Introduction
In forming attitudes about political leaders, voters evaluate not just what leaders say, but how they say it. Facial expressions, voice pitch, and the sentiment of speech all offer salient emotional cues and thus provide key pieces of information for voters about the suitability of individuals for leadership positions (Boussalis and Coan Reference Boussalis and Coan2021; Carpinella and Bauer Reference Carpinella and Bauer2019; Madera and Smith Reference Madera and Smith2009; Sülflow and Maurer Reference Sülflow, Maurer, Veneti, Jackson and Lilleker2019). One place where these expressions are particularly important is in political debates. Not only are debates a central component of candidate selection in most democratic systems (Coleman Reference Coleman2000); they also offer a laboratory for understanding the interplay between verbal communication, nonverbal cues, and voter support for candidates.
Despite considerable academic interest in the study of political debates (Boydstun et al. Reference Boydstun, Glazier, Pietryka and Resnik2014; Druckman Reference Druckman2003; Fridkin et al. Reference Fridkin, Gershon, Courey and LaPlant2021; Nagel, Maurer, and Reinemann Reference Nagel, Maurer and Reinemann2012), questions remain on how emotional displays translate into support among potential voters. Voters evaluate candidates not only on whether they express situationally-appropriate emotions (Brooks Reference Brooks2011) but also whether their emotions convey an ability to lead and to work with others (Boussalis and Coan Reference Boussalis and Coan2021; Masch and Gabriel Reference Masch and Gabriel2020). Candidates are well aware of these expectations and concentrate on displaying emotions that are congruent with leadership roles (Bucy Reference Bucy2016; Masch Reference Masch2020). However, not all individuals seeking leadership positions are equally able to leverage emotional expressions to gain support because voters do not respond to every candidate’s behavior in the same way. Voters apply differing expectations based on the socially meaningful identities of candidates (Hess et al. Reference Hess, Senécal, Kirouac, Herrera, Philippot and Kleck2000), and these identities may further constrain the range of emotions that candidates choose to use. Gender is one such identity (Bauer Reference Bauer2019; Bauer and Carpinella Reference Bauer and Carpinella2018; Renner and Masch Reference Renner and Masch2019). In this paper, we ask, “How does gender shape emotional expression by candidates and voter reactions to these emotions?”
We begin by developing a new theoretical framework that explicitly incorporates gender into explanations of routine emotional displays in leadership debates. Applying gender role theory (Eagly and Karau Reference Eagly and Karau2002), we argue that men and women running for political office will attempt to use emotions in interpersonal exchanges that are associated with political power and their gender (Bucy and Grabe Reference Bucy and Grabe2008; Dittmar Reference Dittmar2015). Voters will respond to these displays, supporting candidates who engage in gender- and role-congruent emotional expression. In doing so, our study brings together research on how candidates use emotions as a functional tool in campaigning with scholarship on how gender constrains the behavior of men and women. Our approach differs from previous examinations of emotions in politics: up to now, the vast majority of research in this area has relied on observational work limited to single debates and does not consider gender. Research on gender and emotions, on the other hand, often relies on extreme emotional displays and experimental approaches that only examine voter reactions. Against this backdrop, our research relies on computational methods to produce and combine multimodal sources of candidate emotion, including indicators of nonverbal, verbal, and vocal emotive displays, with real-time evaluations of voters during televised debates (Boydstun et al. Reference Boydstun, Glazier, Pietryka and Resnik2014).
We test our expectations using a case study of German national elections, drawing on four televised debates that feature Angela Merkel versus her male opponents (2005, 2009, 2013, and 2017) and a debate for smaller parties (2017) that features two women candidates. German debates provide an ideal setting for understanding the role of emotions in politics because they are viewed as the most important event during an election campaign. We argue that Germany—and Angela Merkel—provides a critical case study for understanding the role of gender in leaders’ behavior and voter reactions, as she is arguably the world’s most powerful womanFootnote 1 and is highly constrained in her public behavior (Mushaben Reference Mushaben2017).
To assess our expectations about nonverbal communication and voter response, we examine the images and sound from over 596,000 frames and 22,500 seconds (or more than six hours) across these five debates. Innovations in computational methods for multimodal data collection and analysis offer new opportunities to study how candidates communicate and how voters respond to this communication in real time (Bakker, Schumacher, and Rooduijn Reference Bakker, Schumacher and Rooduijn2021; Dietrich, Hayes, and O’Brien Reference Dietrich, Hayes and O’Brien2019; Joo, Bucy, and Seidel Reference Joo, Bucy and Seidel2019; Masch Reference Masch2020; Williams, Casas, and Wilkerson Reference Williams, Casas and Wilkerson2020). We draw on these innovations to combine emotions detected from facial displays, vocal pitch, and sentiment with real-time responses using representative samples of voters from debates across multiple electoral cycles (Maier and Faas Reference Maier and Faas2019; Nagel, Maurer, and Reinemann Reference Nagel, Maurer and Reinemann2012). Using tools from computer vision, we extract expressions of anger, happiness, and overall levels of facial emotive engagement and combine this with estimates of emotional intensity from vocal pitch and the sentiment of words spoken via text analysis.
Our study offers a number of key findings. First, we find that Merkel expresses less anger than her male opponents, as do the women in the 2017 minor party debate. Second, given the social expectation that women should be communal and caring (and not agentic and aggressive; Cassese and Holman Reference Cassese and Holman2018), we argue that voters will reward women seeking political office who increase expressions of happiness, limit their expressions of anger, and express more emotions overall. Consistent with our expectations, we find that viewers tend to reward Merkel for expressing happiness and punish her for expressing anger, with the opposite effects for her male counterparts. Voters also respond positively when Merkel expresses more emotion (as measured both by her facial expressions and vocal pitch). We find similar effects for female candidate displays in the minor party debate, which highlights the role that gender plays in candidate behavior and voters’ assessments of politicians.
In many ways, our paper’s data are an embarrassment of riches: few scholars have access to multiple iterations of debates that hold the setting constant while examining interpersonal emotional expression, nor is it common to have real-time voter reactions, obtained through a consistent method and from a representative group of voters, across multiple years of debates. That Angela Merkel appears in each of the major debates is an additional benefit, as we can compare her behavior over time. The supplement of the debate between minor party leaders, which featured two other women candidates, provides us with an opportunity to examine how our results replicate with other leaders. Taken together, our fine-grained data on candidates’ repertoire of multiple modes of communication and voter reactions provide a new and unique view of gender and emotions in politics.
Nonverbal and Emotional Communication in Politics
Political leaders seek to garner favor among voters through their words, voices, and facial expression; these “hearts and minds” appeals shape voter evaluations (Carpinella et al. Reference Carpinella, Hehman, Freeman and Johnson2016; Everitt, Best, and Gaudet Reference Everitt, Best and Gaudet2016; Fridkin et al. Reference Fridkin, Gershon, Courey and LaPlant2021). Nonverbal communications—including facial displays and vocal pitch—are a key mechanism by which candidates convey emotions and, in turn, influence voter assessments regarding the acceptability of candidates for leadership positions. Voter’s attitudes can be shaped by candidate nonverbal expressions (Stewart, Salter, and Mehu Reference Stewart, Salter and Mehu2009), including inferring candidate traits like competence and trustworthiness from vocal pitch (Anderson and Klofstad Reference Anderson and Klofstad2012; Carpinella et al. Reference Carpinella, Hehman, Freeman and Johnson2016; Klofstad, Anderson, and Nowicki Reference Klofstad, Anderson and Nowicki2015).
While candidates do not want to appear as too emotional, they also do not want to be perceived as apathetic—candidates will thereby seek to balance the intensity of their emotional expression. Political candidates must also express emotions that are congruent with the role they seek. The acceptability of both the overall level of emotion and the specific emotions expressed by individuals in leadership contests are deeply rooted in evolutionary biology. Humans interpret facial displays of emotions as “ritualized signals” that dictate and maintain relationships (Eibl-Eibesfeldt Reference Eibl-Eibesfeldt, Von Cranach, Foppa, Lepenies and Ploog1979). Besides, humans have “built-in biases to perceive certain gestures and physiognomies as social dominance messages” (Keating Reference Keating, Ellyson and Dovidio1985, 105). Leader facial displays of anger and happiness have the capacity to signal a dominant status to potential followers, while displays of fear and sadness convey submissiveness (see Stewart, Salter, and Mehu Reference Stewart, Salter and Mehu2009).Footnote 2 Therefore, the human desire to select leaders who can “dominate others, and thus show how he or she is able to neutralize external as well as internal threats to the group” means that voters may prioritize candidates who express anger and other agonistic emotions (Boussalis and Coan Reference Boussalis and Coan2021, 7).
Yet, the appearance of domination also needs to be controlled and situationally appropriate, as voters shy away from leaders who would exert too much control over the group (Stewart, Salter, and Mehu Reference Stewart, Salter and Mehu2009). Research also suggests that voters respond positively to the expression of happiness (Sullivan and Masters Reference Sullivan and Masters1988), as this signals the ability of the leader to interact appropriately with others (Masch Reference Masch2020). Thus, people want leaders to express happiness and other hedonic emotions, which represent the ability to affiliate with others. These role expectations shape both candidate behavior, where those seeking political power try to limit their expressions to a narrow range of acceptable emotions (Boussalis and Coan Reference Boussalis and Coan2021; Dittmar Reference Dittmar2015). Individuals seeking political office are well aware of the role congruity expectations that voters have, and they try to express appropriate emotions that will communicate a dominant rank. Displays that signal submissiveness, such as fear and sadness, are deemed incompatible with political leadership and are avoided by office-seeking candidates. This bears out empirically. Studies of candidate nonverbal displays during US elections show that candidates rarely display fear or sadness (Boussalis and Coan Reference Boussalis and Coan2021; Bucy and Grabe Reference Bucy and Grabe2008; Masters et al. Reference Masters, Sullivan, Feola and McHugo1987).
Gender, Emotional Expression, and Voter Reactions
Not all individuals seeking leadership positions are equally able to leverage emotional expressions to gain support because voters do not respond to every candidate’s behavior in the same way. Indeed, “political candidates differ widely in the effectiveness of their nonverbal behavior” (Grabe and Bucy Reference Grabe and Bucy2009, 148). These divergent reactions can be due to charisma, attractiveness, political party, age, and, importantly for us, gender.
Gender shapes which emotions people express, the levels of those emotions, and how others react to those expressions (Bauer and Carpinella Reference Bauer and Carpinella2018; Hess et al. Reference Hess, Senécal, Kirouac, Herrera, Philippot and Kleck2000; Masch Reference Masch2020; Meeks Reference Meeks2012). Gender role theory posits that men and women are socialized into particular roles in society (Barnes, Beall, and Holman Reference Barnes, Beall and Holman2021; Eagly and Karau Reference Eagly and Karau2002). Women are expected to hold communal characteristics, including being “affectionate, helpful, kind, sympathetic, interpersonally sensitive, nurturant, and gentle” (Eagly and Karau Reference Eagly and Karau2002, 574). In comparison, men are expected to present agentic traits, which include being decisive, assertive, and strong leaders. Research suggests that these gendered expectations further constrain both verbal and nonverbal behavior (Everitt, Best, and Gaudet Reference Everitt, Best and Gaudet2016).
Gender role socialization leads to gender differences in the type of emotions express as well as the overall level of these emotions. Women are socialized to feel and express a greater intensity of emotions overall (Kring and Gordon Reference Kring and Gordon1998) and especially the emotions—such as happiness—that facilitate communal skills (Brody Reference Brody2009).Footnote 3 Men, alternatively, are socialized to express fewer emotions generally, but when they do express emotions, they are consistent with the male gender roles of assertiveness and leadership, such as anger (Schneider and Bos Reference Schneider and Bos2019).
These gender roles produce congruency expectations, such that women are expected to act “like women” and men are expected to act “like men” (Eagly and Karau Reference Eagly and Karau2002; Schneider and Bos Reference Schneider and Bos2019). If individuals engage in gender congruent behavior, they receive internal and external rewards while gender incongruent behavior is punished (Bauer Reference Bauer2017; Cassese and Holman Reference Cassese and Holman2018; Eagly and Karau Reference Eagly and Karau2002). These expectations spill over to emotional and nonverbal behavior, where people believe women to be more emotional generally and to express a broader range of emotions, with the exception of anger and pride (Plant et al. Reference Plant, Hyde, Keltner and Devine2000). As such, a woman can be punished for expressing anger and rewarded for happiness and sadness, while a man may experience the opposite (Fischbach, Lichtenthaler, and Horstmann Reference Fischbach, Lichtenthaler and Horstmann2015; Hess et al. Reference Hess, Senécal, Kirouac, Herrera, Philippot and Kleck2000; Meeks Reference Meeks2012).
Yet, gender does not just shape the emotional expression and reactions in the general population. Gender functions in the “processes, practices, images and ideologies, and distribution of power” in society and especially in politics (Acker Reference Acker1992, 567). There thus emerges a challenge for women seeking leadership roles: because of general expectations about the characteristics of leaders, voters may support politicians who express anger and happiness, albeit at appropriate levels (see Brooks Reference Brooks2011). However, gender role expectations mean that women should express happiness and sadness. Women seeking political office are highly aware of the potential of gendered expectations about their behavior from voters (Dittmar Reference Dittmar2015). The easiest solution, then, for women and men seeking positions of power, is to express the emotions that are both political role and gender role consistent, such that
Candidate-H1: Women seeking office will express more happiness than will men, and men will express more anger than will women.
Because we have clear expectations about the emotions of anger and happiness from both leadership role congruity and gender role congruity, we focus on those two discrete emotions. While scholars generally agree that fear and sadness harm candidate images (and thus are rarely found in situations like political debates), other emotions like disgust may be meaningful. Yet it is unclear both how a candidate would be punished or rewarded for such an expression or the role that gender would play.
As we previously noted, voters want leaders who express role-congruent emotions (Klofstad, Anderson, and Nowicki Reference Klofstad, Anderson and Nowicki2015). But voters also apply varying standards to how women and men in public office look and sound (Bauer and Carpinella Reference Bauer and Carpinella2018; Carpinella and Bauer Reference Carpinella and Bauer2019) and may want women and men who express gender-role-congruent emotions (Fischbach, Lichtenthaler, and Horstmann Reference Fischbach, Lichtenthaler and Horstmann2015). In Germany, Masch and colleagues find voters react positively when leaders express happiness (Gabriel and Masch Reference Gabriel and Masch2017; Masch Reference Masch2020). Research also suggests that voters are particularly unlikely to accept masculine behavior from women. For example, research on nonverbal displays and gender finds that voters do not react to men’s agentic nonverbal displays but see women as less likeable when they engage in displays of dominance (Copeland, Driskell, and Salas Reference Copeland, Driskell and Salas1995; Everitt, Best, and Gaudet Reference Everitt, Best and Gaudet2016). If voters want gender- and leader-consistent emotional expression, we would expect that
Voter-H1: Voters will reward women’s happiness and punish their anger, relative to men’s expression of happiness and anger.
Voters may evaluate men and women by not only the specific emotions that they express but also their overall level of emotional expression. Recall that gender role socialization suggests that women are granted broader leeway for general emotional expression and are assumed to feel and express a fuller range of emotions (Plant et al. Reference Plant, Hyde, Keltner and Devine2000). Thus, if men and women in political office behave in a gender-role-congruent manner, we would expect
Candidate-H2: Women will express more emotions overall compared with men.
If voters want leaders who conform to gender roles, they may reward women’s higher levels of emotional expression, even in political settings where emotions are expected to be controlled (Gleason Reference Gleason2020; Masch Reference Masch2020). People generally believe that women express more emotions than do men (Hess et al. Reference Hess, Senécal, Kirouac, Herrera, Philippot and Kleck2000). As such, we expect that
Voter-H2: Voters will react more positively to any emotional expression by women compared with men.
Figure 1 provides an overview of our theoretical expectations at the candidate and voter levels of analysis.
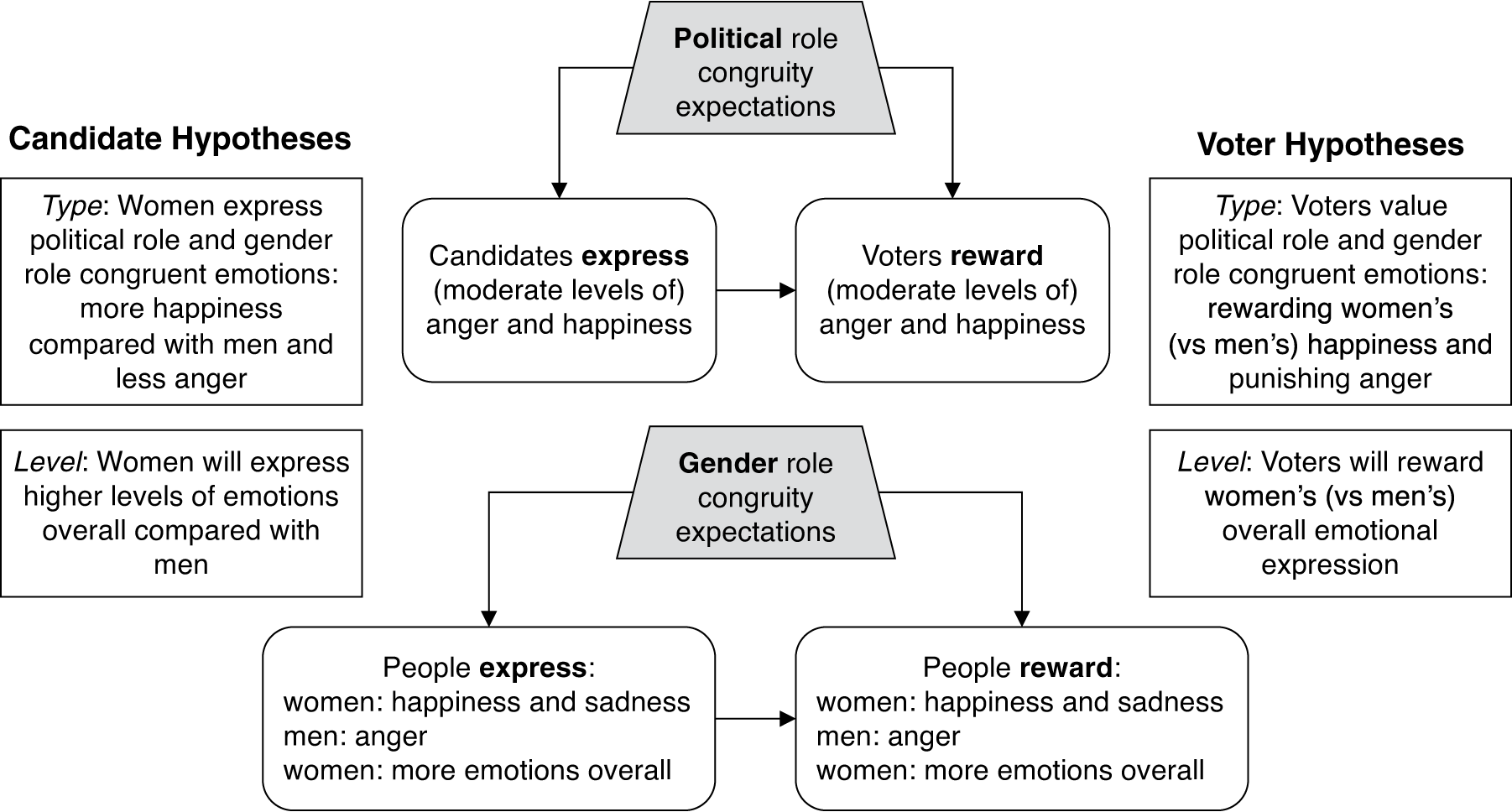
Figure 1. Theoretical Expectations
Political Debates as Emotion-Rich Environments
Political debates are an ideal setting for assessing the role of emotions in candidate behavior and voter decision making because they offer an opportunity for voters to assess not only how candidates present themselves in isolation but also how they compare directly to each other. Studies of debates demonstrate that voters obtain information about candidate traits and electability from on-stage exchanges, and debate performance can ultimately influence vote choice (Benoit, Hansen, and Verser Reference Benoit, Hansen and Verser2003). Of importance for our work, scholars have shown that seeing and hearing debates shifts how people view the candidates (Druckman Reference Druckman2003; Fridkin et al. Reference Fridkin, Gershon, Courey and LaPlant2021).
The debate performance of candidates—and how voters react to those performances—are shaped by the gender composition of who is on stage. We are far from the first to evaluate how gender shapes the use of or response to emotions (e.g., Hess et al. Reference Hess, Senécal, Kirouac, Herrera, Philippot and Kleck2000), including in the political arena (e.g., Bauer Reference Bauer2015; Brooks Reference Brooks2011; Reference Brooks2013; Masch and Gabriel Reference Masch and Gabriel2020). Our approach does differ considerably from previous research that has evaluated the intertwined nature of gender, emotions, and candidate behavior. Foremost, we combine an evaluation of both how political leaders use emotions as functional displays (Van Kleef and Fischer Reference Van Kleef and Fischer2016) and how voters react to those displays. In doing so, we argue that political leaders are deeply aware of which emotive signals voters might deem acceptable and unacceptable; this is particularly true for women seeking positions of power (Dittmar Reference Dittmar2015).Footnote 4 Because of this, it is important to consider the natural presentation of emotions in politics and how voters react to that presentation. This is very different than, for example, an approach that artificially manipulates the description of political leaders who express extreme emotions (i.e., Brooks Reference Brooks2013; Cassese and Holman Reference Cassese and Holman2018). After all, we regularly witness candidates expressing emotions and doing so purposefully and strategically. For instance, Bucy and Grabe (Reference Bucy and Grabe2008) find that political candidates modulate their use of anger displays between relaxed interview settings and more competitive televised debates, opting to show more anger in the latter situation (but the study does not consider gender). We can thus center both candidate strategic behavior and how voters will respond to those displays. Our use of political debates lets us assess how men and women engage in interpersonal emotional displays (Van Kleef and Fischer Reference Van Kleef and Fischer2016), which is a departure from much of the previous work in this area (but see Masch and Gabriel Reference Masch and Gabriel2020). Thus our hypotheses can be directed at assessing comparative behavior between men and women within the same interactive environment; in our case, we use the German leadership debates as our venue.
Our Case: Leadership Debates in Germany
We test our expectations using a case study of German national leadership debates, including a novel combination of data across four (2005, 2009, 2013, and 2017) national debates for the main political parties and a single debate (2017) between minor party leaders. We do not have access to audience reactions for the first televised debates between Gerhard Schröder and Edmund Stoiber in 2002; this debate also does not feature variation on candidate gender.Footnote 5 We argue that these debates provide favorable conditions of internal and external validity for assessing the role of emotions in politics.
Leadership debates play a particularly important role in German politics, with more than 20% of the German electorate watching each debate. The way German election campaigns are financed further elevates the salience of these debates. Parties have strict spending limits, can air only a few ads on TV, and mainly rely on posters, face-to-face campaigning, print advertisements, and social media. The TV debate is the only opportunity to directly address a large proportion of the electorate. As a result, emotional displays during these 90 minutes could potentially convince or deter voters (Maier and Faas Reference Maier and Faas2019) and previous findings underscore the important role that emotions play in German debates and talk shows (e.g., Masch Reference Masch2020). The central role that debates play in German politics is also consistent with the electoral approach in other countries. Most democracies regularly conduct leaders’ debates between candidates or party representatives, and all countries in Europe have held televised debates in the past (Online Appendix Section A: Televised Debates around the World).
Angela Merkel’s participation in these debates provides a unique opportunity to understand gender in debates. As with many other women in power, she came into office during a time of crisis (Beckwith Reference Beckwith2015), was an outsider candidate (Clemens Reference Clemens2006), and matches “the prevailing model of the more constrained and collaborative female executive” (Jalalzai Reference Jalalzai2011, 428). Given the scarcity of women as the heads of powerful nations, Merkel offers us the ideal—and rare—opportunity to study the role of gender and emotions in national political debates.
Merkel’s political style, moreover, suggests that these debates may be a circumstance where we are least likely to find gendered effects for emotions. Merkel has an “almost apolitical style” (Clemens Reference Clemens2006, 43). While Merkel has had “no choice” but to run as a woman for political office (Ferree Reference Ferree2006, 94), Merkel’s personal style is to appear “rational, calm, prudent, and unflappable” (Qvortrup Reference Qvortrup2017, 17). Merkel herself thus may be unlikely to express emotions overall; Masch and Gabriel (Reference Masch and Gabriel2020, 160) note that “Chancellor Merkel does not immediately come to mind as a political leader strongly relying on emotional appeals in the mobilisation of political support.”
The power of her position would also point us toward being unlikely to find gendered effects. Very few women serve as the leaders of their parties in parliamentary democracies generally (O’Brien Reference O’Brien2015), and the structure of German politics and the power of the chancellor constrain women’s access to this powerful position. (Beckwith Reference Beckwith2015; Xydias Reference Xydias2013). Voters may see women in political office through the lens of their position, not their gender, and evaluate their behavior compared with acceptable actions from politicians (Brooks Reference Brooks2013). As the position increases in power, voters may be increasingly less likely to apply gendered expectations to a woman’s behavior (Schneider and Bos Reference Schneider and Bos2019).
The debates themselves offer an ideal setting for testing the role of gender and emotions in politics. Angela Merkel participated in all four of the main debates, starting with competing against the incumbent chancellor Gerhard Schröder in 2005. After the 2005 election, Merkel led a grand coalition between the Christian Democrats (CDU/CSU) and the Social Democrats (SPD). In the three subsequent debates, Merkel (as incumbent chancellor) faced three male candidates from the SPD: Frank-Walter Steinmeier, Peer Steinbrück, and Martin Schulz (Bowler, McElroy, and Müller Reference Bowler, McElroy and Müller2021). We supplement our evaluation of these main debates with data from a 2017 debate featuring the candidates of the five smaller parties with a promising chance of entering the German Bundestag. Notably for our purposes, it also featured women for the first time. Sahra Wagenknecht, the candidate of the far-left (The Left), and Alice Weidel, the candidate of the right-wing populist Alternative for Germany (AfD), competed against three male competitors from the Green Party (Cem Özdemir), the liberal Free Democratic Party (Christian Lindner), and the Christian Social Union, the Bavarian counterpart of the CDU (Joachim Herrmann). We describe the context of the four elections and the perceptions of the candidates’ performances during the debates in Online Appendix Section B: The German Debates.
Measuring Candidate Multimodal Emotion Displays
We employ a set of computational methods to extract granular visual, vocal, and verbal information of debate participants and combine these data with second-by-second real-time response measurements from focus group subjects who watched the debates live (Boussalis et al. Reference Boussalis, Coan, Holman and Müller2021). The following sections describe in detail the steps taken to measure these multimodal candidate signals.
Emotional Expression via Candidate Facial Displays
We build upon burgeoning scholarship that uses computational methods to study images as data (e.g., Cantú Reference Cantú2019; Casas and Williams Reference Casas and Williams2019; Torres and Cantú Reference Torres and Cantú2021) and to capture and analyze facial expressions of political actors (e.g., Boussalis and Coan Reference Boussalis and Coan2021; Joo, Bucy, and Seidel Reference Joo, Bucy and Seidel2019). While there is a strong interest in the nonverbal communication literature for increasingly granular measures of facial expressions, the field continues to be hampered by the methodological challenges involved with manually analyzing the content of images of faces at large scales—for instance, every frame of a set of hours-long debate videos. It takes an average of 10 minutes to apply the widely used Facial Action Coding System (Ekman and Friesen Reference Ekman and Friesen2003) to identify the emotional expression from a face in an image (Stewart, Salter, and Mehu Reference Stewart, Salter, Mehu, Bucy and Holbert2011). Given that our study seeks to classify candidate facial displays of emotion at each frame of five debates, the time and resource costs needed to manually approach this measurement task exceed prohibitive levels.
Fortunately, innovations from the fields of machine learning and computer vision allow us to extract these nonverbal signals using an efficient and reliable process. We first downloaded the debate videos and extracted their frames (n = 595,169). From these frame-level images, we relied on Microsoft Azure Cognitive Services’ Face API to identify the faces in each frame and to extract emotive display from each face. The Face API recognizes human faces and predicts the level of eight emotions (anger, happiness, contempt, disgust, fear, neutral, sadness, and surprise). While the underlying architecture is closed-source, this software relies on deep convolutional neural networks (Krizhevsky, Sutskever, and Hinton Reference Krizhevsky, Sutskever and Hinton2017; LeCun, Bengio, and Hinton Reference LeCun, Bengio and Hinton2015) trained largely on data annotated using the Ekman and Friesen (Reference Ekman and Friesen2003) model of discrete facial expressions (Bargal et al. Reference Bargal, Barsoum, Ferrer and Zhang2016). For each image, the service returns the identity of each face and a confidence score of the eight emotions mentioned above, ranging over the interval [0,1], with all emotion confidence scores for a given image summing to one.Footnote 6 We collapse the frame data to the second-by-second level for each debate to produce average per second facial emotion confidence scores.
Given the theoretical expectations outlined above, our analysis focuses on facial displays of either happiness or anger as well as the expression of any emotion. To validate these measures, we compare the manual coding of a large sample (N = 1,341) of five-second debate clips with our automated measures. The measures demonstrate relatively high correspondence between the model’s predictions and human annotations. We find a closer correspondence between the model predictions and human annotations for happiness than for either anger or any emotion; see Online Appendix Section C: Validating Displays of Emotion and Sentiment. We also evaluate the topics that the speakers reference when they express higher levels of emotion (see Figure A10 in the Online Appendix) as well as reading the debate transcripts at points of heightened emotions. For example, Merkel expresses high levels of happiness when she is talking about increasing employment, while Steinbrück expresses anger over foreign policy, particularly how the United States engaged in action on Syria.
Emotional Intensity via Candidate Vocal Pitch
We next capture the emotional content of a candidate’s vocal characteristics. Following the work of Dietrich, Hayes, and O’Brien (Reference Dietrich, Hayes and O’Brien2019), we operationalize emotional intensity by measuring the fundamental frequency (F0) of the voice of a candidate while speaking during a debate. We extracted the audio from the debate videos and then passed the files to the parselmouth library in Python (Jadoul, Thompson, and De Boer Reference Jadoul, Thompson and De Boer2018), which builds directly upon the source code of Praat (Boersma and Weenink Reference Boersma and Weenink2018). This program converted the debate audio to a Praat sound object that contains 100 “frames” per second, and each “frame” includes at least one “candidate” estimate of F0. We rely on the default Praat frequency settings of 75–600 Hz for candidate recruitment. The program employs a path-finding algorithm to select the best candidate estimate for each frame. These estimates were then used to calculate the average F0 for each second of a given debate. Our study, therefore, measures the average per-second fundamental frequency of the debate audio. This variable is then standardized within each debate for all debate participants.
Sentiment of Speech via Candidate Utterances
We measure statement-level sentiment with a dictionary approach by relying on the German translation of the Lexicoder Sentiment Dictionary, which has been validated extensively for political speech (Proksch et al. Reference Proksch, Lowe, Wäckerle and Soroka2019). The dictionary consists of 3,998 positive and 5,849 negative terms. We identified the words spoken by each politician and passed them through the sentiment dictionary. Following Proksch et al. (Reference Proksch, Lowe, Wäckerle and Soroka2019), we count the number of positive and negative words in each statement, and aggregate sentiment as the logged ratio of positive and negative terms.
Measuring Real-Time Reactions of Debate Audience Members
Our study relies on continuous response measures of debate audience members to observe how voters react to candidates’ visual, vocal, and verbal signals in real time. For the debate in 2005, we use real-time response (RTR) data from Nagel, Maurer, and Reinemann (Reference Nagel, Maurer and Reinemann2012),Footnote 7 and data from the 2009, 2013, and 2017 debates are included in the German Longitudinal Election Study (Rattinger et al. Reference Rattinger, Roßteutscher, Schmitt-Beck, Weßels, Brettschneider, Faas and Maier2011; Reference Rattinger, Roßteutscher, Schmitt-Beck, Weßels, Wolf, Brettschneider and Faas2014; Roßteutscher et al. Reference Roßteutscher, Schmitt-Beck, Schoen, Weßels, Wolf, Faas and Maier2019b). All respondents are eligible voters and were recruited by press releases, leaflets, and posters advertising participation in a study on media reception based on a quota plan drawn up in advance. An average of 90 respondents evaluated each debate (minimum of 46 [2017] to maximum of 154 [2009]); 32 respondents provide second-level RTR data for the debate between the minor parties in 2017 (Roßteutscher et al. Reference Roßteutscher, Schmitt-Beck, Schoen, Weßels, Wolf, Faas and Maier2019a).
To test our hypotheses on voter reactions to emotional displays, we construct a dataset of the real-time response measures at the individual respondent-second level. Therefore, the unit of analysis is the evaluation of candidates in a given second by a respondent. The scale of this measure ranges from 1 to 7. Participants were asked to move the dial to the left (values 1 to 3) if they had a good (bad) impression of the male competitor (Angela Merkel). The stronger this impression was, the further the knob should be turned. If a person had a good (bad) impression of the chancellor (male competitor), they were to move the dial to 5 to 7. The scale value 4 implies a neutral impression or that positive and negative impressions of both candidates canceled each other out. We inverted the values of the measure for observations where the challenger is speaking—that is, higher values indicate more agreement with the current speaker.
Methods
Candidate-Level Methods
In order to test our candidate-level hypotheses, we estimate six statistical models for each debate, where the unit of analysis is candidate-second. The models include the following dependent variables: average confidence scores of (1) happiness, (2) anger, and (3) non-neutral facial displays, as well as the (4) speech sentiment score and indicators of whether a candidate is speaking (5) 1 standard deviation or (6) 1.5 standard deviations above their average vocal pitch.Footnote 8 For Models 1–4 we rely on Prais–Winsten linear regression, and for Models 5 and 6 we use probit regression. The main explanatory variable is a binary variable for whether Angela Merkel (versus her male opponent) is being shown on screen. In all models we also control for the gendered topic by coding topics as feminine, masculine, neutral, and none.Footnote 9 All models also include utterance fixed effects.
Voter-Level Methods
To examine our voter-level hypotheses, we draw on our RTR data. Past scholarship highlights a number of challenges associated with determining a suitable estimation strategy for studies using RTR data (Schill, Kirk, and Jasperson Reference Schill, Kirk and Jasperson2016). One immediate challenge is that the relationship between candidate behavior (e.g., facial expressions, pitch, etc.) and participant response is inherently dynamic and the lag time between an expression and response is not known in advance. To estimate the influence of a candidate’s emotional expressions, we build on previous approaches (Boussalis and Coan Reference Boussalis and Coan2021). Based on information criteria, we determine that four seconds suitably captures the dynamics of our key facial, vocal, and verbal measures, consistent with past scholarship (Boussalis and Coan Reference Boussalis and Coan2021; Nagel, Maurer, and Reinemann Reference Nagel, Maurer and Reinemann2012). While it is standard practice to place constraints on the lag structure in autoregressive distributed lag models to avoid multicollinearity issues (particularly when using small to medium-sized datasets), we leverage a massive sample size to estimate the lag structure directly by including four lags of these key variables. In doing so, we offer a flexible parameterization of the salient dynamics, without making—perhaps inappropriate—assumptions on the underlying lag distribution.
We employ an ordinary least squares regression model to test the voter-level hypotheses, with the seven-point dial score as the dependent variable. The main explanatory variables are a binary variable of whether Angela Merkel (1) or her opponent (0) is the speaker and the standardized per-second average confidence scores of facial displays of emotion across four lags. These models also control for individual-level data on each respondent based on a survey conducted prior to each debate. These variables include respondent age, gender, party identification, self-reported political interest, and political knowledge.Footnote 10 We also control for whether the topic is masculine, feminine, neutral, or none. Standard errors are clustered at the participant level.
Given how individual responses are encoded in our data (i.e., higher values mean greater support for a candidate when they are speaking), we estimate a fully conditional model, interacting whether Merkel is the speaker with all covariates in the model. This approach allows us to estimate our main comparison of interest and ensure that key control variables have a substantively meaningful interpretation.
Results
This section begins by examining our candidate-level hypothesis and then moves to the voter level. As such, this section investigates not only how gender shapes the expression of emotions in debates but also the extent to which those expressions influence voter evaluations.
Candidate Gender and Emotional Expression
We first present descriptive measures to examine candidate nonverbal emotional expressions in the main debates. As shown in Figure 2, all candidates display a high level of happiness in the debates, with Merkel only expressing more happiness than her opponents in one year (2005). While anger is less common, all four men display more anger than Merkel, with values ranging between 0.005 and 0.03. The descriptive findings are consistent with our expectation that men will express more anger.

Figure 2. Average Confidence Scores for Emotional Displays
We test our expectations about the type of emotions (Candidate Hypothesis 1) in Figure 0, which presents the results by debate and includes a full set of controls. Individual descriptive statistics for each candidate are available in Figure A13. Models 1 and 2 examine our expectations regarding facial displays of specific emotions. Here we find mixed results. Merkel is less likely than her male counterparts to express anger in each of the four debates (1% error level), but we find limited evidence that Merkel expresses more happiness. While Merkel expresses more happiness in 2005, this relationship does not hold for subsequent debates.
Next, we turn to general emotional expressions by examining candidate differences for all non-neutral displays, sentiment, and higher-than-average emotional pitch. We expect that women will emote more than men, but find little support for this expectation. As shown in Models 3–6 in Figure 3, Merkel sometimes is more emotional than her counterparts—and sometimes less; this is true for facial emotions, vocal pitch, and sentiment. The overall findings suggest that there are no gender differences in the level of emotive expression.

Figure 3. Candidate-Level Emotions for Main Debates
Note: Prais–Winsten linear regression (Models 1–4) and probit regression (Models 5–6) results of per-second average confidence scores of happiness, anger, non-neutral facial displays, sentiment, and per-second candidate heightened vocal pitch (+1 and +1.5 SD above candidate mean). All models include utterance fixed effects and statement-level controls for masculine, feminine, and “none” debate topics, with neutral topics as the reference category. The x-axes are rescaled for each model to display estimates; see Tables A3–A6 for coefficients. Horizontal bars show 90% and 95% confidence intervals.
We examine the robustness of our findings by applying the same set of analyses to the 2017 debate of minor parties, except that here the main explanatory variable is a binary measure of whether the speaker is female. The results are strikingly similar to those of the debates with Angela Merkel. The female candidates display less anger (5% error level), but they do not display more happiness or general emotional intensity (see Figure 4). The one difference is that women in the minor party debate are more likely to elevate their vocal pitch at our lower threshold (1% error level).

Figure 4. Candidate-Level Results for the 2017 Minor Party Debate
Note: Prais–Winsten linear regression (Models 1–4) and probit regression (Models 5–6). All models include utterance fixed effects and statement-level controls for gendered topics. The x-axes are rescaled for each model. Coefficients are displayed in Table A11. Horizontal bars show 90% and 95% confidence intervals.
Voter Responses to Candidate Emotions
Do these emotional expressions matter for how voters perceive the candidates? To assess our expectations, we turn to the real-time response data. To refresh, our dependent variable is the reaction (on a seven-point scale) to the candidate that is shown speaking on the screen. We estimate a separate model for each debate. We control for the topic of the debate and for respondent gender, political knowledge, and political party affiliation. Given that we are principally interested in the difference in reactions to Merkel’s emotions as compared with her opponent’s emotions, we present the effect of a one-standard-deviation increase in the nonverbal display of the emotion between Merkel and her opponent.
The evidence supports our first expectation for voter reactions: voters reward Merkel’s expression of happiness and punish her facial displays of anger. Starting in panel (a) of Figure 5, Merkel’s expression of happiness is rewarded by voters with positive and significant effects in the 2009, 2013, and 2017 debates. In comparison, we see negative coefficients for her anger in two of the four debates.

Figure 5. Voter Reactions to Candidate Emotions, Main Debates
Note: Panel (a) includes reactions to happiness and anger; panel (b) displays reactions to non-neutral facial emotional expression. Estimates of the cumulative effect (across four lags) of the key textual, vocal, and facial variables of interest (see Tables A7 and A8 for full results). All models include control variables for the gender, age, party identification, political knowledge, and political interest of respondents. The horizontal bars show 90% and 95% confidence intervals.
To examine how voters react to the overall level of emotional expression, we next turn to panel (b) of Figure 5, which presents voter reactions to non-neutral facial displays, vocal pitch, and text sentiment. Across our three measures of emotional intensity, voters generally reward Merkel for her emotional expression, which is consistent with Voter Hypothesis 2. The only exception is non-neutral displays for the 2005 debate, when she was a challenger and was the most expressive out of all four debates. In short, while voters respond negatively to Merkel’s expression of anger (an emotion incongruent with her gender), they reward her happiness and her general emotional expression. The opposite is true for her male opponents, whose anger is rewarded and happiness is punished by voters.
Are these reactions due to reactions to Merkel’s emotions, the emotions of her opponents, or both? To answer this question, we split the models to provide separate assessments of Merkel and her opponents. We again find results (provided in Figure 6) consistent with our expectations. Voters positively evaluate Merkel when she displays happiness and evaluate her negatively when she displays anger, with the exception of 2017. The reverse is generally true for her male counterparts: voters tend to not reward (and sometimes even punish) her opponents for happiness, but reward them for anger, with the exception of 2005. Voters also tend to react positively to Merkel’s general level of emotional expression, as measured through non-neutral facial displays and vocal pitch.

Figure 6. Voter Reactions to Facial Emotions, Sentiment, and Vocal Pitch
Note: Separate models of reactions to Merkel (left-hand panel) and male competitor (right-hand panel). See Tables A9 and A10 for full results.
Across the various specifications presented in Figure 5 and Figure 6, the substantive effects of these coefficients (ranging from 0.05 to 0.2 for a one-standard-deviation increase of the independent variables) translate into real change in evaluations. Although the dials range from 1 to 7, the average and median standard deviation on the level of respondents only amount to around 1. Further, consider that the average and median audience member only moves the RTR dial 2–3 times per minute when candidates are speaking (see Figure A12). Characteristics of the audience itself suggest that one should expect small changes—participants in these studies are more interested in politics and tend to have stable attitudes of the candidates participating in the debates (Maier and Faas Reference Maier and Faas2019, 22). These debates constitute a challenging environment for detecting any changes in candidate evaluations. As a result, that emotions prompt any movement at all—and particularly consistent results across debates and change to the size of 0.2—is substantively meaningful.
It remains possible that voters are simply reacting to Merkel’s unique political style and not her gender. To assess the robustness of our results, we turn back to the 2017 minor party debate. Instead of turning a dial “for” or “against” a particular candidate, voters indicated whether they have a bad impression (lower values) or good impression (higher values) on a 1–7 scale of whichever candidate was speaking. Only 36 eligible voters—a considerably smaller sample of voters than in debates involving Merkel—provided real-time responses during the debate between minor party leaders. Given that women represented both the far-left (Wagenknecht, The Left) and the far-right (Weidel, AfD) parties, the results for candidate gender should not be confounded by ideological positions of parties or candidates in this debate.
While these data need be interpreted with care, given the small number of respondents who watched the minor debate, we again find that voters react negatively to women’s expression of anger and positive sentiment (Figure 7). Unlike in the Merkel debates, however, voters do not reward these women for happiness or the level of their emotion (measured through non-neutral facial expressions, sentiment, or vocal pitch).

Figure 7. Voter Reactions to Candidate Emotions, Minor Parties Debate
Note: Estimate of the cumulative effect (across four lags) of the key facial, sentiment, and vocal pitch variables of interest. See Figure A16 for non-neutral emotions and Table A12 for full results. 90% and 95% confidence intervals.
Algorithmic (Gender) Biases and Measuring Candidate Emotion
The measures of emotion used in our analyses all have the potential to be biased in their evaluations of the behavior of men and women. As machine learning systems get closer to replicating human behavior, they also replicate human biases (Schwemmer et al. Reference Schwemmer, Knight, Bello-Pardo, Oklobdzija, Schoonvelde and Lockhart2020). We recognize that these biases may have important theoretical and practical implications for our research. To evaluate the role of gender biases in our research, we engage in a wide range of analyses.
Facial displays of emotion: Emotion-detection APIs have a number of biases (including gender and racial biases) encoded in to their processes (Buolamwini and Gebru Reference Buolamwini and Gebru2018). For example, Schwemmer et al. (Reference Schwemmer, Knight, Bello-Pardo, Oklobdzija, Schoonvelde and Lockhart2020) find that classifiers are much more likely to assign terms associated with physical appearance to images of female (versus male) members of Congress. There are also gender biases in the classification of specific emotions: a neutral face, happiness, and anger tend to produce the lowest levels of gender bias (Khanal et al. Reference Khanal, Barroso, Lopes, Sampaio and Filipe2018). And while anger generally has higher error rates when compared with happiness, it is more extensively validated than emotions such as disgust and surprise.
We evaluate potential gender biases in our API-based predictions of emotions in facial displays through two separate samples of human annotations (see Online Appendix Section C for details). We start with two trained annotators (who are both women) who coded a large sample (N = 1,341) of five-second debate clips. We compare the RMSE of the model predictions across the gender of the candidate (i.e., Merkel versus her opponents). We find similar levels of performance across candidate gender for any emotion, anger, and happiness, while further confirming that the API performs better for happiness than anger irrespective of the candidate’s gender (Boussalis and Coan Reference Boussalis and Coan2021).
Next, we extend our analysis by using a sample of crowd-sourced annotations to examine whether (a) the annotator’s gender predicts model performance and (b) the interaction between candidate and annotator gender shapes performance. We collected a sample of 467 respondents (54% female and 46% male) and asked each individual to code a sample of 50 debate clips. Out-of-sample performance for each respondent was once again assessed using the RMSE, and we use linear regression to examine the influence of candidate and annotator gender on estimated model performance. We find lower RMSE estimates—and therefore better performance—for female annotators, and these findings hold for each emotion considered in this study. When considering candidate gender, the difference in performance between Merkel and her opponents is insignificant for anger and any emotion. However, the crowd RMSE is better when assessing happiness for Merkel. Last, we do not find evidence for an interactive effect between the respondent and the candidate gender in predicting out-of-sample performance.
Vocal pitch: We next consider several ways that gender might shape the measurement of emotion via vocal pitch. Women have naturally higher vocal pitches, which could shape the assessment of emotions in pitch (Klofstad, Anderson, and Nowicki Reference Klofstad, Anderson and Nowicki2015). Research suggests that gender differences in vocal pitch are an interval shift—women’s vocal pitch has a higher base rate, but vocal pitch follows similar patterns when emotional intensity increases for both men and women (Giannakopoulos and Pikrakis Reference Giannakopoulos and Pikrakis2014).Footnote 11 The gender of the listener can also matter: women more accurately detect emotions from vocal pitch (Lausen and Schacht Reference Lausen and Schacht2018). We estimate separate models for the men and women in the audience in our samples (see Figure A14). These results are consistent with the scholarship. Women in the sample react more to differences in vocal pitch, but both men and women react in similar directions. Still, we recognize that this is but one (narrow) way of measuring gender biases in vocal pitch.
Sentiment analysis: Gender differences appear in the use of language, including the sentiment of text spoken or written by men and women. These differences then are replicated in sentiment analyses, where men’s language is often coded as more negative or more masculine (Roberts and Utych Reference Roberts and Utych2020). Our sentiment measure thus could produce biased results where women’s speech is measured as more positive. To evaluate this possibility, we replicate our findings with the Rauh sentiment dictionary, which is validated against German political speech (Rauh Reference Rauh2018). We find (a) these dictionaries produce correlated scores in our data, (b) the correlation does not vary systematically in one way or another for men or women, and (c) our full models replicate with this alternative dictionary (Figure A7 and Figure A15). We examine the relationship between our sentiment analysis and hand coding (of the “social situation” as positive, negative, or neutral) from a content analysis of the debates. Positive sentiment scores correspond with positive coding (Figure A8). We then draw from Roberts and Utych’s (Reference Roberts and Utych2020) dictionary of words coded as masculine or feminine to estimate whether the masculinity of text might drive differences in our sentiment analysis. Neither Merkel nor the women in the 2017 debate between minor parties spoke with more feminine language (Table A1).
Together, we undertake these validation exercises not to indicate the absence of gender biases in our measures. Rather, we show how the gender biases in our measures are distributed in a somewhat random fashion (akin to measurement error) and should not systematically bias our results in a single direction. We can be more confident in our results precisely because the setting is held relatively constant across all our data, we are dealing with a small number of candidates, and, importantly, all the candidates in our evaluations are white. Research on emotions detection, for example, shows consistent biases in the ability to accurately detect emotions in faces of darker skinned individuals (Buolamwini and Gebru Reference Buolamwini and Gebru2018). We also do not know how facial features of candidates—for example, if candidates varied in attractiveness or babyfacedness or their masculine features (Carpinella and Bauer Reference Carpinella and Bauer2019; Zebrowitz and Montepare Reference Zebrowitz and Montepare2005)—might bias the detection of emotion. These biases limit our ability to ask important questions about emotions in politics. We urge scholars using machine learning to evaluate emotions to employ these—or many other—validation exercises.
Discussion
Despite the importance of political debates and nonverbal cues to electoral outcomes and voter behavior, candidate emotions during debates have received little attention from political scientists. Some of this is due to the methodologically taxing process of manually coding debate images. As a result, the scholarship has often, understandably, relied on snippets of debates, on the text of the debate, or on candidate rhetoric. We are the first, we believe, to employ multiple methods of emotion detection to examine both candidate behavior and voter reactions in multiple entire debates and to apply a gendered emotions frame to understanding political debates. This departure allows for different theoretical and empirical tests than available in prior work.
We argue that combining video, audio, and text data from televised debates allows one to gain a more complete understanding of candidate behavior and voter decision making. Candidates are fundamentally interested in presenting their best self to the public (Bystrom et al. Reference Bystrom, Robertson, Banwart and Kaid2005; Dittmar Reference Dittmar2015). By capturing not just what candidates say, but how they say it and what they look like when they say it, we offer a far more comprehensive evaluation of candidate self-presentation than previously available to scholars. Moreover, the ability to leverage continuous responses from voters in a live audience offers an additional advantage for understanding political behavior. The integration of real-time responses with nonverbal cues from candidates is thus a major methodological improvement on understanding how voters perceive politicians in modern political debates.
Drawing on work from psychology, communications, and gender studies, we bring a robust evaluation of candidate gender into dialogue with scholarship on political debates and nonverbal communication. Relying on theories of role congruity and, particularly, gender role congruity, we argue that candidates express nonverbal cues strategically and that voters respond to these cues. Critically, however, not all male and female candidates are equally able to express these emotions because voters assess nonverbal behavior by whether it meets gendered expectations.
After validating our measures of facial, vocal, and verbal emotional expressions, we classified candidate facial expressions in over 590,000 frames from German televised debates. Consistent with our expectations, we find that Merkel is less likely to express anger than her male opponents. We do not find, however, that she expresses more happiness or is more emotive generally. This may be because men recognize the value in happiness and emotion to attract supporters via these leadership debates, which serve as a key event in the German elections. Examining the debate for minor parties confirms these same patterns: the women participating expressed less anger but similar levels of happiness and overall levels of emotion.
Examining millions of real-time responses from voters reveals that Merkel expresses happiness much more frequently than anger, and voters reward Merkel for her presentation of happiness. Indeed, voters reward Merkel generally for her emotional expressions, compared with her male colleagues who are often punished for their non-neutral displays.
These analyses are just a small piece of what could be learned from nonverbal behavior, particularly in an environment where emotional displays can be obtained at scale through computational methods. Understanding, for example, how voters react when verbal sentiment and nonverbal emotions align or conflict could provide a key to understanding the full context by which voters interpret candidate speech and images during debates. We move beyond a single measure and evaluate multimodal expression concurrently. Subsequent research could engage in even broader evaluations of how candidates temper or emphasize emotions through a combination of face, voice, and sentiment—and how voters respond.
We operationalize vocal emotional intensity in this paper as the fundamental frequency of a voice, which is a common approach to measuring voice pitch. However, pitch is but one potential means of capturing voice affect. For example, scholars have also combined other vocal dimensions such as duration, intensity, tune, and magnitude to infer emotion from voice (e.g., Goudbeek and Scherer Reference Goudbeek and Scherer2010). We hope future iterations of work on the role of emotions in political debates will expand our evaluations of both how candidates use their voices to express distinct emotions and how voters react to these emotional expressions. In doing so, researchers should pay careful attention to the gendered nature of emotions and potential gender biases in these measures.
Our results demonstrate the importance of considering the ways that candidates constrain themselves to fit what they think voters want. Angela Merkel, like other women seeking positions of power, is well aware that her gender shapes how voters react to her. That Merkel—and women in the minor party debate—expresses little anger during these debates suggest that she adjusts her behavior to better fit voter expectations. Yet, adjusting the behavior may also constrain women’s ability to lead in different contexts. Research might examine whether this means that women are less likely to be selected for positions of leadership during times of foreign-policy crisis, when voters might want an “angry” leader who will defend them. Future studies might also consider the ways that powerful women express anger in alternate ways—by expressing surprise or disgust, for example. Our research also speaks to the experiences and judgement of women outside politics. We would expect that women’s anger would be constrained in business and philanthropy settings, just as in politics.
Supplementary Materials
To view supplementary material for this article, please visit http://dx.doi.org/10.1017/S0003055421000666.
Data Availability Statement
Data and code to replicate the results in this paper are posted at the American Political Science Review Dataverse: https://doi.org/10.7910/DVN/NVVVUV.
Acknowledgments
Thanks to Molly McClure, Caitlin Sharma, Helen Retzlaff, and Natalia Umansky for excellent research assistance. We are grateful to Bethany Albertson, Amanda Kass, Lindsey Meeks, Kirsten Rodine-Hardy, and Christina Xydias, and participants at the 2020 European Political Science Association annual conference, the University College London Political Science Departmental Research Seminar, the Digital Democracy Workshop at the University of Zurich, the IMG-DUB workshop in Dublin, the Behavioural Science and Policy seminar at University College Dublin, and the Hot Politics Lab meeting at the University of Amsterdam for their feedback on the project. We also thank Friederike Nagel, Marcus Maurer, and Carsten Reinemann for sharing the content analysis, surveys, and RTR data from the 2005 debate and the team of the German Longitudinal Election Study for making the data for the debates in 2009, 2013, and 2017 publicly available.
Funding Statement
This research was funded through generous support from the Trinity College Dublin Arts and Social Sciences Benefactions Fund 2019–20, from the University College Dublin Ad Astra Start Up Grant, and from the Dunbar Fund, Political Science, Tulane University.
CONFLICT OF INTEREST
The authors declare no ethical issues or conflicts of interests in this research.
ETHICAL STANDARDS
The authors declare the human subjects research in this article was reviewed and approved by Tulane University Institutional Review Board, and certificate numbers are provided in the appendix.
Comments
No Comments have been published for this article.