THE NEXT STEP FOR AI
Publicly accessible generative AI represents the next advance in the AI subfield of machine learning (ML), which itself has already been impacting archaeology (Bickler Reference Bickler2021; Eberl et al. Reference Eberl, Bell, Spencer-Smith, Raj, Sarubbi, Johnson, Rieth, Chaudhry, Aguila and McBride2023). Midjourney, DALL-E (2), and Stable Diffusion are among the most prominent products that create images from text prompts, whereas ChatGPT and Bard are two well-known chatbots that generate text based on LLMs. The DALL-E platform was released by the company OpenAI in early 2021, with a significant update called DALL-E 2 that was made available over the summer of 2022. Midjourney, developed by a small San Francisco company, also became available in mid-2022 and requires a subscription. Stable Diffusion, with links to a German university, differs from the others in that its full computer code is available for anyone to download and use, under a license that tries to enforce only responsible uses of that code. ChatGPT, the release of which in late 2022 brought significant public attention to the generative AI field, is made by OpenAI, with support from and integration with Microsoft. Google responded by releasing its Bard product in early 2023, given concerns that AI chatbots might present competition to their Internet search business by answering user questions in a conversational style.
These new generative AI products are made possible by recent advances in hardware that enhance compute power and by the extremely large datasets that have developed on the Internet over the past two decades. The latest ChatGPT product is based on the LLM called GPT-4, a model of human speech as a mathematical abstraction. The AI uses probabilities to understand textual context and to predict the relationships among words in a human prompt and its own response. The abbreviation GPT stands for Generative Pretrained Transformer. It is called “generative” because it generates new content, and “pretrained” because of the very large dataset of information it is trained on before it sees the human prompts. It can continue to learn on additional data and on the basis of its interactions with humans over time. A “transformer” is a specific type of deep-learning neural network design that has developed recently and that seems to work very well for language learning (Vaswani et al. Reference Vaswani, Shazeer, Parmar, Uszkoreit, Jones, Gomez, Kaiser, Polosukhin, Guyon, Von Luxburg, Bengio, Wallach, Fergus, Vishwanathan and Garnett2017). LLMs learn on datasets that include documents containing tens of trillions of individual words, and the models underpinning text-to-image AI learn on hundreds of millions of images with captions. Therefore, generative AI is “simply remixing and recombining existing writing that's relevant to the prompt” (Newport Reference Newport2023). The models learn to predict the relationships among words or between words and images. The generative AI can then guess what the most likely combination of words or the most likely image configuration should be in the response.
What is important about this recent wave of AI is that it produces convincing results: either text that sounds as though it is written by an actual human, or images that are aesthetically interesting or realistic. This opens significant possibilities for automatically producing things that only humans could produce before. Consequently, ChatGPT was immediately tested on assisting with writing news articles (Acres and ChatGPT Reference Acres2022), and advertisers have turned to the text-to-image generators for their content (Ostler et al. Reference Ostler, Munoz and Krithivasan2023). Do these tools herald a new era of increased capitalistic productivity? Will it come at the expense of jobs for human creators? AI pictures have already been entered into art contests, and have won, even if some consider them as derivatives of the original human-created art samples in their datasets (Ivanova Reference Ivanova2023). While technology specialists have begun warning about the potential for AI to threaten human society, philosophers are renewing efforts to consider if this new AI can truly reason or understand its own conversations (Floridi Reference Floridi2023). Meanwhile, we the users are all testing these systems for the companies, providing our private data and public reactions so that they can improve the products. OpenAI even ran a contest for the first month of public use, asking users to provide direct feedback on their experiences (OpenAI 2022).
In addition to the issue of their potential plagiarism from the training datasets, perhaps the largest impediment to the usefulness of these generative AI platforms is their lack of factual accuracy. We have all by now experienced ChatGPT making up completely fictional statements but presenting them confidently as the truth, a phenomenon called “hallucination” (Ji et al. Reference Ji, Rita Frieske, Yu, Su, Xu, Ishii, Bang, Madotto and Fung2023). As OpenAI itself states, “Fixing this issue is challenging, as . . . during . . . training, there's currently no source of truth” (OpenAI 2022). Furthermore, given how much time it takes to train the computer, the most recent version—ChatGPT-4—contains information based mainly on a dataset that is years old, last updated in September 2021 (OpenAI 2023:10).
Prior AI chatbots had received bad reputations by parroting the hateful speech of the Internet, and although safeguards seem stronger now, users are still finding ways to confound these protections (Taylor Reference Taylor2023). These tools will also reflect the human biases of the underlying datasets and, consequently, perpetuate societal stereotypes in their responses. OpenAI hires human trainers to reinforce the learning of ChatGPT to avoid offensive content, but this is done in what has been recognized as troubling circumstances (Perrigo Reference Perrigo2023). The workers, in low-wage countries, are exposed to disturbing content throughout the day and paid relatively small wages. On the hardware side, we must remain mindful of the quantity of energy required to run generative AI. This has negative implications for the environment and sustainability that we hope can be at least partially mitigated with renewable energy sources such as solar photovoltaic panels plus batteries. In addition, only the largest companies can afford the compute power needed to train these AI products, further exacerbating the inequalities of technological control and access.
POTENTIAL IMPACTS FOR ARCHAEOLOGY AND ARCHAEOLOGISTS
The recent Hollywood writers’ strike highlights the concern that these new AI tools are raising for human livelihoods. When discussing “the rise of the useless class,” the historian Harari (Reference Harari2017) suggested that the “likelihood that computer algorithms will displace archaeologists by 2033 is only 0.7%, because their job requires highly sophisticated types of pattern recognition and doesn't produce huge profits” worth the investment. Perhaps our jobs are not immediately threatened, but it is still worth considering the potential impacts—both positive and negative—on our field.
Research
Initially, I was curious if text-based generative AI could support actual archaeological research. Therefore, I asked specific archaeological questions to various chatbots periodically over the course of several months. In April 2023, I entered the following prompt to ChatGPT: “What was the Late Bronze Age like in the South Caucasus?” As you can see from the response (Figure 1), the date range and generally vague geographical information were correct, but it erroneously emphasized the Iron Age Urartian kingdom (ca. 900–600 BC). Perhaps this relates to the Urartians leaving the very first historical written record in this region. When I asked Google's Bard chatbot a similar question two months later, it erroneously placed the Early Bronze Age Kura-Araxes culture together with two Middle Bronze Age cultures into the Late Bronze Age. Fascinatingly, when I asked the same question again to ChatGPT, its answer was now incorrect in a way very similar to Bard's (Figure 2). This would imply that they were incrementally updated recently on a similar training dataset.
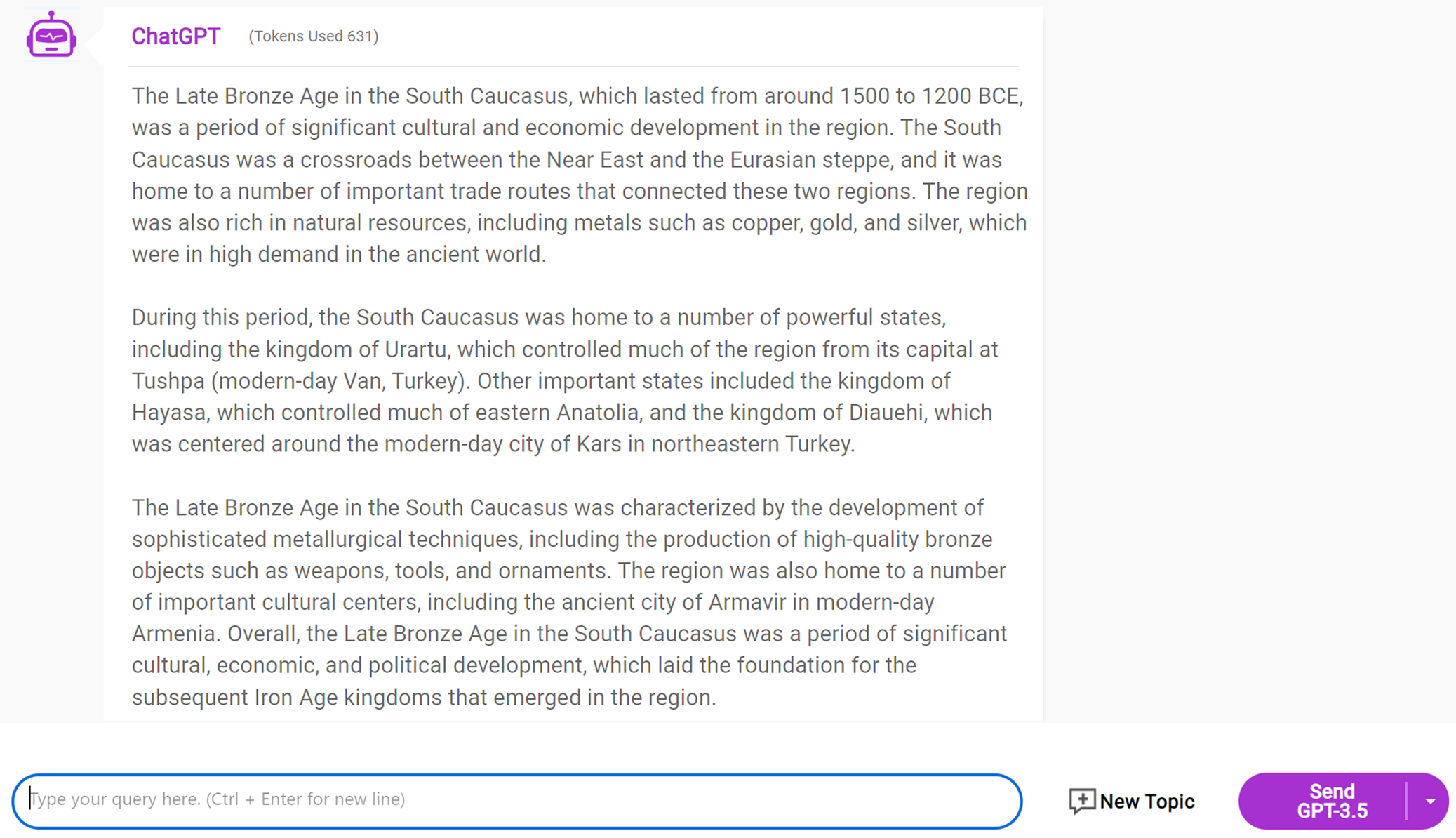
Figure 1. Initial answer from ChatGPT 3.5 in response to the prompt: “What was the Late Bronze Age like in the South Caucasus?” April 17, 2023.

Figure 2. Updated answer for the same question, two months later. The answer was similar whether I used ChatGPT version 4 or 3.5 at this time.
This problem with basic facts suggests that it will be a long time before the chatbots can directly support our archaeological research. The AI programs will need to have a better grasp of current archaeological knowledge and theory before they can synthesize or build new ideas. Perhaps it will be possible to target the training of these programs on the intellectual output of archaeologists specifically, such as all peer-reviewed publications in the field. Would they then be able to combine archaeological evidence and theory? Perhaps they would be able to identify inconsistencies in the research, but would they be capable of creating new valid knowledge?
Agapiou and Lysandrou (Reference Agapiou and Lysandrou2023) attempted to use ChatGPT to summarize the latest developments in remote sensing for archaeology. They asked a series of basic questions about topics such as drones, vegetative cover, data fusion, and buried remains. After reading the results, their conclusion was that “ChatGPT provided apparently satisfactory replies, lacking however any exhaustive analysis compared to traditional literature review methods” (Agapiou and Lysandrou Reference Agapiou and Lysandrou2023:4083). Based on its general answers, the chatbot seems to provide a relatively correct, if basic, overview of how remote sensing is used in archaeology today. It also gives some interesting details about popular drone models and analytical software. However, the answers lack any bibliographic references for this information, preventing further detailed review of the literature. ChatGPT seems to do better with such highly technical topics, but it only compiles the information, without any critique of the sources. Perhaps this tool can be used to support literature reviews, but it has a long way to go before it can do the detailed synthesis of existing articles that would save us time. Yurtsever (Reference Yurtsever2023:37–38) asked ChatGPT to summarize his article on an ancient gymnasium in southern Turkey and found the technical information from the summary to be correct.
Writing
Even if pure research may be far in the future, perhaps the basic writing abilities of the LLM-based chatbots could support our work. After all, some academic articles in the sciences have already begun listing ChatGPT as a coauthor (Stokel-Walker Reference Stokel-Walker2023). This, again, raises the question of whether the recombination of text produced by these AI programs could be considered plagiarism. Furthermore, given their lack of factual knowledge, these AI text generators often invent fake article citations. Will academic archaeological journals such as Advances in Archaeological Practice move to ban the use of programs such as ChatGPT in the writing of submissions?
As someone who works internationally, I can at least see the potential usefulness of ChatGPT to polish the English of nonnative writers or to help with article translations. The written output of these chatbots tends to be quite general in nature, so it may not yet find a place in detailed academic publications. Perhaps the chatbots could write abstracts for articles if we fed them the entire text. Outside of academic publication, could generative AI help write field reports that may contain a lot of boilerplate language? Or even grant applications? On the other hand, many organizations that have been around for a while already have such filler language that they reuse and periodically update. At least it might be useful for us archaeologists to more quickly craft email messages.
Illustration
Since we all spend a lot of time creating illustrations for publications and reports based on the raw data we collect during fieldwork, automatic image generation sounds promising. For example, perhaps we could ask the computer to create a line drawing of the architecture we uncovered at our sites based on our photographs, notes, and 3D models. My simple request to DALL-E 2 to create a drawing (Figure 3) resulted in something that might look like a plan if viewed from far away but that is actually gibberish. Consequently, here again, factual knowledge will be crucial, given that the generative AI will need to start from these known recorded data (Klehm Reference Klehm2023; Petrosyan et al. Reference Petrosyan, Azizbekyan, Gasparyan, Dan, Bobokhyan and Amiryan2021). The recent increase in data collected natively in 3D formats by archaeologists also opens the potential for generating new 3D visual products (Liang Reference Liang2021). Given that archaeological reconstruction is already a process of interpreting data from multiple diverse sources, in theory it might be a challenge well suited to generative AI, which thrives on recognizing and repeating patterns. If AI could create better 3D model reconstructions of ancient sites and artifacts, it could also support our teaching and public outreach with 3D and extended reality (XR) technologies (Cobb and Nieminen Reference Cobb and Nieminen2023). Yet, similar to text, the visual products derived from learning on existing pictures and 3D models from across the Internet might be considered plagiarism and therefore not publishable. If we ask for any sort of depiction of humans, we might also run into the problem of stereotyping based on the original datasets.
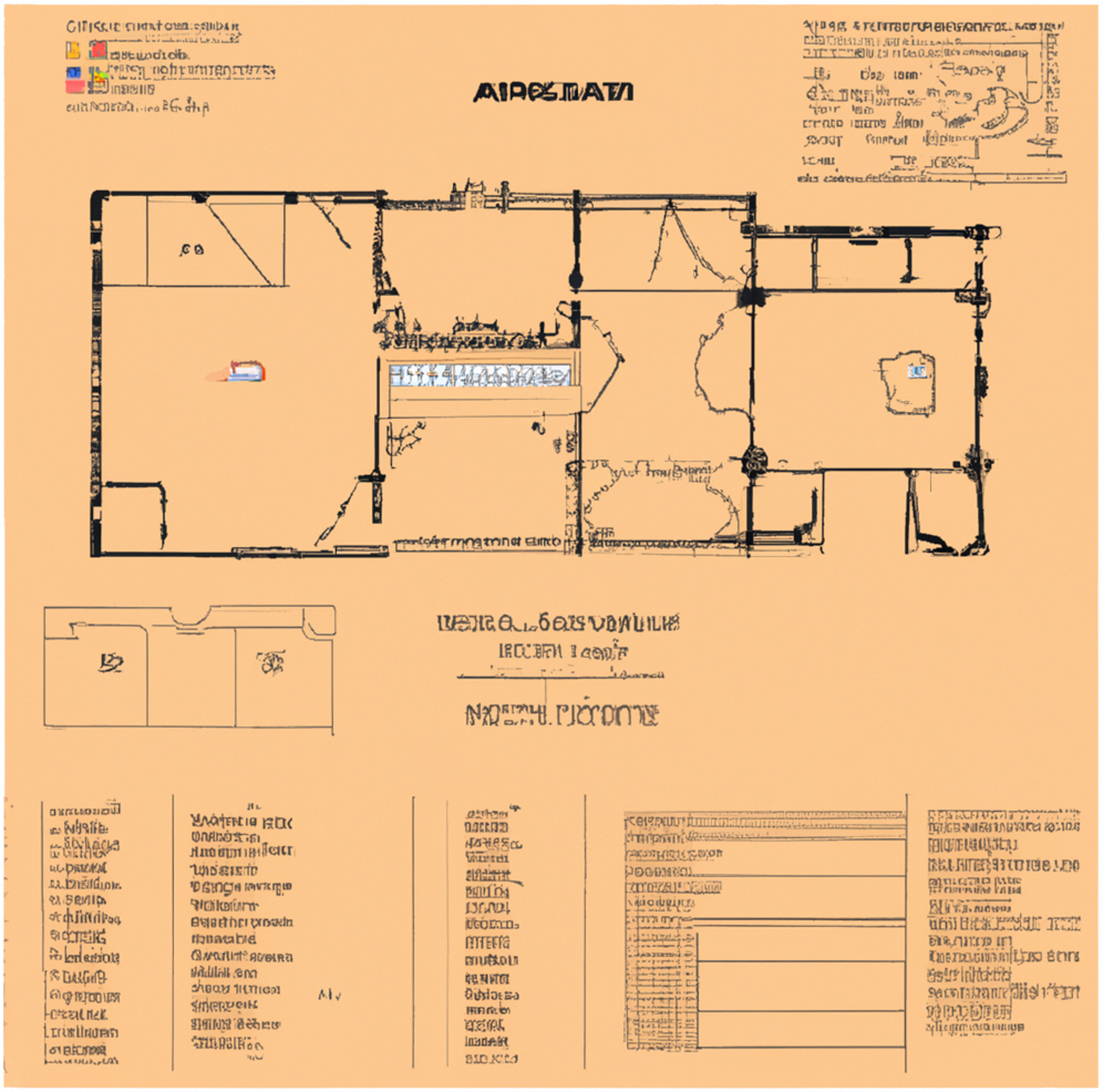
Figure 3. DALL-E 2 generated image from the prompt: “Architectural plan of the archaeological ruins of a building in ancient Armenia,” June 2023.
Teaching
Perhaps the most immediate impact for generative AI will be in our teaching. Some universities have placed an outright ban on the use of these tools by students for completing written assignments. Indeed, the text generated by ChatGPT presents the kind of introductory-level synthesis of topics that may resemble undergraduate papers. The writing may contain general, vague statements and include some factual errors. Tools such as Turnitin have been trying to develop AI-writing detection capabilities for student submissions, but even this company admits that the constant evolution of the technology will make it challenging to adapt (Caren Reference Caren2023). One teaching experiment in North Carolina asked students to “grade” papers produced by ChatGPT, allowing the students themselves to recognize the problems of AI (Howell Reference Howell2023). Some educators are advocating for prioritizing assessment types that cannot be assisted by generative AI, such as handwritten or oral exams. The archaeological field school is one form of education for which student work is unlikely to be replaced anytime soon by AI (Cobb et al. Reference Cobb, Cobb and Azizbekyan2022). Yet our students still need to learn how to write, just as they still need to learn math, even if most calculation is now done by computers.
Ultimately, our students should develop some level of digital literacy with these new tools (Kansa and Kansa Reference Kansa and Kansa2021). The potential new job of a “prompt engineer” has been discussed, denoting someone whose skill is to write the perfect questions to get the best answers from the chatbots. I wonder, though, if this job category will actually materialize, given how easy it already is to chat with current generative AI. Students should at least gain some general understanding of how AI works and how to make use of it in their careers.
On the flip side, generative AI also holds the potential to assist us as teachers. Already, instructors have automatically created or updated course syllabi (Watkins Reference Watkins2022). ChatGPT can grade student papers, including providing students with the significant detailed feedback that would be most useful but would normally take teachers too much time to write. Ultimately, an AI chatbot might hold the potential for supporting individualized learning and giving personal feedback to help each student (Wong Reference Wong2023). Intriguingly, the learning analytics data collected through the interactions between the students and the computer will feed into further refinement of the systems. In this way, the AI can “learn” how better to help students through observing how students complete their assignments.
For our public outreach and education, generative AI might be used for creating content for broad consumption. For example, chatbots might be able to answer basic archaeological questions at museums (Trichopoulos et al. Reference Trichopoulos, Konstantakis, George Caridakis, Katifori and Koukouli2023). Archaeologists might also be able to use voice or video generators to simplify the creation of introductory materials such as audio tours of sites or short documentaries about the human past (Khan Reference Khan2022). Given that we coauthor the content with the AI, we can verify that the information is factually correct before we share it. I also anticipate that generative AI will soon find uses creating content for video games about archaeology (Landa and Thompson Reference Landa and Thompson2023).
MOVING FORWARD
Perhaps we have already hit a plateau in the technological capabilities of generative AI after the recent spurt of advancements. After all, other complex technologies, such as self-driving cars, have long been promised but face many remaining challenges to widescale adoption outside of their current limited use cases. Generative AI may be able to predict what words should go together or what an image should look like, but these skills may only address a small range of practical needs. In particular, I see the lack of factual understanding by the generative AI platforms as currently preventing their widespread usefulness in archaeology, but this could be a solvable problem. The AI should also learn to work with a wider variety of data types: not just texts and images but also the 3D models and structured data that are central to archaeological data collection.
Personally, the only real use I have found so far for ChatGPT is to assist with my computer programming work, and anecdotally, I have heard the same reaction from several colleagues. It can also debug existing code that one gives to it. Perhaps it is not surprising that a tool built by computer programmers would initially be most helpful to programmers. Computer coding is time consuming and may not be the main focus of our work, but it can be immensely useful for curating and analyzing data. The AI can remember the specific words and structure of the coding languages much better than I can, so I have moved from finding example code to copy from an Internet search to just asking ChatGPT to write my programs. It is extremely convenient to be able to ask for the code using natural language. The initial results are never perfect, so I still need to test and update the code, but the work is much faster overall. Perhaps, in the future, we will observe an analogy between programmers touching up their code scripts and humanities scholars touching up their generated manuscripts.
Competing Interests
Peter J. Cobb has been the digital reviews editor for Advances in Archaeological Practice since 2021. No generative AI chatbots were used to contribute text to this article.