistical analyses to give an unbounded measure.
As in Experiment 1, we examined the time course of target identification more in detail in a time window from 200 ms until 1000 ms after the target word onset. Again, we used GAMM, and the model was structured exactly as in the analysis of Experiment 1. The model was fitted using the same steps and procedure as in Experiment 1. Through this process, an autocorrelation parameter of ρ = 0.895 was included, and trial was removed from the input variables. ML score comparisons with chi-square tests between variant models supported the inclusion of list, χ2 (1) = 6.599, p < .001, the difference surface for overlap condition, χ2 (6) = 11.860, p < .001, and proficiency, χ2 (6) = 27.278, p < .001, as input variables. The resulting model consisted of random intercepts for event, a parametric term for list, an interaction between time and proficiency, and a difference surface for overlap condition. This final model explains 38% of the deviance; the estimates for the parametric and smooth terms are summarized in Table 3.
Table 3. Generalized additive mixed model with best fit for target looks in Experiment 2: Parametric coefficients and estimated degrees of freedom (Edf), reference degrees of freedom (Ref. df), F values, and p values for the tensor products

Panel 1 of Figure 5 presents the interaction of proficiency and time as a regression surface. As in the results of Experiment 1, as time progressed, participants were generally more likely to look at the target. Again, lighter gray represent greater likelihood of target looks, while darker gray represents lesser likelihood of target looks, and the contour lines indicate the rate of change. Similar to Experiment 1, as proficiency increases, the participants were more likely to look at the target; lower proficiency learners were less likely to look at the target over time than higher proficiency learners. However, if we compare the shape of the contour lines in Figures 3 and 5 (Panel 1), we can see that this effect in Experiment 2 is less pronounced than in Experiment 1, affecting primarily the lowest proficiency learners with proficiency scores from 5 to 10 (CEFR levels A1 and A2).

Figure 5. The effect of Proficiency over Time for target looks (Panel 1) and the difference surface for OH-PL Overlap (Panel 2) in Exp. 2.
In contrast to Experiment 1, we also observed a significant adjustment due to overlap condition (i.e., significant difference surface), indicating that the Time × Proficiency surface for OH-PL (Figure 5, Panel 2) deviates significantly from the main Time × Proficiency surface (Figure 5, Panel 1). Between the two panels we see a greater influence of proficiency in the second half of the time course. As explained above, Panel 1 of Figure 5 presents the effect of proficiency over time for target looks in the whole data. To aid in the visualization of the effect of overlap condition, Panel 2 depicts the same effect but with the difference surface added to show the effect in the OH-PL-condition (e.g., <paume> /pom/ vs. <pauhu> /pauhu/). Compared to Panel 1, Panel 2 shows that, in the second half of the time course, participants with a proficiency score above 15 (CEFR-levels B2, C1, and C2) were more likely to fixate the target, whereas participants with proficiency scores below 15 (CEFR-levels A1, A2, and B1) were less likely to fixate the target. The significant difference surface for overlap F (8.425, 72,249.49) = 3.839, p < .001, indicated that this adjustment across proficiency was not zero after approximately 600 ms after target word onset.
Thus, when presented with orthographic competitors from the participants’ L1, higher proficiency learners were more likely to look at the target while lower proficiency learners were less likely to look at the target. In addition, learners in the upper half of the proficiency scale behaved more uniformly than learners in the lower half of the proficiency scale. In other words, lower proficiency learners showed more variation in the speed of finding the targets across proficiency scores.
The results of Experiment 2 indicate that the mapping between L2 spoken and written word forms is influenced by between-language competitors from the participants’ L1. This finding is in line with previous findings on language nonselective lexical access in L2 auditory word recognition (e.g., Lagrou, Hartsuiker, & Duyck, Reference Lagrou, Hartsuiker and Duyck2011). As in Experiment 1, the effect of proficiency on matching spoken and written word forms was more pronounced in lower proficiency participants. Furthermore, analysis of the time course of activation in the matching process with GAMM revealed an effect of L1 orthographic overlap that appeared 600 ms after target word onset: compared to the main trend, it was more difficult for the participants in the lower half of the proficiency scale to find the targets (e.g., <paume> /pom/) in the presence of OH-PL L1 competitors (e.g., <pauhu> /pauhu/). The effect of proficiency was also more pronounced in the presence of OH-PL L1 competitors for lower proficiency participants in this same time window.
These results suggest that in spoken word identification in late L2 learners, both orthographically and phonologically similar L1 words are activated early in the recognition process, but later on, orthographic information is activated more than phonological information. In contrast to Veivo and Järvikivi, (Reference Veivo and Järvikivi2013), we did not find evidence for the effect of L1 orthography depending on proficiency. It is likely that, unlike in masked priming that taps into the early phase of processing, in the kind of matching task used in the present study, lower proficiency learners did not rely on sublexical correspondences of the L1 more than on those of the L2. Instead, we observed significantly more activation for orthographically similar L1 words at all proficiency levels. Taken together, these results speak for an orthographically mediated activation in matching the spoken and written word forms, compatible with the orthographic bias hypothesis presented above.
GENERAL DISCUSSION
Our objective in the present study was to investigate the impact of orthographic and phonological information in the matching of spoken and written L2 word forms. This was done in the presence of within-language (Experiment 1) and between-language competitors (Experiment 2). We studied L1 Finnish late learners of L2 French and used the visual world eye-tracking paradigm with printed words as referents. We set out to evaluate the orthographic bias hypothesis, according to which orthographic representations in late L2 learners are more robust than phonological representations. This hypothesis makes the prediction that spoken L2 target words activate mainly orthographic representations, and therefore orthographically similar competitors in the L2 and L1 should delay the mapping between spoken and written L2 word forms more than phonologically similar competitors. Because phonological representations are likely to become more accurate as proficiency increases, proficiency was expected to affect the speed of target identification. For L1 competitors, we predicted that higher proficiency learners might show orthographic effects, whereas lower proficiency learners might rely on L1 sublexical correspondences, which would show as increased activation of phonologically similar L1 competitors.
The results of our within-language experiment (Experiment 1) showed that orthographically and phonologically similar L2 word forms are activated rapidly, around 300 ms after target word onset. This result is in line with previous visual world studies reporting phonological effects (e.g., Huettig & McQueen, Reference Huettig and McQueen2007) and orthographic effects in L1 speakers (Salverda & Tanenhaus, Reference Salverda and Tanenhaus2010). The results of our between-language experiment (Experiment 2) showed that spoken L2 words also activated orthographically and phonologically similar word forms in the participants’ L1, Finnish.
In the within-language experiment (Experiment 1), competitors with an OH-PL overlap did not delay target identification more than competitors with an OL-PH overlap. Written competitors in the OH-PL condition were selected so that the vowel of the nucleus was always pronounced differently from the targets (/sidʀ/ <cidre> vs. /sɛ̃tʀ/ <cintre>), whereas written competitors in the OL-PH condition were always selected so that the vowel of the nucleus was pronounced similarly to the targets (/sidʀ/ <cidre> vs. /sikl/ <cycle>). If the participants had unstable grapheme–phoneme correspondences in the L2, the competitors in the two overlap conditions were not necessarily phonologically different for them. In addition, because the two competitors were allowed to share word-final letters (such as <e>), the competitors may not have been orthographically different enough to produce a significant difference. This result does not necessarily rule out the orthographic bias hypothesis. It is possible that the activation spreads mainly via orthographic representations. In other words, a spoken word like /sidʀ/ activates its written counterpart <cidre>, which then sends activation to both <cintre> and <cycle>. This is likely especially if the learners are not aware of the pronunciation difference between the two written competitors.
In the between-language experiment (Experiment 2), the analysis revealed a nonlinear interaction between time, proficiency, and the type of overlap. When spoken L2 targets like /pom/ <paume> were presented with OH-PL competitors from the participants’ L1 (<pauhu> / pauhu/), these competitors delayed target identification significantly compared to the main trend in a time window from 600 ms to 1000 ms after target onset for lower proficiency participants. In addition, the effect of proficiency was more prominent in the presence of these OH-PL competitors for these participants in the same time window. Unlike in Experiment 1, the degree of phonological overlap between targets and competitors in Experiment 2 was unambiguous for participants at all proficiency levels, because the competitors were in the L1. According to L1 pronunciation rules, there was always a phonological mismatch between L2 targets and L1 OH-PL competitors. Therefore, spoken L2 words such as /pom/ <paume> could activate OH-PL competitors such as /pauhu/ <pauhu> only via orthographic representations.Footnote 9As predicted by the orthographic bias hypothesis, orthographic representations are activated significantly more than phonological representations at this relatively late time point.
Unlike we assumed on the basis of previous results (Veivo & Järvikivi, Reference Veivo and Järvikivi2013), lower proficiency learners were not relying on L1 grapheme–phoneme correspondences in Experiment 2, because written L1 competitors that could be pronounced similarly to the targets did not delay the matching. Instead, the delay in target word recognition due to orthographically similar L1 competitors was even more salient for lower proficiency learners. This is in line with the assumption that the orthographic bias would be more prominent in lower proficiency learners and decrease as proficiency increases. It is also possible that the higher proficiency learners were more able to suppress the irrelevant between-language information and that this suppression was enhanced when the orthographic information made it clearer what the unfolding word form would be. This interpretation is in line with the results of Blumenfeld and Marian (Reference Blumenfeld and Marian2013), which suggest that more efficient cognitive control is associated with reduced cross-linguistic activation in bilinguals in a relatively late phase of lexical competition (633–767 ms).
Based on previous research (e.g., Mitsugi, Reference Mitsugi2016; Veivo & Järvikivi, Reference Veivo and Järvikivi2013; Veivo et al., Reference Veivo, Järvikivi, Porretta and Hyönä2016), we assumed that proficiency would influence the speed of the matching process. Our results confirmed this assumption: in both experiments, higher proficiency learners identified the targets significantly faster than lower proficiency learners. This difference in processing speed suggests that lower proficiency learners have less precise phonological representations to base the matching on (cf. Cook et al., Reference Cook, Pandža, Lancaster and Gor2016). However, the effect of proficiency on the speed of looking at the targets was not linear throughout the proficiency continuum: the lower proficiency learners were not only generally slower in looking at the target than higher proficiency learners but also relatively slower the less proficient they were, unlike learners in the upper half of the proficiency continuum (CEFR-levels B2, C1, and C2) who behaved more homogenously in the task. This suggests that until this point, connections between the modalities develop gradually, but that above this point, they have mostly been acquired.
The results of the present study are discussed above in terms of coactivation orthographic and phonological information in spoken word processing (see, e.g., Grainger et al., Reference Grainger, Diependaele, Spinelli, Ferrand and Farioli2003). This same principle underlies the structure of the Bilingual Language Interaction Network for Comprehension of Speech (BLINCS; Shook & Marian, Reference Shook and Marian2013). In BLINCS, different levels of representation are represented by self-organizing maps that structure language input from both languages according to similarity between units. Further, ortholexical and phonolexical levels of representations are shared between both languages and interconnected via bidirectional links. In this respect, the architecture of BLINCS is similar to the interactive activation models of bilingual written word recognition like BIA+ (Dijkstra & van Heuven, Reference Dijkstra and van Heuven2002).
To our knowledge, there are no current models of L2 spoken word recognition that would comprise a possibility for interaction between the modalities. The BLINCS model was designed to model spoken word recognition in bilingual speakers, but it could be used to describe the coactivation of orthographic information in spoken word recognition also in late L2 learners. The results of the current study suggest that there are two important proficiency-related features that should be considered in modeling L2 spoken word recognition: the relative strength of ortholexical and phonolexical representations as well as the degree of activation of L1 orthography are different depending on the level of L2 proficiency. Although BLINCS assumes that both languages are known equally well, its architecture based on the self-organizing principle fits well for describing also L2 spoken word recognition. Lower proficiency learners have less experience with L2 word forms, especially in speaking, and this lower frequency in the input causes the phonolexical representations to become weaker, or fuzzier (see Cook et al., Reference Cook, Pandža, Lancaster and Gor2016), than ortholexical representations.
The results of our Experiment 2 indicate that lower proficiency learners are also less efficient in inhibiting the activation of L1 word forms. The results of Veivo and Järvikivi (Reference Veivo and Järvikivi2013) suggest that this effect may rise from the sublexical phoneme level. Further, this finding suggests that L2 spoken language processing mechanisms could be qualitatively different at different proficiency levels. In sum, our results show that orthography and L2 proficiency are factors that should be incorporated in any model of L2 spoken word recognition.
In the present study, we compared the use of orthographic and phonological information in the mapping of spoken and written L2 word forms. Based on studies on the importance of orthographic input in L2 acquisition (e.g., Young-Scholten, Reference Young-Scholten, Burmeister, Piske and Rohde2002), it was hypothesized that there is an orthographic bias in late L2 learners’ lexicons with orthographic representations being more robust and accurate than phonological representations. This bias predicts increased orthographic activation in L2 spoken word processing compared to phonological activation. The results of our between-language experiment are compatible with this hypothesis because orthographically similar L1 words delayed the matching process significantly more than phonologically similar L1 words. However, the results of our within-language experiment did not provide straightforward evidence in support of the orthographic bias, and therefore it should be evaluated in subsequent research. Finally, our results suggest that the competences needed for combining phonological and orthographic word forms develop significantly more in the lower half than in the upper half of the proficiency scale. Whether the findings on activation of orthographic information apply to more naturalistic L2 spoken word processing contexts remains a question for future research.
APPENDIX A
TARGETS AND COMPETITORS IN EXPERIMENT 1

APPENDIX B
TARGETS AND COMPETITORS IN EXPERIMENT 2
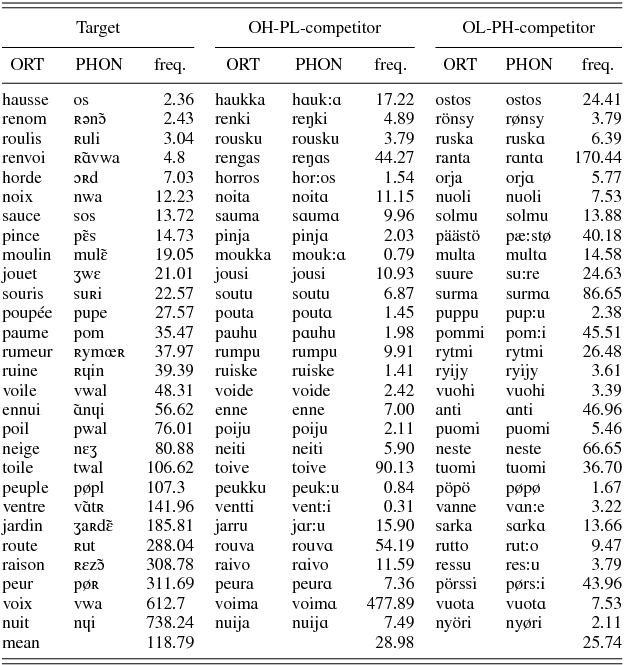