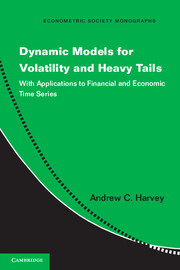
Book contents
- Frontmatter
- Contents
- Preface
- Acronyms and Abbreviations
- 1 Introduction
- 2 Statistical Distributions and Asymptotic Theory
- 3 Location
- 4 Scale
- 5 Location/Scale Models for Non-negative Variables
- 6 Dynamic Kernel Density Estimation and Time-Varying Quantiles
- 7 Multivariate Models, Correlation and Association
- 8 Conclusions and Further Directions
- A Derivation of Formulae in the Information Matrix
- B Autocorrelation Functions
- C GED Information Matrix
- D The Order of GARCH Models
- E Computer Programs
- Bibliography
- Author Index
- Subject Index
- Other titles in the series
4 - Scale
Published online by Cambridge University Press: 05 May 2013
- Frontmatter
- Contents
- Preface
- Acronyms and Abbreviations
- 1 Introduction
- 2 Statistical Distributions and Asymptotic Theory
- 3 Location
- 4 Scale
- 5 Location/Scale Models for Non-negative Variables
- 6 Dynamic Kernel Density Estimation and Time-Varying Quantiles
- 7 Multivariate Models, Correlation and Association
- 8 Conclusions and Further Directions
- A Derivation of Formulae in the Information Matrix
- B Autocorrelation Functions
- C GED Information Matrix
- D The Order of GARCH Models
- E Computer Programs
- Bibliography
- Author Index
- Subject Index
- Other titles in the series
Summary
An established feature of asset returns is that they exhibit volatility clustering. Another stylized fact about returns is that their distributions typically have heavy tails. Although the Gaussian GARCH structure induces excess kurtosis in the returns, it is not usually enough to match the data. As a result, it is now customary to assume that returns have a conditional Student's tv distribution. The GARCH-t model, which was originally proposed by Bollerslev (1987), is widely used in empirical work and as a benchmark for other models.
The t distribution in GARCH-t is employed in the predictive distribution of returns and used as the basis for maximum likelihood estimation of the parameters, but it is not acknowledged in the design of the equation for the conditional variance. The specification of the conditional variance as a linear combination of squared observations is taken for granted, but the consequences are that it responds too much to extreme observations, and the effect is slow to dissipate. These features of GARCH are well-known, and the consequences for testing and forecasting have been explored in a number of papers; see, for example, Franses, van Dijk and Lucas (2004). Other researchers, such as Sakata and White (1998) and Muler and Yohai (2008), have been prompted to develop procedures for robustification; see also Gregory and Reeves (2010).
- Type
- Chapter
- Information
- Dynamic Models for Volatility and Heavy TailsWith Applications to Financial and Economic Time Series, pp. 97 - 148Publisher: Cambridge University PressPrint publication year: 2013