Book contents
- Assessing Measurement Invariance for Applied Research
- Educational and Psychological Testing in a Global Context
- Assessing Measurement Invariance for Applied Research
- Copyright page
- Dedication
- Contents
- Figures
- Tables
- Preface
- Chapter 1 Introduction
- Chapter 2 Observed-Score Methods
- Chapter 3 Item Response Theory
- Chapter 4 Methods Based on Item Response Theory
- Chapter 5 Confirmatory Factor Analysis
- Chapter 6 Methods Based on Confirmatory Factor Analysis
- Appendix A A Brief R Tutorial
- References
- Author Index
- Subject Index
- References
References
Published online by Cambridge University Press: 13 May 2021
- Assessing Measurement Invariance for Applied Research
- Educational and Psychological Testing in a Global Context
- Assessing Measurement Invariance for Applied Research
- Copyright page
- Dedication
- Contents
- Figures
- Tables
- Preface
- Chapter 1 Introduction
- Chapter 2 Observed-Score Methods
- Chapter 3 Item Response Theory
- Chapter 4 Methods Based on Item Response Theory
- Chapter 5 Confirmatory Factor Analysis
- Chapter 6 Methods Based on Confirmatory Factor Analysis
- Appendix A A Brief R Tutorial
- References
- Author Index
- Subject Index
- References
Summary
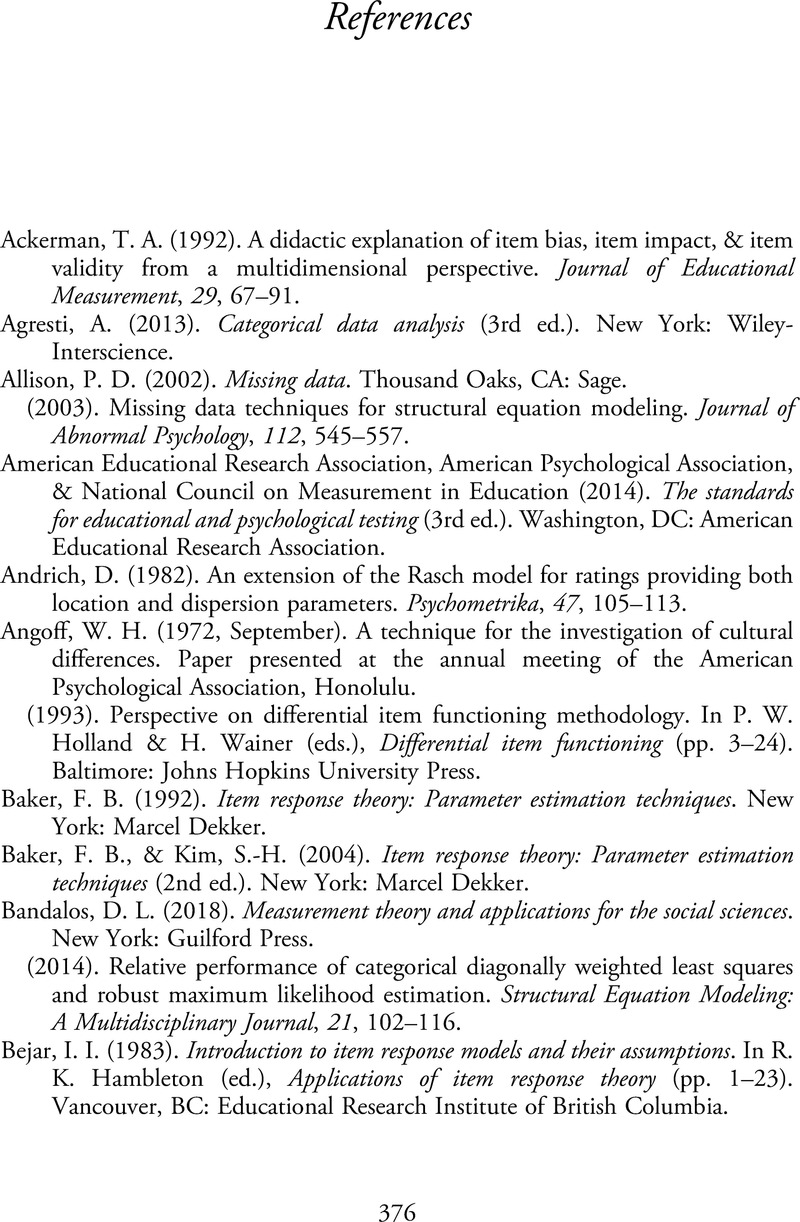
- Type
- Chapter
- Information
- Assessing Measurement Invariance for Applied Research , pp. 376 - 387Publisher: Cambridge University PressPrint publication year: 2021