Nomenclature
- RESO
-
reduced-order extended state observer
- CNFTSMC
-
composite non-singular fast terminal sliding mode controller
- NFTSMC
-
non-singular fast terminal sliding mode controller
1.0 Introduction
As scientific and technological advances rapidly progress, the remarkable applicability of near-space vehicles is increasingly prominent. Among these, stratospheric airship, characterised by low energy consumption, high cost-effectiveness, prolonged flight duration and substantial payload capacity, is extensively utilised in a range of applications such as regional warning, environmental monitoring, communication relay, regional navigation and emergency response [Reference Yao1]. Stratospheric airship adjusts the attitude to track specific trajectories and execute flight missions, thus high-precision and robust finite-time attitude tracking control is pivotal in the design of stratospheric airship flight control system [Reference Zhao, Li, Jiang and Cao2, Reference Sun, Sun, Guo, Yuan and Zhu3]. However, the attitude dynamic model of stratospheric airship exhibits high nonlinearity and strong channel coupling [Reference Yuan, Guo, Zheng, Zhu and Gou4], posing significant challenges to control system design. In addition, model parameter perturbations and complex flight environment [Reference Wu, Wang, Duan, Xie and Wei5, Reference Habibi, Safaei, Voos, Darouach and Sanchez-Lopez6] considerably impact the attitude tracking accuracy of stratospheric airship.
In addressing the challenges encountered in airship attitude control, researchers have undertaken various studies. Reference (Reference Wu, Lin and Zhang7) designed the attitude control system of the airship based on fault-tolerant backstepping control law, facilitating the tracking of desired attitude with robustness to step bias and time-varying bias. Reference (Reference Wang and Li8) developed a full-state feedback controller for airship based on the linear quadratic gaussian/loop transfer recovery algorithm to control the horizontal attitude angle of the airship under wind disturbances. Reference (Reference Le, Wang, Duan and Wu9) devised a self-disturbance attitude tracking controller for the pitch angle and yaw angle of the airship affected by wind field disturbances.
Sliding mode control has prominent advantages such as a simple structure and robustness [Reference Ding, Levant and Li10, Reference Shao, Sun, Xue and Li11], and has been extensively utilised in the design of aircraft control system. In Ref. [Reference Yang, Wu and Zheng12], a terminal sliding mode control method was employed to design an attitude tracking control law, achieving convergence of the attitude tracking error to zero within a finite time. However, the introduced external disturbance torque was deemed insufficient to demonstrate the disturbance rejection capability of the proposed control algorithm. During the modeling process, multiple disturbances such as parameter perturbations and external disturbances caused by complex environment were identified, making it difficult to achieve rapid disturbances suppression relying on the robustness of the control algorithm itself. By designing an extended state observer [Reference Razmjooei, Palli and Abdi13], the total disturbances of the system were regarded as the new state variable, with the disturbances estimation values incorporated into the controller design in the form of feedforward [Reference Wang, Liu, Zhao, Ding and Xu14] to directly suppress the effects of disturbances. Reference (Reference Chen15) employed a traditional nonlinear extended state observer to estimate the total disturbances, with the resulting disturbances estimation information integrated into the design of the terminal sliding mode controller to mitigate the influence of parameter perturbations and external disturbances, thus achieving the desired attitude tracking of the airship. However, in the simulation experiments conducted in Ref. [Reference Chen15], the chosen model mass and moment of inertia of the airship were too large, resulting in limited changes in attitude angles and requiring a substantial control torque, which may exceed the upper limit of the control torque provided by the actual actuator. Taking into account the limitation of the control torque, it is vital to meticulously design and select parameters for the control system to ensure its alignment with the control requirements of the actual actuator. It is worth noting that the traditional nonlinear extended state observer is susceptible to causing phase lag in the system, and the magnitude of the phase lag amplifies with the increasing order of the nonlinear extended state observer. One effective method to address this issue is to reduce the order of the nonlinear extended state observer, namely using a reduced-order extended state observer [Reference Fu and Tan16].
This paper addresses the attitude tracking problem of stratospheric airship under the influence of external disturbances and parameter perturbations. It proposes a composite non-singular fast terminal sliding mode attitude control scheme based on a reduced-order extended state observer. Firstly, the mathematical model of the airship’s attitude motion is established, and the input-output feedback linearisation is utilised to decompose the nonlinear attitude motion system into three-channel subsystems. Subsequently, a reduced-order extended state observer is designed to estimate and compensate for the total disturbances of the system. Based on the disturbances estimation information and the composite non-singular fast terminal sliding mode control algorithm, a composite non-singular fast terminal sliding mode controller based on the reduced-order extended state observer is designed. Finally, the effectiveness of the proposed method is verified through simulation.
This paper makes the following contributions:
-
(1) In comparison to the work presented in Ref. [Reference Chen15], this paper introduces a reduced-order extended state observer that effectively mitigates the phase lag caused by the observer. By estimating real-time system disturbances with smaller gains and a simplified structure, the proposed observer significantly enhances the robustness of the attitude system against multiple disturbances.
-
(2) In the design of the control scheme, this paper proposes a novel composite sliding surface based on the tracking error performance function. Unlike the integral sliding mode employed in Ref. [Reference Wang, Zhou, Chen and Duan17], the proposed composite sliding surface provides superior sliding trajectory for the state variable, resulting in faster and more accurate tracking of the desired attitude angles.
-
(3) Diverging from existing findings in Ref. [Reference Yang18], this paper introduces the hyperbolic sine function as a replacement for the sign function in the control law design, eliminating the chattering phenomenon of sliding mode while ensuring the continuity of control signals.
These contributions substantiate the effectiveness and superiority of the adopted composite nonsingular fast terminal sliding mode attitude control scheme in this study. The proposed method enables rapid and accurate tracking control of stratospheric airship attitude in complex environment with multiple disturbances, thus contributing to the advancement of practical applications for stratospheric airship.
2.0 Preliminaries and problem statement
2.1 Preliminaries
Consider the following system [Reference Yang, Su and Zhang19]:

where
$\lambda = {\left[ {\begin{array}{l}{{\lambda _1},{\lambda _2}, \ldots ,{\lambda _n}}\end{array}} \right]^{\rm{T}}}$
,
$f$
(
$ \cdot )$
is a continuous nonlinear function, assuming the origin is an equilibrium point for system (1).
Lemma 1.
If there exists a Lyapunov function V, such that
$\dot V \le - {\mu _1}V - {\mu _2}{V^{{n_1}}}$
,
${\mu _1} \gt 0$
,
${\mu _2} \gt 0$
and
$0 \lt {n_1} \lt 1$
, then the system (1) is finite-time stable at the origin, and V converges to 0 within a finite time [Reference Mu, Li, Wang, Fan, Zhao and Sun20].

Lemma 2.
If there exists a Lyapunov function V, such that
$\dot V \le - {\mu _1}{V^{{n_1}}}$
,
${\mu _1} \gt 0$
and
$0 \lt {n_1} \lt 1$
, then the system (1) is finite-time stable at the origin, and V converges to 0 within a finite time [Reference Wang, Deng, Xie and Pan21].

2.2 Problem statement
According to Ref. [Reference Ou22], the six degrees of freedom kinematic equation for an airship is given by
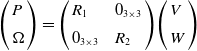
where
$P = \left[ x,y,z\right]^{T},{\rm{\Omega }} = \left[\theta ,\psi ,\phi \right]^{T},V = \left[ u,v,w\right]^{T},W = \left[p,q,r\right]^{T},$
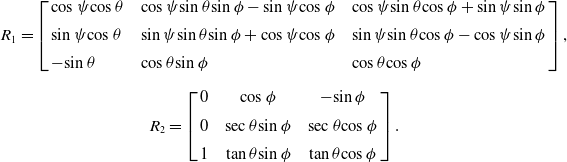
$x$
,
$y$
, and
$z$
are the airship’s displacements along the longitudinal, lateral, and vertical axes;
$\theta ,\psi ,{\rm{and\;}}\phi $
are the airship’s pitch, yaw and roll angles;
$u$
,
$v$
and
$w$
are the velocity vector components along each axis;
$p$
,
$q$
and
$r$
are the airship’s roll, pitch, and yaw angular velocities, respectively.
Assuming the airship is a rigid body and neglecting its elastic deformation, the following ground-coordinate airship dynamic equation can be derived based on the Newton-Euler equation:


Upon substitution of Equations (6) into (5), we get

where
$m$
is the airship’s mass,
${\nu _G}$
is the velocity vector of the centre of gravity,
${\nu _o}$
is the velocity vector of the buoyancy centre,
$\omega $
is the angular velocity vector,
${r_G}$
is the vector from the buoyancy centre to the centre of gravity, and
$F$
is the force acting on the airship.


Upon substitution of Equations (9) into (8), we obtain

where
$H$
is the moment of force relative to the buoyancy centre,
${I_o}$
is the moment of inertia matrix relative to the buoyancy centre, and
$\tau $
is the moment of force acting on the airship.
By the application of the vector derivative rule, the airship dynamic equation in the body coordinate system can be derived.
Based on Equation (7), we derive

where
${\omega ^ \times }$
is the cross product matrix of the angular velocity vector
$\omega $
.
From Equation (10), we have

where
$r_G^ \times $
is the cross product matrix of the angular velocity vector
${r_G}$
.
Combining Equations (11) and (12), we derive the matrix form of the dynamic equation

If we account for the impact of additional mass and additional inertial force, the airship’s dynamic equation can be formulated as

where
${M_a}$
and
${I_a}$
are the additional mass matrix and additional moment of inertia matrix, respectively.
The attitude motion of the stratospheric airship in the geocentric reference frame (
${O_b}{x_b}{y_b}{z_b}$
) and the body reference frame (
${O_e}{x_e}{y_e}{z_e}$
) is shown in Fig. 1.
${C_V}$
is the buoyancy centre of the airship, while
${C_G}$
is the centre of gravity. The vector from the buoyancy centre to the centre of gravity is denoted as
${r_G} = {\left[ {\begin{array}{l}{{x_G},{y_G},{z_G}}\end{array}} \right]^{\rm{T}}}$
.

Figure 1. Attitude motion diagram.
From Equation (4) for the airship’s kinematic Equation and Equation (14) for the airship’s dynamic equation, we can obtain the mathematical model of the airship’s attitude motion
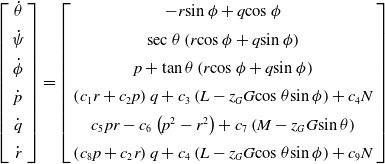
${\rm{where\;}}{c_1} = \frac{{I_{xz}^2 - {I_z}\left( {{I_y} - {I_z}} \right)}}{{I_{xz}^2 - {I_x}{I_z}}}$
;
${c_2} = - \frac{{\left( {{I_x} - {I_y} + {I_z}} \right){I_{xz}}}}{{I_{xz}^2 - {I_x}{I_z}}}$
;
${c_3} = - \frac{{{I_z}}}{{I_{xz}^2 - {I_x}{I_z}}}$
;
${c_4} = - \frac{{{I_{xz}}}}{{I_{xz}^2 - {I_x}{I_z}}}$
;
${c_5} = \frac{{{I_z} - {I_x}}}{{{I_y}}}$
;
${c_6} = \frac{{{I_{xz}}}}{{{I_y}}}$
;
${c_7} = \frac{1}{{{I_y}}}$
;
${c_8} = \frac{{{I_x}\left( {{I_y} - {I_x}} \right) - I_{xz}^2}}{{I_{xz}^2 - {I_x}{I_z}}}$
;
${c_9} = - \frac{{{I_x}}}{{I_{xz}^2 - {I_x}{I_z}}}$
;
$G$
is the gravity of the airship;
$L$
,
$M$
,
$N$
are the roll, pitch and yaw moments applied to the airship, respectively;
${I_x}$
,
${I_y}$
,
${I_z}$
, and
${I_{xz}}$
are the generalised moments of inertia.
During actual flight, the airship may be affected by external disturbances, and there may be some deviations in the model parameters. Let
${I_{x1}} = {I_x} + {\rm{\Delta }}{I_x}$
,
${I_{y1}} = {I_y} + {\rm{\Delta }}{I_y}$
,
${I_{z1}} = {I_z} + {\rm{\Delta }}{I_z}$
, and
${I_{xz1}} = {I_{xz}} + {\rm{\Delta }}{I_{xz}}$
. Where
${\rm{\Delta }}{I_x}$
,
${\rm{\Delta }}{I_y}$
,
${\rm{\Delta }}{I_z}$
, and
${\rm{\Delta }}{I_{xz}}$
are the uncertain parts of the moments of inertia.
By selecting the state variable
$x = {[\theta ,\psi ,\phi ,p,q,r]^T}$
, control variable
$u = {[ - {I_z}L - {I_{xz}}N,M, - {I_{xz}}L - {I_x}N]^T}$
, output variable
$h\left( x \right) = {[\theta ,\psi ,\phi ]^T}$
and external disturbance torque
$d = {[{d_1},{d_2},{d_3}]^T}$
, the mathematical model of the airship attitude motion system can be described as the following nonlinear system

where


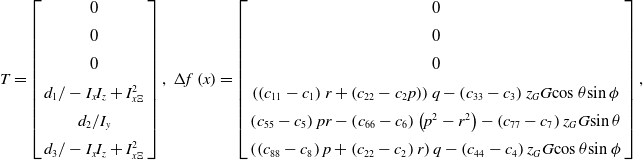
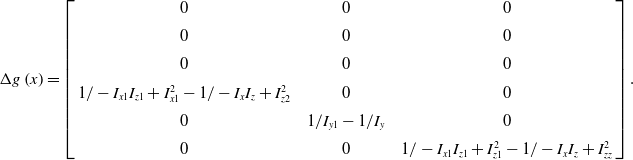
${\rm{where\;}}{c_{11}} = \frac{{I_{xz1}^2 - {I_{z1}}\left( {{I_{y1}} - {I_{z1}}} \right)}}{{I_{xz1}^2 - {I_{x1}}{I_{z1}}}}$
;
${c_{22}} = - \frac{{\left( {{I_{x1}} - {I_{y1}} + {I_{z1}}} \right){I_{xz1}}}}{{I_{xz1}^2 - {I_{x1}}{I_{z1}}}}$
;
${c_{33}} = - \frac{{{I_{z1}}}}{{I_{xz1}^2 - {I_{x1}}{I_{z1}}}}$
;
${c_4} = - \frac{{{I_{xz1}}}}{{I_{xz1}^2 - {I_x}1{I_z}1}}$
;
${c_5} = \frac{{{I_{z1}} - {I_{x1}}}}{{{I_{y1}}}}$
;
${c_6} = \frac{{{I_{xz1}}}}{{{I_{y1}}}}$
;
${c_7} = \frac{1}{{{I_y}1}}$
;
${c_8} = \frac{{{I_{x1}}\left( {{I_{y1}} - {I_{x1}}} \right) - I_{xz1}^2}}{{I_{xz1}^2 - {I_{x1}}{I_{z1}}}}$
.
According to Ref. [Reference Wang, Zhou, Chen and Duan17], the nonlinear attitude system can be transformed into the following system through coordinate transformation and state feedback
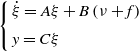
where
$v = {[{v_1},{v_2},{v_3}]^T}$
represents the pseudo-control variable;
$f = {[{f_1},{f_2},{f_3}]^T}$
accounts for system parameter perturbations and external disturbances;
$y$
denotes the system output;
$\xi $
,
$A$
,
$B$
and
$C$
are given by


Remark 1. The pseudo control signal is the output of the subsequent controller in the following section. The correspondence between the pseudo control signal and the actual control signal can be expressed through feedback, represented by
$u = {E^{ - 1}}\left( x \right)\left( { - Q\left( x \right) + \nu } \right)$
, where
$E\left( x \right)$
and
$Q\left( x \right)$
are presented in Ref. [Reference Wang, Zhou, Chen and Duan17]. By selecting an appropriate pseudo control signal and integrating it with feedback, finite time [Reference Ghadiri, Emami and Khodadadi23] tracking control of the system output variable can be achieved.
Remark 2. In the course of airship flight, it is exposed to external disturbances such as wind fields, which can deteriorate the precision of the controller. Owing to current technological constraints, obtaining real-time measurements of wind disturbances is challenging. Additionally, the substantial size of the airship makes it arduous to achieve precise measurement of the model parameters. In the subsequent section, an observer will be designed to estimate
$f$
, without relying on additional sensors.
3.0 Controller design
In this section, we will initially formulate the Reduced-order Extended State Observer (RESO) for each of the three channels to accomplish real-time estimation and compensation of multiple disturbances. Subsequently, leveraging the estimation information, we will proceed to devise the Composite Non-singular Fast Terminal Sliding Mode Controller (CNFTSMC) employing the RESO for each channel, with the objective of attaining high-precision tracking of the airship’s attitude in a multi-disturbance environment. The structure diagram of the attitude control system is illustrated in Fig. 2.
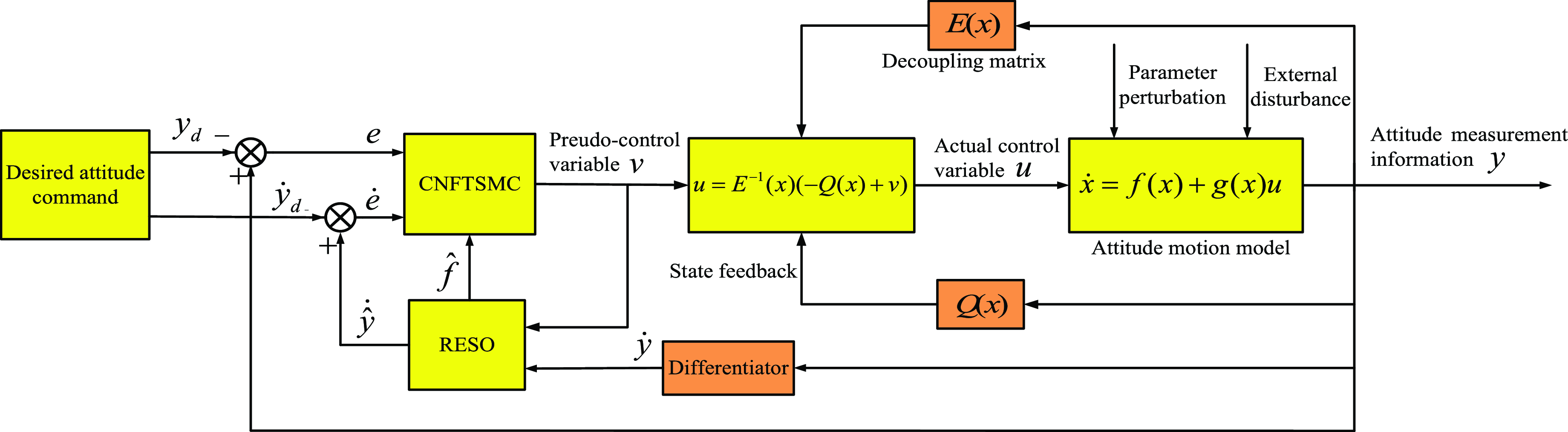
Figure 2. Structure diagram of the attitude control system.
3.1 Design of the RESO
The design of the RESO is exemplified using the pitch angle channel as an example, with similar design method applied to the other channels. Based on Equation (16), the state space representation of the pitch angle channel can be formulated as

To accurately estimate the combined effect of parameter perturbations and external disturbances [Reference Zhai, Li, Xu, Zhang and Li24], define
${\xi _7} = {f_1}$
, and extend the state variables as
${[{\xi _1},{\xi _2},{\xi _7}]^T}$
. Equation (17) can be rewritten as
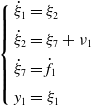
In Equation (18), the formulation of the traditional nonlinear extended state observer (ESO) is as follows:
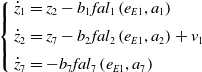
where
${z_1}$
represents the estimated value of the output
${y_1}$
,
${z_2}$
denotes the estimated value of the output derivative
${\dot y_1}$
,
${z_7}$
signifies the estimated value of
${f_1}$
, and
${e_{{{\rm{\;}}_{E1}}}}$
pertains to the estimation error of the pitch angle. So,
${z_1} = {\hat y_1}$
,
${z_2} = {\hat{\hat{y}}_1}$
,
${z_7} = {\hat f_1}$
,
${e_{{{\rm{\;}}_{E1}}}} = {\hat y_1} - {y_1}$
. The expression of
$fa{l_i}\left( {{e_{E1}},{a_i}} \right)$
is as follows [Reference Wang, Yao and Qu25]

where
$0 \lt {a_7} \lt {a_2} \lt {a_1} \le 1$
,
$0 \lt \delta \lt 1$
.
To enhance the accuracy of estimation, the ESO typically applies a larger gain for the observer. Nonetheless, augmenting the gain amplifies the noise, consequently undermining the controller’s performance. Due to the measurability of the state variables in the attitude system,
${e_{{E_2}}} = {\dot{\hat{y}}_1} - {\dot{y}_1}$
is utilised in place of
${e_{E1}}$
to mitigate the gain and suppress the noise. The design of the RESO is as follows:
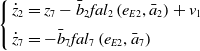
To rectify the issue of poor control accuracy when the error is large, an enhancement is implemented:

In contrast to the ESO, the RESO eliminates the need for secondary estimation of known output variable, diminishes the phase lag attributed to the observer and enhances the accuracy of disturbance estimation. Moreover, the proposed observer is characterised by a simple structure, capable of achieving commendable estimation performance with a smaller gain, and effectively reduces the engineering application cost.
3.2 Design of the CNFTSMC
Using the pitch angle channel as an illustration to design the CNFTSMC. The design method for the remaining channels is similar. Let
${y_{1d}}$
present the desired output,
${y_1}$
denote the actual output, and define the system error [Reference Jahanshahi, Yao, Khan and Moroz26] and its derivative as follows [Reference Chen, Yang and Fu27]:



Specify the composite sliding surfaces as follows:


where
$si{g^{\frac{{{p_1}}}{{{q_1}}}}}\left( {{e_1}} \right) = {\rm{sgn}}\left( {{e_1}} \right)|{e_1}{|^{\frac{{{p_1}}}{{{q_1}}}}}$
,
${\alpha _1} \gt 0$
,
${\beta _1} \gt 0$
,
${\alpha _2} \gt 0$
,
${\beta _2} \gt 0$
,
${w_1} \gt 1$
,
${p_1}$
,
${q_1}$
,
${m_1}$
, and
${n_1}$
are odd numbers, and satisfy
$1 \lt \frac{{{m_1}}}{{{n_1}}} \lt \frac{{{p_1}}}{{{q_1}}} \lt 2$
.
When
$\left| {{e_1}} \right| \lt b$
, take the sliding surface
${s_{11}}$
; when
$\left| {{e_1}} \right| \geqslant b$
, take the sliding surface
${s_{12}}$
, where
$0 \lt b \lt 1$
.
From Equation (26), it can be inferred that

By incorporating Equations (17) and (25) into Equation (28), we obtain

When the system adheres to the sliding surface, the equivalent control law can be derived as follows:

Simultaneously, the subsequent switching control law is

where
${k_1} \gt 0$
,
${\eta _1} \gt 0$
.
Hence, when
$\left| {{e_1}} \right| \lt b$
, the overall control law is

From Equation (27), it can be inferred that

By incorporating Equations (17) and (25) into Equation (33), we obtain

When the system adheres to the sliding surface, the equivalent control law can be derived as follows:

Hence, when
$\left| {{e_1}} \right| \geqslant b$
, the overall control law is

It is worth noting that the discontinuity of the sign function
$sgn\left( \cdot \right)$
in the aforementioned control law can give rise to chattering, which can disrupt actuator performance and excite unmodeled high-frequency dynamic. To mitigate this phenomenon, this paper employs the hyperbolic tangent function
$tanh\left( \cdot \right)$
instead of the sign function [Reference Aghababa and Akbari28]. Specifically,
$sgn\left( {{s_{11}}} \right)$
is substituted with
${\rm{tanh}}\left( {{s_{11}}/\sigma } \right)$
, and
$sgn\left( {{s_{12}}} \right)$
is substituted with
${\rm{tanh}}\left( {{s_{12}}/\sigma } \right)$
, where
$\sigma $
is a very small positive number.
In summary, when
$\left| {{e_1}} \right| \lt b$
, the composite sliding mode control law based on the RESO is

When
$\left| {{e_1}} \right| \geqslant b$
, the composite sliding mode control law based on the RESO is

4.0 Stability analysis
4.1 Observer stability analysis
In this section, we take the pitch channel as an example to demonstrate that the proposed improved RESO guarantees bounded estimation error, with analogous analysis applied to the remaining two channels.
Theorem 1. For the pitch angle channel represented by Equation (17), the estimation error of the proposed improved RESO is bounded.
Proof. Let
${\lambda _i}\left( {{e_{E2}}} \right) = \frac{{fa{l_i}\left( {{e_{{{\rm{\;}}_{E2}}}},{{\bar a}_{{{\rm{\;}}_i}}}} \right)}}{{{e_{{{\rm{\;}}_{E2}}}}}}$
,
${\dot f_1} = \varpi $
, and define
$e_{E3}=\hat{f}_{1}-f_{1}$
as the estimation error of
${f_1}$
.
${e_E} = {[{e_{E2}},{e_{E3}}]^T}$
represents the state error estimation vector. By employing Equation (21), we can deduce the differential equation for
${e_E}$

where
${A_E} = \left[ {\begin{array}{l}{ - {{\bar b}_2}{\lambda _2}\left( {{e_{E2}},{{\bar a}_2}} \right)} 1\\[5pt] { - {{\bar b}_7}{\lambda _7}\left( {{e_{E2}},{{\bar a}_7}} \right)} 0\end{array}} \right]$
,
${B_E} = \left[ {\begin{array}{l}0\\[5pt] 1\end{array}} \right]$
Given that
${\bar b_2} \gt 0$
,
${\bar b_7} \gt 0$
,
${\lambda _2}\left( {{e_{{{\rm{\;}}_{E2}}}},{{\bar a}_{{{\rm{\;}}_2}}}} \right) \gt 0$
,
${\lambda _7}\left( {{e_{{{\rm{\;}}_{E2}}}},{{\bar a}_{{{\rm{\;}}_7}}}} \right) \gt 0$
,
${A_E}$
can be identified as a Hurwitz matrix. Based on the Hurwitz stability theory [Reference Yao and Deng29], it can be inferred that Equation (39) is stable, and
${e_E}$
is bounded. Consequently, the estimation error of the proposed improved RESO is bounded.
4.2 Controller stability analysis
In this section, we illustrate the convergence of the tracking error to zero within a finite time and demonstrate that the actual state can track the desired value within a finite time using the pitch channel as an example.
Based on the stability analysis of the observer, it is known that the estimation error of the proposed improved RESO is bounded. Without loss of generality, assume
${\left| {{f_1} - {{\hat f}_1}} \right|_{{\rm{max}}}} \le {L_1}$
,
${L_1} \lt {\eta _1}$
.
Theorem 2. For system 16, taking the sliding surface 26 and using the control law 37, the attitude tracking error converges to zero within a finite time.
Proof. When
$\left| {{e_1}} \right| \lt b$
, we select the Lyapunov function as

Differentiating Equation (40) yields

By substituting Equation (37) into Equation (41), we obtain

Since
${\left| {{f_1} - {{\hat f}_1}} \right|_{{\rm{max}}}} \le {L_1}$
,
${L_1} \lt {\eta _1}$
, according to Equation (42), we have

Let
${\beta _1}\frac{{{m_1}}}{{{n_1}}}si{g^{\frac{{{m_1}}}{{{n_1}}} - 1}}\left( {{{\dot e}_1}} \right) = \varepsilon $
, since
$1 \lt \frac{{{m_1}}}{{{n_1}}} \lt 2$
, then
$si{g^{\frac{{{m_1}}}{{{n_1}}} - 1}}\left( {{{\dot e}_1}} \right) \gt 0$
, and thus
$\varepsilon \gt 0$
.
From Equation (43), we obtain

Let
$2\varepsilon {k_1} = {\alpha _3}$
,
$\sqrt 2 \varepsilon \left( {{\eta _1} - {L_1}} \right) = {\alpha _4}$
, we have

According to Lemma 1, the sliding variable
${s_{11}}$
converges to zero within a finite time, with the convergence time satisfies
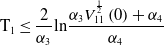
Given that the system state reaches the sliding surface
${s_{11}}$
at
$t = {t_1}$
, where
${t_1} \le {T_1}$
, it ensures that when

From Equation (47), we obtain

Choosing the Lyapunov function as

Differentiating Equation (49) yields

By substituting Equation (48) into Equation (50), we obtain

From Equation 51, we obtain
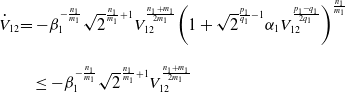
Let
$\beta _1^{ - \frac{{{n_1}}}{{{m_1}}}}{\sqrt 2 ^{\frac{{{n_1}}}{{{m_1}}} + 1}} = {\alpha _5}$
, we have
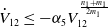
According to Lemma 2, the attitude tracking error
${e_1}$
converges to zero within a finite time, with the convergence time satisfies
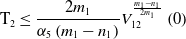
In conclusion, when
$\left| {{e_1}} \right| \lt b$
, the system is finite-time stable, and the overall convergence time satisfies

Theorem 3. For system 16, taking the sliding surface 27 and using the control law 38, the attitude tracking error converges to zero within a finite time.
Proof. When
$\left| {{e_1}} \right| \geqslant b$
, we select the Lyapunov function as

Differentiating Equation (56) yields

By substituting Equation (38) into Equation (57), we obtain

Since
${\left| {{f_1} - {{\hat f}_1}} \right|_{{\rm{max}}}} \le {L_1}$
,
${L_1} \lt {\eta _1}$
, according to Equation (58), we have

From Equation (59), obtain

Let
$2{k_1} = {\alpha _6}$
,
$\sqrt 2 \left( {{\eta _1} - {L_1}} \right) = {\alpha _7}$
, we have

According to Lemma 1, the sliding variable
${s_{12}}$
converges to zero within a finite time, with the convergence time satisfies
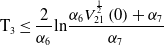
Table 1. Model parameters
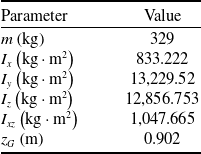
Table 2. External disturbances

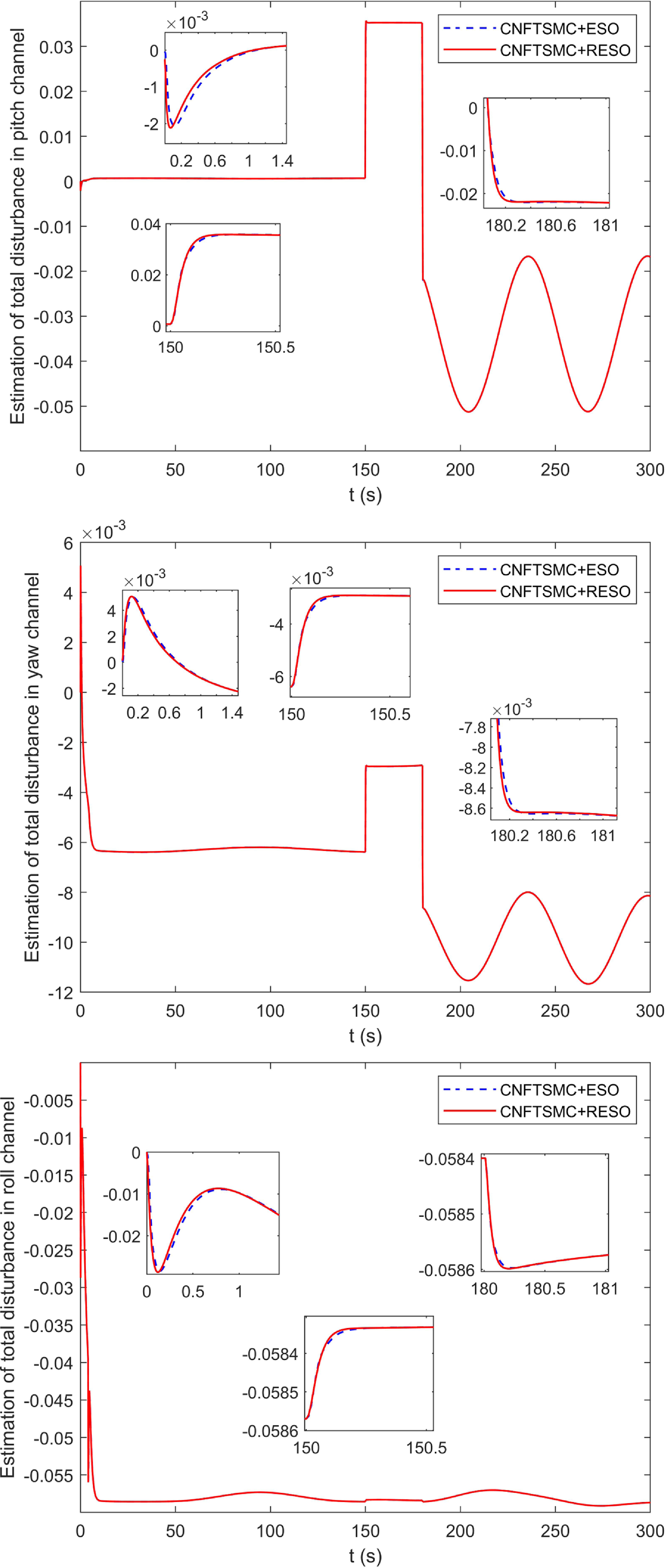
Figure 3. Estimation of total disturbance under two observers.
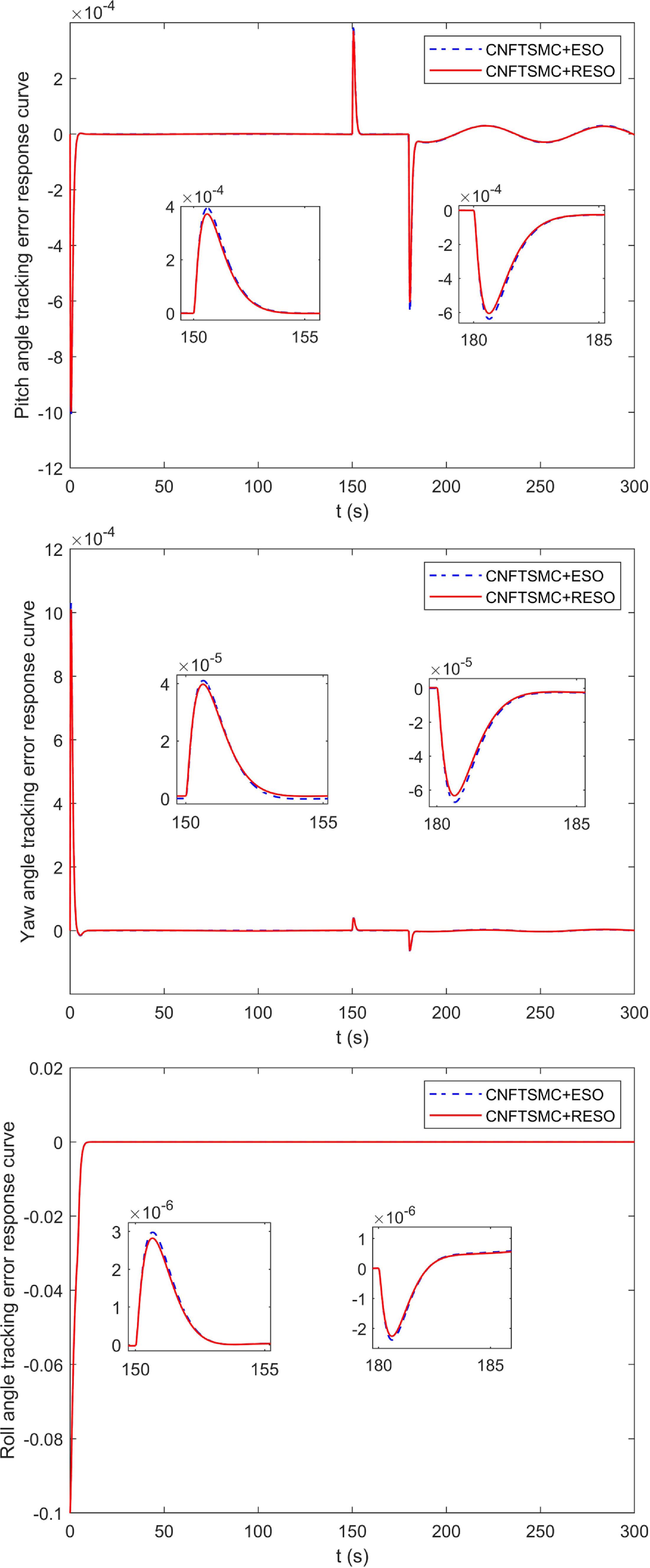
Figure 4. Attitude angle tracking error response curves under two observers.
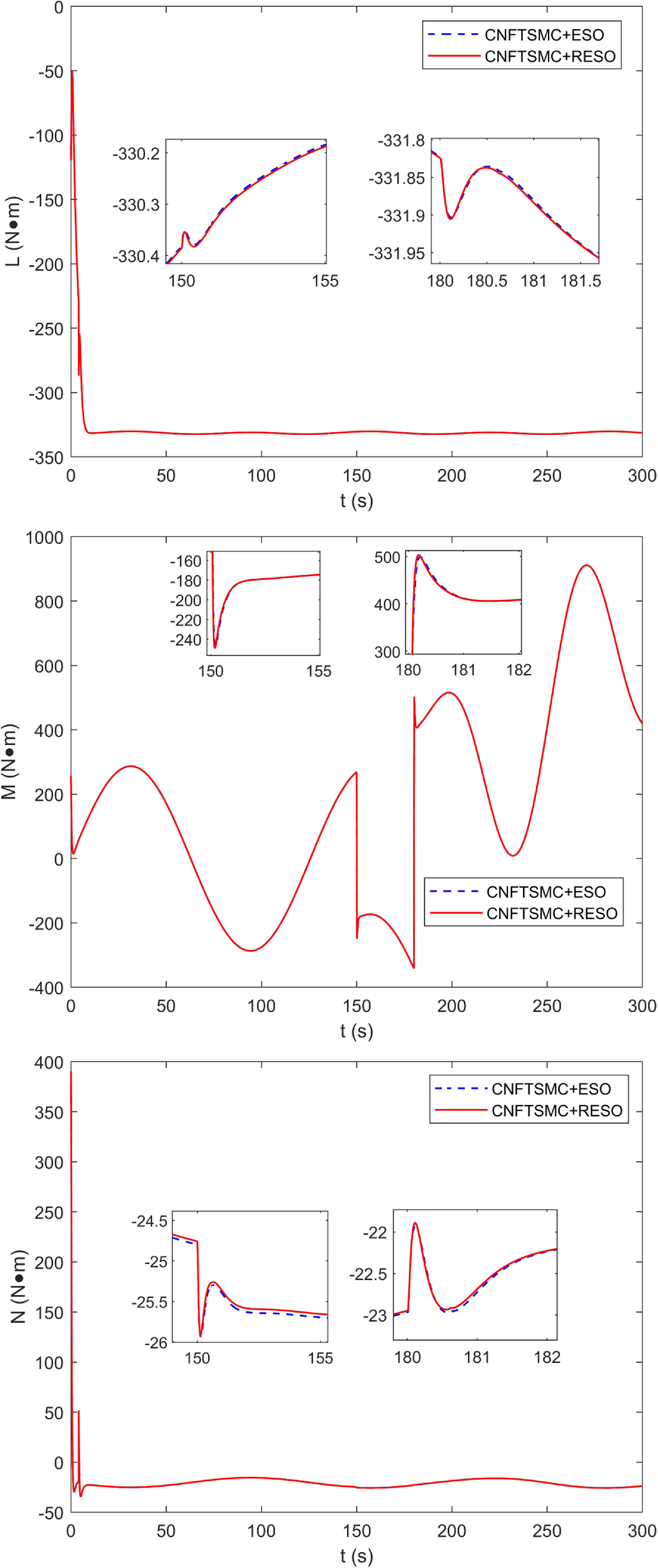
Figure 5. Control moment response curves under two observers.
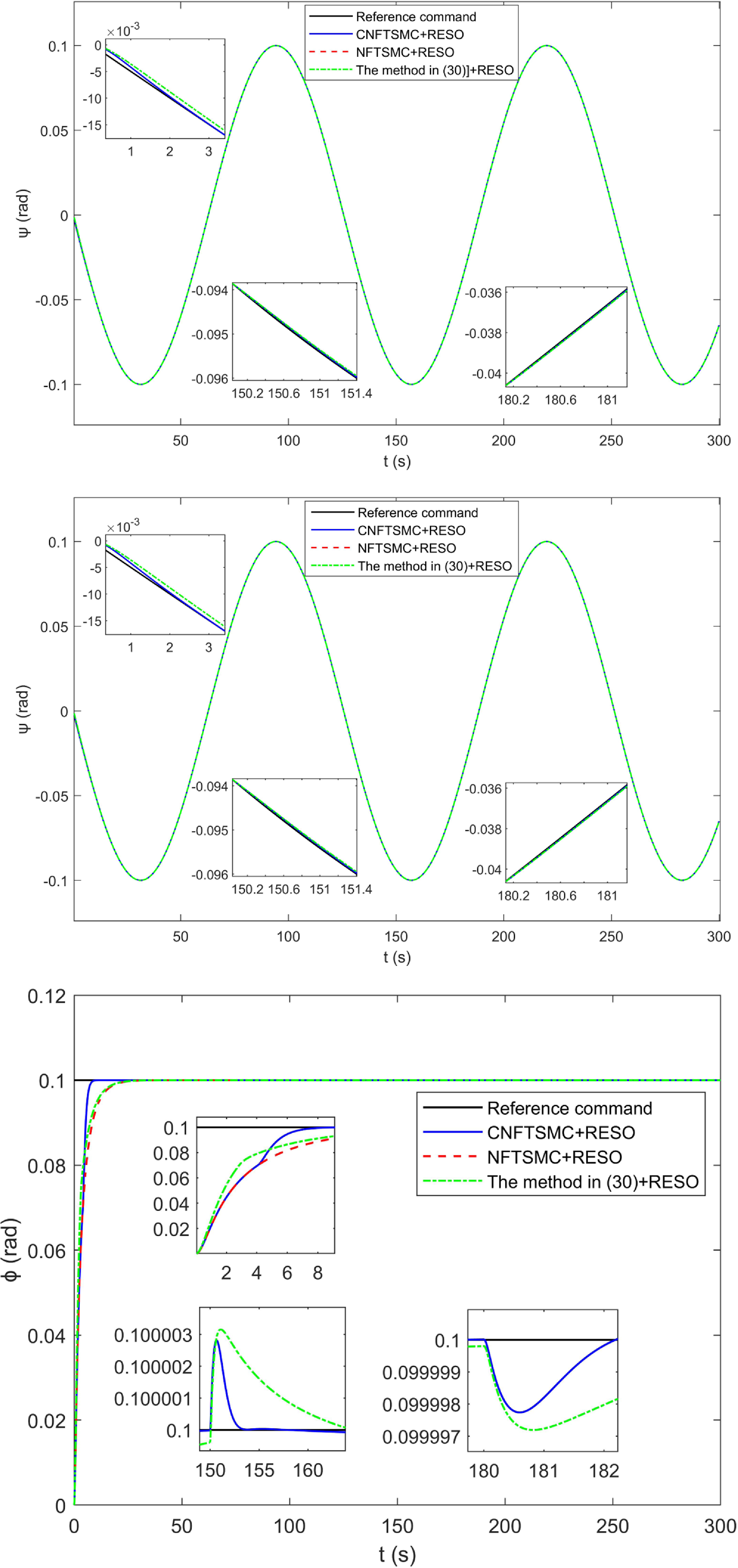
Figure 6. Attitude angle tracking response curves.
In conclusion, when
$\left| {{e_1}} \right| \geqslant b$
, the system is finite-time stable, and the overall convergence time satisfies

5.0 Simulation analysis
5.1 Simulation setup
To validate the effectiveness and superiority of the proposed algorithm, this section conducts two parts of comparative experiments on the decoupled subsystems of pitch, yaw and roll. Firstly, a comparison simulation is carried out between the Composite Non-singular Fast Terminal Sliding Mode Control algorithm with the traditional Nonlinear Extended State Observer (CNFTSMC+ESO) and the Composite Non-singular Fast Terminal Sliding Mode Control algorithm with the Reduced-order Extended State Observer (CNFTSMC+RESO). Secondly, a comparison simulation is conducted among the Composite Non-singular Fast Terminal Sliding Mode Control algorithm with the Reduced-order Extended State Observer (CNFTSMC+RESO), the Non-singular Fast Terminal Sliding Mode Control algorithm with the Reduced-order Extended State Observer (NFTSMC+RESO), and the method in (30) with the Reduced-order Extended State Observer (The method in (30)+RESO). Furthermore, the method in Ref. [Reference Derrouaoui, Bouzid, Belmouhoub and Guiatni30] is identical to the method in Ref. [Reference Derrouaoui, Bouzid, Belmouhoub and Guiatni31]. The experimental airship described in Refs (Reference Wang32, Reference Wang and Shan33) serves as the research object, with the primary model parameters shown in Table 1.
The initial values of the airship attitude system are set as
$\theta \left( 0 \right) = 0$
,
$\psi \left( 0 \right) = 0$
,
$\phi \left( 0 \right) = 0$
,
$p\left( 0 \right) = 0$
,
$q\left( 0 \right) = 0$
,
$r\left( 0 \right) = 0$
. The desired values for pitch, yaw and roll are set as
${\theta _d} = 0.1{\rm{sin}}\left( {0.05t} \right)$
,
${\psi _d} = - 0.1{\rm{sin}}\left( {0.05t} \right)$
,
${\phi _d} = 0.1$
. The model parameter perturbations are set as
${\rm{\Delta }}{I_x} = 0.2{I_x}$
,
${\rm{\Delta }}{I_y} = 0.15{I_y}$
,
${\rm{\Delta }}{I_z} = 0.15{I_z}$
,
${\rm{\Delta }}{I_{xz}} = 0.2{I_{xz}}$
. The external disturbances applied to the system dynamics are specified in Table 2.
Taking the pitch channel as an example, the sliding surface design for the NFTSMC+RESO is given by

The comparative controller design is as follows:


Figure 7. Attitude angle tracking error response curves.
To ensure fairness, the selection of parameters adheres to three principles [Reference Sha, Wang and Liu34]: effective control, uniform variables and moderate control variable values. Taking the pitch channel as an example, the simulation parameters for the remaining two channels are consistent with the pitch channel.
The observer parameter values are as follows:
-
(1) ESO:
${a_1} = 1$ ,
${a_2} = 0.5$ ,
${a_7} = 0.25$ ,
${b_1} = 100$ ,
${b_2} = 1,000$ ,
${b_3} = 6,000$ ,
$\delta = 0.01$ .
-
(2) RESO:
${\bar a_2} = 1$ ,
${\bar a_7} = 0.5$ ,
${\bar a_2} = 100$ ,
${\bar a_7} = 200$ ,
$\delta = 0.01$ .
The simulation parameter values for the controllers are as follows:
-
(1) CNFTSMC:
$b = 0.03$ ,
${\alpha _1} = 0.2$ ,
${\beta _1} = 1.5$ ,
${\alpha _2} = 0.2$ ,
${\beta _2} = 1.5$ ,
${p_1} = 5$ ,
${q_1} = 3$ ,
${m_1} = 57$ ,
${n_1} = 55$ ,
${w_1} = 2$ ,
$\sigma = 1$ ,
${\eta _1} = 0.08$ ,
${k_1} = 2$ .
-
(2) NFTSMC:
${\alpha _2} = 0.2$ ,
${\beta _2} = 1.5$ ,
${w_1} = 2$ ,
$\sigma = 1$ ,
${\eta _1} = 0.08$ ,
${k_1} = 2$ .
Table 3. Maximum absolute error (
${\bf{max}}\left| e \right|$
)

Table 4. Mean absolute error (
$\frac{\sum |e|}{N}$
)
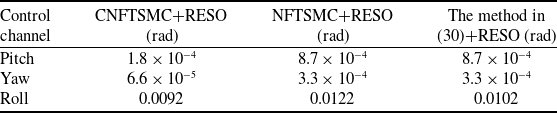
Table 5. Absolute error standard deviation (
$\sqrt{\frac1N\sum(|e|-\frac1N\sum|e|)^2}$
)
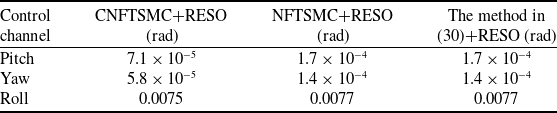
Table 6. Integral absolute error (
$\int_0^t|e|dt$
)
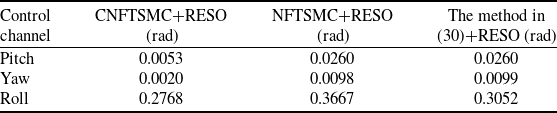
5.2 Simulation results analysis
Figure 3 depicts the estimation response of the two observers to the total disturbances under the CNFTSMC. It is evident that the proposed improved RESO exhibits a more rapid and accurate estimation of the disturbances, thereby mitigating the phase lag induced by the observer. In addition, the tracking error and control input of the two comparative observers are illustrated in Figs. 4 and 5. It is apparent that, for the three channels with uniform control input, the tracking error based on the improved RESO is inferior to that based on the ESO, verifying the effectiveness of the proposed observer.
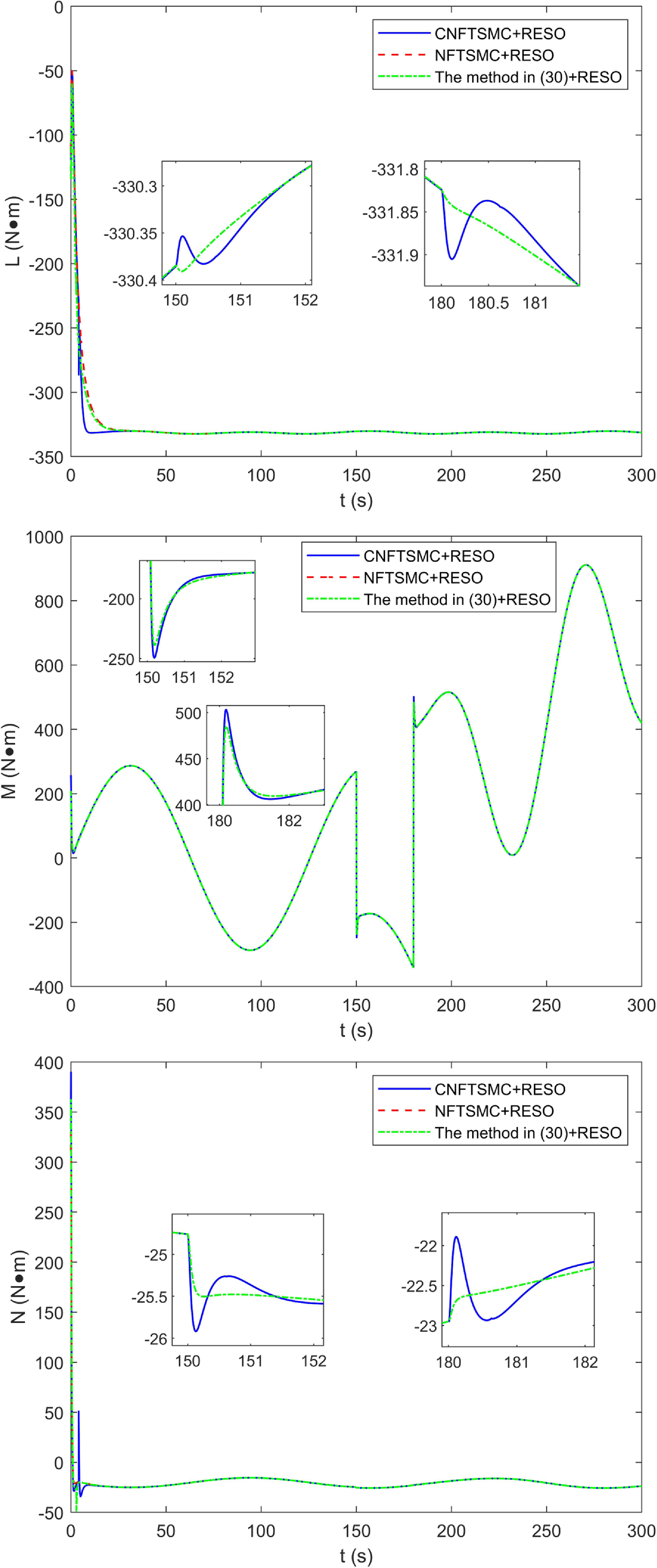
Figure 8. Control moment response curves.
Figure 6 presents the attitude angle tracking response curves for three control methods. It is apparent that the proposed CNFTSMC+RESO, NFTSMC+RESO, and the method in (30)+RESO adeptly compensate for the disturbances and parameter perturbations by utilising estimation information. Consequently, they achieve precise tracking of the pitch, yaw and roll angle commands in a multiple-disturbances environment.
Figure 7 illustrates the attitude angle tracking error response curves for three control methods. We conduct a comparative analysis with regard to their convergence speed and disturbance rejection capability. It is evident that prior to 150s, in the absence of external disturbances, CNFTSMC+RESO demonstrates a faster convergence speed in the pitch and yaw channels compared to NFTSMC+RESO and the method in (30)+RESO. In the roll channel, when the tracking error is greater than or equal to 0.03, CNFTSMC+RESO and NFTSMC+RESO exhibit the same convergence speed, while the method in (30)+RESO achieves a faster convergence speed compared to the other two methods. When the tracking error falls below 0.03, the convergence speed of CNFTSMC+RESO surpasses that of NFTSMC+RESO and the method in (30)+RESO.
It is noteworthy that the method in (30)+RESO exhibits the advantage of a faster convergence speed when the tracking error is larger, and additionally, this study also evaluates the disturbance rejection capability. Between 150s and 180s, when exposed to constant disturbances, or at 180s when confronted with time-varying disturbances, the attitude angle tracking response under CNFTSMC+RESO exhibits a more rapid recovery, resulting in a shorter adjustment time compared to the other two control methods.
Furthermore, in order to comprehensively compare the performance of the control methods, this section introduces performance indicators for tracking errors, which include the maximum absolute error, average absolute error, absolute error standard deviation and integral absolute error [Reference Zhang, Guo, Gong, Zhu and Tian35]. The control performance indicators for attitude tracking errors under the three control methods are shown in Tables 3, 4, 5 and 6. It can be observed that the proposed CNFTSMC+RESO method attains superior control performance indicators in terms of tracking errors, validating the effectiveness and superiority of the proposed control algorithm.
In conclusion, the CNFTSMC+RESO method demonstrates smaller maximum absolute error, average absolute error, absolute error standard deviation and integral absolute error in comparison to NFTSMC+RESO and the method in (30)+RESO. Additionally, it showcases faster convergence speed and stronger capability for suppressing disturbances.
Figure 8 displays the control torque response curves for the three control methods. By utilising hyperbolic tangent function instead of the sign function in the design of the sliding mode controller, all three control algorithms ensure the continuity of the control quantity. From the figure, it can be observed that the control quantities of the three control algorithms are of equivalent magnitude, indicating that they provide comparable control energy required to achieve the control objectives.
6.0 Conclusion
In summary, this paper addresses the attitude control problem of stratospheric airship in the presence of multiple disturbances. A CNFTSMC scheme based on RESO has been proposed to effectively mitigate the impact of parameter perturbations and external disturbances, thereby enhancing control performance. The effectiveness and superiority of the proposed control method have been substantiated through simulation experiments. The simulation results demonstrate that the proposed control scheme ensures precise tracking of the airship’s attitude, significantly improves the response speed, and enhances the anti-interference ability of the attitude tracking.
Furthermore, the control of airship attitude necessitates consideration of the actuators configuration. In future research, we intend to conduct a comprehensive investigation into the manipulation of the airship’s actuators, such as propeller vectoring and control of the airship’s aerodynamic control surfaces.
Acknowledgements
This work was funded by Natural Science Foundation of China (No. U23A20336, No. 52227811 and No. 61733017).